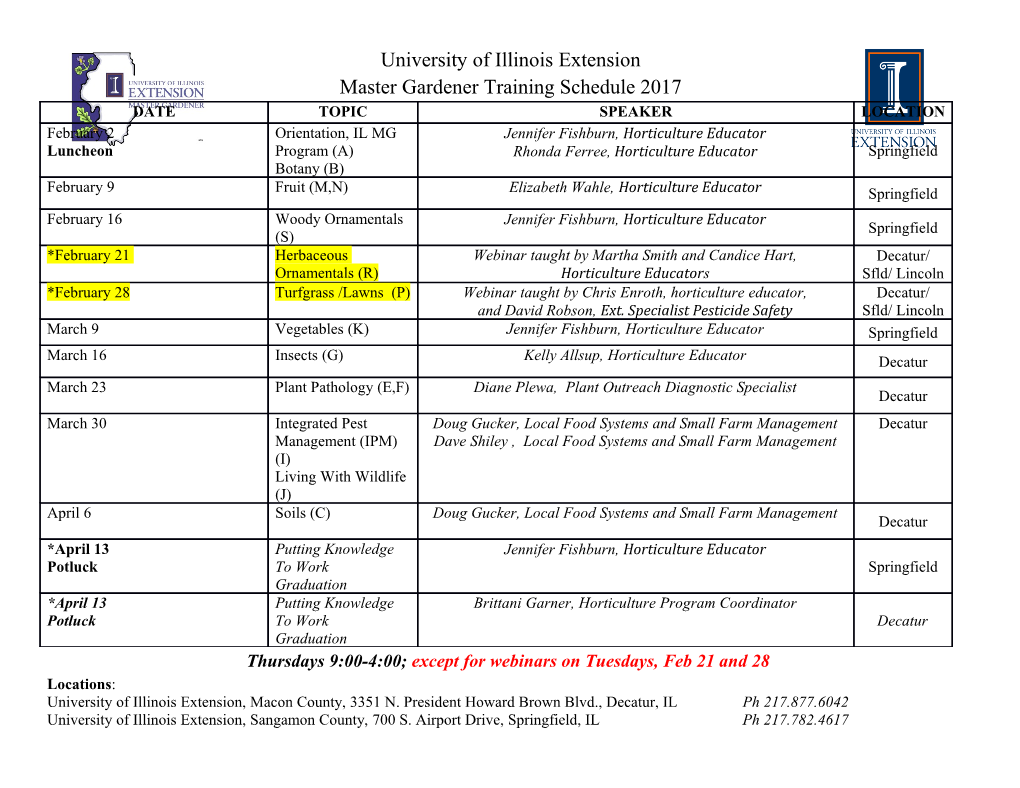
<p> ANALYSIS OF PRODUCTION – AN OVERVIEW </p><p>1. Production Function m Consider a production process involving m outputs y = (y1, y2, …, ym) R produced from n n inputs x = (x1, x2, ..., xn) R . This can also be written in terms of the "netput" vector z (-x, y), where inputs are negative and outputs are positive. The production technology is given by the feasible set F, where z = (-x, y) F means that outputs y can be produced from inputs x. Throughout, we assume that set F is closed (i.e., that it contains its boundary). nm Let g R be some reference bundle satisfying g ≠ 0. Following Luenberger (Microeconomic Theory, 1995), define the shortage function </p><p>S(-x, y, g) = min {: ((-x, y) - g) F}, if there is a such that ((-x, y) - g) F, = + otherwise. The shortage function measures the distance of point z (-x, y) to the boundary of the feasible set, expressed in number of units of the reference bundle g. The properties of the shortage function are: 1/ z (-x, y) F means that S(-x, y, g) 0. Thus, in general, F {z: S(z, g) 0}. 2/ Under free disposal (where z F means that z' F for all z' z), then F = {z: S(z, g) 0}. 3/ S(z + g, g) = + S(z, g). 4/ S(z, g) is convex in z (-x, y) if the set F is convex. 5/ Under free disposal, S(z, g) is non-decreasing in z (-x, y).</p><p>Proof: 1/ Note that S(z, g) > 0 implies that z F. 2/ Consider any z where S(z, g) is finite. Then, S(z, g) 0 implies that [z - S(z, g) g] F. But, under free disposal, [z - S(z, g) g] F implies that [z - S(z, g) g + S(z, g) g] F. Thus, S(z, g) 0 implies that z F, or equivalently F {z: S(z, g) 0}. Combining this result with the previous one yields F = {z: S(z, g) 0}.</p><p>3/ S(z + g, g) = min {: (z + g - g) F},</p><p>= mink {k + : (z - k g) F}, where k = - , = + S(z, g). 4/ Consider any two z and z'. If S(z, g) < and S(z', g) < , then [z - S(z, g) g] F and [z' - S(z', g] F. Let z" = z + (1-) z' for any [0, 1]. The convexity of F implies that [z" - S(z, g) g - (1-) S(z', g) g] F. By definition of the shortage function, it follows that S(z", g) S( z + (1-) z', g) S(z, g) g + (1-) S(z', g) g. The above inequality still holds when S(z, g) < and/or S(z', g) < . This shows that S(z, g) is convex in z when F is convex. 5/ Consider any two points z and z’ satisfying z’ ≤ z. When S(z, g) < ∞, [z - S(z, g) g] F. Under free disposal, [z - S(z, g) g] F and z’ z implies that [z’ - S(z, g) g] F. By definition of the shortage function, this implies that S(z’, g) ≤ S(z, g), i.e. that S(z, g) is non-decreasing in z.