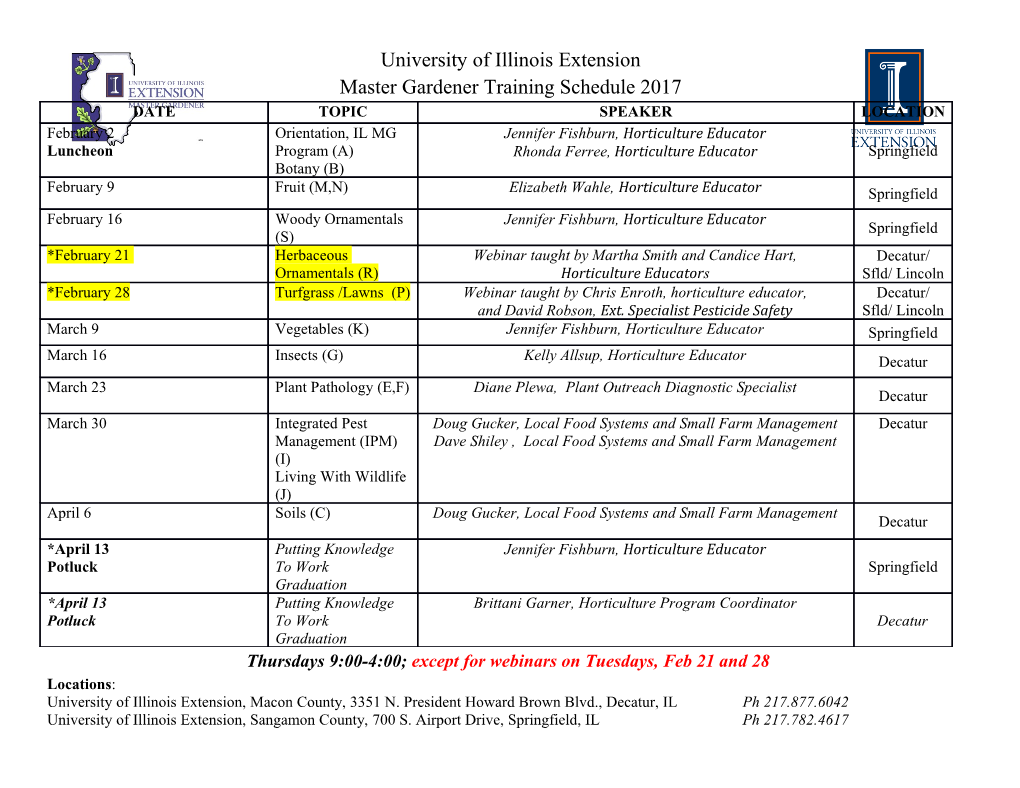
S S symmetry Article Copulaesque Versions of the Skew-Normal and Skew-Student Distributions Christopher Adcock Sheffield University Management School, University College Dublin, Sheffield S10 1FL, UK; c.j.adcock@sheffield.ac.uk Abstract: A recent paper presents an extension of the skew-normal distribution which is a copula. Under this model, the standardized marginal distributions are standard normal. The copula itself depends on the familiar skewing construction based on the normal distribution function. This paper is concerned with two topics. First, the paper presents a number of extensions of the skew-normal copula. Notably these include a case in which the standardized marginal distributions are Student’s t, with different degrees of freedom allowed for each margin. In this case the skewing function need not be the distribution function for Student’s t, but can depend on certain of the special functions. Secondly, several multivariate versions of the skew-normal copula model are presented. The paper contains several illustrative examples. Keywords: copula; multivariate distributions; skew-normal distributions; skew-Student distributions JEL Classification: C18; G01; G10; G12 Citation: Adcock, C.J. Copulaesque 1. Introduction Versions of the Skew-Normal and The recent paper by [1] presents an extension of the skew-normal distribution which Skew-Student Distributions. has subsequently been referred to as a copula. Under this model, the standardized marginal Symmetry 2021, 13, 815. https:// distributions are standard normal and some of the conditional distributions are skew- doi.org/10.3390/sym13050815 normal.The skew-normal distribution itself was introduced in two landmark papers [2] and [3]. These papers have led to a very substantial research effort by numerous authors Academic Editor: Nicola Maria over the last thirty five years. The result of these efforts include, but are certainly not Rinaldo Loperfido limited to, numerous probability distributions, both univariate and multivariate, which are loosely referred to in the literature as skew-elliptical distributions. This term does not Received: 14 April 2021 completely describe the rich features of these distributions, but for convenience will be Accepted: 27 April 2021 Published: 6 May 2021 used in this paper. Notable contributions to these developments include papers by [4–9] among numerous others. Publisher’s Note: MDPI stays neutral The many multivariate distributions that may be referred to as skew-elliptical offer with regard to jurisdictional claims in coherent probability models that are used in a wide variety of applications. However, they published maps and institutional affil- all share the feature that the factor which perturbs symmetry is applied as a multiplier to iations. a distribution that is elliptically symmetric. Consequently the marginal distributions are all prescribed. For example, the multivariate skew-t distribution described in [5] leads to marginal distributions that are all univariate skew-t distributions with the same degrees of freedom. While such a restriction may be acceptable or even irrelevant for some purposes, it is nonetheless the case that some multivariate applications have marginal distributions Copyright: © 2021 by the authors. Licensee MDPI, Basel, Switzerland. that are different. As is very well known, there is a large literature based on copulas This article is an open access article which derives from the original paper of [10]. The purpose of a copula is to separate the distributed under the terms and modeling of the dependence structure of set of variables from the analysis of the marginal conditions of the Creative Commons distributions. By implication, the marginal distributions need not be the same; that is, they Attribution (CC BY) license (https:// may differ by more than just scale and location. creativecommons.org/licenses/by/ In a recent paper concerned primarily with projection pursuit, ref [1] presents a 4.0/). trivariate distribution based on the skew-normal which is also in a general sense a copula. Symmetry 2021, 13, 815. https://doi.org/10.3390/sym13050815 https://www.mdpi.com/journal/symmetry Symmetry 2021, 13, 815 2 of 32 The adjective general is used to refer to the fact that although the distribution does not satisfy the theoretical requirements laid down in [10] there is nonetheless separation between the modeling of the dependence structure and the treatment of the marginal distributions. The skew-normal and skew-Student copulas as they are termed in this paper are amenable to theoretical study, at least to some extent. Loperfido’s model also leads naturally to various extensions, which are of theoretical interest and which have the potential to provide tools for empirical research. The purpose of this expository paper is to describe some of the properties of these new distributions and to present a number of extensions. As