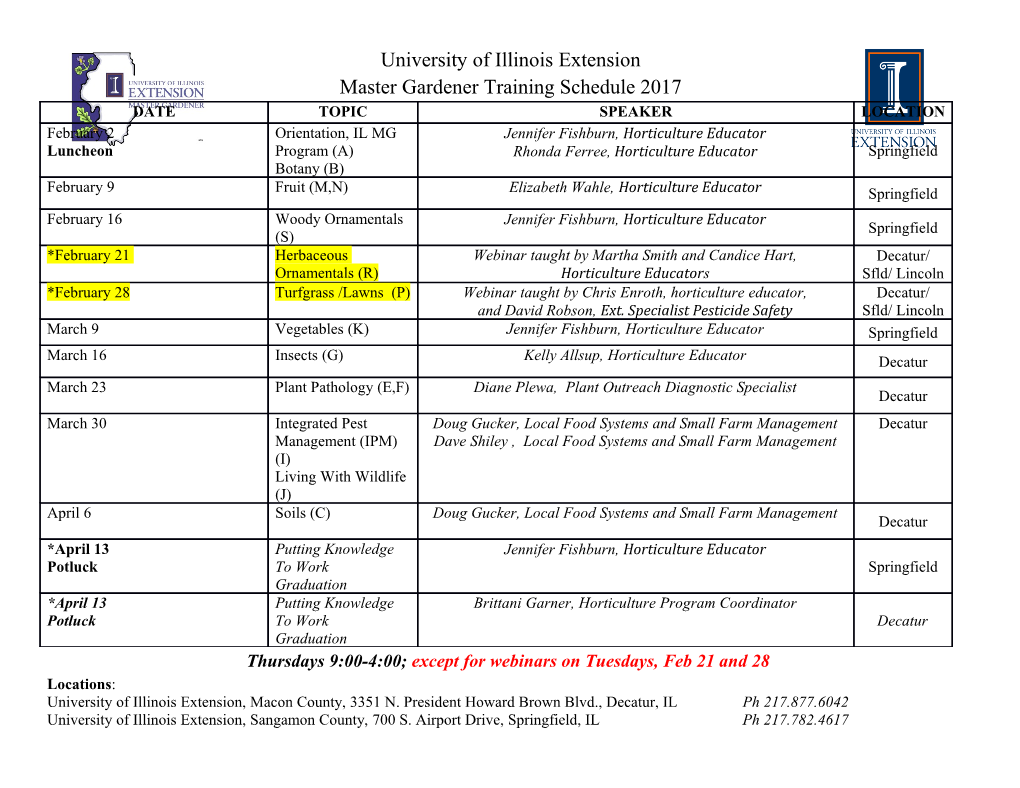
Machine Learning Srihari Discrete Probability Distributions Sargur N. Srihari 1 Machine Learning Srihari Binary Variables Bernoulli, Binomial and Beta 2 Machine Learning Srihari Bernoulli Distribution • Expresses distribution of Single binary-valued random variable x ε {0,1} • Probability of x=1 is denoted by parameter µ, i.e., p(x=1|µ)=µ – Therefore p(x=0|µ)=1-µ • Probability distribution has the form Bern(x|µ)=µ x (1-µ) 1-x • Mean is shown to be E[x]=µ Jacob Bernoulli • Variance is var[x]=µ (1-µ) 1654-1705 • Likelihood of n observations independently drawn from p(x|µ) is N N x 1−x p(D | ) = p(x | ) = n (1− ) n µ ∏ n µ ∏µ µ n=1 n=1 – Log-likelihood is N N ln p(D | ) = ln p(x | ) = {x ln +(1−x )ln(1− )} µ ∑ n µ ∑ n µ n µ n=1 n=1 • Maximum likelihood estimator 1 N – obtained by setting derivative of ln p(D|µ) wrt m equal to zero is = x µML ∑ n N n=1 • If no of observations of x=1 is m then µML=m/N 3 Machine Learning Srihari Binomial Distribution • Related to Bernoulli distribution • Expresses Distribution of m – No of observations for which x=1 • It is proportional to Bern(x|µ) Histogram of Binomial for • Add up all ways of obtaining heads N=10 and ⎛ ⎞ m=0.25 ⎜ N ⎟ m N−m Bin(m | N,µ) = ⎜ ⎟µ (1− µ) ⎝⎜ m ⎠⎟ Binomial Coefficients: ⎛ ⎞ N ! ⎜ N ⎟ • Mean and Variance are ⎜ ⎟ = ⎝⎜ m ⎠⎟ m!(N −m)! N E[m] = ∑mBin(m | N,µ) = Nµ m=0 Var[m] = N (1− ) 4 µ µ Machine Learning Srihari Beta Distribution • Beta distribution a=0.1, b=0.1 a=1, b=1 Γ(a + b) Beta(µ |a,b) = µa−1(1 − µ)b−1 Γ(a)Γ(b) • Where the Gamma function is defined as ∞ Γ(x) = ∫u x−1e−u du a=2, b=3 0 a=8, b=4 • a and b are hyperparameters that control distribution of parameter µ • Mean and Variance Beta distribution as function of µ a ab E [µ] = var[µ] = For values of hyperparameters a and b a + b (a + b)2 (a + b +1) 5 Machine Learning Srihari Bayesian Inference with Beta • MLE of µ in Bernoulli is fraction of observations with x=1 – Severely over-fitted for small data sets • Likelihood function takes products of factors of the form µx(1-µ)(1-x) • If prior distribution of µ is chosen to be proportional to powers of µ and 1-µ, posterior will have same functional form as the prior – Called conjugacy • Beta has form suitable for a prior distribution of p(µ) 6 Machine Learning Srihari Bayesian Inference with Beta Illustration of one step in process • Posterior obtained by multiplying beta a=2, b=2 prior with binomial likelihood yields p(µ) p(µ | m,l,a,b) α µ m+a−1(1− µ)l+b−1 – where l=N-m, which is no of tails N=m=1, with x=1 p(x=1/µ)= – m is no of heads µ1(1-µ)0 • It is another beta distribution Γ(m + a + l + b) p(µ | m,l,a,b) = µ m+a−1(1− µ)l+b−1 Γ(m + a)Γ(l + b) a=3, b=2 – Effectively increase value of a by m and b by l p(µ/x=1) – As number of observations increases distribution becomes more peaked 7 Machine Learning Srihari Predicting next trial outcome • Need predictive distribution of x given observed D – From sum and products rule 1 1 p(x = 1| D) = p(x = 1, µ | D)dµ = p(x = 1| µ)p(µ | D)dµ = ∫0 ∫0 1 = µp(µ | D)dµ = E[µ | D] ∫0 • Expected value