</p><p>Note 1: Result 1/ implies that S(z, g) 0 when z is feasible. And result 2/ shows that, under free disposal, the function S(z, g) 0 provides a complete characterization of the production technology. As such S(-x, y, g) = 0 is an implicit multi-input multi-output production function. Note 2: When S(z, g) is twice continuously differentiable in z, then results 3/ and 4/ imply that [S/z] g = 1, [2S/z2] = a symmetric matrix,</p><p>1 = positive semi-definite if the set F is convex, [2S/z2] g = 0. Note 3: Consider the case where g = (0, …0, 1). Then, the shortage function becomes </p><p>S(z, g) = min {: (-x, y1, …, ym-1, ym - ) F}, </p><p>= min {ym - k: (-x, y1, …, ym-1, k) F}, with k = ym - , </p><p>= ym + mink{-k: (-x, y1, …, ym-1, k) F}, </p><p>= ym - maxk{k: (-x, y1, …, ym-1, k) F},</p><p>= ym - g(x, y1, …, ym-1), where g(x, y1, …, ym-1) = maxk{k: (-x, y1, …, ym-1, k) F}. Here, g(x, y1, …, ym-1) is the standard production function for the m-th output, given inputs x and the first (m-1) outputs (y1, …, ym-1). In this case, the shortage function S(z, g) is simply [ym - g(x, y1, …, ym-1)], and S(z, g) 0 simply means that ym g(x, y1, …, ym-1). In addition, using result 4/, the convexity of the feasible set F is equivalent to the concavity of g(x, y1, …, ym-1). Under differentiability, this corresponds to the concept of diminishing 2 2 marginal productivity, where F/(x, y1, …, ym-1) is a symmetric, negative semi-definite matrix, with 2 2 2 2 F/xi 0, i = 1, …, n, and F/yj 0, j = 1, …, m-1. Finally, using results 3/ and 4/ (with z (-x, y)), under a convex technology exhibiting free disposal, the production function g(x, y1, …, ym-1) is non-decreasing in x, </p><p> non-increasing in (y1, …, ym-1), </p><p> and concave in (x, y1, …, ym-1). </p><p>2. Alternative Representations of the Production Technology 2.1. The directional distance function nm Let g R be some reference bundle satisfying g ≠ 0. Following Chambers, Chung and Fare, (Journal of Economic Theory, 1996), define the directional distance function </p><p>D(-x, y, g) = max {: ((-x, y) + g) F}, if there is a such that ((-x, y) + g) F, = - otherwise. </p><p>Note that D(z, g) = max {: (z + g) F}, </p><p>= max {-: (z - g) F}, where = -, </p><p>= -min {: (z - g) F}, = -S(z, g). Thus, the directional distance function D(z, g) is just the negative of the shortage functions S(z, g): D(z, g) = -S(z, g). The properties of the directional distance function are: 1/ z (-x, y) F means that D(-x, y, g) 0. Thus, in general, F {z: D(z, g) 0}. 2/ Under free disposal (where z F means that z' F for all z' z), then F = {z: D(z, g) 0}. 3/ D(z + g, g) = - + D(z, g). 4/ D(z, g) is concave in z = (-x, y) if the set F is convex. 5/ Under free disposal, D(z, g) is non-increasing in z (-x, y).</p><p>Note 1: Result 1/ implies that D(z, g) 0 when z is feasible. And result 2/ shows that, under free disposal, the function D(z, g) 0 provides a complete characterization of the production technology. Again, D(-x, y, g) = 0 is an implicit multi-input multi-output production function. Note 2: When D(z, g) is twice continuously differentiable in z, then results 3/ and 4/ imply that [D/z] g = -1, [2D/z2] = a symmetric matrix, = negative semi-definite if the set F is convex, </p><p>2 [2D/z2] g = 0. Note 3: Consider the case where g = (0, …0, 1). Then, the directional distance function