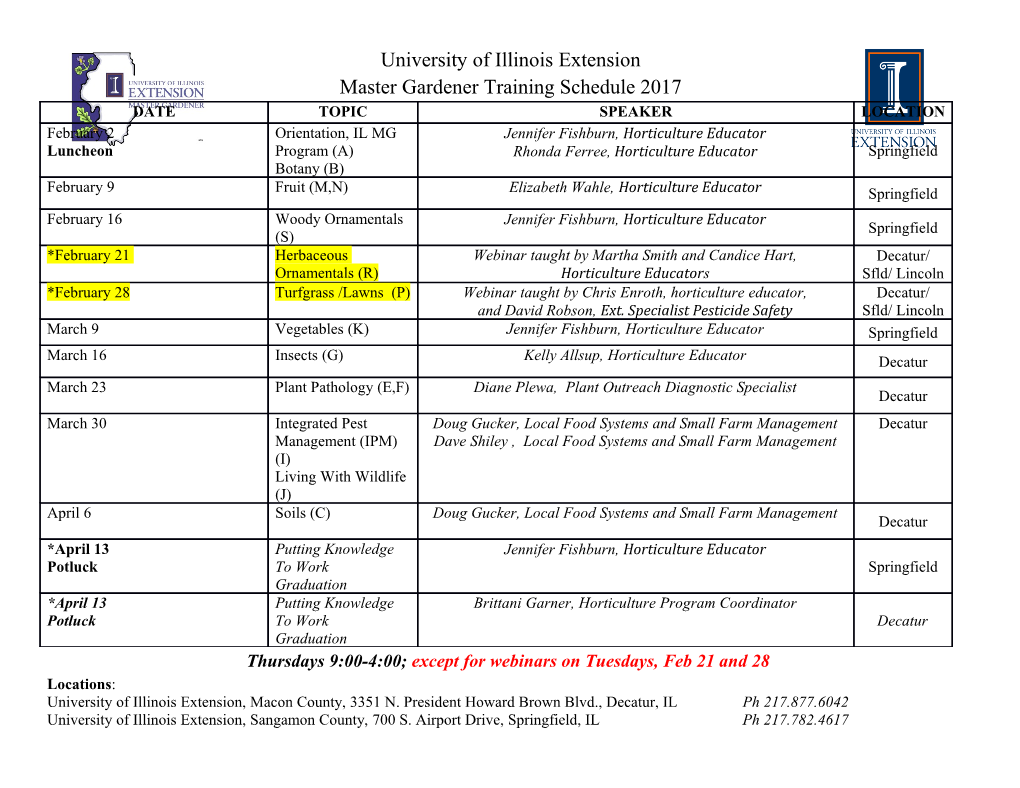
Parsing the SYNTAGRUS Treebank of Russian Joakim Nivre Igor M. Boguslavsky Leonid L. Iomdin Vaxj¨ o¨ University and Universidad Politecnica´ Russian Academy Uppsala University de Madrid of Sciences [email protected] Departamento de Institute for Information Inteligencia Artificial Transmission Problems [email protected] [email protected] Abstract example, Nivre et al. (2007a) observe that the lan- guages included in the 2007 CoNLL shared task We present the first results on parsing the can be divided into three distinct groups with re- SYNTAGRUS treebank of Russian with a spect to top accuracy scores, with relatively low data-driven dependency parser, achieving accuracy for richly inflected languages like Arabic a labeled attachment score of over 82% and Basque, medium accuracy for agglutinating and an unlabeled attachment score of 89%. languages like Hungarian and Turkish, and high A feature analysis shows that high parsing accuracy for more configurational languages like accuracy is crucially dependent on the use English and Chinese. A complicating factor in this of both lexical and morphological features. kind of comparison is the fact that the syntactic an- We conjecture that the latter result can be notation in treebanks varies across languages, in generalized to richly inflected languages in such a way that it is very difficult to tease apart the general, provided that sufficient amounts impact on parsing accuracy of linguistic structure, of training data are available. on the one hand, and linguistic annotation, on the other. It is also worth noting that the majority of 1 Introduction the data sets used in the CoNLL shared tasks are Dependency-based syntactic parsing has become not derived from treebanks with genuine depen- increasingly popular in computational linguistics dency annotation, but have been obtained through in recent years. One of the reasons for the growing conversion from other kinds of annotation. And interest is apparently the belief that dependency- the data sets that do come with original depen- based representations should be more suitable for dency annotation are generally fairly small, with languages that exhibit free or flexible word order less than 100,000 words available for training, the and where most of the clues to syntactic structure notable exception of course being the Prague De- are found in lexical and morphological features, pendency Treebank of Czech (Hajicˇ et al., 2001), rather than in syntactic categories and word order which is one of the largest and most widely used configurations. Some support for this view can be treebanks in the field. found in the results from the CoNLL shared tasks This paper contributes to the growing litera- on dependency parsing in 2006 and 2007, where ture on dependency parsing for typologically di- a variety of data-driven methods for dependency verse languages by presenting the first results on parsing have been applied with encouraging results parsing the Russian treebank SYNTAGRUS (Bo- to languages of great typological diversity (Buch- guslavsky et al., 2000; Boguslavsky et al., 2002). holz and Marsi, 2006; Nivre et al., 2007a). There are several factors that make this treebank However, there are still important differences in an interesting resource in this context. First of parsing accuracy for different language types. For all, it contains a genuine dependency annotation, © Joakim Nivre, Igor