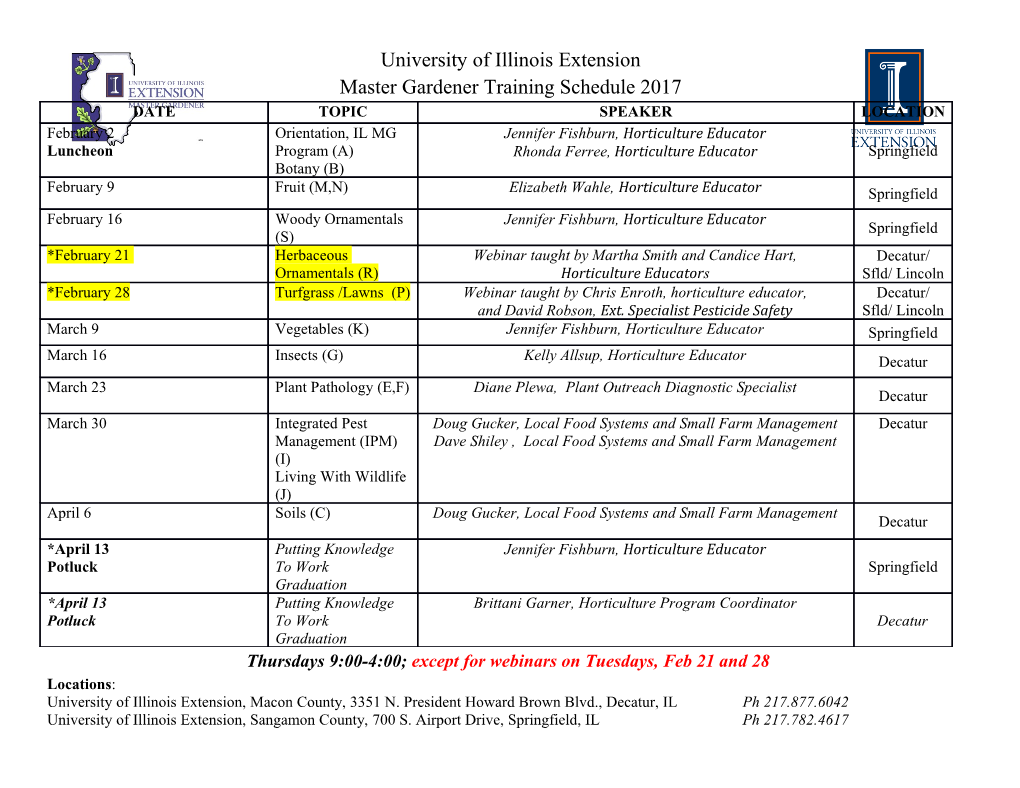
Big Data Sources – Web, Social media and Text Analytics Social media and official statistics Piet Daas THE CONTRACTOR IS ACTING UNDER A FRAMEWORK CONTRACT CONCLUDED WITH THE COMMISSION Eurostat Social media and official statistics • Overview • Social media • Potential uses • Risks when using social media 2 Eurostat Social media platforms In the Netherlands 1) Twitter 2) Facebook 3) Hyves 3 Eurostat • Dutch are very active on social media! – Around 70% according to a recent study • Readily available • Potential source of information on what goes on in ‘Dutch’ society (active on social media) • As an auxiliary source? In addition to survey and admin. data • Any other possibilities? • Explore! • lt 4 4 Map by Eric Fischer (via Fast Company) Eurostat Examples of Social media studies performed at Statistics Netherlands 1. Compare Twitter topics and statistical publication theme's 2. Social media sentiment and consumer confidence 3. ‘Measure’ other basic emotions in social media 4. Social cohesion and Twitter (for an area in NL) 5. Selectivity: background characteristics of Twitter users 6. Event detection on Dutch highways 7. Profiles of companies vs. persons (self-employed) 8. … 5 Eurostat 1. Twitter topics • What are people talking about? • Decided to use Twitter A. Collect as many Twitter usernames (ID’s) as possible Expected between 150,000 – 400,000 (as indicated by studies of others) B. Identify users from a particularr country Location info provided (country, municipality) C. Collect tweets from all those users (if publicly available) Max 3600 per user, 200 per request D. Identify topics discussed in the tweets 6 Eurostat A. Collect usernames • Breadth first algorithm / snowball sampling • Started with a user with many followers A famous Dutch politician with 79,798 followers • Collect the followers of her followers etc. By Twitter REST API, 12 user accounts and PHP-scripts • After 4 weeks we obtained 4,413,391 unique users (id’s) ! Collected user id, username, location and profile information 7 Eurostat B) Identify ‘Dutch’ users • By using location information provided • A considerable number of users do this Checked the location names provided – Inclusion and exclusion list A total of 380,415 (~9%) users were identified as located in the Netherlands 38% of the users, 1,661,467, provided no location info 8 Eurostat C) Collect Tweets • For the 380,415 users the 200 most recent tweets were collected • A total of 12,093,065 messages was obtained • 39% of the users had no ‘tweets’ • Some characteristics 9 Eurostat D) Identify topics • Used 2 approaches 1) Hashtags (1,750,074 with 1 hashtag, 14.5%) Hashtag (#) identifies ‘keyword’ – E.g. #ned, #fail, #wk2010 Manual and text-mining approach 2) Without hashtags (10,330,613 in total, 85.4%) Manual (sample) Text-mining approach failed here – Result of the large ‘Other’ group 10 Eurostat Findings: Dutch Twitter topics (3%) (7%) (3%) (10%) (7%) (3%) (5%) (46%) 11 12 million messages Eurostat 4. Social cohesion • Can the relations between Twitter users be used to study other social phenomena • E.g. Social cohesion • Social cohesion is the set of characteristics that keep a group able to function as a unit. What constitutes group cohesion really depends on whom you ask. For instance, psychologists look at