becomes </p><p>D(z, g) = g(x, y1, …, ym-1) - ym, where g(x, y1, …, ym-1) = maxk{k: (-x, y1, …, ym-1, k) F} is the standard production function for the m-th output, given inputs x and the first (m-1) outputs (y1, …, ym-1). In this case, the directional distance function is simply D(z, g) = [g(x, y1, …, ym-1) - ym] and D(z, g) 0 simply means that ym g(x, y1, …, ym- 1). </p><p>2.2. Shephard input distance function Following Shephard (Theory of Cost and Production Function, 1970), define Shephard input distance function </p><p>DI(-x, y) = max {: (-x/, y) F}, if there is a such that (-x/, y) F, = - otherwise.</p><p>Shephard input distance function DI(z) measures the distance from point z (-x, y) to the boundary of the feasible set, expressed as a proportion of the input vector x. Letting g = (x, 0), note that the directional distance function becomes </p><p>D(z, (x, 0)) = max {: (-x + x, y) F}, </p><p>= max {1 -1/: (-x/, y) F}, where = 1/(1-), </p><p>= 1 + max {-1/: (-x/, y) F}, </p><p>= 1 - 1/max {: (-x/, y) F},</p><p>= 1 - 1/DI(z). Thus, given z (-x, y), the directional distance function D(z, g) and Shephard input distance function DI(z) exhibit the following relationship: D(z, (x, 0)) = 1 - [1/DI(z)], or equivalently DI(z) = 1/[1 - D(z, (x, 0))]. </p><p>Note that, for any k > 0, DI(-k x, y) = max {: (-k x/, y) F} = max {k : (-x/, y) F} = k DI(-x, y), implying that DI(-x, y) is homogeneous of degree 1 in x. </p><p>In general, z (-x, y) F implies that DI(z) ≥ 1. And under free input disposal, DI(z) ≥ 1 provides a complete characterization of the production technology, with DI(z) = 1 providing an implicit function representation of the multi-input multi-output production frontier. </p><p>2.3. Farrell input distance function Following Farrell ("The Measurement of Production Efficiency" Journal of the Royal Statistical Society, 1957), define Farrell input distance function </p><p>DF(-x, y) = min {: (-x, y) F}, if there is a such that (-x, y) F, = otherwise.</p><p>Given z (-x, y), note that Shephard input distance function satisfies </p><p>DI(z) = max {: (-x/, y) F},</p><p>= max {1/: (-x, y) F}, where = 1/, </p><p>= 1/min {: (-x, y) F},</p><p>= 1/DF(z). Thus, Farrell input distance function DF(z) and Shephard input distance function DI(z) are inverse functions of each other: DF(z) = 1/DI(z), or equivalently DI(z) = 1/DF(z). </p><p>In general, z (-x, y) F implies that DF(z) ≤ 1. And under free input disposal, DF(z) ≤ 1 provides a complete characterization of the production technology, with DF(z) = 1 providing an implicit function representation of the multi-input multi-output production frontier. </p><p>2.4. Shephard output distance function 3 Following Shephard (Theory of Cost and Production Function, 1970), define Shephard output distance function </p><p>DO(-x, y) = min {: (-x, y/) F}, if there is a such that (-x, y/) F, = otherwise.