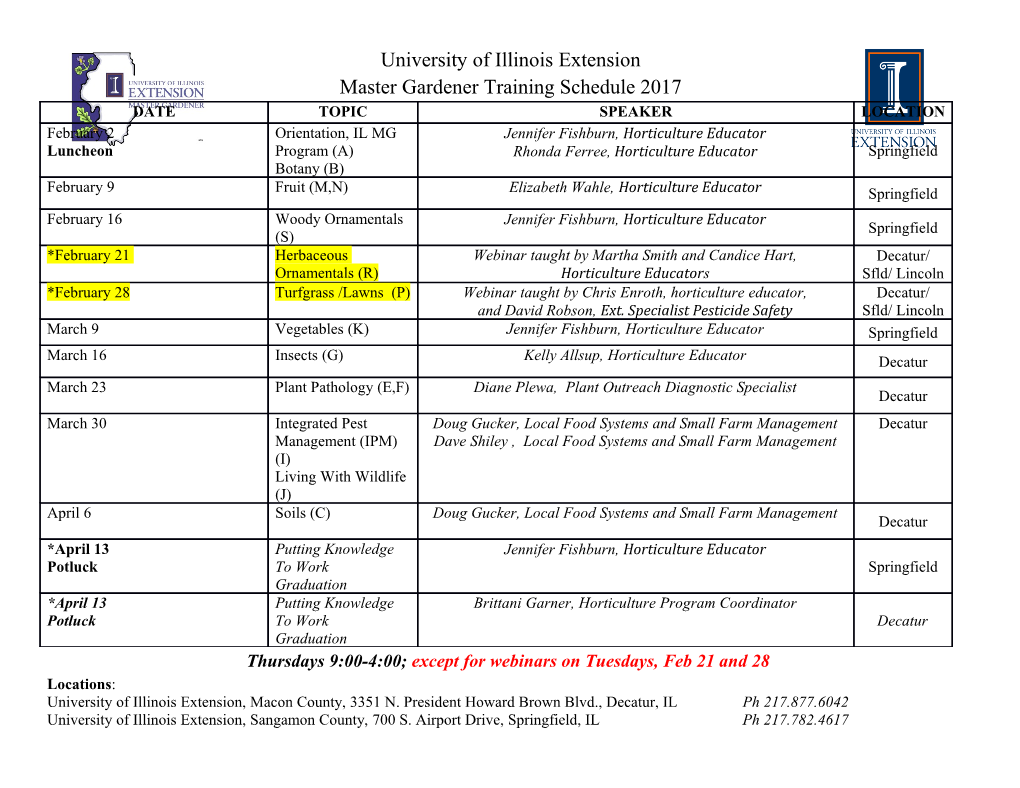
On the Parameterized Complexity of Polytree Learning Niels Grüttemeier , Christian Komusiewicz and Nils Morawietz∗ Philipps-Universität Marburg, Marburg, Germany {niegru, komusiewicz, morawietz}@informatik.uni-marburg.de Abstract In light of the hardness of handling general Bayesian networks, the learning and inference prob- A Bayesian network is a directed acyclic graph that lems for Bayesian networks fulfilling some spe- represents statistical dependencies between vari- cific structural constraints have been studied exten- ables of a joint probability distribution. A funda- sively [Pearl, 1989; Korhonen and Parviainen, 2013; mental task in data science is to learn a Bayesian Korhonen and Parviainen, 2015; network from observed data. POLYTREE LEARN- Grüttemeier and Komusiewicz, 2020a; ING is the problem of learning an optimal Bayesian Ramaswamy and Szeider, 2021]. network that fulfills the additional property that its underlying undirected graph is a forest. In One of the earliest special cases that has received atten- this work, we revisit the complexity of POLYTREE tion are tree networks, also called branchings. A tree is a LEARNING. We show that POLYTREE LEARN- Bayesian network where every vertex has at most one par- ING can be solved in 3n · |I|O(1) time where n is ent. In other words, every connected component is a di- the number of variables and |I| is the total in- rected in-tree. Learning and inference can be performed in stance size. Moreover, we consider the influence polynomial time on trees [Chow and Liu, 1968; Pearl, 1989]. of the number of variables d that might receive a Trees are, however, very limited in their modeling power nonempty parent set in the final DAG on the com- since every variable may depend only on at most one other plexity of POLYTREE LEARNING. We show that variable. To overcome this problem, a generalization of branchings called polytrees has been proposed. A poly- POLYTREE LEARNING has no f(d) · |I|O(1)-time algorithm, unlike Bayesian network learning which tree is a DAG whose underlying undirected graph is a for- est. An advantage of polytrees is that the inference task can be solved in d O(1) time. We show that, in 2 · |I| can be performed in polynomial time on them [Pearl, 1989; contrast, if d and the maximum parent set size are Guo and Hsu, 2002]. POLYTREE LEARNING, the problem bounded, then we can obtain efficient algorithms. of learning an optimal polytree structure from parent scores, however, remains NP-hard [Dasgupta, 1999]. We study exact 1 Introduction algorithms for POLYTREE LEARNING. Bayesian networks are the most important tool for modelling statistical dependencies in joint probability distributions. A Related Work. POLYTREE LEARNING is NP-hard even if every parent set with strictly positive score has size arXiv:2105.09675v1 [cs.DS] 20 May 2021 Bayesian network consists of a DAG (N, A) over the vari- [ ] able set N and a set of condiditional probability tables. Given at most 2 Dasgupta, 1999 . Motivated by the contrast a Bayesian network and the observed values on some of its between the NP-hardness of POLYTREE LEARNING and variables, one may infer the probability distributions of the the fact that learning a tree has a polynomial-time algo- remaining variables under the observations. One of the draw- rithm, the problem of optimally learning polytrees that are backs of using Bayesian networks is that this inference task close to trees has been considered. More precisely, it is NP-hard. Moreover, the task of constructing a Bayesian has been shown that the best polytree among those that network with an optimal network structure is NP-hard as can be transformed into a tree by at most k edge dele- O(k) O(1) well, even on very restricted instances [Chickering, 1995]. tions can be foundin n |I| time [Gaspers et al., 2015; In this problem, we are given a local parent score func- Safaei et al., 2013] where n is the number of variables and |I| N\{v} is the overall input size. Thus, the running time of these algo- tion fv : 2 → N0 for each variable v and the task is to learn a DAG (N, A) such that the sum of the parent scores rithms is polynomial for every fixed k. As noted by Gaspers over all variables is maximal. et al. [2015], a brute-force algorithm for POLYTREE LEARN- ING would need to consider nn−2 · 2n−1 directed trees. Poly- ∗Supported by the Deutsche Forschungsgemeinschaft (DFG), trees have been used, for example, in image-segmentation for project OPERAH, KO 3669/5-1. microscopy data [Fehri et al., 2019]. A Our Results. We obtain an algorithm