of the posterior distribution can be shown to be m + a p(x = 1| D) = m + a + l + b – Which is fraction of observations (both fictitious and real) that correspond to x=1 • Maximum likelihood and Bayesian results agree in the limit of infinite observations – On average uncertainty (variance) decreases with observed data 8 Machine Learning Srihari Summary of Binary Distributions • Single Binary variable distribution is represented by Bernoulli • Binomial is related to Bernoulli – Expresses distribution of number of occurrences of either 1 or 0 in N trials • Beta distribution is a conjugate prior for Bernoulli – Both have the same functional form 9 Machine Learning Srihari Sample Matlab Code Probability Distributions • Binomial Distribution: – Probability Density Function : Y = binopdf (X,N,P) returns the binomial probability density function with parameters N and P at the values in X. – Random Number Generator: R = binornd (N,P,MM,NN) returns n MM-by-NN matrix of random numbers chosen from a binomial distribution with parameters N and P • Beta Distribution – Probability Density Function : Y = betapdf (X,A,B) returns the beta probability density function with parameters A and B at the values in X. – Random Number Generator: R = betarnd (A,B) returns a matrix of random numbers chosen from the beta distribution with parameters A and B. Machine Learning Srihari Multinomial Variables Generalized Bernoulli and Dirichlet 11 Machine Learning Srihari Generalization of Binomial Histogram of Binomial for • Binomial N=10 and µ=0.25 – Tossing a coin – Expresses probability of no of successes in N trials • Probability of 3 rainy days in 10 days • Multinomial – Throwing a Die – Probability of a given frequency for each value • Probability of 3 specific letters in a string of N • Probability Calculator – http://stattrek.com/Tables/Multinomial.aspx 12 Machine Learning Srihari Generalized Bernoulli: Multinoulli • Bernoulli distribution x is 0 or 1 Bern(x|µ)=µ x (1-µ) 1-x • Discrete variable that takes one of K values (instead of 2) – Represent as 1-of-K scheme • Represent x as a K-dimensional vector • If x=3 then we represent it as x=(0,0,1,0,0,0)T K – Such vectors satisfy x = 1 ∑k=1 k – If probability of xk=1 is denoted µk then distribution of x is given by K x T p( | ) = k where = ( ,.., ) Generalized Bernoulli x µ ∏µk µ µ1 µK k=1 13 Machine Learning Srihari MLE of Multinoulli Parameters • Data set D of N indep observations x1,..xN th • where the n observation is written as [xn1,.., xnK] • Likelihood function has the form N K K K x x ∑n nk m p(D | ) nk ( ) k µ = ∏∏ µk = ∏ µk = ∏ µk n=1 k=1 k=1 k=1 • where mk=Σn xnk is the no. of observations of xk=1 • Maximum likelihood solution – Maximize ln p(D|µ) with Lagrangian constraint that the µk must sum to one, i.e., maximize K ⎛ K ⎞ mk ln µk + λ µk − 1 m µML = k ∑k ⎜ ∑ ⎟ k= 1N ⎝ k=1 ⎠ • Setting derivative wrt µ to zero k ML mk µk = N – which is fraction of N observations for which xk=1 14 Machine Learning Srihari Generalized Binomial Distribution • Multinomial distribution (with K-state variable) K ⎛ N ⎞ m Mult m m ..m | µ,N = µ k ( 1 2 K ) ⎜ m m ..m ⎟ ∏ k ⎝ 1 2 k ⎠ k=1 µ = 1 ∑k k – Where the normalization coefficient is the no of ways of partitioning N objects into K groups of size m ,m ..m 1 2 k • Given by ⎛ N ⎞ N ! = ⎜ m m ..m ⎟ ⎝ 1 2 k ⎠ m1 !m2 !..mk ! 