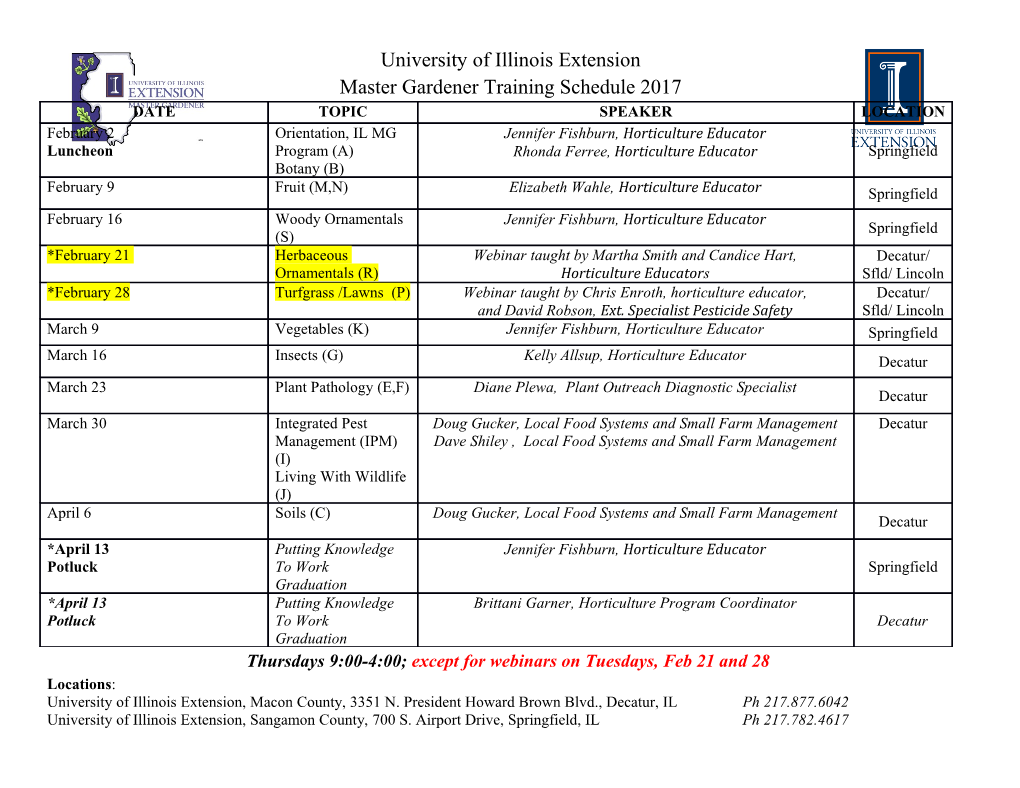
Reservoir Computing approaches to EEG-based Detection of Microsleeps Sudhanshu Ayyagari Department of Electrical and Computer Engineering A thesis presented for the degree of Doctor of Philosophy University of Canterbury Christchurch, New Zealand February, 2017 "Facts are stubborn, but statistics are more pliable" - Mark Twain Abstract Long-haul truck drivers, train drivers, and commercial airline pilots routinely experience monotonous and extended driving periods in a sedentary position, which has been associated with drowsiness, microsleeps, and serious accidents. Consequently, the detection and preferably prediction of the microsleeps in subjects working in these high-risk occupations is very important to workplace safety. Therefore, the aim of this project was EEG-based characterization and early detection of microsleeps during a sustained attention task. The overall approach was to identify reliable physiological cues of lapses of sustained attention and microsleeps, to develop a microsleep system which could be used to detect, or better yet, predict the onset of microsleeps in real time and trigger an alert to rouse the user from an impending microsleep. The main motivation of this project was to develop a state- of-the-art lapse detection system by employing novel classifier schemes based on reservoir computing (RC), specifically echo state networks (ESNs) with cascaded-leaky-integrator- neurons and liquid state machines (LSM) to increase current benchmark performances on microsleep detection. This is the first project and study to have implemented and evaluated EEG-based microsleep detection using RC models for the characterization and detection of microsleeps from the underlying EEG. Moreover, the novelty of the ESN-based cascaded-leaky-integrator neuron approach is in its simplicity (as networks with only 8 or less neurons could achieve optimal performance) and its superior microsleep detection performance. In this research, previously collected behavioral EEG data from fifteen healthy male, non- sleep-deprived volunteers performing a 1D-visuomotor tracking task for 1 hour, was used to form classifier models capable of detecting microsleeps with second-scale resolution. The performance of the microsleep detector was measured both in terms of its ability to detect the lapses-of-responsiveness states and microsleep states (in 1-s epochs). The previous lapse detection benchmark performance on this data, used a simple linear discriminant analysis (LDA)-based classifier, fitted with a meta-learner model. This LDA-based system reported the best performance in terms of its mean phi correlation (φ) = 0.39± 0.06, receiver operator characteristics. An epoch length of 2 s and an overlap window of 1 s (50%) between successive epochs were used in the analysis (AUC-ROC) = 0.86 ± 0.03, and precision recall (AUC-PR) = 0.43± 0.09. Models based on EEG power spectra, and power in the traditional bands, were used to detect the changes in the EEG during microsleeps. Normalized EEG epochs with z-scores > 30 were excluded from analysis, resulting in rejection of 8.3% of the epochs. This process was referred i to as data pruning. Reduced features from 6 independent feature reduction schemes including, principal components analysis (PCA), kernel PCA (KPCA), probabilistic PCA (PPCA), symmetric neighbourhood embedding (SNE), Nearest neighbour embedding (NNE), and stochastic proximity embedding (SPE) were passed as an input to the classifier models. Classifier models evaluated included the RC-based models including the ESNs with sigmoidal neurons, cascaded ESNs with leaky-integrator neurons and LSMs. The RC-based models were compared to other standard classifier models, such as, support vector machines with polynomial kernel (SVMP), LDA, spiky neural networks (SNN), and k-nearest neighbour (KNN) classifiers. Best microsleep detection was achieved using cascaded ESNs with cascaded-leaky-integrator neurons and 50-60 PCs from PCA of the overall 544 power spectral features. This configuration resulted in φ = 0.51 ± 0.07 (mean ± SE), AUC-ROC = 0.88 ± 0.03, and AUC-PR = 0.44 ± 0.09. LSM-based detectors had a lower performance of φ = 0.42 ± 0.06, AUC-ROC = 0.83 ± 0.03, and AUC-PR = 0.43±0.06, compared to the cascaded-leaky-ESN approach. The PCA-based feature reduction modules showed the highest overall performances of the 6 feature-reduction schemes evaluated. This high performance of PCA-modules was found on all classifier schemes. PPCA-based methods followed the PCA schemes in terms of the best microsleep detection performances. Analysis also showed that creating multiple microsleep detection models (ensemble learning) and combining them to form an overall detector resulted in an improvement in performance over a single classifier model. Microsleep detection was also found to have higher accuracy than the other metrics of flatspots, video microsleeps and definite microsleeps. To study the effect of pruning the data, performances were determined for the classifiers