that solves POLY- that has no children is a sink.Given a vertex v, we let Pv := TREE LEARNING in 3n · |I|O(1) time. This is the first algo- {u | (u, v) ∈ A} denote the parent set of v. The skeleton rithm for POLYTREE LEARNING where the running time is of a directed graph (N, A) is the undirected graph (N, E) singly-exponential in the number of variables n. Therunning where {u, v} ∈ E if and only if (u, v) ∈ A or (v,u) ∈ time is a substantial improvement over the brute-force algo- A. A directed acyclic graph is a polytree if its skeleton is a rithm mentioned above, thus positively answering a question forest, that is, the skeleton is acyclic [Dasgupta, 1999]. Asa of Gaspers et al. [2015] on the existence of such algorithms. shorthand, we write P × v := P ×{v} for a vertex v and a We then study whether POLYTREE LEARNING is amenable set P ⊆ N. to polynomial-time data reduction that shrinks the instance I Problem Definition. Given a vertex set N, a family F := if |I| is much bigger than n. Using tools from parameter- {f : 2N\{v} → N | v ∈ N} is a family of local scores ized complexity theory, we show that such a data reduction v 0 for N. Intuitively, fv(P ) is the score that a vertex v obtains algorithm is unlikely. More precisely, we show that (un- if P is its parent set. Given a directed graph D := (N, A) we der standard complexity-theoretic assumptions) there is no A define score(A) := v∈N fv(Pv ). We study the following polynomial-time algorithm that replaces any n-variable in- computational problem. stance I by an equivalent one of size nO(1). In other words, P it seems necessary to keep an exponential number of parent POLYTREE LEARNING sets to compute the optimal polytree. Input: A set of vertices N, local scores F = {fv | v ∈ We then consider a parameter that is potentially much N}, and an integer t ∈ N0. Question smaller than n and determine whether POLYTREE LEARN- : Is there an arc-set A ⊆ N × N such ING can be solved efficiently when this parameter is small. that (N, A) is a polytree and score(A) ≥ t? The parameter d, whichwe callthenumberof dependent vari- Given an instance I := (N, F,t) of POLYTREE LEARN- ables, is the number of variables v for which at least one en- ING, an arc-set A is a solution of I if (N, A) is a try of fv is strictly positive. The parameter essentially counts polytree and score(A) ≥ t. Without loss of general- how many variables might receive a nonemptyparent set in an ity we may assume that fv(∅) = 0 for every v ∈ optimal solution. We show that POLYTREE LEARNING can N [Grüttemeier and Komusiewicz, 2020a]. Throughout this be solved in polynomial time when d is constant but that an work, we let n := |N|. O(1) algorithm with running time g(d) · |I| is unlikely for any Solution Structure and Input Representation. We as- computable function g. Consequently, in order to obtain pos- sume that the local scores F are given in non-zero represen- itive results for the parameter d, one needs to consider further tation. That is, f (P ) is part of the input if it is different restrictions on the structure of the input instance. We make a v from 0. For N = {v1,...,vn}, the local scores F are repre- first step in this direction and consider the case where all par- sented by a two-dimensional array [Q ,Q ,...,Q ], where ent sets with a strictly positive score have size at most p. Us- 1 2 n each Qi is an array containing all triples (fvi (P ), |P |, P ) ing this parameterization, we show that every input instance where f (P ) > 0. The size |F| is defined as the number ωdp O(1) vi can be solved in 2 ·|I| time where ω is the matrix mul- of bits needed to store this two-dimensional array. Given an tiplication constant. With the current-best known value for ω instance I := (N, F,t), we define |I| := n + |F| + log(t). dp O(1) this gives a running time of 5.18 · |I| . We then consider For a vertex v, we call PF (v) := {P ⊆ N \ {v} | again data reduction approaches. This time we obtain a posi- fv(P ) > 0} ∪ {∅} the set of potential parents of v. Given tive result: Any instance of POLYTREE LEARNING where p is a yes-instance I := (N, F,t) of POLYTREE LEARNING, constant can be reduced in polynomial time to an equivalent A there exists a solution A such that Pv ∈ PF (v) for ev- O(1) A one of size d . Informally, this means that if the instance ery v ∈ N: If there is a vertex with Pv 6∈ PF (v) has only few dependent variables, the parent sets with strictly we can simply replace its parent set by ∅.
Details
-
File Typepdf
-
Upload Time-
-
Content LanguagesEnglish
-
Upload UserAnonymous/Not logged-in
-
File Pages10 Page
-
File Size-