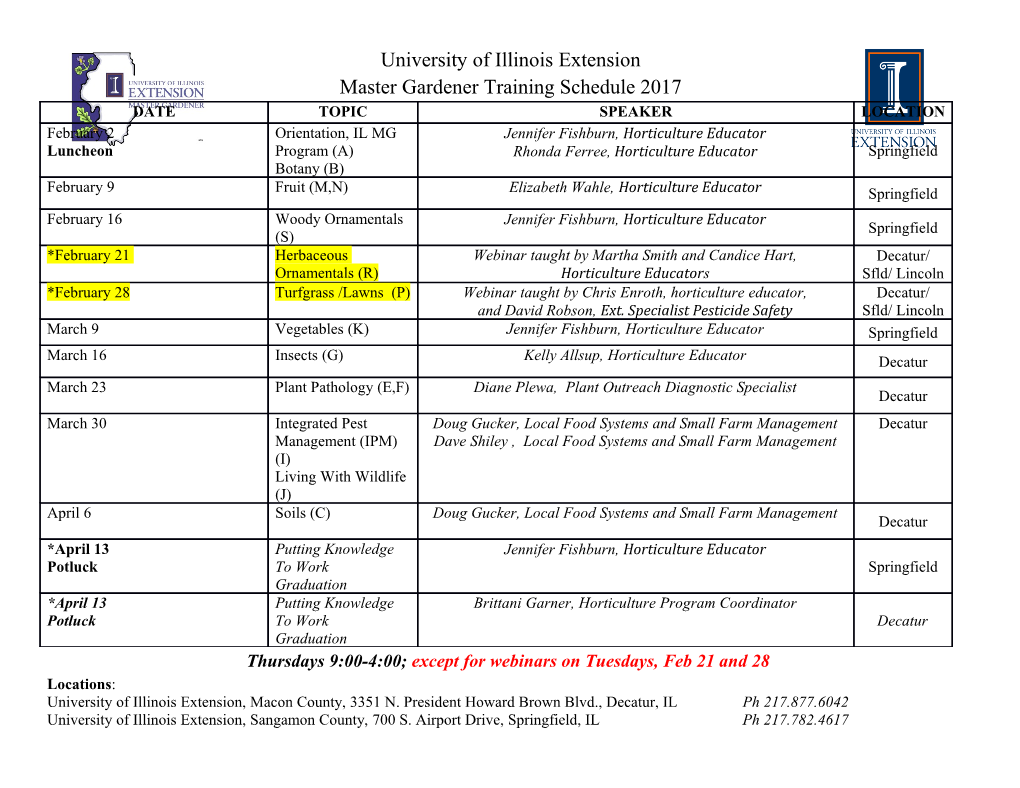
Improving on the James-Stein Estimator Yuzo Maruyama The University of Tokyo Abstract: In the estimation of a multivariate normal mean for the case where the unknown covariance matrix is proportional to the identity matrix, a class of generalized Bayes estimators dominating the James-Stein rule is obtained. It is noted that a sequence of estimators in our class converges to the positive-part James-Stein estimator. AMS 2000 subject classifications: Primary 62C10, 62C20; secondary 62A15, 62H12, 62F10, 62F15. Keywords and phrases: minimax, multivariate normal mean, generalized Bayes, James-Stein estimator, quadratic loss, improved variance estimator. 1. Introduction Let X and S be independent random variables where X has the p-variate normal 2 2 2 distribution Np(θ, σ Ip) and S/σ has the chi square distribution χn with n degrees of freedom. We deal with the problem of estimating the mean vector θ relative to the quadratic loss function kδ−θk2/σ2. The usual minimax estimator X is inadmissible for p ≥ 3. James and Stein (1961) constructed the improved estimator δJS = 1 − ((p − 2)/(n + 2))S/kXk2 X, JS which is also dominated by the James-Stein positive-part estimator δ+ = max(0, δJS) as shown in Baranchik (1964). We note that shrinkage estimators such as James-Stein’s procedure are derived by using the vague prior informa- tion that λ = kθk2/σ2 is close to 0. It goes without saying that we would like to get significant improvement of risk when the prior information is accurate. JS JS Though δ+ improves on δ at λ = 0, it is not analytic and thus inadmissi- ble. Kubokawa (1991) showed that one minimax generalized Bayes estimator derived by Lin and Tsai (1973) dominates δJS. This estimator, however, does not improve on δJS at λ = 0. Therefore it is desirable to get analytic improved estimators dominating δJS especially at λ = 0. In Section 2, we show that the estimators in the subclass of Lin-Tsai’s minimax generalized Bayes estimators M M 2 δα = (1 − ψα (Z)/Z)X, where Z = kXk /S and R 1 α(p−2)/2 −α(n+p)/2−1 M 0 λ (1 + λz) dλ ψα (z) = z R 1 α(p−2)/2−1 −α(n+p)/2−1 0 λ (1 + λz) dλ JS M with α ≥ 1, dominate δ . It is noted that δα with α = 1 coincides with M Kubokawa’s estimator. Further we demonstrate that δα with α > 1 improves JS M on δ especially at λ = 0 and that δα approaches the James-Stein positive-part estimator when α tends to infinity. 