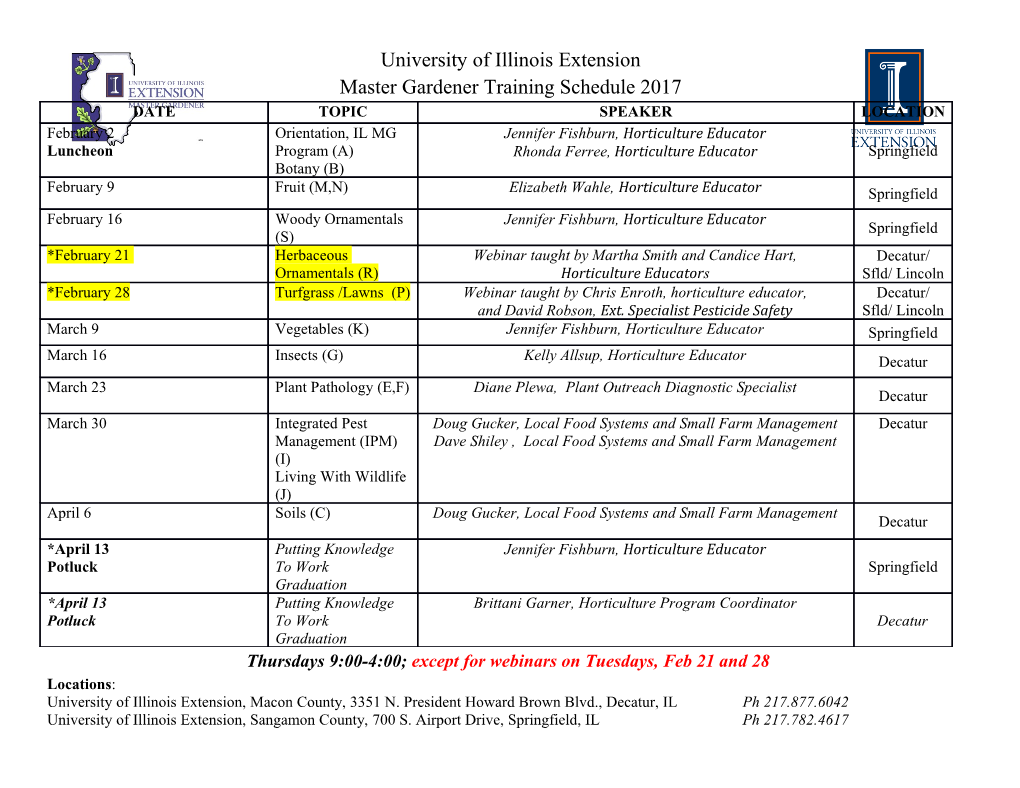
Social Media Stories. Event detection in heterogeneous streams of documents applied to the study of information spreading across social and news media Béatrice Mazoyer To cite this version: Béatrice Mazoyer. Social Media Stories. Event detection in heterogeneous streams of documents applied to the study of information spreading across social and news media. Social and Information Networks [cs.SI]. Université Paris-Saclay, 2020. English. NNT : 2020UPASC009. tel-02987720 HAL Id: tel-02987720 https://tel.archives-ouvertes.fr/tel-02987720 Submitted on 4 Nov 2020 HAL is a multi-disciplinary open access L’archive ouverte pluridisciplinaire HAL, est archive for the deposit and dissemination of sci- destinée au dépôt et à la diffusion de documents entific research documents, whether they are pub- scientifiques de niveau recherche, publiés ou non, lished or not. The documents may come from émanant des établissements d’enseignement et de teaching and research institutions in France or recherche français ou étrangers, des laboratoires abroad, or from public or private research centers. publics ou privés. Social Media Stories Event detection in heterogeneous streams of documents applied to the study of information spreading across social and news media Thèse de doctorat de l’Université Paris-Saclay École doctorale n◦ 573 : approches interdisciplinaires, fondements, applications et innovation (Interfaces) Spécialité de doctorat : Informatique Unité de recherche : Université Paris-Saclay, CentraleSupélec, Mathématiques et Informatique pour la Complexité et les Systèmes, 91190, Gif-sur-Yvette, France. Référent : CentraleSupélec Thèse présentée et soutenue à Paris, le 31 août 2020, par Béatrice MAZOYER Composition du jury: Julien Velcin Président Professeur, Université Lyon-2 Dominique Cardon Rapporteur et Examinateur Professeur, Sciences Po Paris Marianne Clausel Rapporteure et Examinatrice Professeur, Université de Lorraine Fabien Tarissan Examinateur Chargé de recherche, Ecole Normale Supérieure de Paris- Saclay Ekaterina Zhuravskaya Examinatrice Professeur, Paris School of Economics Céline Hudelot Directrice Professeur, CentraleSupélec Julia Cagé Co-directrice Maître de Conférences (HDR), Sciences Po Paris Nicolas Hervé Co-directeur Chercheur, Institut National de l’Audiovisuel NNT: 2020UPASC009 Thèse de doctorat de Thèse En mémoire de Marie-Luce Viaud Acknowledgements First of all, I would like to solemnly thank the members of my jury who agreed to evaluate my work. In particular, I would like to thank Marianne Clausel and Dominique Cardon for agreeing to report my thesis in this very particular context of lockdown, where no one knew exactly what their schedule would be in the coming months. Thank you to you, as well as to Ekaterina Zhuravskaya, Fabien Tarissan and Julien Velcin for taking part in my thesis defence on August 31, a date on which everyone would undoubtedly have liked to enjoy the summer a little longer. My thanks go then to my thesis director, Céline Hudelot, and my co-directors, Julia Cagé and Nicolas Hervé. Céline, thank you for agreeing to supervise this bi-disciplinary thesis, which probably gave you more work than a thesis entirely in your discipline. Thank you also for taking the time come to INA, or to meet me at Centrale or in Paris. Finally, thank you for your methodological advice, your scientific rigour and your careful rereading. Julia, thank you for your energy and kindness, which encouraged me during the last months of my thesis. Thank you for having welcomed me in your office so often, and for having sometimes left me the keys. Finally, thank you for your hard work to help me make sense of the data. Nicolas, thank you for having been my daily contact at INA, for having answered all my questions without ever getting impatient, and for having made the completion of my thesis your priority among all your responsibilities. You made sure that I always had the space I needed to store my tweets, and the computing power to index and analyse them, and you were always concerned about my working conditions. I would also like to add a word of thanks to Marie-Luce Viaud, who came up with the idea for this thesis and was my co-director until her death. I think we all still miss her luminous intelligence and