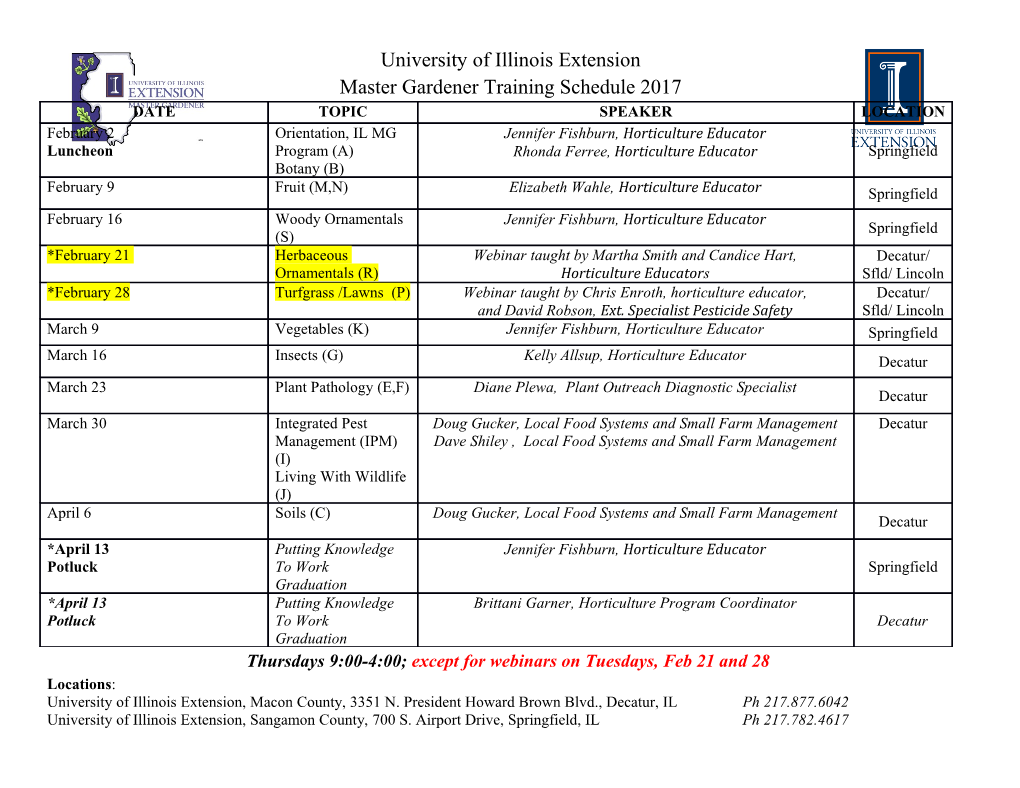
STRUCTURAL ANALYSIS AND SEGMENTATION OF MUSIC SIGNALS A DISSERTATION SUBMITTED TO THE DEPARTMENT OF TECHNOLOGY OF THE UNIVERSITAT POMPEU FABRA FOR THE PROGRAM IN COMPUTER SCIENCE AND DIGITAL COMMUNICATION IN PARTIAL FULFILLMENT OF THE REQUIREMENTS FOR THE DEGREE OF - DOCTOR PER LA UNIVERSITAT POMPEU FABRA Bee Suan Ong 2006 © Copyright by Bee Suan Ong 2006 All Rights Reserved ii Dipòsit legal: B.5219-2008 ISBN: 978-84-691-1756-9 DOCTORAL DISSERTATION DIRECTION Dr. Xavier Serra Department of Technology Universitat Pompeu Fabra, Barcelona This research was performed at theMusic Technology Group of the Universitat Pompeu Fabra in Barcelona, Spain. Primary support was provided by the EU projects FP6-507142 SIMAC http://www.semanticaudio.org. iii Abstract Automatic audio content analysis is a general research area in which algorithms are developed to allow computer systems to understand the content of digital audio signals for further exploitation. Automatic music structural analysis is a specific subset of audio content analysis with its main task to discover the structure of music by analyzing audio signals to facilitate better handling of the current explosively expanding amounts of audio data available in digital collections. In this dissertation, we focus our investigation on four areas that are part of audio-based music structural analysis. First, we propose a unique framework and method for temporal audio segmentation at the semantic level. The system aims to detect structural changes in music to provide a way of separating the different “sections” of a piece according to their structural titles (i.e. intro, verse, chorus, bridge). We present a two-phase music segmentation system together with a combined set of low-level audio descriptors to be extracted from music audio signals. Two different databases are used for the evaluation of our approach on a mainstream popular music collection. The experiment results demonstrate that our algorithm achieves 72% accuracy and 79% reliability in a practical application for identifying structural boundaries in music audio signals. Secondly, we present our framework and approach for music structural analysis. The system aims to discover and generate unified high-level structural descriptions directly from the music signals. We compare the applicability of tonal-related features generated using two different methods (the Discrete Fourier Transform and the Constant-Q Transform) to reveal repeated patterns in music for music structural analysis. Three different audio datasets, with more than 100 popular songs in various languages from different regions of the world, are used to evaluate and compare the performance of our framework with the existing system. Our approach achieves overall precision and recall rates of 79% and 85% respectively for correctly detecting significant structural boundaries in the music signals from three datasets. iv Thirdly, we identify significant representative audio excerpts from music signals based on music structural descriptions. The system extracts a short abstract that serves as a thumbnail of the music and generates a retrieval cue from the original audio files. To obtain valid subjective evaluation