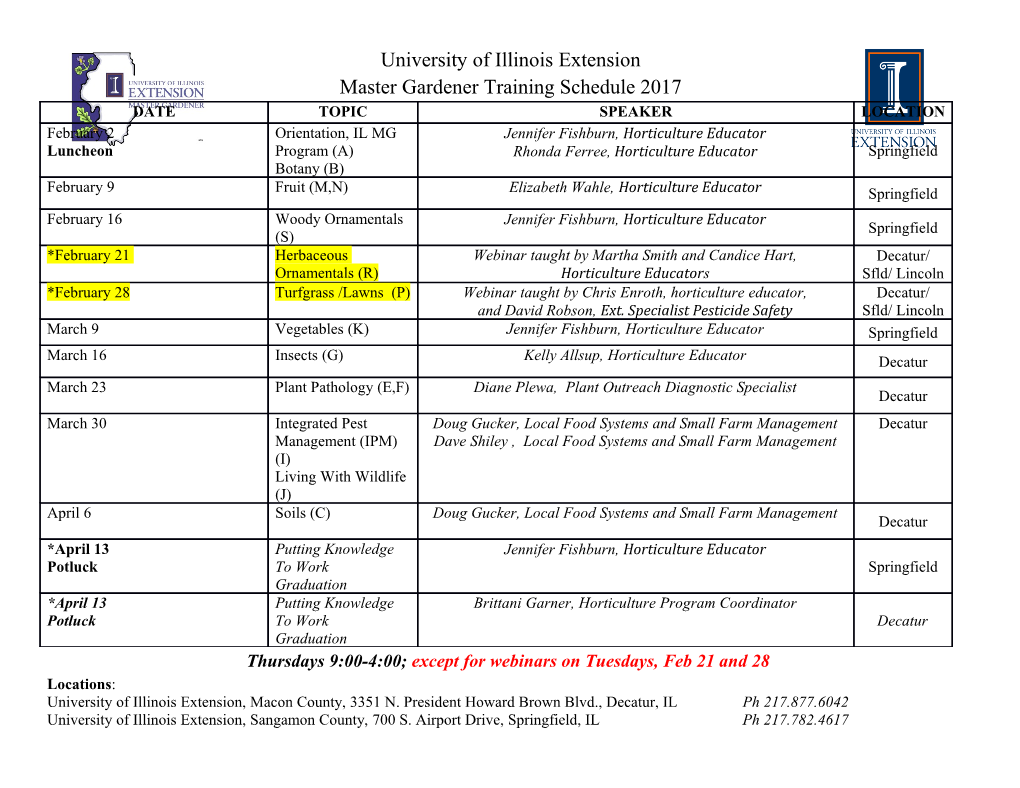
Hardness of Approximation For approximation algorithms we generally focus on NP-hard problems - these problems do not have an exact solution unless P = NP. Despite being very hard to solve exactly, some of these problems are quite easy to approximate (e.g., 2-approximation for the vertex-cover problem). Formally, an algorithm A for an optimization problem P is an α-approximation algorithm, if for any instance I of P , A(I) < α:OP T (I) when P is a minimization problem, and OP T (I) < α:A(I) if P is a maximization problem1. Here A(I) is the solution by algorithm A and OP T (I) is the optimal solution, both for the instance I. Here α is a function on the size of I, α : jIj ! R+, and is often called approximation factor or approximation guarantee for A. 1 Reduction from NP-Complete Problems There are many ways to show that for an optimization problem P , there is no α-approximation algorithm for P unless P = NP. However we will only consider the most straight-forward approach. Our approach is very similar to the reduction approach for showing that a problem is NP-hard. Lets recall. For proving that a decision problem P is NP-complete, we first find a known NP-complete problem L and show that there is a reduction function f from the instances of L to the instances of P , such that yes instances of L map to the yes instances of P and no instances of L to the no instances of P ; see Fig. 1. Figure 1: Reduction for showing NP-completeness. 1some authors have alternate definition for the case of maximization problem 1 To prove that there is no approximation algorithm for an optimization prob- lem P with approximation factor ≤ α, we show that yes instances of a known NP-complete problem L maps to instances of P with OP T < k1; and no in- k2 stances map to instances of P with OP T > k2. Here k1 < k2 and α < ; see k1 Fig. 2. Note that the reduction will slightly change for maximization problem. What will be the change? Figure 2: Gap-introducing reduction (for minimization problem). Thus the reduction makes a gap between the instances with OP T < k1 and the instances with OP T > k2 and this gap is \big" enough so that the flexibility of α cannot \cross" it. We call this kind of reduction gap-introducing reduction. Let's explain. Assume we have such a gap-introducing reduction f. Then assuming that there is an α-approximation algorithm A for P , we can use A to solve the NP-complete problem L in the following way. For any instance I of L, run A on f(I). If I 2 L, then OP T (f(I)) < k1, and so, A(f(I)) < k2 since A is α-approximation algorithm and α < k2 . On the other hand if I2 = L, then k1 OP T (f(I)) > k2 and so, A(f(I)) > k2. Therefore, checking whether A(f(I)) is < k2 or > k2 is sufficient to solve L, which is impossible unless P = NP. Thus there cannot be α-approximation for P . Let's see some examples. Traveling SalesPerson Problem. We know that TSP problem is NP-hard (by a reduction from Hamiltonian-Cycle problem). We also know that if triangle- inequality holds, then there is a 2-approximation and even a 1:5-approximation algorithm for TSP. Here we show that the general case of TSP (without triangle- inequality) has no approximation algorithm unless P = NP. We again use re- duction from HC, although this time it is a gap-introducing reduction. Theorem 1. For any polynomially-computable function α, there is no α(n)- approximation algorithm for TSP on a n-vertex graph, unless P = NP. 2 Proof. For any instance < G > of HC, containing a graph G = (V; E), our re- duction function f constructs an instance < G0; w > of TSP containing another graph G0 = (V 0;E0) and a weight assignment w : E0 ! R+ for the edges of G0. Here G0 is a complete graph on the vertex set V . For each edge e of G0, we assign w(e) = 1 if e is present in G; otherwise w(e) = α(n)n + 1. With this reduction G0 has a TSP with cost n when G is Hamiltonian; otherwise every TSP tour in G0 has cost > α(n):n. Therefore any polynomial-time α(n)-approximation algorithm for TSP can solve HC in polynomial time. Graph-Coloring It is NP-complete to decide whether a graph G has 3- coloring. We use this to show that the optimal graph-coloring problem cannot be approximation within any approximation ratio < 4=3, unless P = NP. Theorem 2. There is no α-approximation algorithm for graph coloring for α < 4=3, unless P = NP. Proof. The proof is actually very easy. Try the following: • Show that any α-approximation algorithm for the graph-coloring problem, with α < 4=3 solves the 3-coloring problem in polynomial time. • What is the gap-introducing reduction? 