M. Boguslavsky, and Leonid theoretically grounded in the long tradition of de- L. Iomdin, 2008. Licensed under the Creative Com- pendency grammar for Slavic languages, repre- mons Attribution-Noncommercial-Share Alike 3.0 Unported license (http://creativecommons.org/licenses/by-nc-sa/3.0/). sented by the work of Tesniere` (1959) and Mel’cukˇ Some rights reserved. (1988), among others. Secondly, with close to 641 Proceedings of the 22nd International Conference on Computational Linguistics (Coling 2008), pages 641–648 Manchester, August 2008 500,000 tokens, the treebank is larger than most dated between 1960 and 2008, texts of online other available dependency treebanks and provides news, etc.) and it is growing steadily. It is an inte- a good basis for experimental investigations us- gral but fully autonomous part of the Russian Na- ing data-driven methods. Thirdly, the Russian lan- tional Corpus developed in a nationwide research guage, which has not been included in previous ex- project and can be freely consulted on the Web perimental evaluations such as the CoNLL shared (http://www.ruscorpora.ru/). tasks, is a richly inflected language with free word Since Russian is a language with relatively free order and thus representative of the class of lan- word order, SYNTAGRUS adopted a dependency- guages that tend to pose problems for the currently based annotation scheme, in a way parallel to the available parsing models. Taken together, these Prague Dependency Treebank (Hajicˇ et al., 2001). factors imply that experiments using the SYNTA- The treebank is so far the only corpus of Russian GRUS treebank may be able to shed further light supplied with comprehensive morphological anno- on the complex interplay between language type, tation and syntactic annotation in the form of a annotation scheme, and training set size, as deter- complete dependency tree provided for every sen- minants of parsing accuracy for data-driven depen- tence. dency parsers. Figure 1 shows the dependency tree for the The experimental parsing results presented in sentence Naibol~xee vozmuwenie uqastnikov this paper have been obtained using MaltParser, mitinga vyzval prodolawi$is rost cen a freely available system for data-driven depen- na benzin, ustanavlivaemyh neftnymi kom- dency parsing with state-of-the-art accuracy for panimi (It was the continuing growth of petrol most languages in previous evaluations (Buchholz prices set by oil companies that caused the greatest and Marsi, 2006; Nivre et al., 2007a; Nivre et al., indignation of the participants of the meeting). In 2007b). Besides establishing a first benchmark for the dependency tree, nodes represent words (lem- the SYNTAGRUS treebank, we analyze the influ- mas), annotated with parts of speech and morpho- ence of different kinds of features on parsing ac- logical features, while arcs are labeled with syntac- curacy, showing conclusively that both lexical and tic dependency types. There are over 65 distinct morphological features are crucial for obtaining dependency labels in the treebank, half of which good parsing accuracy. All results are based on in- are taken from Mel’cuk’sˇ Meaning Text Theory ⇔ put with gold standard annotations, which means (Mel’cuk,ˇ 1988). Dependency types that are used that the results can be seen to establish an upper in figure 1 include: bound on what can be achieved when parsing raw 1. predik (predicative), which, prototypically, text. However, this also means that results are represents the relation between the verbal comparable to those from the CoNLL shared tasks, predicate as head and its subject as depen- which have been obtained under the same condi- dent; tions. The rest of the paper is structured as follows. 