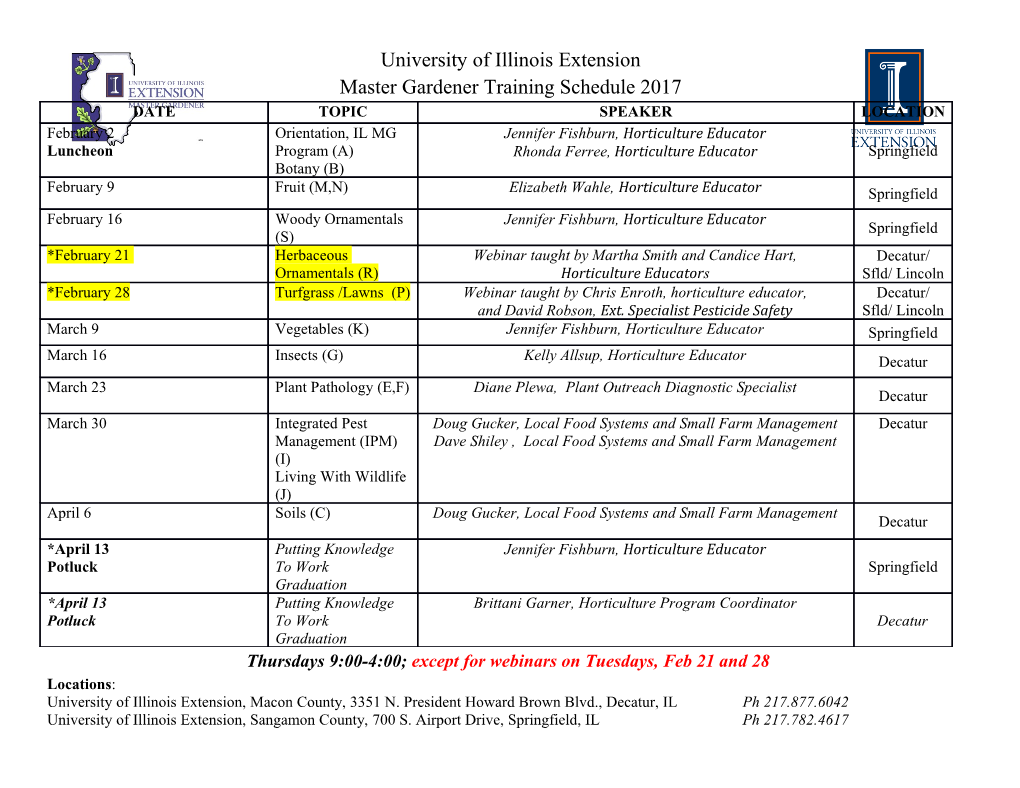
MSc. Thesis (MSCV) Department of Applied Computer Science University of Bourgogne Non-destructive assessment of quality and maturity of mangoes based on image analysis Kumar Ankush A Thesis submitted in July 2020 for the Degree of MSc in Computer Vision (VIBOT/MSCV) Master thesis supervised by Dr. Emile Faye and Dr. Julien Sarron of the UPR HortSys of CIRAD. Abstract Quality and maturity estimation of mangoes are important for the organization of harvesting date and post-harvest conservation. Although extensive fruit quality estimations exist, they are mostly destructive in nature and available tools for non-destructive estimation are limited. Maturity estimation rely on visual inspection of the fruit, in-hand feel or destructive measure- ment based on quality assessment. Thus, non-destructive tools for an accurate estimation of the quality and the maturity of the fruit have yet to be developed, especially for smallhold- ers. The aim of this study was to develop a tool for non-destructive assessment of quality and maturity of mangoes based on image analysis. This experiment studied 1040 lateral RGB images of 520 mangoes of di↵erent stages of maturity and harvested in two orchards in West Africa. Upon performing digital image segmentation on the images of mangoes, six image fea- tures were calculated with the use of digital image processing functions in MATLAB and four destructive features were taken in consideration. Then, correlations between destructive and non-destructive features of mangoes were explored. No research is ever quite complete. It is the glory of a good bit of work that it opens the way for something still better, and this repeatedly leads to its own eclipse... Mervin Gordon Contents Acknowledgments iv 1 Background 1 1.1 Introduction....................................... 1 1.2 Problemdefinition ................................... 6 1.3 Stateoftheart..................................... 7 2 Methodology 8 3Results 12 3.1 First part of the objective: segmentation of images . 12 3.2 Second part of the objective: classification of mango images into sunny and shade side ........................................... 12 3.3 Third part of the objective: calculation of color and shape image features . 13 4 Discussions 15 A The first appendix 17 Bibliography 23 i List of Figures 1.1 Production of Mangoes, mango steens and guavas in West Africa . 3 1.2 Production of Mangoes, mango steens and guavas in Senegal [3] . 4 1.3 Production of Mangoes, mango steens and guavas in Cote d’Ivoire [3] . 5 2.1 Mango being drilled for pulp collection . 10 2.2 Kent Maturity Scale for Mangoes . 11 A.1 Mango Sample Batch and segmentation images . 18 A.2 Mango Sample Batch and segmentation images . 19 ii List of Tables 3.1 Batch accuracy of classification . 14 iii Acknowledgments I take this opportunity to express my sincere and heartfelt gratitude to my Master’s thesis supervisor Dr. Emile Faye for believing in my capabilities and encouraging me to understand and link complex concepts. I would like to thank Dr. Julien Sarron. I really appreciate your constant support through- out broadening my knowledge and professional development. You taught me to be like a sponge and absorb all the knowledge that comes along the way and that there is no evidence or measure of failure or success if you do not even try. I am thankful to Dr. Lew and Dr. Fofi for their guidance during the coursework. I am highly indebted to Herma, Alice, Elizabeth and Aurelie for all the administrative and official assistance. Lastly, I express gratitude towards my dear VIBOT batchmates for their unconditional support throughout this Master’s Programme. I will not be able to thank enough my parents who always strenghthen me by teaching to stand tall and fight hard to find the way through the world of uncertainties. I feel blessed to have my wife Pratima Gurung’s immense support and love throughout my journey. iv Chapter 1 Background 1.1 Introduction There is a pressing need for fruit maturity estimation for increased food security and less wastage of perishable food in either storage of transportation. According to an estimation of Food and Agriculture Organization of the United Nations (FAO), in 2011 a third or 1.3 billion metric tons of the world’s food was lost or wasted each year [5] with about 88 million metric tons wasted in Europe [6] with associated cost around 148 billion Euros. In order to reduce the food waste proper grading of food is important to decrease food loss and increase profitability of the farmers. Skin colour is an acceptable and recognized maturity indexing technique for many fruits [20]. Since most fruits show demonstrable changes on the outside with change in their physio-chemical properties, such as hormone secretion and change in polarization of reflected light, change in skin colour is however the most easily perceivable one. Having an index-scale to grade the change becomes useful in plucking, handling and sorting and thus becomes a key step in