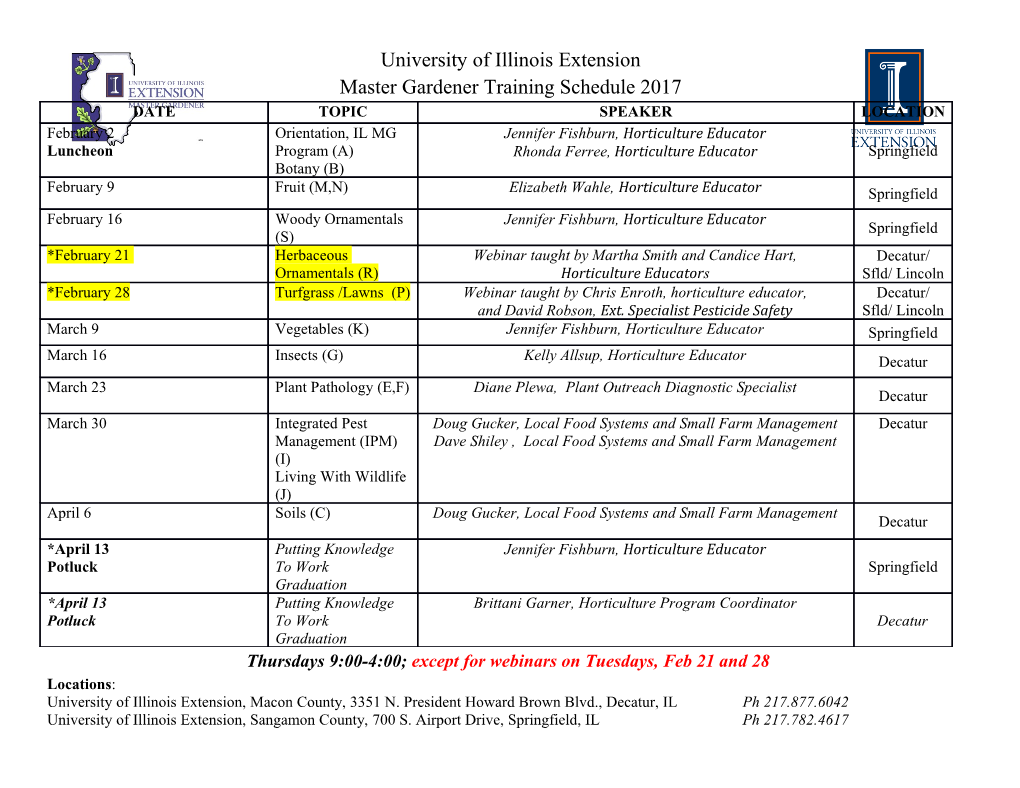
Approximations to Joint-ML and ML Symbol-Channel Estimators in MUD CDMA Thomas Fabricius Ole Nørklit Informatics and Mathematical Modelling Algorithm Design Technical University of Denmark Nokia Mobile Phones Copenhagen, Denmark Copenhagen, Denmark Abstract—In this contribution will we conceptually derive two symbol- ML. The reason for proposing these new estimators, is to re- channel estimators, the joint-ML and the ML, both having exponential veal the better performance achieved from using both the apri- complexity. Pragmatically we derive three approximations with polyno- mial complexity, one to the joint-ML: the pseudo-joint-ML; two to the ori statistics of the symbols and the properties of the signa- ML: the naive-ML and the linear-response-ML. We asses the resulting ture waveform in addition to a correct ML estimator of the average bit error rates empirically. Performance gains of several dBs are channel. The approximations applied are inspired from [6] observed from using the ML based approximations compared to the joint- on Independent Component Analysis (ICA), being similar to ML. Blind MUD CDMA [7]. The derivation is based on a rela- Keywords—CDMA, Multiuser Detection, Blind channel estimation tively simple channel model: Synchronous Users in Flat Fading CDMA; but the methods also applies to more general I. INTRODUCTION models. The paper is organised as follows: First we introduce Traditional code division multiple access (CDMA) systems the channel model; then we review optimal symbol detection are interference limited due to receivers designed using a code according to both minimum probability of error and minimum matched filter or correlator followed by detection. However, BER. Next we derive the joint-ML detector and its approxima- communication networks with high data rates, force the use of tion: the pseudo-joint-ML. Lastly we derive the ML detector short spreading codes. This will generate additional multiple and its approximations: the naive-ML and the linear-response- access interference (MAI) and in such situations interference ML. The performance of the detectors are assessed empirically will limit the receiver performance. As shown by Verdu´ [1] and discussed, followed by a conclusion. CDMA systems need not to be interference limited; when us- ing an optimal multiuser detector (MUD) instead of the tra- II. USERS CDMA IN FLAT FADING ditional receiver. These optimal receivers have exponential Assuming a flat slow fading channel1, and BPSK modula- complexity, so sub-optimal solutions to the multiuser detection tion the received base band CDMA signal can be modelled as problem have been proposed [1], [2]. In traditional settings the channel is estimated from pilot sequences, but blind estimation ¢¥¤ ¦£!#"%$&¢'¤§¦ ¡£¢¥¤§¦©¨ of the channel has received focus, aiming to increase the spec- (1) tral efficiency. A blind linear detector that minimises the out- put energy (MOE) is reported in [1], asymptotically the detec- *),+ ¤ ¢¥¤§¦ tor approaches the linear MMSE detector. In [3] the received where is time and is the ( ’th users signature waveform .