1 Y. Maruyama/Improving on the JSE 2 2. Main results M We first represent ψα (Z) through the hypergeometric function ∞ n X (a)n(b)n x F (a, b, c, x) = 1 + for (a) = a · (a + 1) ··· (a + n − 1). (c) n! n n=1 n The following facts about F (a, b, c, x), from Abramowitz and Stegun (1964), are needed; Z x ta−1(1 − t)b−1dt = xa(1 − x)bF (1, a + b, a + 1, x)/a for a, b > 1, (2.1) 0 c(1 − x)F (a, b, c, x) − cF (a, b − 1, c, x) + (c − a)xF (a, b, c + 1, x) = 0, (2.2) (c−a−b)F (a, b, c, x)−(c−a)F (a−1, b, c, x)+b(1−x)F (a, b+1, c, x) = 0, (2.3) F (a, b, c, 1) = ∞ when c − a − b ≤ −1. (2.4) Making a transformation and using (2.1), we have R 1 α(p/2−1) −α(n+p)/2−1 0 λ (1 + λz) dλ R 1 α(p/2−1)−1 −α(n+p)/2−1 0 λ (1 + λz) dλ α(p/2 − 1) F (1, α(n + p)/2 + 1, α(p/2 − 1) + 2, z/(z + 1)) = . α(p/2 − 1) + 1 F (1, α(n + p)/2 + 1, α(p/2 − 1) + 1, z/(z + 1)) Moreover by (2.2) and (2.3), we obtain p − 2 n + p ψM (z) = 1 − . α n + 2 (n + 2)F (1, α(n + p)/2, α(p − 2)/2 + 1, z/(z + 1)) + p − 2 (2.5) Making use of (2.5), we can easily prove the theorem. M JS Theorem 2.1. The estimator δα with α ≥ 1 dominates δ . M Proof. We shall verify that ψα (z) with α ≥ 1 satisfies the condition for dom- inating the James-Stein estimator derived by Kubokawa (1994): for δψ = (1 − ψ(Z)/Z)X, ψ(z) is nondecreasing, limz→∞ ψ(z) = (p − 2)/(n + 2), and ψ(z) ≥ M M ψ1 (z). Since F (1, α(n+p)/2, α(p−2)/2+1, z/(z +1)) is increasing in z, ψα (z) M is increasing in z. By (2.4), it is clear that limz→∞ ψα (z) = (p − 2)/(n + 2). M M In order to show that ψα (z) ≥ ψ1 (z) for α ≥ 1, we have only to check that F (1, α(n + p)/2, α(p − 2)/2 + 1, z/(z + 1)) is increasing in α, which is easily verified because the coefficient of each term of the r.h.s. of the equation F (1, α(n + p)/2, α(p − 2)/2 + 1, z/(z + 1)) p + n z (p + n)(p + n + 2/α) z 2 (2.6) = 1 + + + ··· p − 2 + 2/α 1 + z (p − 2 + 2/α)(p − 2 + 4/α) 1 + z is increasing in α. We have thus proved the theorem. Y. Maruyama/Improving on the JSE 3 JS JS M Fig 1. Comparison of the risks of the estimators δ , δ+ , δα with α = 1, 2, 10 M Now we investigate the nature of the risk of δα with α ≥ 1. By using Kubokawa’s (1994) method, the risk difference between the James-Stein esti- M mator and δα at λ = 0 is written as Z ∞ M M Z z JS M d M ψα (z) − ψ1 (z) −1 R(0, δ )−R(0, δα ) = 2(n+p) ψα (z) M s h(s)dsdz, 0 dz 1 + ψ1 (z) 0 R ∞ 2 where h(s) = 0 vfn(v)fp(vs)dv and fp(t) designates a density of χp. Therefore M we see that Kubokawa’s (1991) estimator (δα with α = 1) does not improve M upon the James-Stein estimator at λ = 0. On the other hand, since ψα (z) is M strictly increasing in α, δα with α > 1 improves on the James-Stein estimator M especially at λ = 0 and the risk R(λ, δα ) at λ = 0 is decreasing in α. Figure 1 gives a comparison of the respective risks of the James-Stein estimator, its M positive-part