</p><p>Shephard output distance function DO(z) measures the distance from point z (-x, y) to the boundary of the feasible set, expressed as a proportion of the output vector y. Letting g = (0, y), note that the directional distance function becomes </p><p>D(z, (0, y)) = max {: (-x, y + y) F}, </p><p>= max {-1 + 1/: (-x, y/) F}, where = 1/(1+), </p><p>= -1 + 1/[min {: (-x, y/) F}], </p><p>= [1/DO(z)] - 1. Thus, given z (-x, y), the directional distance function D(z, g) and Shephard output distance function DO(z) exhibit the following relationship: D(z, (0, y)) = [1/DO(z)] - 1, or equivalently DO(z) = 1/[1 + D(z, (0, y))]. </p><p>Note that, for any k > 0, DO(-x, k y) = max {: (-x, k y/) F} = max {k : (-x, y/) F} = k DO(-x, y), implying that DO(-x, y) is homogeneous of degree 1 in y. </p><p>In general, z (-x, y) F implies that DO(z) ≤ 1. And under free output disposal, DO(z) ≤ 1 provides a complete characterization of the production technology, with D0(z) = 1 providing an implicit function representation of the multi-input multi-output production frontier. </p><p>3. Returns to Scale Assume that 0 F. By definition, the technology F exhibits increasing return to scale (IRTS) if k F F, constant return to scale (CRTS) if k F = F, decreasing returns to scale (DRTS) if k F F, for all k > 1.</p><p>Returns to scale can be expressed in terms of any of the functions representing the production technology. For example, for any (0, 1), the directional distance function D(z, g) satisfies 禳< IRTS 镲 D( z, g) 睚= D(z, g) under CRTS . 镲 铪> DRTS Proof: For any (0, 1), consider the case where there is a satisfying ( z + g) F. Then, the directional distance function becomes</p><p>D( z, g) = max {: ( z + g) F}, </p><p>= max {: (z + g) (1/) F}, where = /, 禳< IRTS 镲 睚= D(z, g) when (1/) F F, i.e., under CRTS . 镲 铪> DRTS</p><p>Next consider the case where z (-x, y) is on the production frontier and satisfies D(z, g) = 0. </p><p>4 禳< IRTS 镲 The above result becomes D( z, g) 睚= 0 under CRTS . When D(z) is differentiable in z (-x, y), 镲 铪> DRTS differentiating D(( - ε) z, g) with respect to ε (evaluated at = 1 and ε = 0) gives IRTS n m i1 (D/xi) xi + j1 (D/yj) yj 0 under CRTS . DRTS</p><p>When g = (0, …, 0, 1), we know that D(z, g) = g(x, y1, …, ym-1) - ym. The above result becomes IRTS n m-1 i1 (g/xi) xi - ym + j1 (g/yj) yj 0 under CRTS , DRTS or, assuming that x > 0, y > 0, and using ym = g(x, y1, …, ym-1), IRTS n m-1 i1 ln(g)/ln(xi) 1 - j1 (ln(g)/ln(yj) under CRTS , DRTS where ln(g)/ln(xi) and ln(g)/ln(yj) are production elasticities. Define the scale elasticity SE as n m-1 SE = i1 ln(g)/ln(xi)/[1 - j1 (ln(g)/ln(yj)]. It follows that the scale elasticity SE satisfies IRTS SE 1 under CRTS . DRTS</p><p>Note: When z is feasible (i.e., when z ≡ (-x, y) F), we have IRTS DF(z) DO(z) under CRTS , DRTS</p><p> showing that DF(z) and DO(z) are the same under CRTS. However, DF(z) and DO(z) differ under non-constant returns to scale.