described below, these distributions are tractable to some extent, but numerous results of interest must be computed numerically. It is of particular interest to note that the some of the developments described in the paper are dependent on Meijer’s G function ([11]) and Fox’s H-function ([12]) and that there are therefore computational and methodological issues to be resolved. The structure of the paper is as follows: Section2 summarizes a basic bivariate skew-normal copula and presents some of its properties. The bivariate model of this section is used to illustrate the difference from a bivariate normal copula that does satisfy the conditions of in [10]. Section3 presents a conditional version of this distribution that has close connections to the original skew-normal distribution. Section4 presents an extended version of the distribution; that is a distribution that is analogous to the extended skew-normal. Section5 extends the results to an n-variate skew-normal copula and Section6 to Student versions. This distribution in particular allows the marginal distributions to have different degrees of freedom. Section7 presents results for a different multivariate setup. In this, a vector of m + n variables may be partitioned into components of length m and n each of which has a marginal multivariate normal distribution. The properties of this distribution are briefly discussed, including an outline of a multivariate Student version. Section8 presents three numerical examples. As this is an expository paper, several of the sections also contain brief discussions of technical issues that are outstanding and which could be the subject of future work. The final section of the paper contains a short summary. Many of the results in this paper require numerical integration. Example results are generally computed to four decimal places. The usual notation f and F are used to denote the standard normal density and distributions functions, respectively. To avoid proliferation of subscripts the notation f (.) is used indifferently to denote a density function. Other notation, if not defined explicitly in the text, is that in common use. 2. The Skew-Normal Distribution as a Copula The skew-normal copula was introduced by [1]. In their paper, the distribution is presented ariate form. Its principles may be demonstrated by a bivariate form of the distribution with probability density function f (x1, x2) = 2f(x1)f(x2)F(lx1x2). (1) The scalar parameter l 2 R determines the extent of the dependence between X1 and X2. In Section5, the bivariate distribution is extended to n variables. To accom- modate this extension it is convenient to employ the notation X ∼ SNCn(l), where T X = (X1, X2,..., Xn) . As [1] shows, in the bivariate case the marginal distribution of each Xi is N(0, 1) and the conditional distribution of X1 given that X2 = x2 is skew-normal with density function f (x1jX2 = x2) = 2f(x1)F(lx1x2). (2) There is an analogous expression for the density function of the conditional distribu- 2 tions of X2 given that X1 = x1. It may also be noted that X2 is distributed independently of 2 2 X1 as a c(1) variable, with the analogous result for the distributions of X2 and X1. Hence 2 = 2 the Xi , i 1, 2 are independently distributed as c(1). Symmetry 2021, 13, 815 3 of 32 To illustrate the difference from the formal definition of a copula, consider the well- known Gaussian copula. In the bivariate case, dependence between X1 and X2 is described by the function n −1 −1 o F2,C F (U1), F (U2) , where F2,Cf..g denotes the distribution function of a bivariate normal distribution with zero means and correlation matrix C and the U1,2 are each uniformly distributed on [0, 1]. The formulation at Equation (2) uses only the standard univariate normal distribution function. Sketches of the skew-normal density function for l 2 f−10.0, −2.6, 0.0, 10.0g are shown in Figure1. Sketches of the bivariate density function for l = 1.0 and −5.0 are shown in Figure2. The bi-modal nature of the density function when l = −5.0 is noteworthy.p Indeed it is straight forward to show that the density function is bi-modal if jlj > p/2, with the model values being at points ±(X, X) depending on l. Examples of modal values are shown in Table1 for a range of values of l. 0.9 0.8 0.7 0.6 0.5 0.4 0.3 0.2 0.1 0 -4.0 -3.0 -2.0 -1.0 0.0 1.0 2.0 3.0 4.0 -10 -2.6 0 10 The Figure shows plots of the standardized skew-normal density function for = -10.0, -2.6, 0.0 & 10. Figure 1. Skew-normal Density Functions; l = −10.0, −2.6, 0.0, 10.0. 1.80E-01 1.60E-01 1.60E-01 1.40E-01 1.40E-01 1.20E-01 1.20E-01 1.00E-01 1.00E-01 8.00E-02 8.00E-02 6.00E-02 6.00E-02 4.00E-02 4.00E-02 2.00E-02 2.00E-02 0.00E+00 0.00E+00 The Figures show plots of the bivariate skew-normal copula density function for - 4.0 <, x, y < 4.0 for = 1.0 and = -5.0.
Details
-
File Typepdf
-
Upload Time-
-
Content LanguagesEnglish
-
Upload UserAnonymous/Not logged-in
-
File Pages32 Page
-
File Size-