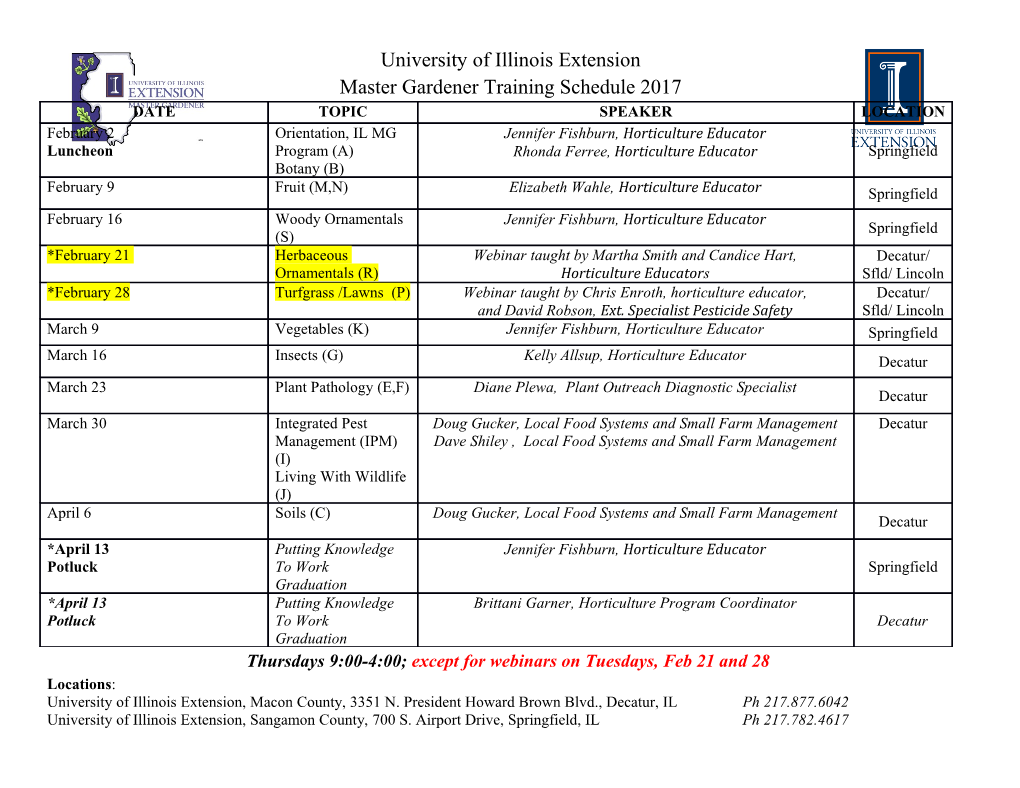
Rattlesnake Genome Supplemental Materials 1 1 SUPPLEMENTAL MATERIALS 2 Table of Contents 3 1. Supplementary Methods …… 2 4 2. Supplemental Tables ……….. 23 5 3. Supplemental Figures ………. 37 Rattlesnake Genome Supplemental Materials 2 6 1. SUPPLEMENTARY METHODS 7 Prairie Rattlesnake Genome Sequencing and Assembly 8 A male Prairie Rattlesnake (Crotalus viridis viridis) collected from a wild population in Colorado was 9 used to generate the genome sequence. This specimen was collected and humanely euthanized according 10 to University of Northern Colorado Institutional Animal Care and Use Committee protocols 0901C-SM- 11 MLChick-12 and 1302D-SM-S-16. Colorado Parks and Wildlife scientific collecting license 12HP974 12 issued to S.P. Mackessy authorized collection of the animal. Genomic DNA was extracted using a 13 standard Phenol-Chloroform-Isoamyl alcohol extraction from liver tissue that was snap frozen in liquid 14 nitrogen. Multiple short-read sequencing libraries were prepared and sequenced on various platforms, 15 including 50bp single-end and 150bp paired-end reads on an Illumina GAII, 100bp paired-end reads on an 16 Illumina HiSeq, and 300bp paired-end reads on an Illumina MiSeq. Long insert libraries were also 17 constructed by and sequenced on the PacBio platform. Finally, we constructed two sets of mate-pair 18 libraries using an Illumina Nextera Mate Pair kit, with insert sizes of 3-5 kb and 6-8 kb, respectively. 19 These were sequenced on two Illumina HiSeq lanes with 150bp paired-end sequencing reads. Short and 20 long read data were used to assemble the previous genome assembly version CroVir2.0 (NCBI accession 21 SAMN07738522). Details of these sequencing libraries are in Supplemental Table S1. Prior to assembly, 22 reads were adapter trimmed using BBmap (Bushnell 2014) and we quality trimmed all reads using 23 Trimmomatic v0.32 (Bolger et al. 2014). We used Meraculous (Chapman et al. 2011) and all short-read 24 Illumina data to generate a contig assembly of the Prairie Rattlesnake. We then performed a series of 25 scaffolding and gap-filling steps. First, we used L_RNA_scaffolder (Xue et al. 2013) to scaffold contigs 26 using the complete transcriptome assembly (see below), SSPACE Standard (Boetzer et al. 2010) to 27 scaffold contigs using mate-pair reads, and SSPACE Longread to scaffold using long PacBio reads. We 28 then used GapFiller (Nadalin et al. 2012) to extend contigs and fill gaps using all short-read data cross 29 five iterations. We merged the scaffolded assembly with a contig assembly generated using the de novo 30 assembly tool in CLC Genomics Workbench (Qiagen Bioinformatics, Redwood City, CA, USA). 31 We improved the CroVir2.0 assembly using the Dovetail Genomics HiRise assembly v2.1.3- 32 59a1db48d61f method (Putnam et al. 2016), leveraging both Chicago and Hi-C sequencing. This 33 assembly method has been used to improve numerous draft genome assemblies (e.g., Jiao et al. 2017; 34 Rice et al. 2017). Chicago assembly requires large amounts of high molecular weight DNA from a very 35 fresh tissue sample. We thus extracted high molecular weight genomic DNA from a liver of a closely 36 related male to the CroVir2.0 animal (i.e., from the same den site). This animal was collected and 37 humanely euthanized according to the Colorado Parks and Wildlife collecting