cultivation management. Producers also strive to prevent products with defective colors from entering the market, as well as to ensure that individual products are packed in batches of similar colour. It is crucial to identify maturity index of fruits since immature fruits exhibit erratic ripening behavior if handled improperly [15]. It almost certainly leads to improper flavor, aroma and texture of the fruit, ultimately leading to its rejection either during transport or in retail. By addressing these key concerns will impact in three big ways. First, it will reduce food loss. Streamlining the process of harvesting, picking of fruits, appropriate packaging and trans- 1 Chapter 1: Background 2 port will result in less food which is lost before it can be served on a plate. Second, it will reshape the workforce and organize the logistics efficiently [16]. Knowing certain packages must be transported on a priority and carefully, will give access to prioritize specific markets and conveyance. Third, it will optimize long term storage and retail. Fruits can be sold at priority in retail depending on their maturity, thus reducing loss to the seller and leading to more value delivered to the consumer. However, these estimations aren’t easy to perform by humans. In a study by Paulus et al. , even trained operators faced difficulties in reproducing the estimation of quality where parameters such as shape, size and colour were involved and termed it as ‘inconsistency’ [26]. As the number of parameters increased, which is an increase in complexity and decision making process, the error in classification also increased. Area of Computer Vision o↵ers powerful tools for automated inspection of fruits and vegeta- bles. The artificial vision system is capable of achieving beyond human capacity in evaluating long-term process accurately in much shorter time through image capturing devices. It can simplify tedious monitoring process flow that take complex operations to be performed. With use of devices which can ‘see’ electromagnetic waves outside of visible range have brought more data points, thus adding more features for accurate analysis [35]. Hyperspectral analysis can even ‘look into’ food damage through bruise of infection under the peel, helping to isolate such food and hence controlling the spread of disease or release of excess plant hormones from bruised parts of the food. Choi KH et al. in 1994 in accordance with United States Department of Agriculture pro- posed a classification of tomatoes in 6 classes [7]. Similar attempts were made by Liming X et al. in 2010 for classification of strawberries [17]. Martynenko et al. in 2008 developed a process for estimating changes in density and porosity of ginseng roots during drying, thus eliminat- ing use of scanning electron microscope in imaging [19].The ultimate aim of many computer vision-based inspection systems is to estimate one or more features of the product of interest at a given time and compare them to the consistency that is usually associated with maturity, absence of deformities and blemishes, etc. Other systems are designed to determine the prod- uct’s evolution over time to determine whether a given process is valid. Mango (Mangifera indicaL.) is the world’s 5th most produced fruit (50 metric tonnes) [9], grown mainly in semi-arid, tropical and subtropical areas. The fruit is produced mostly by In- dia (16 Mt) and China (4.3 Mt) in South-East Asia. Mexico (23% of world exports), Brazil (14.3%) and Peru (10.3%) are the main exporting countries [9]. All of Africa accounts for 9% 3 1.1 Introduction while West Africa 4% (1.5 Mt) of the world’s mango production [4]. In West Africa, however, production has doubled over the last decade, marking the country with the highest rate of growth in production in the world. Figure 1.1: Production of Mangoes, mango steens and guavas in West Africa Cote d’Ivore and Senegal combined are major mango producing countries in West Africa with 93,267 tonnes and 133,636 tonnes of produce in 2018 respectively [10]. Of these, around 10,000 and 60,000 tonnes were exported to the Europe [4]. A chart representation of the produc- tion of mango, mangosteens and guavas as reported by the FAO has been included as Figure 1.2 and Figure 1.3. Mangoes are one of the climacteric fruits – fruits that ripen, become softer and sweeter post-harvest. Such fruits are not considered to be of desired eating quality at the time of initial maturity. It takes a ripening period to develop desired taste and texture at the time of con- sumption. The maturing process is driven by genetic, environmental and biochemical events resulting in biochemical and physical changes such as loss of ascorbic acid [14], increase in total soluble solids including sugar and starch [25]; physical changes such as weight, firmness, size and color [24], and aroma changes, nutritional value and flavour.
Details
-
File Typepdf
-
Upload Time-
-
Content LanguagesEnglish
-
Upload UserAnonymous/Not logged-in
-
File Pages29 Page
-
File Size-