based on human perception, we conducted an online listening test using a database of 18 music tracks comprising popular songs from various artists. The results indicate strong dependency between subjects’ musical backgrounds and their preference for specific approaches in extracting good music summaries. For song title identification purposes, both evaluated objective and subjective results are consistent. Fourthly, we investigate the applicability of structural descriptions in identifying song versions in music collections. We use tonal features of the short excerpts, extracted from the audio signals based on our prior knowledge of the music structural descriptions, to estimate the similarity between two pieces. Finally, we compare song version identification performance of our approach with an existing method [Gómez06b] on the same audio database. The quantitative evaluation results show that our approach achieves a modest improvement in both precision and recall scores compared to previous research work. To conclude, we discuss both the advantages and the disadvantages of our proposed approach in the song version identification task. v Acknowledgements I would like to thank my supervisor, Dr. Xavier Serra, for giving me the opportunity and financial support to work in the Music Technology Group. He has introduced me to the field of music content processing. This work would not have been possible without his help. I would also like to thank my research project manager, Perfecto Herrera, for his limitless patience in discussing this work with me regardless of his many other commitments. During my study, I have learned a lot from his helpful insights and valuable suggestions. I want to thank all my colleagues from SIMAC research group, Emilia Gomez, Fabien Gouyon, Enric Guaus, Sebastian Streich, Pedro Cano and Markus Koppenberger for providing a very rare kind of stimulating and supportive environment. I am very grateful to their excellent technical advice and insight throughout this work. Without their presence, I would still be wandering. For assistances in manually annotating our used test data, I would also like to thank Edgar Barroso and Georgios Emmanouil. Without them, I will not have sufficient test data to conduct any kind of experiments. For moral and emotional support, I would like to thank my dear big sister, Dr. Rosa Sala Rose, for her love, encouragement and for creating such a fun and enjoyable experience throughout my stay in Spain. Finally, I wish to thank my family back in Malaysia for their unlimited support for my study trip to Spain. Particularly, I wish to express my profound gratitude to my parents, whose love, support and teachings made me that way I am. vi Contents Abstract……………………………………………………………………………………….. iv Acknowledgement……………………………………………………………………………. vi 1 Introduction………………………………………………………………...……………..... 1 1.1 Motivation and Goal…………………………………………………...…………….... 1 1.2 Multimedia Content Analysis ……………………………………………………….... 4 1.3 Music Audio Content Analysis………………………………………..……………..... 5 1.4 Music Structural Analysis……………………………………………..……………..... 5 1.5 Applications…………………………………………………………...……………..... 6 1.6 Scope…………………………………………………………………..……………..... 7 1.7 Summary of the PhD work…………………………………………….