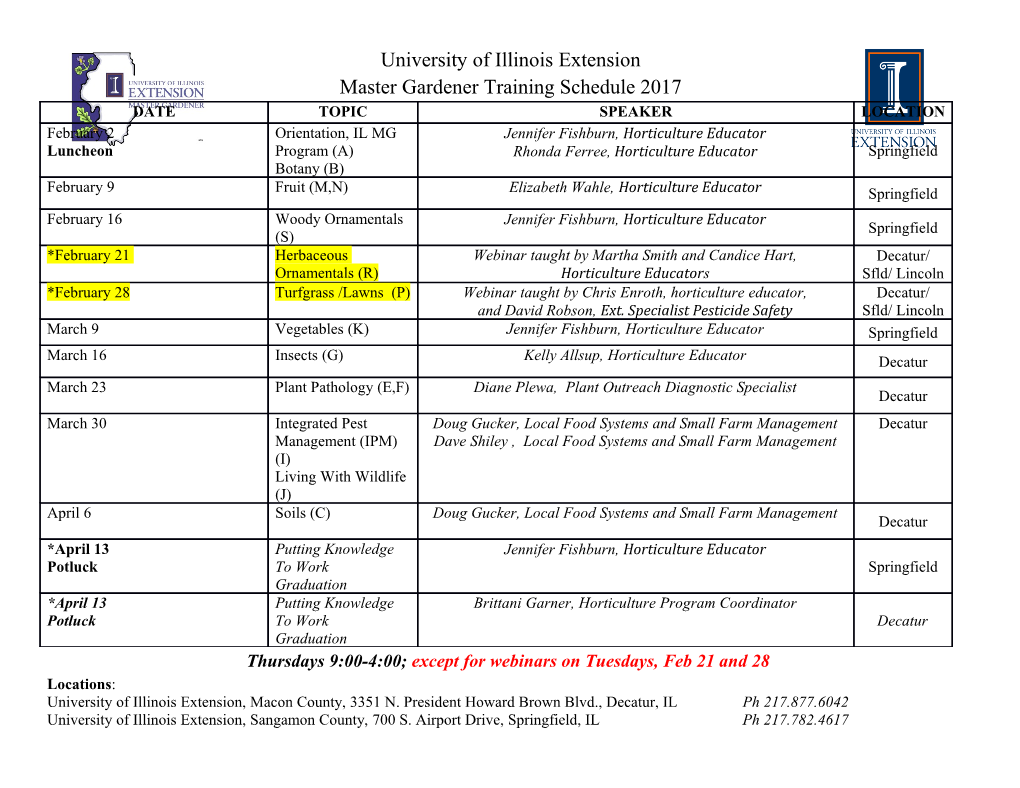
Advanced Algorithms Computer Science, ETH Z¨urich Mohsen Ghaffari These notes will be updated regularly. Please read critically; there are typos throughout, but there might also be mistakes. Feedback and comments would be greatly appreciated and should be emailed to ghaff[email protected]. Last update: March 10, 2020 Contents Notation and useful inequalities I Approximation algorithms1 1 Greedy algorithms3 1.1 Minimum set cover........................3 2 Approximation schemes 11 2.1 Knapsack............................. 12 2.2 Bin packing............................ 14 2.3 Minimum makespan scheduling................. 20 3 Randomized approximation schemes 27 3.1 DNF counting........................... 27 3.2 Counting graph colorings..................... 32 4 Rounding ILPs 37 4.1 Minimum set cover........................ 38 4.2 Minimizing congestion in multi-commodity routing...... 43 5 Probabilistic tree embedding 51 5.1 A tight probabilistic tree embedding construction....... 52 5.2 Application: Buy-at-bulk network design............ 60 5.3 Extra: Ball carving with O(log n) stretch factor........ 61 II Streaming and sketching algorithms 65 6 Warm up 67 6.1 Typical tricks........................... 67 6.2 Majority element......................... 68 7 Estimating the moments of a stream 71 7.1 Estimating the first moment of a stream............ 71 7.2 Estimating the zeroth moment of a stream........... 73 7.3 Estimating the kth moment of a stream............. 80 8 Graph sketching 89 8.1 Warm up: Finding the single cut................ 90 8.2 Warm up 2: Finding one out of k > 1 cut edges........ 92 8.3 Maximal forest with O(n log4 n) memory............ 93 III Graph sparsification 97 9 Preserving distances 99 9.1 α-multiplicative spanners..................... 100 9.2 β-additive spanners........................ 102 10 Preserving cuts 111 10.1 Warm up: G = Kn ........................ 113 10.2 Uniform edge sampling...................... 113 10.3 Non-uniform edge sampling................... 115 IV Online algorithms and competitive analysis 121 11 Warm up: Ski rental 123 12 Linear search 125 12.1 Amortized analysis........................ 125 12.2 Move-to-Front........................... 126 13 Paging 129 13.1 Types of adversaries....................... 130 13.2 Random Marking Algorithm (RMA).............. 131 14 Yao's Minimax Principle 135 14.1 Application to the paging problem............... 136 15 The k-server problem 137 15.1 Special case: Points on a line.................. 138 16 Multiplicative Weights Update (MWU) 143 16.1 Warm up: Perfect expert exists................. 144 16.2 A deterministic MWU algorithm................ 144 16.3 A randomized MWU algorithm................. 145 16.4 Generalization........................... 147 16.5 Application: Online routing of virtual circuits......... 