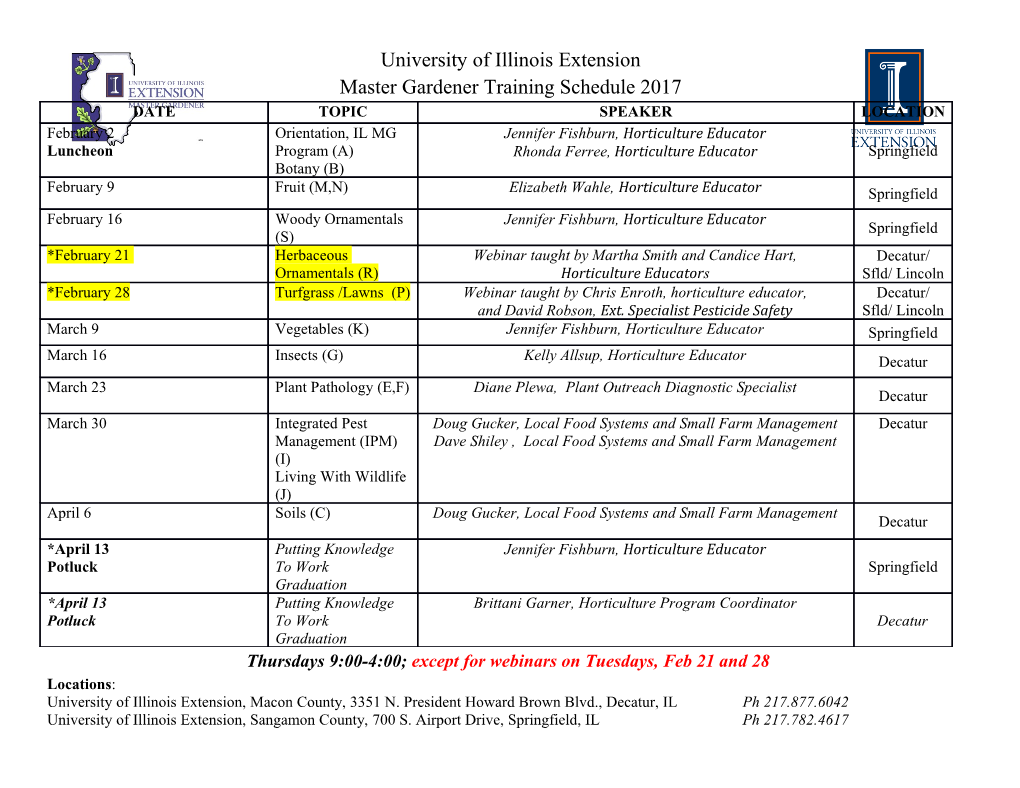
Briefings in Bioinformatics Advance Access published August 20, 2013 BRIEFINGS IN BIOINFORMATICS. page1of13 doi:10.1093/bib/bbt051 A comparative study of statistical methods used to identify dependencies between gene expression signals Suzana de Siqueira Santos, Daniel YasumasaTakahashi, Asuka Nakata and Andre¤Fujita Submitted: 18th April 2013; Received (in revised form): 10th June 2013 Abstract One major task in molecular biology is to understand the dependency among genes to model gene regulatory net- works. Pearson’s correlation is the most common method used to measure dependence between gene expression signals, but it works well only when data are linearly associated. For other types of association, such as non-linear Downloaded from or non-functional relationships, methods based on the concepts of rank correlation and information theory-based measures are more adequate than the Pearson’s correlation, but are less used in applications, most probably because of a lack of clear guidelines for their use. This work seeks to summarize the main methods (Pearson’s, Spearman’s and Kendall’s correlations; distance correlation; Hoeffding’s D measure; Heller^Heller^Gorfine measure; mutual in- http://bib.oxfordjournals.org/ formation and maximal information coefficient) used to identify dependency between random variables, especially gene expression data, and also to evaluate the strengths and limitations of each method. Systematic Monte Carlo simulation analyses ranging from sample size, local dependence and linear/non-linear and also non-functional relationships are shown. Moreover, comparisons in actual gene expression data are carried out. Finally, we provide a suggestive list of methods that can be used for each type of data set. Keywords: correlation; dependence; network; data analysis at Princeton University on August 25, 2013 INTRODUCTION particular measure of stochastic dependence among In bioinformatics, the notion of dependence is cen- many. It is the canonical measure in the world of tral to model gene regulatory networks. The func- multivariate normal distributions, and more generally tional relationships and interactions among genes for spherical and elliptical distributions. However, and their products are usually inferred by using stat- empirical research in molecular biology shows that istical methods that identify dependence among the distributions of gene expression signals may signals [1–4]. not belong in this class [4]. To identify associations One well-known method to identify dependence not limited to linear associations, but dependent in between gene expression signals is the Pearson’s cor- a broad sense, several methods have been de- relation. But Pearson’s correlation, although it is one veloped, most of them based on ranks or information of the most ubiquitous concepts in modern molecu- theory. lar biology, is also one of the most misunderstood The main aim of this article is to clarify the essen- concepts. Some of the confusion may arise from the tial mathematical ideas behind several methods literary use of the word to cover any notion of de- [Pearson’s correlation coefficient [5,6], Spearman’s pendence. To a statistician, correlation is only one correlation coefficient [7], Kendall’s correlation Corresponding author. Andre´ Fujita, Department of Computer Science, Institute of Mathematics and Statistics, University of Sa˜o Paulo, Rua do Mata˜o, 