…………….... 8 1.8 Thesis Outline………………………………………………………………………..... 9 1.9 Description of Test Databases Used in Each Chapter…................................................ 11 2 Literature Review…………………………………………………………..……………..... 13 2.1 Introduction…..………………………………………………………..……………..... 13 2.2 Related Work in Automatic Music Structural Analysis……………….……………..... 15 2.2.1 Audio Features…………………………………………………………………..... 15 2.2.1.1 Timbre-related features……………………………………………………..... 16 2.2.1.2 Harmonic and Melody-related features……………………………………..... 18 2.2.1.3 Dynamics-related features…………………………………………………..... 21 2.2.2 Feature Extraction Approach……………………………………..……………..... 22 2.2.3 Audio Segmentation……………………………………………………………..... 23 2.2.3.1 Model-free Segmentation…………………………………….……………..... 25 2.2.3.2 Model-based Segmentation…………………………………..……………..... 27 2.2.4 Music Structure Discovery………………………………………..…………….... 27 vii 2.2.4.1 Self-Similarity Analysis……………………………………...……………..... 28 2.2.4.2 Dynamic Programming……………………………………………………..... 32 2.2.4.3 Clustering………………………………………………….....……………..... 33 2.2.4.4 Hidden Markov Modeling…………………………………………………..... 35 2.3 Discussion……………………………………………………………..……………..... 37 2.4 Summary……………………………………………………………………………..... 39 3 Semantic Audio Segmentation……………………………………………..……………..... 41 3.1 Approach……………………………………………………………………………..... 42 3.1.1 Feature Extraction………………………………………………...……………..... 43 3.1.2 Phase 1 – Rough Segmentation…………………………………...……………..... 45 3.1.3 Phase 2 – Segment Boundaries Refinement…………………………………….... 55 3.2 Evaluation……………………………………………………………...…………….... 57 3.2.1 Datasets…………………………………………………………...……………..... 58 3.2.2 Procedure………………………………………………………….…………….... 58 3.2.3 Results and Discussion………………………………………………………….... 59 3.3 Summary……………………………………………………………………………..... 65 4 Music Structural Analysis Based on Tonal Features……………………………………..... 67 4.1 Approach……………………………………………………………………………..... 68 4.1.1 Feature Extraction………………………………………………...……………..... 70 4.1.2 Similarity Measurement…………………………………………..……………..... 73 4.1.3 Pre-processing…………………………………………………….……………..... 76 4.1.4 Repetition Detection (Listing the repeated sections) …………….……………..... 81 4.1.5 Integrating the Repeated Sections………………………………...……………..... 83 4.1.6 Repetitive Segments Compilation………………………………...……………..... 86 4.1.7 Boundaries Adjustment based on Semantic Audio Segmentation.. …………….... 87 4.1.8 Modulation Detection……………………………………………..…………….... 90 4.1.9 Structural Description Inference………………………………….……………..... 93 4.2 Evaluation……………………………………………………………...…………….... 94 4.2.1 Data set…………………………………………………………………………..... 94 4.2.2 Quantitative Performance……………………………………………………….... 95 4.2.3 Results and Discussion………………………………………………………….... 95 4.3 Summary……………………………………………………………………………..... 104 5 Identifying Representative Audio Excerpts from Music Audio………………………….... 105 viii 5.1 Audio Excerpt Identification and Extraction………………………….……………..... 106 5.1.1 First-30-seconds…………………………………………………..……………..... 106 5.1.2 Most-repetitive…………………………………………………………………..... 107 5.1.3 Segment-to-Song………………………………………………….……………....
Details
-
File Typepdf
-
Upload Time-
-
Content LanguagesEnglish
-
Upload UserAnonymous/Not logged-in
-
File Pages181 Page
-
File Size-