147 Notation and useful inequalities Commonly used notation •P : class of decision problems that can be solved on a deterministic sequential machine in polynomial time with respect to input size •NP : class of decision problems that can be solved non-deterministically in polynomial time with respect to input size. That is, decision prob- lems for which \yes" instances have a proof that can be verified in polynomial time. •A : usually denotes the algorithm we are discussing about •I : usually denotes a problem instance • ind.: independent / independently • w.p.: with probability • w.h.p: with high probability We say event X holds with high probability (w.h.p.) if 1 Pr[X] ≥ 1 − poly(n) 1 say, Pr[X] ≥ 1 − nc for some constant c ≥ 2. • L.o.E.: linearity of expectation • u.a.r.: uniformly at random • Integer range [n] = f1; : : : ; ng • e ≈ 2:718281828459: the base of the natural logarithm Useful distributions Bernoulli Coin flip w.p. p. Useful for indicators Pr[X = 1] = p E[X] = p Var(X) = p(1 − p) Binomial Number of successes out of n trials, each succeeding w.p. p; Sample with replacement out of n items, p of which are successes n Pr[X = k] = pk(1 − p)n−k k E[X] = np Var(X) = np(1 − p) ≤ np Geometric Number of Bernoulli trials until one success Pr[X = k] = (1 − p)n−1p 1 [X] = E p 1 − p Var(X) = p2 Hypergeometric r successes in n draws without replacement when there are K successful items in total Kn−k r r−k Pr[X = k] = n r k [X] = r · E n k n − k n − r Var(X) = r · · · n n n − 1 Exponential Parameter: λ; Written as X ∼ Exp(λ) ( λe−λx if x ≥ 0 Pr[X = x] = 0 if x < 0 1 [X] = E λ 1 Var(X) = λ2 Remark If x1 ∼ Exp(λ1); : : : ; xn ∼ Exp(λn), then • minfx1; : : : ; xng ∼ Exp(λ1 + ··· + λn) λk • Pr[k j xk = minfx1; : : : ; xng] = λ1+···+λn Useful inequalities n k n en k • ( k ) ≤ k ≤ ( k ) n k • k ≤ n 1 n −1 • limn!1(1 − n ) = e P1 1 π2 • i=1 i2 = 6 • (1 − x) ≤ e−x, for any x • (1 + 2x) ≥ ex, for x 2 [0; 1] x x • (1 + 2 ) ≥ e , for x 2 [−1; 0] −x−x2 1 • (1 − x) ≥ e , for x 2 (0; 2 ) 1 1 • 1−x ≤ 1 + 2x for x ≤ 2 Theorem (Linearity of Expectation). n n X X E( aiXi) = aiE(Xi) i=1 i=1 Theorem (Variance). 2 2 V(X) = E(X ) − E(X) Theorem (Variance of a Sum of Random Variables). 2 2 V(aX + bY ) = a V(X) + b V(Y ) + 2abCov(X; Y ) Theorem (AM-GM inequality). Given n numbers x1; : : : ; xn, x + ··· + x 1 n ≥ (x ∗ · · · ∗ x )1=n n 1 n The equality holds if and only if x1 = ··· = xn. Theorem (Markov's inequality). If X is a nonnegative random variable and a > 0, then (X) Pr[X ≥ a] ≤ E a Theorem (Chebyshev's inequality). If X is a random variable (with finite expected value µ and non-zero variance σ2), then for any k > 0, 1 Pr[jX − µj ≥ kσ] ≤ k2 Theorem (Bernoulli's inequality). For every integer r ≥ 0 and every real number x ≥ −1, (1 + x)r ≥ 1 + rx Theorem (Chernoff bound). For independent Bernoulli variables X1;:::;Xn, Pn let X = i=1 Xi. Then, −2 (X) Pr[X ≥ (1 + ) · (X)] ≤ exp( E ) for 0 < E 3 −2 (X) Pr[X ≤ (1 − ) · (X)] ≤ exp( E ) for 0 < < 1 E 2 By union bound, for 0 < < 1, we have −2 (X) Pr[jX − (X)j ≥ · (X)] ≤ 2 exp( E ) E E 3 Remark 1 There is actually a tighter form of Chernoff bounds: e 8 > 0; Pr[X ≥ (1 + ) (X)] ≤ ( )E(X) E (1 + )1+ Remark 2 We usually apply Chernoff bound to show that the probability 2 − E(X) of bad approximation is low by picking parameters such that 2 exp( 3 ) ≤ δ, then negate to get Pr[jX − E(X)j ≤ · E(X)] ≥ 1 − δ. Theorem (Probabilistic Method). Let (Ω; A;P ) be a probability space, Pr[!] > 0 () 9! 