</p><p>Proof: DF(-x, y) = min {: (-x, y) F}</p><p>= min {: (-x, y/) (1/) F}, where (0, 1] (since z ≡ (-x, y) F), IRTS min {: (-x, y/) F} ≡ DO(-x, y) when (1/) F F, i.e. under CRTS . DRTS</p><p>4. Production Behavior 4.1. Cost Minimization Consider a firm facing the production technology F. Assume that the firm faces input prices r = 5 n n (r1, …, rn) R and takes such prices as given. The firm cost is written as r x = i1 ri xi. Under cost minimizing behavior, we have c C(r, y) = r x (r, y) = minx {r x: (-x, y) F}, where xc(r, y) is the cost minimizing input demand functions, and C(r, y) is the cost function. When F is represented by the production function ym g(x, y1, …, ym-1), this can be written as c C(r, y) = r x (r, y) = minx {r x: ym g(x, y1, …, ym-1)}. </p><p>The associated Lagrangean is L = r x + [ym - g(x, y1, …, ym-1)], where is a Lagrange multiplier. Under differentiability, the first order necessary conditions for interior solutions to the cost minimization problem are: r = (g/x), (FOC) implying </p><p>MRSij (g/xi)/(g/xj) = ri/rj, for all i j. And using the envelope theorem, </p><p>C/ym = , (Env1)</p><p>C/yj = - g/yj, j = 1, …, m-1. (Env2)</p><p>Note: When evaluated at x = xc(r, y), the scale elasticity SE can be alternatively written as m SE = 1/[ j1 [(ln(C)/ln(yj)]], IRTS m implying that j1 [(ln(C)/ln(yj)] 1 under CRTS . DRTS n m-1 Proof: SE = i1 ln(g)/ln(xi)/[1 - j1 (ln(g)/ln(yj)], n m-1 = i1 (g/xi)(xi/ym)/[1 - j1 (g/yj)(yj/ym)], n m-1 = i1 (ri/)(xi/ym)/[1 - j1 (g/yj)(yj/ym)], using (FOC), n m-1 = i1 [ri/(C/ym)](xi/ym)/[1 + j1 [(C/yj)/(C/ym)](yj/ym)], using (Env1)-(Env2), m = C/[ j1 [(C/yj) yj], m = 1/[ j1 [(ln(C)/ln(yj)]]. </p><p>4.2. Profit maximization m In addition, assume that the firm faces output prices p = (p1, …, pm) R and takes such prices m as given (i.e., it is a firm in a competitive market). The firm revenue is written as p y = j1 pj yj, and the m n firm profit as p y - r x = j1 pj yj - i1 ri xi. Under profit maximizing behavior, we have * * (p, r) = p y (p, r) - r x (p, r) = maxx,y {p y - r x: (-x, y) F}, </p><p>= maxy {maxx {p y - r x: (-x, y) F}}, </p><p>= maxy {p y - minx {r x: (-x, y) F}, = maxy {p y - C(r, y)}, where y*(p, r) and x*(p, r) are the profit maximizing choice functions, and (p, r) is the profit function. </p><p>When F is represented by the production function ym g(x, y1, …, ym-1), this can be written as * * (p, r) = p y (p, r) - r x (p, r) = maxx,y {p y - r x: ym g(x, y1, …, ym-1)}, Under differentiability, the first order conditions for interior solutions to the profit maximization problem are: 6 MVPi pm (g/xi) = ri, for inputs i = 1, …, n, and</p><p>MVPj pm (-g/yj) = pj, for outputs j = 1, …, m-1, or</p><p> pj = C/yj, for outputs j = 1, …, m, (corresponding to marginal cost pricing).