-/0-01 signal is projected into a subspace, where a linear decorrelating with unit energy and is the bit duration, is 2 ( or linear MMSE detector is formed, by only knowing the sig- user ( ’s ’th bit in the block of duration , is the ’th $&¢¥¤§¦ nature waveform from the user of interest. In [4] the likelihood users fading coefficient, and is a stationary white complex for the unknown channel is established by using the statistical Gaussian process with unit spectral density. )4+ properties of the symbols and the signature waveform of the We process the signal ¡£¢¥¤§¦ with the conventional matched 1 user of interest. Besides taking the a priori statistics of the -5 filter bank, each filter indexed by (3 , followed by in- symbols into account, the next natural step is to include the a tegration and sampling at each bit instant to get the sufficient "£6 priori statistics of the fading. The last approach is employed in statistics2 (for , , and ) [5], resulting in a Space Alternating Generalized EM (SAGE) CB algorithm. ¨;:#< ¡£¢'¤§¦ ¢¥¤> ¦@?A¤&¨ !D$ 7 98 98 98¥ Our contribution is twofold, first we derive the joint-ML 98¥ (2) = symbol-channel estimator and an approximation to this, de- noted pseudo-joint-ML detector. Secondly we derive the ML E We assume nothing about the distribution of the fading. F detector for the channel i.e. marginalising out the symbols, and We can understand this change to sufficient statistics as a projection onto a two approximations to this: naive-ML and Linear-Response- subspace spanned by the signature waveforms. B ¢'¤§¦ ¢¥¤§¦ 8 where 8 is the correlation between signal and , IV. JOINT-ML SYMBOL-CHANNEL ESTIMATION $ and 98' is a Gaussian random variable with both real and Constructing the joint-ML estimator, we divide the maximi- " 6 imaginary part having zero mean and variance . Using the sation into two parts; one for the symbols given the channel " 6 badcCG above we have the joint likelihood for , , and and one for the channel given the symbols. Using of the - joint likelihood (3) the symbol maximisation becomes 6 ¡£¢¥¤§¦ / / © / " ¨ ¦ ¨ " © 6 ! $ 3 ¢ ¨eNTG Zhg;H ¤ i© ¦ © ¢¥¤ i© ¦ ¨ ¨ ¨ (9) (3) <0PR VYX S fU - $ ¢¥¤ © ¦"!#©%$ ¢¥¤ © ¦"&(' ¨ ¨ " 6 which is identical to (8) for known . The update for the chan- ¢¥¤ ¦ ¨ where we have introduced matrix notation using 8 nel given the symbols is a convex optimisation problem, hence B 7 ¢ ¦ ¨ ¢ ¦ ¨*) ¢ ¦ ¢+©.¦ ¨ 8 8 8 8 8 we can take the partial derivative with respect to the channel ( ( 3 , ¨ , , and "£6 ¢ ¦ # ! 8 and , equate to zero and solve , means transpose and complex conjugate, and is j j ! !ml5$ 3 ¢ ¦ ¨ gkNG ¢7¤ ¦ the usual matrix trace. gkNG ¨ (10) 3 3 PTIMAL ETECTORS 3 .no© ¤p¤ ! © ¤ $ ! ! ! ! III. O D !#q ¨ ¨ ¨ 6 " ¨ Given the noisy data and the channel one goal is to minimise (11) the expected BER, another to minimise the probability of error. 2 j lsrIt5u 3 3 ¢ ¦ ¨ ¢ ¦ ¢M©.¦ ! gkNG%¢ ¦ ! 8 8 8 8 2wv ¨ The expected BER is defined as where ¨ and of a matrix means the diagonal of that matrix arranged as a col- - - ,.-0/ !%3 ¨ - # <>= ? @9ACB 21 umn vector. Since the symbols are discrete the optimisation in ¨547698;: ¨ (4) 2 equation (9) has to be carried out by enumeration3, i.e. ex- A <>= ? @ B 1 haustive search, to surely obtain the global joint-ML solution. where the average 4¥698;: , as indicated, is taken with " 6 rIt5u ¤ We see that the evaluation of can be omitted if only the respect to all bit realizations ¨ and all noise realizations . ¢ ¦ ¦ 6 ¡¢¥¤ / / " ¦ yx{z symbol estimates are of interest. Asymptotically 2 ¦ The joint distribution ¨ is found by the likelihood (3) multiplied by the a priori distribution of the transmitted the joint-ML detector will minimise the probability of error, ¢ ¦ ¡ since