2 Ω Combinatorics taking k elements out of n: n • no repetition, no ordering: k n! • no repetition, ordering: (n−k)! n+k−1 • repetition, no ordering: k • repetition, ordering: nk Part I Approximation algorithms 1 Chapter 1 Greedy algorithms Unless P = NP, we do not expect efficient algorithms for NP-hard prob- lems. However, we are often able to design efficient algorithms that give solutions that are provably close/approximate to the optimum. Definition 1.1 (α-approximation). An algorithm A is an α-approximation algorithm for a minimization problem with respect to cost metric c if for any problem instance I and for some optimum solution OP T , c(A(I)) ≤ α · c(OP T (I)) Maximization problems are defined similarly with c(OP T (I)) ≤ α·c(A(I)). 1.1 Minimum set cover Consider a universe U = fe1; : : : ; eng of n elements, a collection of subsets Sm S = fS1;:::;Smg of m subsets of U such that U = i=1 Si, and a non- 1 + negative cost function c : S! R . If Si = fe1; e2; e5g, then we say Si covers elements e1, e2, and e5. For any subset T ⊆ S, define the cost of T as the cost of all subsets in T . That is, X c(T ) = c(Si) Si2T Definition 1.2 (Minimum set cover problem). Given a universe of elements U, a collection of subsets S, and a non-negative cost function c : S! R+, find a subset S∗ ⊆ S such that: ∗ S (i) S is a set cover: ∗ S = U Si2S i (ii) c(S∗), the cost of S∗, is minimized 1If a set costs 0, then we can just remove all the elements covered by it for free. 3 4 CHAPTER 1. GREEDY ALGORITHMS Example S1 e1 S2 e2 S3 e3 S4 e4 e5 Suppose there are 5 elements e1, e2, e3, e4, e5, 4 subsets S1, S2, S3, S4, 2 and the cost function is defined as c(Si) = i . Even though S3 [ S4 covers all vertices, this costs c(fS3;S4g) = c(S3) + c(S4) = 9 + 16 = 25. One can verify ∗ ∗ that the minimum set cover is S = fS1;S2;S3g with a cost of c(S ) = 14. Notice that we want a minimum cover with respect to c and not the number of subsets chosen from S (unless c is uniform cost). 1.1.1 A greedy minimum set cover algorithm Since finding the minimum set cover is NP-complete, we are interested in al- gorithms that give a good approximation for the optimum. [Joh74] describes a greedy algorithm GreedySetCover and proved that it gives an Hn- 2 approximation . The intuition is as follows: Spread the cost c(Si) amongst the vertices that are newly covered by Si. Denoting the price-per-item by ppi(Si), we greedily select the set that has the lowest ppi at each step unitl we have found a set cover. Algorithm 1 GreedySetCover(U; S; c) T ; .
Details
-
File Typepdf
-
Upload Time-
-
Content LanguagesEnglish
-
Upload UserAnonymous/Not logged-in
-
File Pages168 Page
-
File Size-