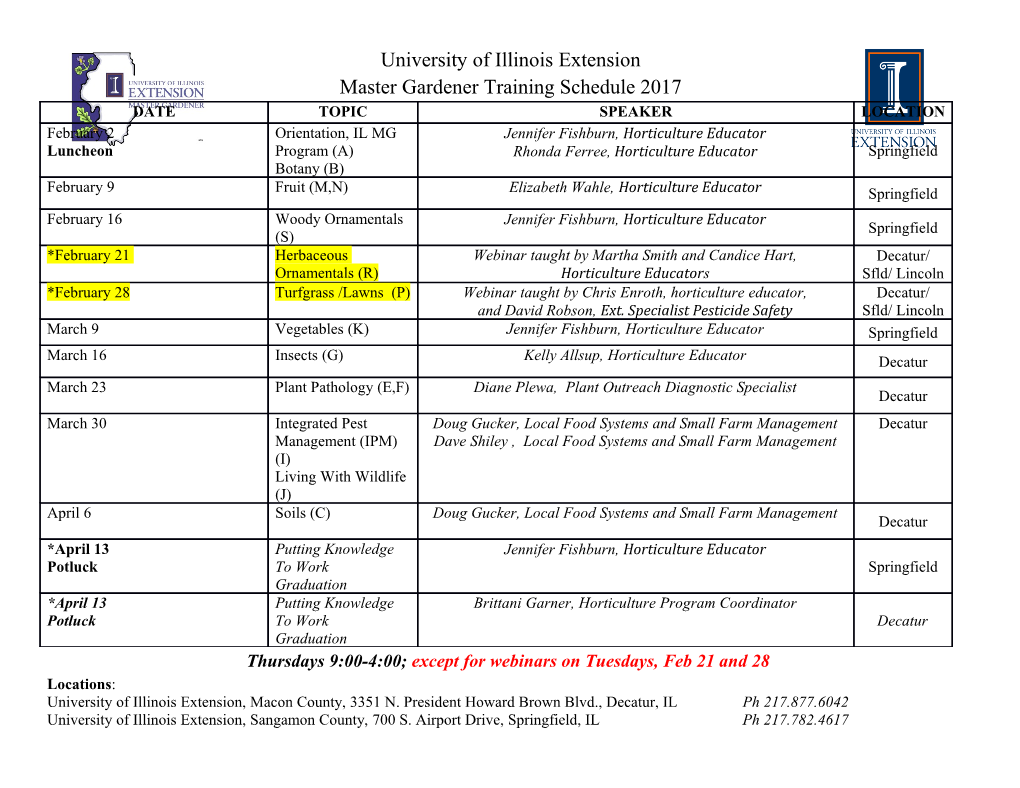
Effective search space reduction for spell correction using character neural embeddings Harshit Pande Smart Equipment Solutions Group Samsung Semiconductor India R&D Bengaluru, India [email protected] Abstract of distance computations blows up the time com- plexity, thus hindering real-time spell correction. We present a novel, unsupervised, and dis- For Damerau-Levenshtein distance or similar edit tance measure agnostic method for search distance-based measures, some approaches have space reduction in spell correction using been tried to reduce the time complexity of spell neural character embeddings. The embed- correction. Norvig (2007) does not check against dings are learned by skip-gram word2vec all dictionary words, instead generates all possi- training on sequences generated from dic- ble words till a certain edit distance threshold from tionary words in a phonetic information- the misspelled word. Then each of such generated retentive manner. We report a very high words is checked in the dictionary for existence, performance in terms of both success rates and if it is found in the dictionary, it becomes a and reduction of search space on the Birk- potentially correct spelling. There are two short- beck spelling error corpus. To the best of comings of this approach. First, such search space our knowledge, this is the first application reduction works only for edit distance-based mea- of word2vec to spell correction. sures. Second, this approach too leads to high time complexity when the edit distance threshold is 1 Introduction greater than 2 and the possible characters are large. Spell correction is now a pervasive feature, with Large character set is real for Unicode characters presence in a wide range of applications such used in may Asian languages. Hulden (2009) pro- as word processors, browsers, search engines, poses a Finite-State-Automata (FSA) algorithm OCR tools, etc. A spell corrector often re- for fast approximate string matching to find sim- lies on a dictionary, which contains correctly ilarity between a dictionary word and a misspelled spelled words, against which spelling mistakes word. There have been other approaches as well are checked and corrected. Usually a measure using FSA, but such FSA-based approaches are of distance is used to find how close a dictio- approximate methods for finding closest match- nary word is to a given misspelled word. One ing word to a misspelled word. Another more re- popular approach to spell correction is the use of cent approach to reduce the average number of dis- Damerau-Levenshtein distance (Damerau, 1964; tance computations is based on anomalous pattern Levenshtein, 1966; Bard, 2007) in a noisy chan- initialization and partition around medoids (de nel model (Norvig, 2007; Norvig, 2009). For Amorim and Zampieri, 2013). huge dictionaries, Damerau-Levenshtein distance In this paper, we propose a novel, unsuper- computations between a misspelled word and all vised, distance measure agnostic, highly accurate, dictionary words lead to long computation times. method of search space reduction for spell cor- For instance, Korean and Japanese may have as rection with a high reduction ratio. Our method many as 0.5 million words1. A dictionary fur- is unsupervised because we use only a dictionary ther grows when inflections of the words are also of correctly spelled words during the training pro- considered. In such cases, since an entire dic- cess. It is distance measure agnostic because once tionary becomes the search space, large number the search space has been reduced then any dis- 1http://www.lingholic.com/how-many-words-do-i-need- tance measure of spell correction can be used. It to-know-the-955-rule-in-language-learning-part-2/ is novel because to the best of our knowledge, it 170 Proceedings of the 15th Conference of the European Chapter of the Association for Computational Linguistics: Volume 2, Short Papers, pages 170–174, Valencia, Spain, April 3-7, 2017. c 2017 Association for Computational Linguistics is the first application of neural embeddings learn- along with the inherent differences in approach ing word2vec techniques (Mikolov et al., 2013a; render their method and our method not entirely Mikolov et al., 2013b) to spell correction. The comparable. goal of this paper is not to find a novel spell cor- rection algorithm. Rather, the goal is to reduce the 3 Method time complexity of spell correction by reducing Recent word2vec techniques (Mikolov et al., the search space of words over which the search 2013a; Mikolov et al., 2013b) have been very ef- for correct spelling is to be done. The reduced fective for representing symbols such as words in search space contains only a fraction of words of n an n-dimensional space R by using information the entire dictionary, and we refer to that fraction from the context of the symbols. These vectors are as reduction