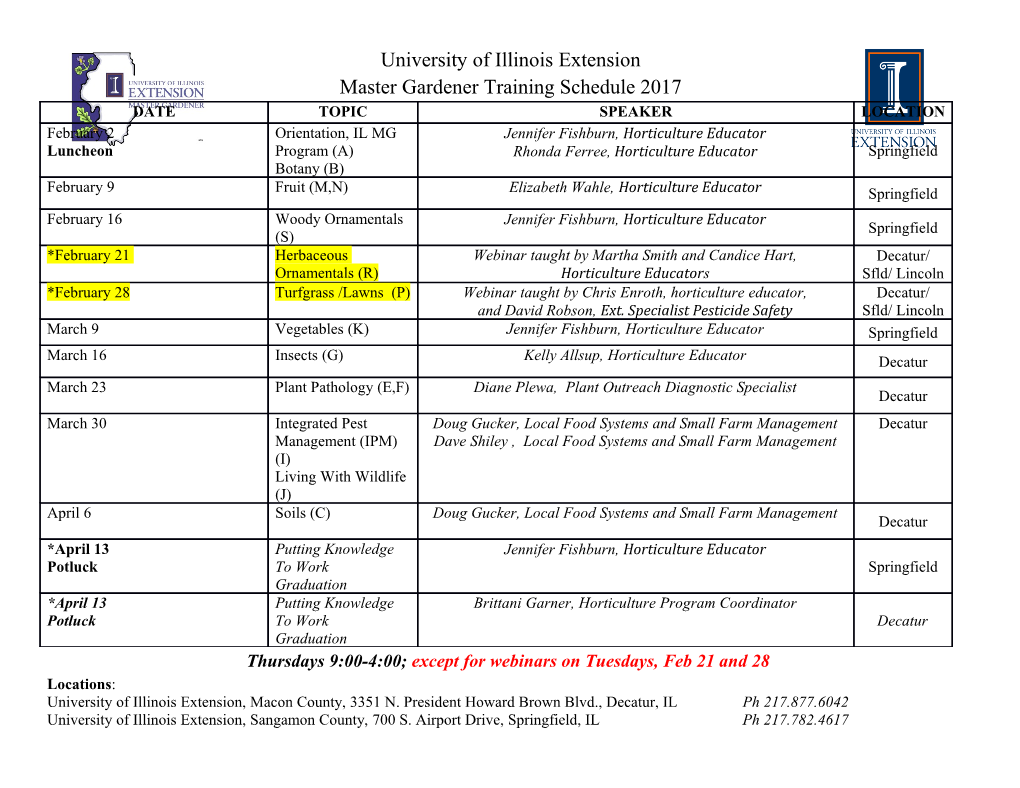
C E N Bioinformatics courses T R E Principles of Bioinformatics (BSc) & F B O I Fundamentals of Bioinformatics R O I I N N (MSc) T F E O G R R M A A T T I I Lecture 3: (local) alignment and V C E S homology searching V U Centre for Integrative Bioinformatics VU (IBIVU) Faculty of Exact Sciences / Faculty of Earth and Life Sciences 1 http://ibi.vu.nl, [email protected], 87649 (Heringa), Room P1.28 Divergent evolution sequence -> structure -> function • Common ancestor (CA) CA • Sequences change over time • Protein structures typically remain the same (robust against Sequence 1 ≠ Sequence 2 multiple mutations) • Therefore, function normally is Structure 1 = Structure 2 preserved within orthologous families Function 1 = Function 2 “Structure more conserved than sequence” 2 Reconstructing divergent evolution Ancestral sequence: ABCD ACCD (B C) ABD (C ø) mutation deletion ACCD or ACCD Pairwise Alignment AB─D A─BD 3 Reconstructing divergent evolution Ancestral sequence: ABCD ACCD (B C) ABD (C ø) mutation deletion ACCD or ACCD Pairwise Alignment AB─D A─BD true alignment 4 Pairwise alignment examples A protein sequence alignment MSTGAVLIY--TSILIKECHAMPAGNE----- ---GGILLFHRTHELIKESHAMANDEGGSNNS * * * **** *** A DNA sequence alignment attcgttggcaaatcgcccctatccggccttaa att---tggcggatcg-cctctacgggcc---- *** **** **** ** ****** 5 Evolution and three-dimensional protein structure information Multiple alignment Protein structure What do we see if we colour code the space-filling (CPK) protein model? • E.g., red for conserved alignment positions to blue for variable (unconserved) positions. 6 Evolution and three-dimensional protein structure information Isocitrate dehydrogenase: The distance from the active site (in yellow) determines the rate of evolution (red = fast evolution, blue = slow evolution) Dean, A. M. and G. B. Golding: Pacific Symposium on 7 Bioinformatics 2000 Can we just transfer information about structure and/or function? • Structure (and function) more CA conserved than sequence • Sequence -> structure ->function Sequence 1 ≠ Sequence 2 • So, if the sequences already tell us it’s the same thing (homolog), then certainly the Structure 1 Structure 2 structures and functions are = supposed to be the same. • This works most of the time, Function 1 = Function 2 but there are cases where likely homology does not bear out. 8 What function does your gene have • We are going to use the homology principle • We are going to seriously search through sequence databases – Non-redundant (NR) database > 7 million sequences – Each and every sequence should be considered 9 Sequence searching - challenges • Exponential growth of databases Log function: Straight line implies exponential growth 10 Bioinformatics justification • “Mind the Gap” • There are far more sequence data than structural/functional data • We need to fill this gap by analysis and prediction pipelines 11 12 PRALINE web-interface 13 Frequently