1010–Cidade Universita´ria–SP, 05508-090, Brazil. Tel.: þ 55 11 3091 5177; Fax.: þ 55 11 3091 6134; E-mail: [email protected] Suzana de Siqueira Santos obtained her BS in Computer Science in 2012 at the University of Sa˜o Paulo. She is currently a master course student in Computer Science at the same university. DanielYasumasaTakahashi received his Ph.D. in Bioinformatics in 2009 at the University of Sa˜o Paulo. He is currently a post-doc at Princeton University. Asuka Nakata received her Ph.D. in Biosciences in 2009. She is currently a post-doc at the University of Tokyo. Andre¤Fujita received his Ph.D. in Bioinformatics in 2007. He is currently Assistant Professor at the University of Sa˜o Paulo. ß The Author 2013. Published by Oxford University Press. For Permissions, please email: [email protected] page 2 of 13 de Siqueira Santos et al. coefficient [8], distance correlation [9], Hoeffding’s MEASURES OF DEPENDENCE D measure [10], Heller–Heller–Gorfine (HHG) BETWEEN RANDOM VARIABLES measure [11], mutual information (MI) [12] and Let ðÞx1, y1 , ðÞx2, y2 , ..., ðÞxn, yn be a set of joint n maximal information coefficient (MIC) [13]] used observations from two univariate random variables X to identify dependence between random variables and Y. that anyone wishing to model dependent phenom- The test of dependence between X and Y is ena should know. Furthermore, we illustrate by described as a hypothesis test as follows: Monte Carlo simulations where we varied sample size, local dependence and linear/non-linear and H0: X and Y are not dependent (null hypothesis). also non-functional relationships, the strengths and H1: X and Yare dependent (alternative hypothesis). limitations concerning the different measures used to identify dependent signals. Finally, we illustrate In the next section, we will describe frequently used the application of the methods in actual gene ex- methods to identify dependent data and also show by pression data with known dependence structure be- simulations that some methods such as Pearson’s, tween the genes. Thus we hope to provide the Spearman’s and Kendall’s correlations can only Downloaded from necessary elements for a better comprehension of detect linear or non-linear monotonic (strictly the methods and also the choice of a suitable increasing or strictly decreasing function, i.e. a func- dependence test method, based on practical con- tion that preserves the given order) relationships, straints and goals. whereas others such as distance correlation, HHG measure, Hoeffding’s D measure and MI are able http://bib.oxfordjournals.org/ to identify non-monotonic and non-functional rela- tionships also. Furthermore, we will see that STATISTICAL INDEPENDENCE although MIC is not mathematically proven to be BETWEEN TWO RANDOM consistent against all general alternatives, it can detect VA R I A BLES some non-monotonic relationships. Statistical independence indicates that there is no re- lation between two random variables. If the variables at Princeton University on August 25, 2013 are statistically independent, then the distribution of Pearson’s product-moment correlation one of them is the same no matter at which fixed coefficient levels the other variable is considered, and observa- The Pearson’s product-moment correlation or tions for such variables will lead correspondingly to simply Pearson’s correlation [5,6] is a measure of nearly equal frequency distributions. On the other linear