license and UNC IACUC Rattlesnake Genome Supplemental Materials 3 38 protocols detailed above. Hi-C sequencing data were derived from the venom gland of the same animal 39 (see details below on venom gland Hi-C and RNA-seq experimental design). The assembly was carried 40 out using the existing CroVir2.0 draft genome assembly, short read data used in the previous assembly, 41 Chicago, and Hi-C datasets. The HiRise assembly method then mapped Chicago and Hi-C datasets to the 42 draft assembly and generated a model fit of the data based on insert size distributions (Supplemental Fig. 43 S1; Supplemental Material 2). Models were generated with read pairs that mapped within the same 44 scaffold and were used in successive join, break, and final join phases of the pipeline to perform final 45 scaffolding. Dovetail Genomics HiRise assembly resulted in a highly contiguous genome assembly 46 (CroVir3.0) with a physical coverage of greater than 1,000× (Supplemental Table S2). 47 We estimated the size of the genome using k-mer frequency distributions (19, 23, and 27mers) quantified 48 using Jellyfish (Marçais and Kingsford 2011). Raw Illumina 100bp paired-end reads (Supplemental Table 49 S1) were quality trimmed using Trimmomatic (Bolger et al. 2014) using the settings LEADING:10, 50 TRAILING:10, SLIDINGWINDOW:4:15, and MINLEN:36. The total number of output sequences and 51 bases were 400,983,222 and 38,471,185,282, respectively. Quality trimmed reads were then used for 52 Jellyfish k-mer counting, and the Jellyfish k-mer table output per k-mer was used to estimate genome size 53 with GCE (Liu et al. 2013). 54 We generated transcriptomic libraries from RNA sequenced from 16 different tissues: two venom gland 55 tissues; 1 day and 3 days post-venom extraction (see Hi-C and RNA sequencing of Venom Gland section 56 below), one from pancreas, and one from tongue were taken from the Hi-C sequenced genome animal. 57 Additional samples from other individuals included a third venom gland sample from which venom had 58 not been extracted (‘unextracted venom gland’), three liver, three kidney, two pancreas, and one each of 59 skin, lung, testis, accessory venom gland, shaker muscle, brain, stomach, ovaries, rictal gland, spleen, and 60 blood tissues. Total RNA was extracted using Trizol, and we prepared RNAseq libraries using an NEB 61 RNA-seq kit for each tissue, which were uniquely indexed and run on multiple HiSeq 2500 lanes using 62 100bp paired-end reads (Supplemental Table S3). We used Trinity v. 20140717 (Grabherr et al. 2011) 63 with default settings and the ‘--trimmomatic' setting to assemble transcriptome reads from all tissues. The 64 resulting assembly contained 801,342 transcripts comprising 677,921 Trinity-annotated genes, with an 65 average length of 559 bp and an N50 length of 718 bp. 66 Repeat Element Analysis 67 Annotation of repeat elements was performed using homology-based and de novo prediction approaches. 