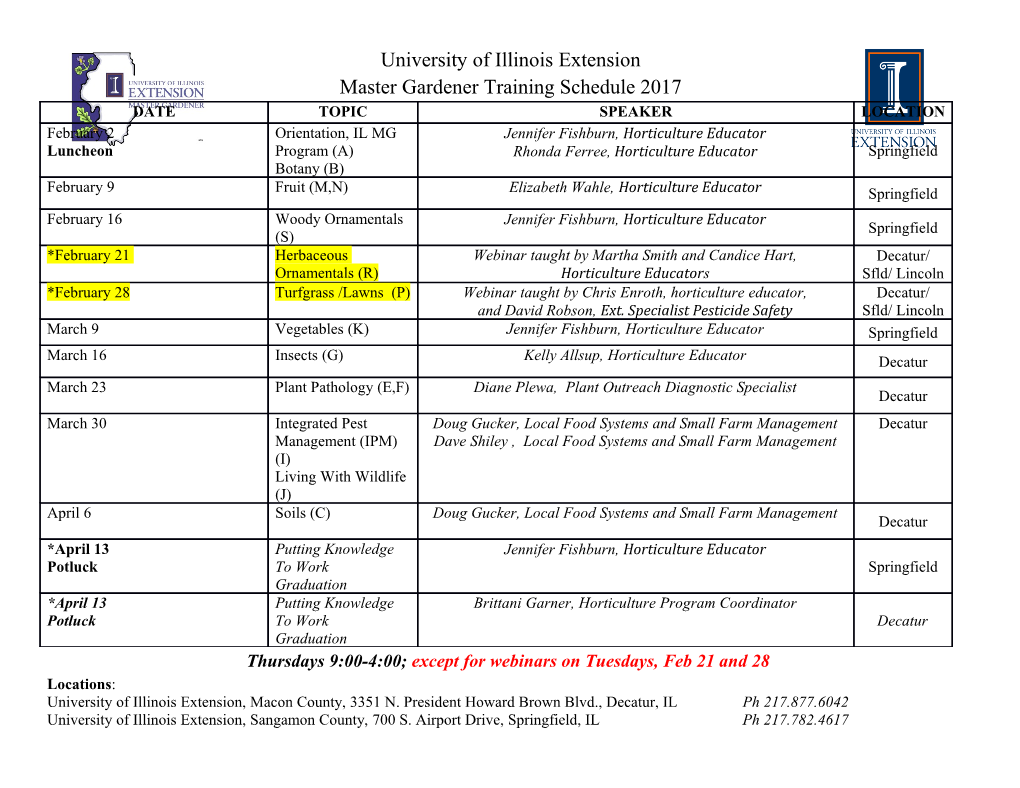
Research Article GU J Sci 31(3): 967-983 (2018) Gazi University Journal of Science http://dergipark.gov.tr/gujs Log-Gamma - Rayleigh Distribution: Properties and Applications Samir ASHOUR 1, Darwish DARWISH 1, Ahmad SAAD 1,* 1 Institute of Statistical Studies & Research, Cairo University, 5 Ahmed Zowil St., Orman, Giza, Egypt Article Info Abstract In this paper, for the first time we defined and studied a new two parameter lifetime model by Received: 14/08/2017 using the T-X method, called the Log-Gamma Rayleigh distribution. This distribution can be Accepted: 20/04/2018 considered as a new generalization of the Gamma distribution and the Rayleigh distribution. We obtain some of its mathematical properties. Some structural properties of the new distribution are studied. Maximum likelihood estimation method is used for estimating the model Keywords parameters. An application to real data set is given to show the flexibility and potentiality of the T-X method new model. Gamma distribution Rayleigh distribution Quantile function Hazard function Moments Maximum likelihood estimation 1. INTRODUCTION Eugene et al. [6] for the first time introduced the beta-generated family of distributions. They noted that the probability density function pdf of the beta random variable and the cumulative distribution function CDF of any distribution are between 0 and 1. The beta-generated random variable X is defined with the following CDF and pdf Fx() G()() x b t dt (1) 0 and 1 g() x f () x F11 ()[1 x F ()] x B(,) where b(t) is the pdf of the beta random variable with parameters and , F(x) and f(x) are the CDF and the pdf of any random variable. Many authors derived and studied many beta-generated distributions in the literature, for example beta- Gumbel (Nadarajah and Kotz, [12]), beta-Weibull (Famoye et al. [7]), beta-exponential (Nadarajah and Kotz, [13]), beta-gamma (Kong et al., [8]), beta-Pareto (Akinsete et al., [1]), beta-generalized exponential (Barreto-Souza et al., [5]), beta-generalized Pareto (Mahmoudi, [10]), and beta-Cauchy (Alshawarbeh, et al., [2]). *Corresponding author, e-mail: [email protected] 968 Samir ASHOUR et al. / GU J Sci, 31(3): 967-983 (2018) Alzaatreh et al. [3] presented a new general method for generating new distributions, called T-X family of distributions. This method depending on replace the beta pdf in (1) with a pdf of any continuous random variable and applying a function W(F(x)) that satisfies the following conditions: 1- “W(F(x)) [a, b].” 2- “W(F(x)) is differentiable and monotonically non-decreasing.” 