humour, and I wish she could have been there to see this project through. I was fortunate during my thesis to be able to count on exceptional colleagues. Thanks to Pierre Letessier for his technical advice, on Docker, Elasticsearch or nearest neighbor search, and to Zeynep Pehlivan who gave me the benefit of her long experience in collecting tweets. Thanks to Laurent Cabaret i ii for his advice on Python optimization. I would also like to thank Agnès Saulnier, for all the times she drove me home from the INA, and never complained that I talked to her about my thesis. The same thanks goes to David Doukhan, who was leaving even later than Agnès. Thanks to Jean-Etienne Noiré, Marc Evrard and Thomas Petit, who helped to make my lunch breaks funnier. Finally, a thought for Haolin Ren, who shared my office for many months, and has now returned to China. I also had the unconditional support of my family during these three years. I am extremely grateful to my parents, Martine Créac’h and Bernard Mazoyer, for always believing in my qualities, which gave me the strength to carry out this long work. Thanks to my father for his enormous work in correcting all my articles and this thesis manuscript, and to my brothers, Johan and Simon Mazoyer, who took the time to discuss with me and to share with me their own experience of the thesis. A huge thank you also to my parents-in-law, Martine Avenel-Audran and Maurice Audran, who welcomed me in their home throughout the confinement and made the final writing period much simpler. Finally, I would like to thank Martin for his patience and tenderness, and for all the happiness I owe him. Contents List of Figures 7 List of Tables 9 1 Introduction 13 1.1 Objective . 13 1.2 Scope of the study . 14 1.3 Empirical challenges . 17 1.4 Detailed Outline . 20 2 Building a corpus for event detection on Twitter 23 2.1 Introduction . 23 2.1.1 Representativity and completeness of the collected data . 23 2.1.2 Quality of the annotated subset . 25 2.2 State of the art: event detection corpora . 25 2.3 Tweet collection . 28 2.3.1 Constraints . 28 2.3.2 Proposed collection strategy . 30 2.3.3 Experimental setup . 31 2.3.4 Evaluation of the collection strategy . 32 2.3.5 Evaluate the share of collected tweets . 33 2.4 Tweet annotation . 37 2.4.1 Media event selection . 37 2.4.2 Twitter events selection . 38 1 2 CONTENTS 2.4.3 Annotation procedure . 39 2.5 Evaluation of the created corpus . 42 2.5.1 Corpus characteristics . 42 2.5.2 Intra-event diversity . 43 2.5.3 Annotator agreement . 44 2.6 Conclusion . 46 3 Detecting Twitter events 47 3.1 Introduction . 47 3.2 State of the art . 49 3.2.1 Twitter event detection approaches . 49 3.2.2 Text embeddings . 57 3.2.3 Text-Image event detection . 59 3.3 Our event detection approach . 61 3.3.1 Requirements . 61 3.3.2 Modified First Story Detection algorithm . 62 3.4 Other tested approaches . 65 3.4.1 Support Vector Machines (SVM) . 65 3.4.2 DBSCAN . 66 3.4.3 Dirichlet Multinomial Mixture (DMM) model . 66 3.5 Experimental setup . 66 3.5.1 Choice of parameters . 66 3.5.2 Types of representations . 69 3.5.3 Evaluation metrics . 75 3.6 Results . 76 3.6.1 Comparison of text embeddings . 76 3.6.2 Comparison of text-image embeddings . 80 3.6.3 Comparison of event detection methods . 81 3.6.4 Results on the entire collection . 82 3.7 Conclusion . 83 CONTENTS 3 4 Linking Media events and Twitter events 85 4.1 Introduction . 85 4.2 Related Work . 86 4.2.1 Social content alignment . 86 4.2.2 Matching tweet-article pairs . 86 4.2.3 Social content retrieval . 87 4.2.4 Joint event detection . 88 4.3 Methodology . 90 4.3.1 First Story Detection . 90 4.3.2 Event-similarity graph . 91 4.3.3 Community detection . 93 4.4 Experimental Setup . 95 4.4.1 Dataset . 95 4.4.2 Evaluation metric . 96 4.4.3 Parameter tuning . 96 4.5 Results . 96 4.5.1 Effect of word similarity, URLs and hashtags . 97 4.5.2 Effect of the minimum cosine similarity between 2 events (s).............. 97 4.5.3 Effect of the maximum time distance between 2 events (∆).............. 97 4.5.4 Results on the entire corpus . 100 4.6 Conclusion . 100 5 Social media and newsroom production decisions 101 5.1 Introduction . ..
Details
-
File Typepdf
-
Upload Time-
-
Content LanguagesEnglish
-
Upload UserAnonymous/Not logged-in
-
File Pages215 Page
-
File Size-