rule and δα with α = 1, 2 and 10 for p = 8 and n = 4. In the M figure ’noncentrality parameter’ denotes kθk/σ and Mα denotes the risk of δα . M This figure reveals that the risk behavior of δα with α = 10 is similar to that of the James-Stein positive-part estimator. In fact, we have the following result. M Proposition 2.1. δα approaches the James-Stein positive-part estimator when M JS α tends to infinity, that is, limα→∞ δα = δ+ . Proof. Since F (1, α(n + p)/2, α(p − 2)/2 + 1, z/(z + 1)) is increasing in α, by the P∞ monotone convergence theorem this function converges to i=0{((p − 2)(z + Y. Maruyama/Improving on the JSE 4 1))−1(n + p)z}i. Considering two cases: (n + p)z < (≥)(p − 2)(z + 1), we obtain M limα→∞ ψα (z) = z if z < (p − 2)/(n + 2); = (p − 2)/(n + 2) otherwise. This completes the proof. We, here, consider the connection between the estimation of the mean vec- tor and the variance. It is noted that the James-Stein estimator is expressed as 2 2 2 −1 2 (1−((p−2)/kXk )ˆσ0)X, forσ ˆ0 = (n+2) S and thatσ ˆ0 is the best affine equiv- ariant estimator for the problem of estimating the variance under the quadratic 2 2 2 loss function (δ/σ − 1) . Stein (1964) showed thatσ ˆ0 is inadmissible. George (1990) suggested that it might be possible to use an improved variance estimator to improve on the James-Stein estimator. In the same way as Theorem 2.1, we have the following result, which includes Berry’s (1994) and Kubokawa et al.’s (1993). 2 Theorem 2.2. Assume that σˆIM = φIM (Z)S is an improved estimator of variance, which satisfies Brewster and Zidek’s (1974) condition, that is, φIM (z) BZ is nondecreasing and φ (z) ≤ φIM (z) ≤ 1/(n + 2), where 1 1 R λp/2−1(1 + λz)−(n+p)/2−1dλ φBZ (z) = 0 . n + p + 2 R 1 p/2−1 −(n+p)/2−2 0 λ (1 + λz) dλ 2 2 JS Then δIM = (1 − {(p − 2)/kXk }σˆIM )X dominates δ . It should be, however, noticed that the estimator derived from Theorem 2.2 2 2 is obviously inadmissible, since (1 − {(p − 2)/kXk }σˆIM ) sometimes takes a negative value. References Abramowitz, M. and Stegun, I. A. (1964). Handbook of mathematical func- tions with formulas, graphs, and mathematical tables. National Bureau of Standards Applied Mathematics Series 55. MR0167642 (29 ##4914) Baranchik, A. J. (1964). Multiple regression and estimation of the mean of a multivariate normal distribution. Technical Report, Department of Statistics, Stanford University. Berry, J. C. (1994). Improving the James-Stein estimator using the Stein variance estimator.
Details
-
File Typepdf
-
Upload Time-
-
Content LanguagesEnglish
-
Upload UserAnonymous/Not logged-in
-
File Pages5 Page
-
File Size-