3- “W(F(x)) → a as x → and W(F(x)) → b as x → .” Let X be a random variable with pdf f (x) and CDF F(x), and let T be a continuous random variable with pdf r(t) and CDF R(t) defined on [a, b] for −∞ < a < b < ∞. Alzaatreh et al. [3] defined the CDF and pdf of a new family of distributions as W( F ( x )) G( x ) r ( t ) dt R { W ( F ( x ))} (2) a and d g() x [ W (())][ F x r W (())] F x dx Recently, Amini et al. [4] introduced two new general families of continuous distributions called log gamma- generated families(LG-G) of distributions as follows: For any continuous parent distribution F(x) of a random variable X with corresponding parent pdf f(x), the two new LG-G families are given with the following two pdfs: p q p1 q1 f() x log1() F x 1() F x f (), x p , q 0 1 ()p and p q p1 q1 f() x log() F x F () x f (),, x p q 0 2 ()p where (.) is the complete gamma function. Note that the log gamma- generated families which introduced by Amini et al. [4] are two special cases from T-X method defined by Alzaatreh et al. [3]. Take the W(F(x)) = (1 − F(x)) in (2), and let the random variable T follows the log-gamma distribution with the following CDF and pdf x R() x (log) x11 x ,0 x 1,, 0 (3) () 0 r() x (log) x11 x ,0 x 1,, 0 (4) () From (2), (3) and (4) we will define the log-gamma -X family based on T-X method with the following CDF and pdf 969 Samir ASHOUR et al. / GU J Sci, 31(3): 967-983 (2018) (1Fx ( )) G() x (log) x11 x , x ,,0 () 0 (5) , log(1Fx ( )) 1 () and fx() 11 g() x log(1())1(), F x F x x ,,0 (6) () where (.,.) is the lower incomplete gamma function defined by x (,)x eu u 1 du 0 Merovci and Elbatal [11] defined and studied a generalization of the Rayleigh distribution called the Weibull Rayleigh distribution (WR). In this paper we present a new generalization of the Gamma distribution and the Rayleigh distribution called the Log-Gamma Rayleigh distribution. 2. THE LOG-GAMMA - RAYLEIGH DISTRIBUTION (LGR) If the random variable X have the Rayleigh distribution with pdf and CDF given, respectively, by x 2 x 2 “ f( x ) e2 , x 0 , 0” (7) 2 and x 2 F( x ) 1 e2 2 , x 0 , 0 (8) then using (5), (6), (7) and (8) the log-gamma - Rayleigh distribution (LGR) is defined with CDF x 2 (,)2 G( x ) 1 2 , x 0 , , , 0 () The corresponding pdf of the LGR is x 2 2 xx 22 “ g() x ()(),1 e x 0,,,0 ” 22( ) 2 The LGR distribution has the following special cases: 1) When 1 , the gamma distribution is obtained as 970 Samir ASHOUR et al. / GU J Sci, 31(3): 967-983 (2018) (,)y G( y ) , y 0 , , 0 () with 1 y g( y ) y1 e , y 0 , , 0 () x 2 where y 2 2 2) When 1 , the Rayleigh distribution in (7) and (8) is obtained. Hence the log-gamma - Rayleigh distribution can be considered as a generalization of the