</p><p>5. Economies of scope In the multi-output case (with m > 1), consider partitioning the output set M = {1, …, m} into K partitions, with M = {M1, …, MK} where Mk identifies the k-th "product line." Start with a given firm producing outputs y = (y1, …, ym) > 0. Consider splitting this firm into K specialized firms, where the k-th k k k k k firm specializes in the k-th product line and produces y = (y1 , …, ym ) where yj = yj if j Mk, and yj = 0 K k if j Mk, k = 1, …, K. Following Baumol et al., given y = k1 y , the technology is said to exhibit economies of scope at point y if K k C(r, y) < k1 C(r, y ). Alternatively, the technology is said to exhibit diseconomies of scope at point y if K k C(r, y) > k1 C(r, y ). Since economies of scope identify a cost advantage to producing multiple outputs, they can provide an economic rationale for the existence of multi-product firms and for the analysis of mergers. </p><p>6. Duality</p><p>Under some regularity conditions, the production function g(x, y1, …, ym-1), the cost function C(r, y), and the profit function π(p, r) have been shown to be dual to each others. This means that knowing one is equivalent to knowing the others. As a result, under perfect competition, the analysis of production issues can be done in an equivalent way using either a production function, a cost function, or a profit function. </p><p>6.1. Duality between profit function (p, r) and production function g(x, y1, …, ym-1) The following duality relationships hold * * (p, r) = p y (p, r) - r x (p, r) = maxx,y {p y - r x: ym g(x, y1, …, ym-1)} m-1 n = maxx,y {pm g(x, y1, …, ym-1) + j1 pj yj - i1 ri xi}, and, assuming that the set F is convex and satisfies free disposal, n m-1 g(x, y1, …, ym-1) = minp/pm0,r/pm0{[(p/pm, r/pm) + i1 (ri/pm) xi - j1 (pj/pm) yj]}.</p><p>6.2. Duality between cost function C(r, y) and profit function (p, r) The following duality relationships hold * * (p, r) = p y (p, r) - r x (p, r) = maxy {p y - C(r, y)}, and, assuming that the output possibility set Y(x) = {y: (-x, y) F} is convex and satisfies free output disposal, </p><p>C(r, y) = maxp0 {p y - (p, r)}.</p><p>6.3. Duality between cost function C(r, y) and directional distance function D(-x, y, g) Following Luenberger (1995) and Chambers et al. (1996), the following duality relationships hold c C(r, y) = r x (r, y) = minx {r x: D(-x, y, g) 0} = minx {r x - D(-x, y, g) [r gx]} when g = (gx, 0), and, assuming that the input requirement set X(y) {x: (-x, y) F} is convex and satisfies free input disposal, </p><p>7 D(-x, y, g) = minr0 {r x - C(r, y): r gx = 1},</p><p>= minr0 {[r x - C(r, y)]/[r gx]}, where g = (gx, 0). </p><p>6.4. Duality between profit function (p, r) and directional distance function D(-x, y, g)</p><p>Letting g = (gx, gy), the following duality relationship holds (Luenberger, 1995). Assuming that [p gy + r gx] > 0, </p><p>(p, r) maxx,y {p y - r x: (-x, y) F}, m+n = maxx,y {p y - r x + D(-x, y, g) [p gy + r gx], (-x, y) R }. (Dual-1) Proof: First, we show that, for any z = (-x, y), the following inequality holds: (p, r) p y - r x + D(z, g) </p><p>[p gy + r gx]: z = (-x, y) F}. This inequality always holds if D(z, g) = -. Consider the case </p><p> where D(z, g) > -. It follows that (z + D(z, g) g) F, which implies (p, r) p [y + D(z, g) gy] - r [x – D(z, g) gx]. </p><p>Next, for any z F, we prove the converse: (p, r) p y - r x + D(z, g) [p gy + r gx]: z = (-x, y) </p><p>F}. Noting that z F implies D(z, g) 0, and using [p gy + r gx] > 0, we have</p><p>(p, r) maxx,y {p y - r x: (-x, y) F},</p><p> maxx,y {p y - r x + D(z, g) [p gy + r gx]: (-x, y) F}, m+n maxx,y {p y - r x + D(z, g) [p gy + r gx]: z = (-x, y) R }. </p><p>Note 1: The maximization in (Dual-1) does not impose the restriction (-x, y) F. This reflects the fact that, for profit maximization, the directional distance function D(z, g) provides all the relevant information about technology. </p><p>Note 2: (Dual-1) is particularly convenient when g is chosen such that [p gy + r gx] = 1. Then the directional distance function D(z, g) shows up in simple additive form in the objective function of (Dual-1).</p><p>Using the normalization rule [p gy + r gx] = 1, the following duality relationship holds (Luenberger, 1995). Assuming that the feasible set F is convex, </p><p>D(-x, y, g) = infp,r {(p, r) - p y + r x: [p gy + r gx] = 1}. (Dual-2) + Proof: Define D (-x, y, g) infp,r {(p, r) - p y + r x: [p gy + r gx] = 1}. From (Dual-1), we have (p, r) p m+n y - r x + D(-x, y, g) [p gy + r gx], for any (-x, y) R }. It follows that [(p, r) – [p y - r x]]/[p gy + + r gx] D(-x, y, g), which implies D (-x, y, g) D(-x, y, g). To prove (Dual-2), we need to prove the reverse inequality (under convexity of the set F): D+(z, g) D(z, g), z = (-x, y). For simplicity, assume that D(z, g) > -. Let za = z + D(z, g) g, which satisfies za F and D(za, g) = 0. Thus, za is on the boundary of F. F being a convex set, by the supporting hyperplane theorem, there is an h such that h z h za for all z. Normalizing h, define q = h/[h g], which satisfies q g = 1. It follows that q = (p, r) is feasible point in the minimization problem (Dual-2). Since the hyperplane defined by q supports F at za = z + D(z, g) g, we obtain (q) = q [z + D(z, g) g] = q z + D(z, g). Hence, D(z, g) = (q) - q z. The definition of D+ implies D+(z, g) (q’) – q’ z for any q’ satisfying q’ z = 1. Combining these two results gives D+(z, g) D(z, g). </p><p>Note 3: The results (Dual-1) and (Dual-2) could be equivalently expressed using the shortage function S(z, g), with S(z, g) = -D(z, g). </p><p>6.5. Duality between cost function C(r, y) and Shephard input distance function DI(-x, y) Following Shephard (1970), the following duality relationships hold c C(r, y) = r x (r, y) = minx {r x: DI(-x, y) 1} 8 = minx {[r x]/DI(-x, y)}. and, assuming that the input requirement set X(y) {x: (-x, y) F} is convex and satisfies free input disposal, </p><p>DI(-x, y) = minr0 {r x: C(r, y) 1},</p><p>= minr0 {[r x]/C(r, y)}. </p><p>9</p>
Details
-
File Typepdf
-
Upload Time-
-
Content LanguagesEnglish
-
Upload UserAnonymous/Not logged-in
-
File Pages9 Page
-
File Size-