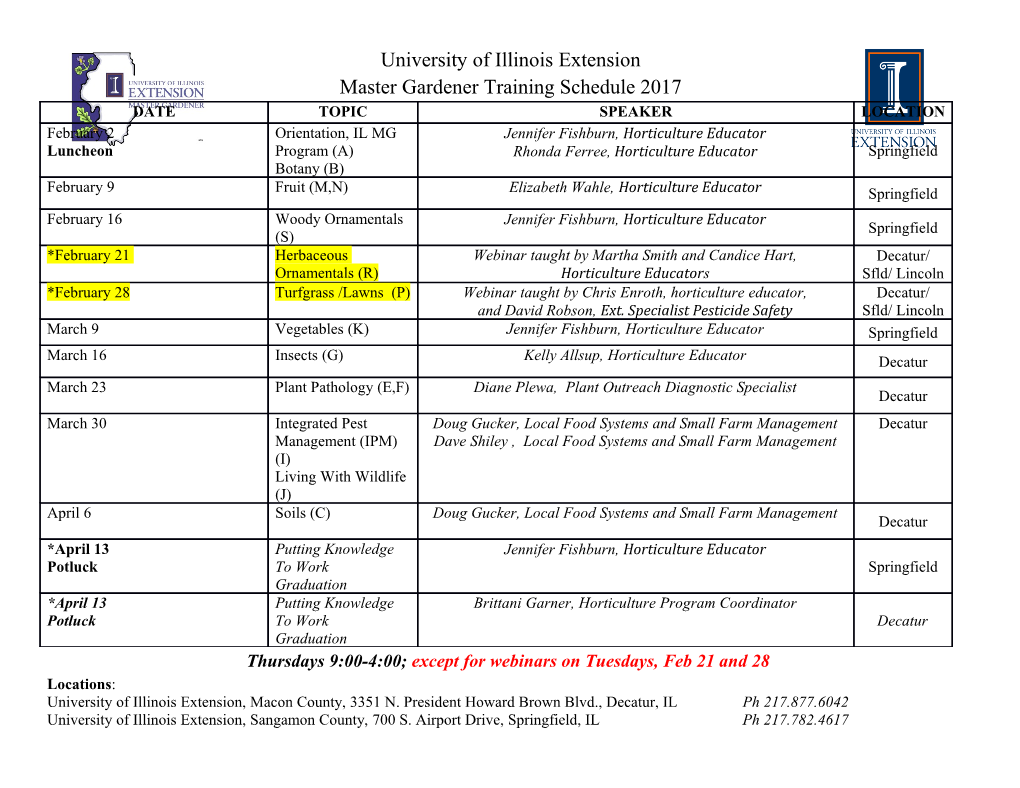
Recurrent Neural Networks for Noise Reduction in Robust ASR Andrew L. Maas1, Quoc V. Le1, Tyler M. O’Neil1, Oriol Vinyals2, Patrick Nguyen3, Andrew Y. Ng1 1Computer Science Department, Stanford University, CA, USA 2EECS Department, University of California - Berkeley, Berkeley, CA, USA 3Google, Inc., Mountain View, CA, USA [amaas,quocle,toneil]@cs.stanford.edu, [email protected], [email protected], [email protected] Abstract models and experimented with simple noise conditions – often a single background noise environment. Recent work on deep neural networks as acoustic mod- Our novel approach applies large neural network els for automatic speech recognition (ASR) have demon- models with several hidden layers and temporally recur- strated substantial performance improvements. We intro- rent connections. Such deep models should better capture duce a model which uses a deep recurrent auto encoder the complex relationships between noisy and clean utter- neural network to denoise input features for robust ASR. ances in data with many noise environments and noise The model is trained on stereo (noisy and clean) audio levels. In this way, our work shares underlying princi- features to predict clean features given noisy input. The ples with deep neural net acoustic models, which recently model makes no assumptions about how noise affects the yielded substantial improvements in ASR [5]. We exper- signal, nor the existence of distinct noise environments. iment with a reasonably large set of background noise Instead, the model can learn to model any type of distor- environments and demonstrate the importance of models tion or additive noise given sufficient training data. We with many hidden layers when learning a denoising func- demonstrate the model is competitive with existing fea- tion. ture denoising approaches on the Aurora2 task, and out- Training noise reduction models using stereo (clean performs a tandem approach where deep networks are and noisy) data has been used successfully with the used to predict phoneme posteriors directly. SPLICE algorithm [6]. SPLICE attempts to model the Index Terms: neural networks, robust ASR, deep learn- joint distribution between clean and noisy data, p(x; y). ing Separate SPLICE models are trained for each noise con- dition and noise level. SPLICE is similar to a linear neu- 1. Introduction ral network with fixed non-linear basis functions. Our model attempts to learn the basis functions with multi- Robust automatic speech recognition (ASR), that with ple hidden layers as opposed to choosing nonlinearities background noise and channel distortion, is a fundamen- which may be important a priori. tal problem as ASR increasingly moves to mobile de- We demonstrate our model’s ability to produce vices. Existing state-of-the-art methods for robust ASR cleaned utterances for the Aurora2 robust ASR task. The use specialized domain knowledge to denoise the speech method outperforms the SPLICE denoising algorithm, as signal [1] or train a word-segment discriminative model well as the hand-engineered ETSI2 advanced front end robust to noise [2]. The performance of such systems (AFE) denoising system. We additionally perform con- is highly dependent upon the methods’ designers imbu- trol experiments to assess our model’s ability to gener- ing domain knowledge like temporal derivative features alize to an unseen noise environments, and quantify the or signal filters and transforms. importance of deep and temporally recurrent neural net- Neural network models have also been applied to work architecture elements. learn a function to map a noisy utterance x to a clean version of the utterance y [3]. Such function approxima- 2. Model tors encode less domain-specific knowledge and by in- creasing hidden layer size can approximate increasingly For robust ASR we would like a function f(x) ! y nonlinear functions. Previous work also introduced neu- which maps the noisy utterance x to a clean utterance ral network models which capture the temporal nature of y with background noise and distortion removed. For speech using recurrent connections, and directly estimate example we might hand engineer a function f(x) using word identity for isolated digit recognition [4]. However, band pass filters to suppress the signal at frequencies we previous work has focused on fairly small neural network suspect contain background noise. However engineer- ing such functions is difficult and often doesn’t capture Output the rich complexity present in noisy utterances. Our ap- y1 y2 yN 4 4 4 proach instead learns the function f(x) using a broad W W W class of nonlinear function approximators – neural net- h3 works. Such models adapt to model the nonlinear rela- W3 W3 W3 tionships between noisy and