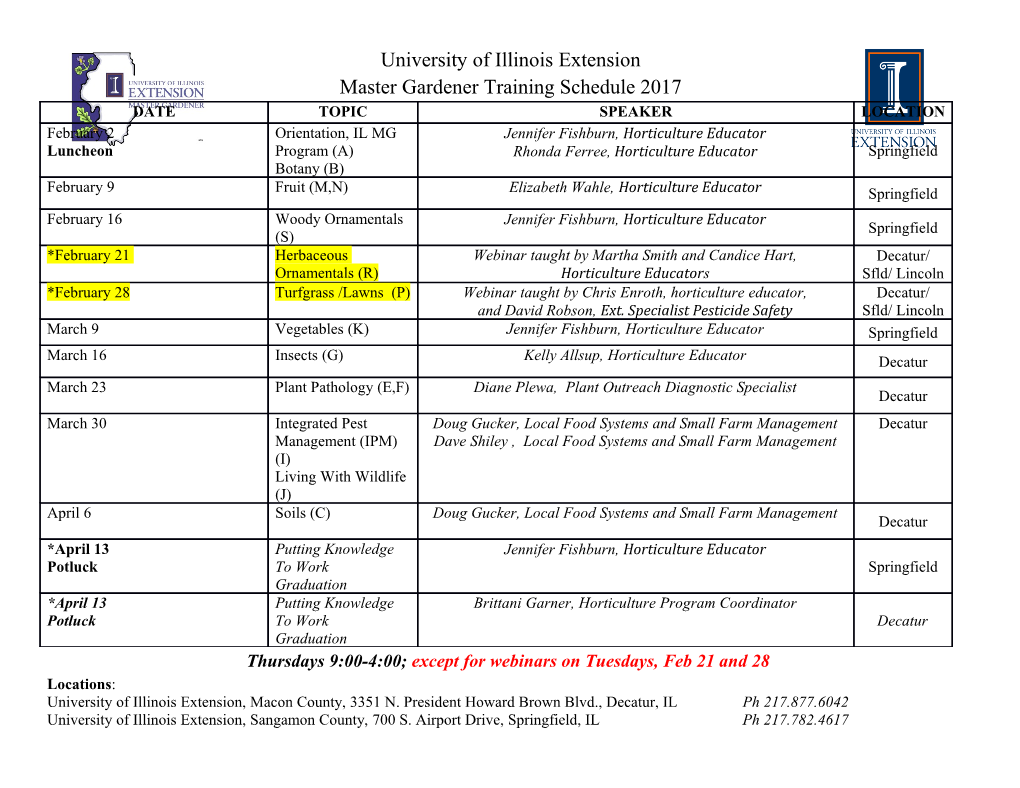
Psychonomic Bulletin & Review 2010, 17 (5), 624-629 doi:10.3758/PBR.17.5.624 BRIEF REPORTS Subjective randomness and natural scene statistics ANNE S. HSU University College London, London, England TH OM A S L. GRIFFIT H S University of California, Berkeley, California A ND ET ha N SC H REI B ER University of California, Los Angeles, California Accounts of subjective randomness suggest that people consider a stimulus random when they cannot detect any regularities characterizing the structure of that stimulus. We explored the possibility that the regularities people detect are shaped by the statistics of their natural environment. We did this by testing the hypothesis that people’s perception of randomness in two-dimensional binary arrays (images with two levels of intensity) is inversely related to the probability with which the array’s pattern would be encountered in nature. We estimated natural scene probabilities for small binary arrays by tabulating the frequencies with which each pattern of cell values appears. We then conducted an experiment in which we collected human randomness judgments. The results show an inverse relationship between people’s perceived randomness of an array pattern and the prob- ability of the pattern appearing in nature. People are very sensitive to deviations from their expec- dimensional binary arrays used in many subjective ran- tations about randomness. For example, the game Yahtzee domness experiments, explanations are more difficult to involves repeatedly rolling 5 six-sided dice. If you were to come by. A common finding in these experiments is that roll all sixes 6 times in a row, you would probably be quite people consider arrays in which cells take different values surprised. The probability of such a sequence arising by from their neighbors (such as the one-dimensional array chance is 1/630. However, the low probability of such an 0010101101) more random than arrays in which cells take event is not sufficient to explain its apparent nonrandom- the same values as their neighbors (such as 0000011111) ness, since any other ordered sequence of the same num- (Falk & Konold, 1997). This result makes it clear that ber of dice rolls has the same probability. Consequently, people are sensitive to certain regularities, such as cells recent accounts of human subjective randomness (our having the same values as their neighbors. However, it is sense of the extent to which an event seems random) have difficult to explain why these regularities should be more focused on the regularities in an event. These regularities important than others that seem a priori plausible, such as suggest that a process other than chance might be at work neighboring cells differing in their values. (Falk & Konold, 1997; Feldman, 1996, 1997; Griffiths In this article, we explore a possible explanation for the & Tenenbaum, 2003, 2004). The basic idea behind these origins of the regularities that influence subjective random- accounts is that stimuli will appear random when they do ness judgments for one class of stimuli: two- dimensional not express any regularities. binary arrays. These stimuli are essentially images, with An important challenge for any account of subjective the cells in the array having the appearance of a grid of randomness based on the presence of regularities is to ex- black and white pixels (see Figure 1). We might thus ex- plain why people should be sensitive to a particular set pect that the kinds of regularities detected by the visual of regularities. In the example given above, systematic system should play an important role in determining their runs of the same number may suggest loaded dice or some perceived randomness. A great deal of recent research sug- other nonrandom process influencing the outcomes. How- gests that the human visual cortex efficiently codes for the ever, for other kinds of stimuli, such as the one- or two- structure of natural scenes—scenes containing natural ele- T. L. Griffiths, [email protected] © 2010 The Psychonomic Society, Inc. 624 RANDOMNESS AND NATU R AL SCENES 625 Figure 1. An example of a two-dimensional binary array. This is a screenshot from the experiment, indicating how the participants made their responses. ments, such as trees, flowers, and shrubs, that represent the denote the probability that X will be generated by chance visual environment in which humans evolved (Olshausen and P(X | regular) be the probability of X under the regular & Field, 2000; Simoncelli & Olshausen, 2001). We con- generating process, Bayes’s rule gives the posterior odds sider the possibility that the kinds of regularities that peo- in favor of random generation as ple detect in two- dimensional binary arrays are those that PX(|random ) PX(|random)(P random) are characteristic of natural scenes. = , (1) Preliminary support for the idea that the statistics of PX(|regular ) PX(|regular)(P regular) natural