used (input) format to describe protein sequences: Fasta Format Sequence start indicator ‘>’ Sequence name Sequence Fasta files can contain many sequences starting with a ‘>’ symbol. The ‘>’ symbol each time signifies a new sequence. Ess. Bioinf.14 P.25 A protein sequence alignment MSTGAVLIY--TSILIKECHAMPAGNE----- ---GGILLFHRTHEHATECHAMPAGNEGGSNNS * * * ********* A DNA sequence alignment attcgttggcaaatcgcccctatccggccttaa att---tggcggatcg-cctctacgggcc---- *** **** **** ** ****** DISCLAIMER: Alignment should only be applied to (putative) homologous sequences!! All sequences are supposed to derive from a common ancestor. Ideally, an orthologous set of sequences gets aligned. 15 How many pair-wise alignments T D W V T A L K T D W L - - I K Combinatorial explosion - 1 gap in 1 sequence: n+1 possibilities - 2 gaps in 1 sequence: (n+1)n - 3 gaps in 1 sequence: (n+1)n(n-1), etc. 2n (2n)! 22n = ~ n (n!)2 √πn 2 sequences of 300 a.a.: ~1088 alignments 600 2 sequences of 1000 a.a.: ~10 alignments! 16 Technique to overcome the alignment combinatorial explosion: Dynamic Programming (DP) • Break alignment problem up in smaller subproblems and solve these iteratively • Alignment is simulated as a Markov process, all sequence positions are seen as independent and identically distributed (i.i.d). • Chances of sequence events are independent o Therefore, probabilities per aligned position are multiplied o Amino acid matrices contain so-called log-odds values (log10 of the probabilities), so probabilities can be summed [log(ab)=log(a)+log(b)] 17 History of Dynamic Programming algorithm 1970 Needleman-Wunsch global pair-wise alignment Needleman SB, Wunsch CD (1970) A general method applicable to the search for similarities in the amino acid sequence of two proteins, J Mol Biol. 48(3):443-53. • Align sequences in their entirety 1981 Smith-Waterman local pair-wise alignment Smith, TF, Waterman, MS (1981) Identification of common molecular subsequences. J. Mol. Biol. 147, 195-197. • Only align subsequences with sufficient evolutionary memory (conservation) • BLAST incorporates an heuristic version of local pairwise alignment 18 Pairwise sequence alignment Global dynamic programming (DP) MDAGSTVILCFVG M Evolution D A A S T I L C G Residue Exchange S Matrix Search matrix Gap penalties MDAGSTVILCFVG- (open,extension) MDAAST-ILC--GS 19 Dynamic programming matrix j i Gap in sequence i Gap in sequence j Match/mismatch The cell [i, j] contains the alignment score of the best scoring alignment of subsequence 1..i and 1..j, that is, the subsequences up to [i, j] Cell [i, j] does not ‘know’ what that best scoring alignment is (it is one or a number of alternatives) out of very many possibilities, leading to [i, j]) By going through the matrix in row-wise fashion, each time extend alignment from cell [i, j] 20 Global dynamic programming j-1 j Value from i-1 residue i exchange matrix (or match/ mismatch) H(i-1,j-1) + s(i,j) diagonal H(i,j) = Max H(i-1,j) - g vertical