when presented with unpruned data in its entirety for training. Performance was compared with a previous study which used a long short-term memory (LSTM) recurrent neural network (RNN) for which φ = 0.38 ± 0.07, AUC-ROC = 0.84 ± 0.02, and AUC-PR = 0.41 ± 0.08). Similar to the pruned datasets, ESNs with cascaded-leaky-integrator neuron models outperformed all the other classifier schemes and set a new benchmark for EEG-based microsleep detection of φ = 0.44 ± 0.06, AUC-ROC = 0.88 ± 0.04, and AUC-PR = 0.45 ± 0.09. This performance, albeit lower than for the pruned datasets, is deemed the best overall performance for microsleep detection as it was for the full behavioural dataset. In summary, the cascaded-leaky-integrator-ESN approach has provided a new benchmark performance for microsleep detection, which is significantly higher (p = 0.012) than by all previous approaches. Notwithstanding, the performance of these EEG‐based microsleep detection systems is still considered to be modest. Further research is needed to identify ii additional cues in the EEG leading to devices capable of more accurate detection and prediction of microsleeps. iii "Science is not about building a body of known 'facts'. It is a method for asking awkward questions and subjecting them to a reality-check, thus avoiding the human tendency to believe whatever makes us feel good." - Terry Pratchett iv Acknowledgements When I began this PhD I was devoid of all confidence, background and good sense, and accordingly gave myself even odds on completing it; that it ever arrived at the binders is very much a reflection on the people I have had around me. Accordingly, over the last four years I feel privileged to have ‘cut my teeth’ in a good portion of very exciting science and feel satisfied with the challenges that I have overcome. I am glad that I have undertaken this ginormous task as it has allowed me to rebuild several aspects of my mental self and hence accomplish the goals I had set out for myself when I started in this programme. None of it would however be possible if I was not lucky enough to be surrounded by some very special and talented people. I describe a few of them here, who have helped me considerably through this journey and also made it memorable and adventurous. I am enormously grateful to my advisors Dr Steve Weddell and Prof Richard Jones for their time, advice and guidance. Over the past four years, Steve has served at various times as a good boss, a mentor, a collaborator, a middleman and a friend. Four years is a long time for anything, and I do not think I would have made it this far without Steve, who kept on believing in me long before I have. In addition to our academic collaboration, I greatly value the close personal rapport that Steve and I have forged over the years. I have great difficulty imagining a kinder or more encouraging individual. Richard with his leadership, guidance, meticulous attention to detail and friendly encouraging attitude, has allowed me to work hard and take pride in my work. Richard balanced his careful, thorough scepticism of every word, figure, and thought I presented him with a strong undercurrent of encouragement and support, which improved me greatly as an independent researcher and a young professional. I would also like to thank both of you for tolerating, and sometimes even supporting, the distracting side projects I tend to succumb to, and always letting me find my own way back to research productivity. While on the subject of kind and thoughtful advisors, I have been fortunate enough to have had a host of wonderful mentors: Prof Phil Bones for his constant encouragement, support and advice throughout this PhD programme. Phil’s insights, both practical and philosophical, have greatly influenced me as a young researcher. Needless to say I am extremely grateful to Phil for organizing and chairing my oral confirmation proceeding. v Prof Mark Billinghurst and the Human Interface Technology Laboratory at the University of Canterbury for offering me the opportunity to work as a research assistant on the ‘Cosense’ project. The valuable research insights I have picked up from Mark during our collaboration were extremely helpful during the latter phases of my PhD programme. It still amazes me that I had such good luck in stumbling upon someone whose style and approach suited me so perfectly. I would also like to thank the SAMSUNG Thinktank group for funding this project. I would also like to express my gratitude to all the people who have enriched my stay at the Electrical Engineering department and helped me in so many ways. In particular, I would like to thank my friends and colleagues, Mr. Reza Shoorangiz, Ms. Nellie Sibaeva, Mr Paul Agger and Mr David Healy for their infectious energy, friendship and constant encouragement. Finally, I am eternally indebted to my parents for their never-ending support that I have received.
Details
-
File Typepdf
-
Upload Time-
-
Content LanguagesEnglish
-
Upload UserAnonymous/Not logged-in
-
File Pages212 Page
-
File Size-