scenes may explain subjective randomness was where P(random) and P(regular) are the prior probabili- provided by a study conducted by Schreiber and Griffiths ties assigned to the random and regular processes, respec- (2007). In this study, human randomness judgments were tively. Only the first term on the right-hand side of this ex- found to correspond to the predictions of a simple proba- pression, the likelihood ratio, changes as a function of X, bilistic model estimated from images of natural scenes. making it a natural measure of the amount of evidence X This model examined the frequency with which neighbor- provides in favor of a random generating process. Hence, ing regions of an image had the same intensity value. It we can define the randomness of a stimulus X as was found that neighboring regions tend to have similar PX(|random) intensity values, providing a potential explanation for why random()X = log , (2) people consider binary arrays in which neighboring cells PX(|regular) differ in their values more random. However, a full charac- where the logarithm simply places the result on a linear terization of natural scene statistics is not feasible for large scale. binary arrays, due to the exponentially large number of The measure of subjective randomness defined in patterns of cell values that can be expressed in such arrays. Equation 2 has been used to model human randomness In our present work, we use small binary arrays, which judgments for single-digit numbers and one-dimensional allow us to directly estimate a probability distribution over binary arrays (Griffiths & Tenenbaum, 2001, 2003, all possible patterns of cell values from images of natural 2004). Following Schreiber and Griffiths (2007), we ex- scenes. We then conduct an experiment with human partic- amine how subjective randomness might be applied to ipants to examine the relationship between this probability two- dimensional binary arrays of the kind shown in Fig- distribution and the subjective randomness of the image. ure 1. A reasonable choice of P(X | random) is to assume that each cell in the array takes on a value of 1 or 0 with Subjective Randomness As Bayesian Inference equal probability, making P(X | random) 5 1/2m, where m One explanation for human randomness judgments is is the number of cells in the array. However, defining to view them as the result of an inference as to whether an P(X | regular) is more challenging. Direct estimation of observed stimulus, X, was generated by chance or by some the probability of all 2m binary arrays becomes intractable other, more regular process (Feldman, 1996, 1997; Grif- as m becomes large. Thus, we use 4 3 4 binary arrays for fiths & Tenenbaum, 2003, 2004). If we let P(X | random) which we can exhaustively tabulate the frequencies of all 626 HSU , GR IFFIT H S , AND SC hr EIBE R Figure 2. Examples of natural scene images used in our study. The images are from a data set used in the study “Spatiochromatic Receptive Field Properties Derived From Information-Theoretic Analyses of Cone Mosaic Responses to Natural Scenes,” by E. Doi, T. Inui, T. W. Lee, T. Wachtler, and T. J. Sejnowski, 2003, Neural Computation, 15, published by MIT Press. Printed with the authors’ permission. patterns that can appear, providing a nonparametric esti- METHOD mate of P(X | regular) that allows for a comprehensive test of our hypothesis. Participants We estimated the values of P(X |regular) for a set of The participants were 77 members of the University of California, Berkeley community, who participated for course credit. stimuli X corresponding to 4 3 4 binary arrays. Since there 16 are only 2 possible patterns that can be expressed in such Stimuli an array, we can count the frequency with which each pat- The participants were shown one hundred 4 3 4 binary arrays. tern appears in natural images. These stimuli were extracted The stimuli were restricted to evenly balanced 4 3 4 patches, con- from a set of images of natural scenes that have been used in taining an equal number of black and white pixels, in order to avoid previous research (Doi, Inui, Lee, Wachtler, & Sejnowski, any effects resulting from the ratio of black to white pixels in each patch. All stimuli with this property were rank-ordered by the prob- 2003). This set consisted of 62 still nature shots containing ability with which they appeared in natural scenes. We determined trees, flowers, and shrubs, as shown in Figure 2. There were the stimulus for which this probability equaled P(X | random) 5 no images of humans, animals, or cityscapes. Image patches 1/216, and labeled this the neutral stimulus because its probability of varying sizes were extracted from each natural image to is equal under the regular and random generating processes and its measure statistics at a range of scales.
Details
-
File Typepdf
-
Upload Time-
-
Content LanguagesEnglish
-
Upload UserAnonymous/Not logged-in
-
File Pages6 Page
-
File Size-