15 Machine Learning Srihari Dirichlet Distribution Lejeune Dirichlet 1805-1859 • Family of prior distributions for parameters µk of multinomial distribution • By inspection of multinomial, form of conjugate prior is K α −1 p(µ | α) α µ k where 0 ≤ µ ≤ 1 and µ = 1 ∏ k k ∑k k k=1 • Normalized form of Dirichlet distribution K K Γ(α ) α −1 Dir(µ | α) = 0 µ k where α = α ∏ k 0 ∑ k Γ(α )...Γ(α ) k=1 k=1 1 k 16 Machine Learning Srihari Dirichlet over 3 variables αk=0.1 • Due to summation µ =1 constraint ∑k k – Distribution over Plots of Dirichlet =1 space of { } is distribution over the αk µk simplex for various confined to the settings of parameters simplex of αk dimensionality K-1 3 3 Γ(α ) α −1 =10 Dir(µ | α) = 0 µ k where α = α αk ∏ k 0 ∑ k – For K=3 Γ(α )...Γ(α ) k=1 k=1 1 3 17 Machine Learning Srihari Dirichlet Posterior Distribution • Multiplying prior by likelihood K p( | D, ) p(D | ) p( | ) αk +mk −1 µ α α µ µ α α∏ µk k =1 • Which has the form of the Dirichlet distribution p(µ | D,α) = Dir(µ |α + m) Γ(α + N) K 0 αk +mk −1 = ∏ µk Γ(α1 + m1)..Γ(α K + mK ) k =1 18 Machine Learning Srihari Summary of Discrete Distributions • Bernoulli (2 states) : Bern(x|µ)=µ x (1-µ) 1-x ⎛ ⎞ ⎛ N ⎞ m N −m ⎜ N ⎟ N ! – Binomial: Bin(m | N, µ) = ⎜ ⎟µ (1− µ) ⎜ ⎟ = ⎜ ⎟ ⎝⎜ m ⎠⎟ m!(N −m)! ⎝ m ⎠ • Generalized Bernoulli (K states): K p(x | µ) xk where µ ( ,.., )T = ∏ µk = µ1 µK k =1 ⎛ N ⎞ K Mult m m ..m | , N mk – Multinomial ( 1 2 K µ ) = ⎜ ⎟∏ µk ⎝m1m2..mk ⎠ k =1 • Conjugate priors: – Binomial is Beta Γ(a + b) Beta(µ | a,b) = µ a−1(1− µ)b−1 Γ(a)Γ(b) – Multinomial is Dirichlet Γ(α ) K K Dir(µ |α) = 0 µαk −1 where α = α ∏ k 0 ∑ k 19 Γ(α1)...Γ(α k ) k =1 k =1 Machine Learning Srihari Distributions: Landscape Discrete- Binary Bernoulli Binomial Beta Discrete- Multivalued Multinomial Dirichlet Continuous Gaussian Wishart Student’s-t Gamma Exponential Angular Von Mises Uniform 20 Machine Learning Srihari Distributions: Relationships Discrete- Conjugate Binary N=1 Prior Beta Binomial Bernoulli Continuous variable N samples of Bernoulli Single binary variable between {0,1] K=2 Discrete- Multinomial Large Conjugate Prior Multi-valued One of K values = Dirichlet N K-dimensional K random variables binary vector between [0.1] Continuous Student’s-t Gamma Wishart Exponential Generalization of ConjugatePrior of univariate Conjugate Prior of multivariate Special case of Gamma Gaussian robust to Gaussian precision Gaussian precision matrix Gaussian Outliers Infinite mixture of Gaussians Gaussian-Gamma Gaussian-Wishart Conjugate prior of univariate Gaussian Conjugate prior of multi-variate Gaussian Unknown mean and precision Unknown mean and precision matrix Angular Von Mises Uniform 21 .
Details
-
File Typepdf
-
Upload Time-
-
Content LanguagesEnglish
-
Upload UserAnonymous/Not logged-in
-
File Pages21 Page
-
File Size-