clean data present in given h2 … training data. Furthermore, we can construct various net- W2 W2 W2 work architectures to model the temporal structure of h1 speech, as well as enhance the nonlinear capacity of the W1 W1 W1 model by building deep multilayer function approxima- Input [0 x1 x2] [x1 x2 x3] [x(N-1) xN 0] tors. Given the noisy utterance x, the neural network out- puts a prediction ^y =f(x) of the clean utterance y. The Figure 1: Deep Recurrent Denoising Autoencoder. A error of the output is measured via squared error, model with 3 hidden layers that takes 3 frames of noisy jj^y − yjj2; (1) input features and predicts a clean version of the center frame where jj · jj is the `2 norm between two vectors. The neural network learns parameters to minimize this error on a given training set. Such noise removal functions are considers small temporal windows of the utterance inde- often studied in the context of specific feature types, such pendently. Intuitively this seems a bad assumption since as cepstra. Our model is instead agnostic as to the type of speech and noise signals are highly correlated at both features present in y and can thus be applied to any sort long and short timescales. To address this issue we add of speech feature without modification. temporally recurrent connections to the model, yielding a recurrent denoising autoencoder (RDAE). A recurrent 2.1. Single Layer Denoising Autoencoder network computes hidden activations using both the input features for the current time step x and the hidden rep- A neural network which attempts to reconstruct a clean t resentation from the previous time step h(1)(x ). The version of its own noisy input is known in the literature t−1 full equation for the hidden activation at time t is thus, as a denoising autoencoder (DAE) [7]. A single hidden (1) (1) (1) (1) layer DAE outputs its prediction y^ using a linear recon- h (xt) = σ(W xt + b + Uh (xt−1)); (4) struction layer and single hidden layer of the form, which builds upon the DAE (Equation 3) by adding a (1) y^ = V h (x) + c (2) weight matrix U which connects hidden units for the cur- h(1)(x) = σ(W (1)x + b(1)) (3) rent time step to hidden unit activations in the previous time step. The RDAE thus does not assume independence The weight matrices V and W (1) along with the bias vec- of each input window x but instead models temporal de- tors c and b(1) parameters of the model. The hidden layer pendence which we expect to exist in noisy speech utter- representation h(1)(x) is a nonlinear function of the input ances. vector x because σ()_ is a point-wise nonlinearity. We use 1 the logistic function σ(z) = 1+ez in our work. 2.3. Deep Architectures Because an utterance x is variable-length and train- A single layer RDAE is a nonlinear function, but perhaps ing an autoencoder with high-dimensional input is expen- not a sufficiently expressive model to capture the com- sive, it is typical to train a DAE using a small temporal plexities of noise environments and channel distortions. context window. This increases computational efficiency We thus make the model more nonlinear and add free and saves the model from needing to re-learn the same parameters by adding additional hidden layers. Indeed, denoising function at each point in time. Furthermore, much of the recent success of neural network acoustic this technique allows the model to handle large variation models is driven by deep neural networks – those with in utterance durations without the need to zero pad inputs more than one hidden layer. Our models naturally ex- to some maximum length. Ultimately the entire clean ut- tend to using multiple hidden layers, yielding the deep terance prediction ^y is created by applying the DAE at denoising autoencoder (DDAE) and the deep recurrent each time sample of the input utterance – much in the denoising autoencoder (DRDAE). Figure 1 shows a DR- same way as a convolutional filter. DAE with 3 hidden layers. Note that recurrent connec- tions are only used in the middle hidden layer in DRDAE 2.2. Recurrent Denoising Autoencoder architectures. The conventional DAE assumes that only short context With multiple hidden layers we denote the ith hidden (i) regions are needed to reconstruct a clean signal, and thus layer’s activation in response to input as h (xt). Deep hidden layers, those with i > 1 compute their activation SNR MFCC AFE Tandem DRDAE as, Clean 0.94 0.77 0.79 0.94 20dB 5.55 1.70 2.21 1.53 (i) (i) (i−1) (i) h (xt) = σ(W h (xt) + b ): (5) 15dB 14.98 3.08 4.57 2.25 10dB 36.16 6.45 7.05 4.05 Each hidden layer h(i) has a corresponding weight ma- 5dB 64.25 14.16 17.88 10.68 trix W (i) and bias vector b(i).
Details
-
File Typepdf
-
Upload Time-
-
Content LanguagesEnglish
-
Upload UserAnonymous/Not logged-in
-
File Pages4 Page
-
File Size-