dependence, as the slope obtained by the hand, if there is dependence, then the levels of one linear regression of Y by X is Pearson’s correlation of the variables vary with changing levels of the multiplied byP that ratio ofP standard deviations. n n x y other. In other words, under independence, know- i¼1 i i¼1 i Let x ¼ n and y ¼ n be the means of X ledge about one feature remains unaffected by infor- and Y, respectively. Then, the Pearson’s correlation mation provided about the other, whereas under coefficient rPearson is defined as follows: dependence, it follows which level of one variable P n ðÞÀ ðÞÀ occurs as soon as the level of the other variable is qffiffiffiffiffiffiffiffiffiffiffiffiffiffiffiffiffiffiffiffiffiffiffiffiffiffiffiffiffiffiffiffiffiffiffiffiffiffiffiffiffiffiffiffiffiffiffiffiffiffiffiffiffiffiffiffiffii¼1 xi x yi y rPearsonðÞ¼X, Y P P n 2 n 2 known. i¼1 ðÞxi À x i¼1 ðÞyi À y Formally, two random variables X and Y with cumulative distribution functions FXðÞx and FY ðÞy , For joint normal distributions, Pearson’s correl- and probability densities fX ðÞx and fY ðÞy , are inde- ation coefficient under H0 follows a Student’s pendent if and only if the combined random variable t-distribution with n À 2 degrees of freedom. The ðÞX, Y has a joint cumulative distribution function t statistic is as follows: pffiffiffiffiffiffiffiffiffiffiffi FX, Y ðÞ¼x, y FXðÞx FY ðÞy , or, equivalently, a joint r ðÞX, Y n À 2 density f ðÞ¼x, y f ðÞx f ðÞy . We say that two t ¼ pPearsonffiffiffiffiffiffiffiffiffiffiffiffiffiffiffiffiffiffiffiffiffiffiffiffiffiffiffiffiffiffiffiffiffiffi X, Y X Y 1 À r2 ðÞX, Y random variables X and Y are dependent if they Pearson are not independent. The problem then is how to When the random variables are not jointly nor- measure and detect dependence from the observa- mally distributed, the Fisher’s transformation is used tion of the two random variables. to get an asymptotic normal distribution. Correlation and dependence in biological data page 3 of 13 In the case of perfect linear dependence, we have monotonically increasing relationship (for all x1 and rPearsonðÞ¼ÆX, Y 1. The Pearson correlation is þ1 x2 such that x1 < x2,wehavey1 < y2), and À1in in the case of a perfect positive (increasing) linear the case of a perfect monotonically decreasing rela- relationship and À1 in the case of a perfect negative tionship (for all x1 and x2 such that x1 < x2,wehave (decreasing) linear relationship. In the case of linearly y1 > y2). In the case of monotonically independent independent random variables, rPearsonðÞ¼X, Y 0, random variables, rSpearmanðÞ¼X, Y 0, and in the and in the case of imperfect linear dependence, case of imperfect monotonically dependence, À1 < rPearsonðÞX, Y < 1. These last two cases are À1 < rSpearmanðÞX, Y < 1. Again, similarly to the ones for which misinterpretations of correlation Pearson’s correlation coefficient, rSpearmanðÞ¼X, Y 0 are possible because it is usually assumed that non- does not mean that random variables X and Y are correlated X and Y means independent variables, independent, but only that they are monotonically whereas in fact, they may be associated in a non- independent. linear fashion that Pearson’s correlation coefficient The R function for Spearman’s test is cor.test with is not able to identify.
Details
-
File Typepdf
-
Upload Time-
-
Content LanguagesEnglish
-
Upload UserAnonymous/Not logged-in
-
File Pages13 Page
-
File Size-