H(i,j-1) - g horizontal This is a recursive formula Gap penalty 21 Substitution matrices for a.a. n Amino acids are not equal: 1. Some are similar and easily substituted: • biochemical properties • structure http://www.people.virginia.edu/~rjh9u/aminacid.html orange: nonpolar and hydrophobic. green: polar and hydrophilic magenta box are acidic 2. Some mutations occur light blue box are basic more often due to similar codons n The two above give us substitution matrices http://www.cimr.cam.ac.uk/links/codon.htm 22 BLOSUM 62 substitution matrix Henikoff & Henikoff, PNAS 89:10915; 1993 Positive values - Preferred substitution Negative values - Avoided substitution Zero values -Randomly expected M[i-1,j-1] + S(x[i],y[j]) M[i,j]= max M[i,j-1]-2 Gap Penalty M[i-1,j]-2 23 Substitution Matrices: DNA define a score for match/mismatch of letters Simple: A C G T A 1 -1 -1 -1 M[i-1,j-1] ±1 M[i,j]= max M[i,j-1]-2 C -1 1 -1 -1 M[i-1,j]-2 G -1 -1 1 -1 T -1 -1 -1 1 Used in genome alignments: A C G T A 91 -114 -31 -123 M[i-1,j-1] + S(x[i],y[j]) M[i,j]= max M[i,j-1]-2 C -114 100 -125 -31 M[i-1,j]-2 G -31 -125 100 -114 This is how the substitution T -123 -31 -114 91 scores are used 24 Example: global alignment of two sequences • Align two DNA sequences: – GAGTGA – GAGGCGA (note the length difference) • Parameters of the algorithm: – Match: score(A,A) = 1 – Mismatch: score(A,T) = – 1 – Gap: g = 2 M[i-1,j-1] ±1 M[i,j]= max M[i,j-1]-2 M[i-1,j]-2 The algorithm. Step 1: init • Create the M[i-1,j-1] ±1 M[i,j]= max M[i,j-1]-2 matrix M[i-1,j]-2 • Initiation – 0 at [0,0] j→ 0 1 2 3 4 5 6 – Apply the i ↓ - G A G T G A equation… 0 - 0 1 G 2 A 3 G 4 G 5 C 6 G 7 A The algorithm. Step 1: init M[i-1,j-1] ±1 M[i,j]= max M[i,j-1]-2 M[i-1,j]-2 j - G A G T G A - 0 -2 -4 -6 -8 -10 -12 • Initiation of the G -2 matrix: A -4 – 0 at pos [0,0] G -6 – Fill in the first row i -8 using the “è” rule G – Fill in the first C -10 column using “ê” G -12 A -14 The algorithm. Step 2: fill in M[i-1,j-1] ±1 M[i,j]= max M[i,j-1]-2 M[i-1,j]-2 j - G A G T G A - 0 -2 -4 -6 -8 -10 -12 • Continue filling G -2 1 -1 -3 in the matrix, A -4 -1 2 remembering -6 i G from which cell G -8 the result comes C -10 (arrows) G -12 A -14 The algorithm. Step 2: fill in M[i-1,j-1] ±1 M[i,j]= max M[i,j-1]-2 M[i-1,j]-2 j - G A G T G A - 0 -2 -4 -6 -8 -10 -12 • We are done… G -2 1 -1 -3 -5 -7 -9 • Where’s the A -4 -1 2 0 -2 -4 -6 -6 -3 0 3 1 -1 -3 result? i G G -8 -5 -2 1 2 2 0 C -10 -7 -4 -1 0 1 1 G -12 -9 -6 -3 -2 1 0 A -14 -11 -8 -5 -4 -1 2 The algorithm. Step 2: fill in M[i-1,j-1] ±1 M[i,j]= max M[i,j-1]-2 M[i-1,j]-2 j - G A G T G A - 0 -2 -4 -6 -8 -10 -12 • We are done… G -2 1 -1 -3 -5 -7 -9 • Where’s the A -4 -1 2 0 -2 -4 -6 -6 -3 0 3 1 -1 -3 result? i G G -8 -5 -2 1 2 2 0 The lowest- C -10 -7 -4 -1 0 1 1 G -12 -9 -6 -3 -2 1 0 rightmost cell A -14 -11 -8 -5 -4 -1 2 The algorithm.
Details
-
File Typepdf
-
Upload Time-
-
Content LanguagesEnglish
-
Upload UserAnonymous/Not logged-in
-
File Pages108 Page
-
File Size-