ratio. So, our method is used as a also called neural embeddings because of the one filter before a spell correction algorithm. We dis- hidden layer neural network architecture used to cuss a closely related work in Section 2, which is learn these vectors. In our method, the main idea followed by description of our method in Section is to represent dictionary words as n-dimensional 3. Then we present our experiments and results in vectors, such that with high likelihood the vec- Section 4, which demonstrates the effectiveness of tor representation of the correct spelling of a mis- our approach. spelled word is in the neighborhood of the vector representation of the misspelled word. To quickly 2 Related Work explore the neighborhood of the misspelled word As discussed in Section 1, there have been studies vector, fast k-nearest-neighbor (k-NN) search is to reduce the time complexity of spell correction done using a Ball Tree (Omohundro, 1989; Liu et by various methods. However, the recent work of al., 2006; Kibriya and Frank, 2007). A Ball Tree de Amorim and Zampieri (2013) is closest to our (aka Metric Tree) retrieves k-nearest-neighbors of work in terms of the goal of the study. We briefly a point in time complexity that is logarithmic of describe their method and evaluation measure, as the total number of points (Kibriya and Frank, it would help us in comparing our results to theirs, 2007). There are other methods, such as Locally- though the results are not exactly comparable. Sensitive Hashing (LSH) and KD-Tree, which can De Amorim and Zampieri (2013) cluster a dic- also be used to perform fast k-NN search. We use tionary based on anomalous pattern initialization Ball Tree because in our experiments, Ball Tree and partition around medoids, where medoids be- outperforms both KD-Tree and LSH in terms of come the representative words of the clusters and speed of computation. the candidacy of a good cluster is determined by We treat a word as a bag of characters. For each computing the distance between the misspelled character, an n-dimensional vector representation word and the medoid word. This helps in reduc- is learned using all the words from a dictionary of ing the average number of distance computations. correctly spelled words. Each word is then repre- Then all the words belonging to the selected clus- sented as an n-dimensional vector formed by sum- ters become candidates for further distance com- ming up the vectors of the characters in that word. putations. Their method on average needs to per- Then in a similar manner, a vector is obtained for form 3,251.4 distance calculations for a dictionary the misspelled word by summing up the vectors of 57,046 words. This amounts to 0.057 reduction of the characters in the misspelled word. We start ratio. They also report a success rate of 88.42% with a few notations: on a test data set known as Birkbeck spelling er- • n : dimension of neural embedding ror corpus.2 However, it is important to note that they define success rate in a rather relaxed manner • m : window size for word2vec training - one of the selected clusters contains either the • W : set of all dictionary words correct spelling or contains a word with a smaller • w : input misspelled word distance to the misspelled word than the correct • k : size of the reduced search space word. Later in Section 4, we define a stricter and • C : set of all the language characters present natural definition of success rate for our studies. in W This difference in relaxed vs strict success rates • C2V map : a map of all characters in C to 2http://www.dcs.bbk.ac.uk/ ROGER/corpora.html their n-dimensional vector representations 171 • V 2W map : a map of vectors to the list of vowels or consonants but not both, then it retains words represented by the vectors3 phonetic information of a word. Phonetic infor- • BT : a Ball Tree of all the vectors mation is vital for correcting spelling mistakes. Our method is divided into two procedures. 3.2 Procedure 2: search space reduction The first procedure is a preprocessing step, which 1. Compute vw, the n-dimensional vector repre- needs to be done only once, and the second proce- sentation of misspelled word w, by summing dure is the search space reduction step. up the vector representations of the characters in w (using C2V map). 3.1 Procedure 1: preprocessing 1. Prepare sequences for word2vec training: 2. Find kNearNeighb : k nearest-neighbors of each word w0 in W is split into a sequence vw using BT . such that each symbol of such sequence con- tains the longest possible contiguous vowels4 3. Using V 2W map fetch the reduced search or consonants but not both. E.g. “affiliates” space of words corresponding to each vector generates the sequence “a ff i l ia t e s” in kNearestNeighb 2.
Details
-
File Typepdf
-
Upload Time-
-
Content LanguagesEnglish
-
Upload UserAnonymous/Not logged-in
-
File Pages5 Page
-
File Size-