68 Homology-based methods of transposable element identification (e.g., RepeatMasker) cannot recognize Rattlesnake Genome Supplemental Materials 4 69 elements that are not in a reference database, and have low power to identify fragments of repeat elements 70 belonging to even moderately diverged repeat families (Platt et al. 2016). Since the current release of the 71 Tetrapoda RepBase library (v.20.11, August 2015; Bao et al. 2015) is unsuitable for detailed repeat 72 element analyses of most squamate reptile genomes, we performed de novo identification of repeat 73 elements on 6 snake genomes (Crotalus viridis, Crotalus mitchellii, Thamnophis sirtalis, Boa constrictor, 74 Deinagkistrodon acutus, and Pantherophis guttatus) in RepeatModeler v.1.0.9 (Smit and Hubley 2015) 75 using default parameters. Consensus repeat sequences from multiple species were combined into a large 76 joint snake repeat library that also includes previously identified elements from an additional 12 snake 77 species (Castoe et al. 2013). All genomes were annotated with the same library with the exception of the 78 green anole lizard, for which we used a lizard specific library that includes de novo repeat identification 79 for Pogona vitticeps, Ophisaurus gracilis, and Gekko japonicus. To verify that only repeat elements were 80 included in the custom reference library, all sequences were used as input in a BLASTx search against the 81 SwissProt database (UniProt 2017), and those clearly annotated as protein domains were removed. 82 Finally, redundancy and possible chimeric artifacts were removed through clustering methods in CD-HIT 83 (Li and Godzik 2006) using a threshold of 0.85. 84 Homology-based repeat element annotation was performed in RepeatMasker v.4.0.6 (Smit et al. 2015) 85 using a PCR-validated BovB/CR1 LINE retrotransposon consensus library (Castoe et al. 2013), the 86 Tetrapoda RepBase library, and our custom library as references. Output files were post-processed using a 87 modified implementation of the ProcessRepeat script (RepeatMasker package). 88 Gene Annotation 89 We used MAKER v. 2.31.8 (Cantarel et al. 2008) to annotate protein-coding genes in an iterative fashion. 90 Several sources of empirical evidence of protein-coding genes were used, including the full de novo C. 91 viridis transcriptome assembly and protein datasets consisting of all annotated proteins from NCBI for 92 Anolis carolinensis (Alfoldi et al. 2011), Python molurus bivittatus (Castoe et al. 2013), Thamnophis 93 sirtalis (Perry et al. 2018), and Ophiophagus hannah (Vonk et al. 2013), and from GigaDB for 94 Deinagkistrodon acutus (Yin et al. 2016). We also included 422 protein sequences for 24 known venom 95 gene families that were used to infer Python venom gene homologs in a previous study (Reyes-Velasco et 96 al. 2015). Prior to running MAKER, we used BUSCO v. 2.0.1 (Simão et al. 2015) and the full C. viridis 97 genome assembly to iterative train AUGUSTUS v. 3.2.3 (Stanke and Morgenstern 2005) HMM models 98 based on 3,950 tetrapod vertebrate benchmarking universal single-copy orthologs (BUSCOs). We also ran 99 this analysis on the previous genome assembly (CroVir2.0) as a comparison, and provide the details of 100 these analyses in Supplemental Table S4. We ran BUSCO in the ‘genome’ mode and specified the ‘-- Rattlesnake Genome Supplemental Materials 5 101 long' option to have BUSCO perform internal AUGUSTUS training. We ran MAKER with the 102 ‘est2genome=0’ and ‘protein2genome=0’ options set to produce gene models using the AUGUSTUS 103 gene predictions with hints supplied from the empirical transcript and protein sequence evidence.
Details
-
File Typepdf
-
Upload Time-
-
Content LanguagesEnglish
-
Upload UserAnonymous/Not logged-in
-
File Pages56 Page
-
File Size-