2 Reduction from Inapproximable Problems Here we see a slightly different reduction. Rather than using a gap-introducing reduction from a known NP-complete problem, we use a gap-preserving re- duction from a known inapproximable problem. Here we find a reduction f from instances of our problem P to a problem L, which cannot be approxi- mated within some factor β as follows. The instance I of L with OP T (I) < k1 maps to instances f(I) of P with OP T (f(I)) < k3, and the instances I with OP T > k2 maps to instances f(I) with OP T (f(I)) > k4. Here β = k2=k1 and α < k4=k3; see Fig. 3. Thus if there is no β-approximation of L, then there is also no α-approximation for P . Figure 3: Gap-preserving reduction. 3 We give an example of gap-preserving reduction from Max-3SAT to Independent- Set and Clique Problem. The reduction is the usual reduction we saw from 3SAT to Independent-Set problem. Put a vertex for each literal in each clause of a 3CNF formula F . add a triangle between the vertices for literals in the same clause, also add edges between xi and xi for each variable xi. If G is a con- structed graph. then F has k satisfiable clauses for some truth assignment if and only if there is an independent set in G of size k, and this happens if and only if there is a clique of size k in G. This implies the following: Lemma 1. If there is an α-approximation for the Max-3SAT problem, then there is also an α-approximation for Independent-Set and Clique problem. For the Max-3SAT problem, we saw a randomized 7=8-approximation al- gorithm { an algorithm with with an expected approximation guarantee 7=8. Interestingly it can be shown that there is no (exact, not randomized) (7=8−)- approximation algorithm for Max-3SAT for any > 0, unless some big open problem gets solved. We will not cover the proof for this in this class. We actually will use another inapproximation result for Max-3SAT problem whose proof is also out-of-scope for this course: Proposition 1. There exists some constant > 0 for which there is no (1 + )- approximation algorithm for the Max-3SAT problem unless P = NP. From Lemma 1 and Proposition 1, we have the following lemma. Lemma 2. There exists some constant > 0 for which there is no (1 + )- approximation algorithm for the Independent-Set and the Clique problem unless P = NP. We will now claim an stronger inapproximability result for the Independent set and the Clique problem. We claim that there is no constant-approximation algorithm for these two problems. To prove this we use the following lemma. Lemma 3. If there is an α-approximationp algorithm for the Independent-Set/Clique problem, then there is also a α approximation algorithm for the Independent- Set/Clique problem. Proof. We prove only for the case of Clique problem, since the proof for the Independent-Set problem follows (and can also be done in a similar manner). This is also one example of a gap-preserving reduction but from the Clique problem to itself. Given a graph G = (V; E), we construct a graph G × G, such that G has a clique of size k if and only if G × G has a clique of size k2. The vertex set of G × G is V × V , and there is an edge in G × G between < u; v > and < w; x > if BOTH the following conditions hold: • u = w or (u; w) 2 E • (v = x) or (v; x) 2 E 4 With this construction, for any clique with vertex set S in G, S × S induces a clique in G × G. Thus a k-clique in G gives a k2-clique in G × G. Conversely, 0 if there is a clique with vertices S in G × G, then both the sets S1 = fuj < 0 0 0 u; v >2 S g and S2 = fvj < u; v >2 S g induce cliques in G. SincepS ⊆ S1 ×S2, 0 0 jS j ≤ jS1jjS2j. Thus at least one of S1 and S2 has size at most S . Hence a k2-clique in G × G also gives a k-clique in G. Now if there is some α-approximation algorithm A for the clique problem, we run A on G × G, and using the above procedure find a clique in G of p 2 size at mostp B(G) = A(G × G).
Details
-
File Typepdf
-
Upload Time-
-
Content LanguagesEnglish
-
Upload UserAnonymous/Not logged-in
-
File Pages5 Page
-
File Size-