individuals' traits and similarities among the group members. Social psychologists treat cohesion as a trait that combines with others in order to influence the way the group does things. Sociologists tend to look at cohesion as a structural issue, measuring how the interlocking parts of the whole group interact to allow the group to function. Beyond all these disciplinary differences, there are some generalizations we can make about how groups function as a unit. • We studied a particular area in the Netherlands and collected the accounts of all active users on Twitter. • We subsequently looked at their relations (friends and followers) 12 Eurostat 4. Network Visualization (2 Tweeps or more) About 2000 active Twitter accounts Tweeps: Bidirectional following Colours indicate municipalities 13 Eurostat 4. Network data: ‘Twitter friends’ About 2000 active Twitter accounts14 14 Eurostat 4. Risk of studying a small subset • Around 2000 Twitter users were found • Inhabitants in that area ~40.000 • Is a small part of the population • Can be very noisy data (non-representative social media) • Difficult to generalize findings Need more info for that 15 Eurostat Its all about subpopulations Twitter Village study The Netherlands 16 Eurostat 5) Selectivity: Twitter user characteristics • Only a part of the Dutch are active on Twitter • If we want to use this source we need more info • By determining their ‘background’ characteristics – Such as gender, age, income, level of education etc. • What are the possibilities? • Feature extraction is the way to go – For gender 17 Eurostat 4) Picture 3) Messages content 1)Name 2) Short bio 18 Studied a Twitter sample • From a list of Dutch Twitter users (~330.000) • A random sample of 1000 unique ids was drawn • Of the sample: • 844 profiles still existed 844 had a name 583 provided a short bio 473 created ‘tweets’ Default Twitter picture 804 had a ‘non-default’ picture 409 Men (49%) 282 Women (33%) 153 ‘Others’ (18%) – companies, organizations, dogs, cats, ‘bots’.. 19 Eurostat Gender findings: 1) First name • Used Dutch ‘Voornamenbank’ website (First name database) • Score between 0 and 1 (female – male); 676 of 844 (80%) names were registered • Unknown names scored -1 (usually companies/organizations) 20 Eurostat Gender findings: 2) Short bio • If a short bio is provided • Quite a number of people mention their ‘position’ in the family • Mother, father, papa, mama, ‘son of’, etc. • Sometimes also occupations are mentioned that reflect the gender (‘studente’) • 155 of 583 (27%) indicated their gender in short bio • Need to check both English and Dutch texts 21 Eurostat Gender findings: 3) Tweets content – In cooperation with University of Twente (Dong Nguyen) – Machine learning approach that determines gender specific writing style ‐ Language specific: Messages limited to one language (but extendible) 22 ‐ 437 of 473 (92%) persons that created tweets could be classified Eurostat Gender findings: 4) Profile picture 1 3 2 • Use OpenCV to process pictures • 1) Face recognition • 2) Standardisation of faces (resize & rotate) • 3) Classify faces according to gender • - 603 of 804 (75%) profile pictures had 1 or more faces on it 23 Eurostat Gender findings: overall results (1) Diagnostic Odds Ratio (log) Diagnostic Odds Ratio = First name 4.33 (TP/FN) / (FP/TN) Short bio 