2. 1-kompl (first complement), which denotes Section 2 introduces the SYNTAGRUS treebank, the relation between a predicate word as head section 3 describes the MaltParser system used in and its direct complement as dependent; the experiments, and section 4 presents experimen- 3. agent (agentive), which introduces the rela- tal results and analysis. Section 5 contains conclu- tion between a predicate word (verbal noun sions and future work. or verb in the passive voice) as head and its agent in the instrumental case as dependent; YN AG US 2 The S T R Treebank 4. kvaziagent (quasi-agentive), which relates The Russian dependency treebank, SYNTAGRUS, any predicate noun as head with its first syn- is being developed by the Computational Linguis- tactic actant as dependent, if the latter is tics Laboratory, Institute of Information Trans- not eligible for being qualified as the noun’s mission Problems, Russian Academy of Sciences. agent; Currently the treebank contains over 32,000 sen- 5. opred (modifier), which connects a noun tences (roughly 460,000 words) belonging to texts head with an adjective/participle dependent if from a variety of genres (contemporary fiction, the latter serves as an adjectival modifier to popular science, newspaper and journal articles the noun; 642 Figure 1: A syntactically annotated sentence from the SYNTAGRUS treebank. 6. predl (prepositional), which accounts for the that cannot be reliably resolved without extra- relation between a preposition as head and a linguistic knowledge. The parser processes raw noun as dependent. sentences without prior part-of-speech tagging. Morphological annotation in SYNTAGRUS is Dependency trees in SYNTAGRUS may contain based on a comprehensive morphological dictio- non-projective dependencies. Normally, one token nary of Russian that counts about 130,000 entries corresponds to one node in the dependency tree. (over 4 million word forms). The ETAP-3 mor- There are however a noticeable number of excep- phological analyzer uses the dictionary to produce tions, the most important of which are the follow- morphological annotation of words belonging to ing: the corpus, including lemma, part-of-speech tag 1. compound words like ptidesti tany$i and additional morphological features dependent (fifty-storied), where one token corresponds on the part of speech: animacy, gender, number, to two or more nodes; case, degree of comparison, short form (of adjec- tives and participles), representation (of verbs), as- 2. so-called phantom nodes for the representa- pect, tense, mood, person, voice, composite form, tion of hard cases of ellipsis, which do not and attenuation. correspond to any particular token in the sen- Statistics for the version of SYNTAGRUS used tence; for example, kupil rubaxku, a on for the experiments described in this paper are as galstuk (I bought a shirt and he a tie), which follows: is expanded into kupil rubaxku, a on kupilPHANTOM galstuk (I bought a shirt and • 32,242 sentences, belonging to the fiction he boughtPHANTOM a tie); genre (9.8%), texts of online news (12.4%), 3.
Details
-
File Typepdf
-
Upload Time-
-
Content LanguagesEnglish
-
Upload UserAnonymous/Not logged-in
-
File Pages8 Page
-
File Size-