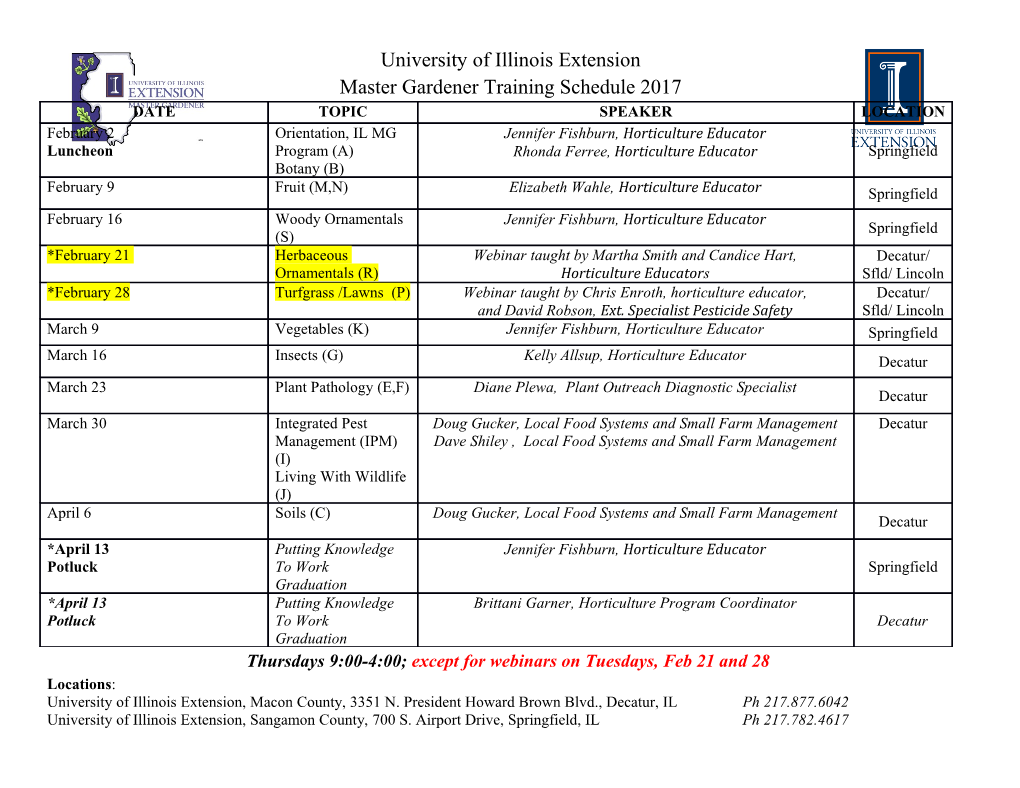
International Journal of Geo-Information Article On the Statistical Distribution of the Nonzero Spatial Autocorrelation Parameter in a Simultaneous Autoregressive Model Qing Luo 1,2 , Daniel A. Griffith 3 and Huayi Wu 1,2,* 1 State Key Laboratory of Information Engineering in Surveying, Mapping and Remote Sensing, Wuhan University, Wuhan 430079, China; [email protected] 2 Collaborative Innovation Center of Geospatial Technology, Wuhan University, Wuhan 430079, China 3 School of Economic, Political, and Policy Sciences, The University of Texas at Dallas, Richardson, TX 75080, USA; dagriffi[email protected] * Correspondence: [email protected]; Tel.: +86-27-6877-8311 Received: 27 October 2018; Accepted: 6 December 2018; Published: 12 December 2018 Abstract: This paper focuses on the spatial autocorrelation parameter r of the simultaneous autoregressive model, and furnishes its sampling distribution for nonzero values, for two regular square (rook and queen) tessellations as well as a hexagonal case with rook connectivity, using Monte Carlo simulation experiments with a large sample size. The regular square lattice directly relates to increasingly used, remotely sensed images, whereas the regular hexagonal configuration is frequently used in sampling and aggregation situations. Results suggest an asymptotic normal distribution for estimated r. More specifically, this paper posits functions between r and its variance for three adjacency structures, which makes hypothesis testing implementable and furnishes an easily-computed version of the asymptotic variance for r at zero for each configuration. In addition, it also presents three examples, where the first employed a simulated dataset for a zero spatial autocorrelation case, and the other two used two empirical datasets—of these, one is a census block dataset for Wuhan (with a Moran coefficient of 0.53, allowing a null hypothesis of, e.g., r = 0.7) to illustrate a moderate spatial autocorrelation case, and the other is a remotely sensed image of the Yellow Mountain region, China (with a Moran coefficient of 0.91, allowing a null hypothesis of, e.g., r = 0.95) to illustrate a high spatial autocorrelation case. Keywords: simultaneous autoregressive model; spatial autocorrelation parameter; nonzero null hypothesis; sampling distribution; asymptotic variance 1. Introduction Spatial autocorrelation (SA) is a common phenomenon of spatial data analyses, where there is a common naive hypothesis that SA is zero (e.g., [1–3]) for datasets involving, for example, georeferenced demographic, social economic, and remotely sensed image variables. A more reasonable postulate would be nonzero SA. Gotelli and Ulrich [4] (p. 171) also pointed out that one of the important challenges in null modeling testing in ecology is “creating null models that perform well with ... varying degrees of SA in species occurrence data”. This is a challenge that is not unique to ecology. A main problem hindering the positing of a nonzero SA hypothesis, or varying degrees of SA, is the unknown sampling distribution of the SA parameter, which may be denoted by r of the simultaneous autoregressive (or SAR, which is called the “spatial error model” in spatial econometrics [5] (p. 5)) model in this paper. This parameter quantifies the degree of self-correlation in the error term (e.g., [6] (p. 42, which can be rewritten as Equation (1)); as when it deviates further from zero, its distribution becomes more skewed and peaked, and its variance decreases. Figure1 shows this phenomenon, ISPRS Int. J. Geo-Inf. 2018, 7, 476; doi:10.3390/ijgi7120476 www.mdpi.com/journal/ijgi ISPRS Int. J. Geo-Inf. 2018, 7, x 476 FOR PEER REVIEW 22 of of 25 from zero, its distribution becomes more skewed and peaked, and its variance decreases. Figure 1 whereshows itsthis connectivity phenomenon, matrix where comes its connectivity from the Wuhan matrix census comes blockfrom datathe Wuhan that are census employed block in data the thatmoderate are employed SA case. in Four the histogramsmoderate SA with case. density Four curveshistograms for differentwith densityr values curves of 0,for 0.5, different 0.9, and values0.99 reveal of 0, that0.5, 0.9, as r andincreases, 0.99 reveal the that distribution as increases, moves the further distribution from zero moves (see further the red from reference zero (see line perpendicularthe red reference to theline horizontal perpendicular axis), to and the its hori shapezontal becomes axis), and narrower. its shape becomes narrower. FigureFigure 1. 