gamma and Rayleigh distributions. If we set , then the CDF of the LGR can be written as 2 x 2 (,) G( x ) 1 2 , x 0 , , 0 (9) () and, the corresponding pdf of the LGR is given by x 2 2 xx 2 “ g( x ) ( )1 e , x 0 , , 0 ” (10) ( ) 2 “Figures 1 and 2 illustrates some of the possible shapes of the pdf and CDF of LGR for selected values of the parameters and , respectively” New Log Gamma-Rayligh pdf New Log Gamma-Rayligh pdf gxi .711.2 gxi 1.21.2 1 1 gx .721.4 gxi 1.21.4 i g x .731.6 gxi 1.21.6 i g x .741.8 gxi 1.21.8 i g x .752.0 gxi 1.22.0 i 1.2 2.2 gxi .762.2 gxi 0.5 0.5 gxi 1.22.4 gxi .772.4 gxi 1.22.4 gxi .782.4 0 0 0 1 2 3 0 1 2 3 xi xi Figure 1. Density function gx(;,) of the LGR The graphs in Figure 1 indicate that the LGR is unimodal. 971 Samir ASHOUR et al. / GU J Sci, 31(3): 967-983 (2018) New Log Gamma-Rayligh CDF New Log Gamma-Rayligh CDF 1.1 1 Gxi .22.2 Gxi .20.6 1 Gxi .22.3 Gxi .22.6 G x .22.4 Gxi .24.6 i Gxi .26.6 Gxi .22.5 Gxi .28.6 Gxi .22.6 Gxi .30.6 0.5 .22 .7 Gxi 0.5 Gx .32.6 i Gxi .22.8 Gxi .34.6 Gxi .22.9 0 0 0 0 1 2 3 0 1 2 3 0 xi 3.25 x i Figure 2. Cumulative function Gx(;,) of the LGR Some remarks of LGR: 1) If a random variable Y follows the log-gamma distribution with parameters and , then the random 21 variable X log( ) follows the LGR. Y 2) If a random variable Z follows the gamma distribution with parameters and , then the random 2 variable XZ follows the LGR. 2.1. Survival and Hazard Functions The survival function of the LGR is x 2 (,) Sx() 2 () “The hazard rate function and reversed hazard rate function of the LGR will be” x 2 2 x 1 2 gx() xe() Hx()2 1Gx ( ) x 2 (,) 2 and x 2 2 x 1 2 gx() xe() ()x 2 Gx() x 2 ()(,) 2 2 972 Samir ASHOUR et al. / GU J Sci, 31(3): 967-983 (2018) Figures 3 and 4 illustrates some of the possible shapes of the survival and hazard rate function of LGR for selected values of the parameters and , respectively Survival of New Log Gamma-Rayligh Survival of New Log Gamma-Rayligh Sxi .20.6 1 Sxi .22.2 1 Sxi .22.6 Sxi .22.3 Sxi .24.6 Sxi .22.4 Sx .26.6 Sxi .22.5 i S x .28.6 Sx .22.6 i i S x .30.6 0.5 Sx .22.7 i i 0.5 S x .32.6 S x .22.8 i i S x .34.6 S x .22.9 i i 0 0 0 1 2 3 0 1 2 3 xi xi Figure 3. Survival function Sx(;,) of the LGR Hazard of New Log Gamma-Rayligh Hazard of New Log Gamma-Rayligh 10 10 H x .711.2 Hx 1.21.2 i i H x .721.4 H x 1.21.4 8 i 8 i H x 1.21.6 Hxi .731.6 i H x 1.21.8 Hxi .741.8 i 6 6 H x 1.22.0 Hxi .752.0 i .76 2.2 Hxi 1.22.2 Hxi 4 4 H x .772.4 Hxi 1.22.4 i H x .782.4 Hxi 1.22.4 i 2 2 0 0 0 1 2 3 0 1 2 3 xi xi Figure 4.
Details
-
File Typepdf
-
Upload Time-
-
Content LanguagesEnglish
-
Upload UserAnonymous/Not logged-in
-
File Pages17 Page
-
File Size-