the channel estimate will approach the actual channel and symbols ¨ equation (9) becomes equivalent to equation (8). This results 6 6 ¦ ¦ ¢ / " ¦&¨D¡ ¤ ¡ ¢7¤ / / / " ¡¢ ¦ ¦ ¦ ¨ ¨ ¨ (5) in the complexity being equivalent to the complexity of (8). where the dependence on © is omitted due to the fact that it is A. Pseudo-joint-ML Estimation known. Since the complexity of the joint-ML estimator is exponen- It can be shown, under some regularity conditions, that the tial in we derive a local search algorithm for the symbols. ¦ ¦ 3 ¢ ¦ ¨ - 8 ba;cIG ¦ ¦ expected BER subject to the constraint that ¨ is Taking the partial derivative of of the joint likelihood ¦ ¦ ¢ ¦ ¨ - 8 ¨ minimised by (3) with respect to ¨ , subject to the constraint , 3 ¨FE GIH <>= ? @9AJB 1 equating to zero, and solving yields ¨ : ¨%47698 (6) / ¤ E GIH ¨FE GIH|¢ ! ¢7¤ ¢+© ¦ ¦ for all given received data , working element wise. v ¨ ¨ (12) The probability of error is defined as the probability that at E GIH where acts on each element. Taking partial derivatives with least one of the 2 bits in the block are detected wrongly. We "£6 3 ¡ ¢+K ¨,- ¦ ¨ - L) ¢ L ¦ ! respect to and , equating to zero and solving yields the 2 ¨ ¨ can define ¨ and so the " 6 probability of error becomes equations (10) and (11) for and . The equations holds in minima since the gradient of the joint 6 ¦ 3 ¡¢MK ¨ - / " ¦©¨ - ) ¢ # ! ¦ A <>= ? @ B 1 2 likelihood equals zero. Solving the equations can be carried ¦ ¨ 47698;: ¨ (7) out in many ways leading to e.g. hard decision successive in- which is shown to be minimised for any received data ¤ by terference cancellation [2] (HSIC) or hard decision parallel in- terference cancellation (HPIC). Updating equation (12) suffers 6 3 ¨ONI G Z[N ¡¢ ¦ ¤ / / " ¦\ ¨ ¦ ¨ (8) <QPR from local minima and hence depends on the initial values. TS ¥UWVYX $ Thus, it does not in general achieve the joint-ML solution, this Equation (8) is the well known Maximum A Posterior (MAP) is the reason for the name pseudo-joint-ML. In this context 6 solution, which reduces to maximum likelihood for uniform we can see the update of and " as channel estimation us- ¡¢ ¦ ing hard decision feedback from equation (12). We can see the prior distribution ¨ . ¢"^`_ ¦ The complexity per bit for both methods are ] due to pseudo-joint-ML as trying to minimise the probability of error. } the summation and exhaustive search, which is intractable due Equation (10) can eventually be substituted back into (9) before the enu- to the exponential law in . meration. ¢ ¦ ¤ V. ML CHANNEL ESTIMATOR we now propose an arbitrary distribution ¨ which has sup- ¦ 6 ¡ ¢ ¤ / / © / " ¦ ¦ port where the posterior distribution ¨ has Since the joint likelihood is the likelihood for the symbols support. Using Jensens inequality we obtain jointly with the channel, we now derive the correct likelihood ¦ ¡ ¢7¤ / / © / "£6 ¦ for the channel alone. ¦ ¨ 6 ¢ ¦ adcCG / © / " ¦¦¥ a;cIGY¡ ¢7¤F¦ ¤ ¦ ¨ ¦ In ML settings it is common to distinguish between hidden ¢ ¤ <0PR ¨ VYX ¥U variables (incomplete data), visible variables (complete data $ besides the incomplete data) and parameters4. The likelihood (18) ¢ ¦ ¨ ¡ ¢ ¦ ¤ / /T© / "£6 ¦ " 6 ¤ ¨ ¦ of the channel parameters and is found as the joint like- Equality is obtained if ¨ which can be proved by Bayes rule.
Details
-
File Typepdf
-
Upload Time-
-
Content LanguagesEnglish
-
Upload UserAnonymous/Not logged-in
-
File Pages5 Page
-
File Size-