1. SelectedSelected frequency frequency distributions distributions of of simultaneous simultaneous autoregressive autoregressive (SAR)-estimated r.. To gain an understanding of the complications relatedrelated to figuringfiguring out the sampling distribution of the test statistic statistic under under the the nonzer nonzeroo hypothesis, hypothesis, it it is is always always enlightening enlightening to to look look back back to to Pearson’s Pearson’s r (i.e.,r (i.e., correlation correlation coefficient) coefficient) in in standard standard statistics statistics and and the the autocorrelation autocorrelation coefficient coefficient in in time series models, because the SA coefficient coefficient resembles the “a “auto”uto” version of the former, and extends the latter from aa uni-directionuni-direction (time (time dimension) dimension) to to a multi-directionsa multi-directions (space (space dimension) dimension) case. case. A parallel A parallel to what to whatis commonly is commonly done in done spatial in scenarios spatial isscenarios that a routine is that null a hypothesisroutine null often hypothesis sets the (auto)correlation often sets the (auto)correlationcoefficient to zero coefficient in classical to statistics zero in orclassical time series statisti (e.g.,cs or [7, 8time]). In series order (e.g., to test [7,8]). the nonzeroIn order correlationto test the nonzerocoefficient correlation in standard coefficient statistics, in a Fisher’sstandard Z-transformation statistics, a Fisher’s is used Z-transformation so that the transformed is used so statistic that the is transformedapproximately statistic normally is approximately distributed and itsnormally variance distributed is stabilized and (e.g., its [ 9variance] (pp. 55–56); is stabilized [10] (p. 487); (e.g., [11 [9]]). (pp.However, 55–56); for [10] the (p. original 487); [11]).r, the However, distribution for the becomes original skewed , the as distributionr approaches becomes its extreme skewed values. as approachesFortunately, its Provost extreme [12 values.] suggests Fortunately, closed-form Provos representationst [12] suggests of closed-form the density representations function and integer of the densitymoments function of the sampleand integer correlation moments coefficient of the sample to furnish correlation a solution coefficient for the distribution to furnish a problem. solution Forfor thetime distribution series autocorrelations, problem. For time the research series autocorrelat on a statisticalions, the distribution research on of a statistical nonzero coefficient distribution was of adeveloped nonzero coefficient from a more was practicaldeveloped viewpoint. from a more For pr example,actical viewpoint. Ames and For Reiterexample, [13 ]Ames established and Reiter the [13]distribution established of autocorrelation the distribution coefficients of autocorrelation in economic coefficients time series in by economic using empirical time series datasets by sousing that empiricalhypothesis datasets testing forso thethat significance hypothesis of (auto)correlationtesting for the significance among economic of (auto)correlation variables could haveamong “a economicmore appropriate variables basis” could (p. have 638) “a than more the appropriate null hypothesis basis” on (p. a basis 638) ofthan zero the (auto)correlation. null hypothesis Moreon a basisrecent of literature zero (auto)correlation. [14] pertaining Mo to thisre recent topic focusedliterature on [14] the pertaining effect of the to nonzerothis topic coefficient focused on the effectdistribution of the nonzero of the Durbin-Watson coefficient on testthe estimator.distribution Similarly of the Durbin-Watson to the time series test literature, estimator. studies Similarly on the to thedistribution time series of the nonzeroliterature, autocorrelation studies on coefficient/parameterthe distribution of arethe rare nonzero in spatial autocorrelation statistics. One coefficient/parameterrelated work dates back are to rare 1967, in inspatial which statistics Mead [15. One] verified related that work the Fisher’sdates back Z-transformation to 1967, in which did Meadnot help [15] to verified obtain a that stabilized the Fisher’s variance, Z-transformation and even its generalized did not help form to obtain could onlya stabilized help in variance, instances and of a veryeven smallits generalizedr (i.e., 0, ± 0.05,form ±could0.1). Thisonly earlier help in work instances provides of evidencea very small that the (i.e., variance 0, ±0.05, problem ±0.1). seems This earlierto be a majorwork obstacleprovides for evidence establishing that athe sampling variance distribution problem forseems nonzero to ber. Thisa major paper obstacle furnishes for a possibleestablishing solution a sampling for this distribution problem by for representing nonzero . the This variance paper furnishes of rˆ as a function a possible of solutionr, and validates for this problemthe asymptotic by representing normality of the this variance distribution of through as a Montefunction
Details
-
File Typepdf
-
Upload Time-
-
Content LanguagesEnglish
-
Upload UserAnonymous/Not logged-in
-
File Pages25 Page
-
File Size-