2.70 Tweet content 1.96 Random guessing log(DOR) = 0 Picture (faces) 0.57 ‐ Multi-agent findings • Need ‘clever’ ways to combine these • Take processing efficiency of the ‘agent’ into consideration 24 Eurostat Gender findings: overall results (2) Combine findings in the best possible way Unassigned (%) Approach used 844 (100%) 1. Use short bio scores (very precise for females) 689 (82%) 2. Use first name scores 153 (18%) 3. Use Tweet content 29 (3.4%) 4. Use picture 20 (2.4%) 5. Assign male gender Final log(DOR) is 7.02, an accuracy of 96.5%! What about other characteristics? 25 Eurostat Concluding remarks • Social media is a difficult source to study – Contains a lot of ‘noise’ • Social media is a secondary data source • Produced for a ´reason´ not identical to way we want to use it – A paradigm shift is needed (for primary oriented people) – Try to improve quality (reduce noise; apply filter) – Benefit from the large volume of data available • Analysing texts and pictures is different/difficult • Learn by doing and by cooperating with experts • Interesting results but • It is a relatively new area for official statistics, so a lot needs to be checked (this takes time) • There are possibilities for official statistics but • Is everybody in the office ready? 26 Eurostat Risks when using social media • Platforms: There are several platforms • Populations: Mixture of several target populations (e.g. persons, companies, others) • Selective: Only measuring the part of the population active on it • Dynamic: Users may hop on and off • Skewed: Not every account is equally active over time • Language: Has its own vocabulary and abbrev. 27 Eurostat • Introduction to Twitter access and API • Exercises Eurostat Various ways of accessing Twitter Eurostat What is API access? • In computer programming, an application programming interface (API) is a set of routine definitions, protocols, and tools for building software and applications. • An API expresses a software component in terms of its operations, inputs, outputs, and underlying types, defining functionalities that are independent of their respective implementations, which allows definitions and implementations to vary without compromising the interface. A good API makes it easier to develop a program by providing all the building blocks, which are then put together by the programmer. • Its an interface by which you can interact with an application Eurostat Twitter Rest API object In R: via twitteR package Link: https://cran.r-project.org/ web/packages/twitteR/ In Python: via tweepy Link: http://www.tweepy.org Code examples will be given! More on: https://dev.twitter.com/overview/api/twitter-libraries 31 Eurostat Rate limits !! 32 Eurostat Exercises • 1) Connect with Twitter API (need the keys) • 2) Find a famous Twitter user from your country and extract some basic info • id, name, nr of followers, followers list, description, location (etc) • 3) Download n tweets of this user (n = 200) • check for duplicates and retweets • 4) From tweets get most frequent used • Hashtags (#word), links (http://), mentions (@user) • 5) Create a wordcloud of words in tweets • With and without stopwords, and without hashtags 33 Eurostat.
Details
-
File Typepdf
-
Upload Time-
-
Content LanguagesEnglish
-
Upload UserAnonymous/Not logged-in
-
File Pages33 Page
-
File Size-