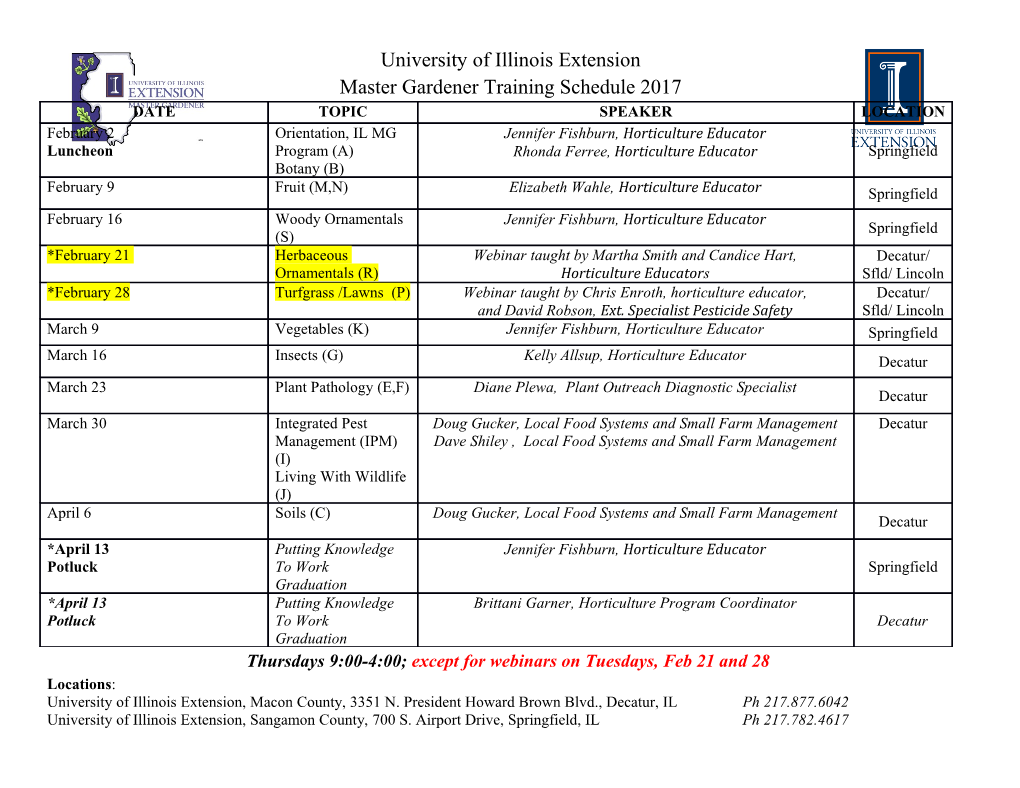
<p> Computational Aspects of the Simplex Method</p><p>MSIS 685: Linear Programming</p><p>Lecture 9</p><p>Professor: Farid Alizadeh Scribe: Shuguang Liu Lecture Date: Nov. 5 1998</p><p>We have addressed the Simplex Method to solve Linear Programming Problem. By introducing matrix notation, we know that solving linear systems can be viewed as proceeding matrix operations such as matrix addition, subtraction, multiply, inverse, etc. When we implement simplex method as a computer program, however, we do not practice matrix operations but solve corresponding equation systems. In order to know how much the computing load of the system is, how we can reduce it and to what degree we can reduce it, first we need some elementary knowledge of computing load. Then we will try to decrease the computation.</p><p>1. Basic to Computing Load</p><p>1.1 Computing Loads of Matrix and Vector Operations Let A,B R nn and U, V R n . (1) UV T is a rank one matrix. (2) Computing A -1 requires o(n 3 ) flops, which means that solving system AX b requires o(n 3 ) flops. We denote this by A -1 o(n 3 ). (3) AB o(n 3 ). (4) AU, U T A o(n 2 ). (5) UV T , U T AV o(n 2 ).</p><p>If L and U denote lower and upper triangle marixs respectively, then decomposing A into L and U requires o(n 3 ) flops : A LU o(n 3 ). Matrix decomposition is three times less time-consuming than matrix inverse.</p><p>1.2 Sherman-Merrison Formula (SMF) Suppose we know A and A-1, we need to compute (A+UVT)-1. We can use Sherman- Merrison Formula, which is as follows A1UV T A1 (A UV T )1 A1 1V T A1U Computing Load of SMF Computing the right hand of the formula needs (n2), because A-1 is known, from (5) computing VTA-1U needs (n2), and it can be shown that computing A-1UVTA-1 also requires (n2) flops. To compute A-1UVTA-1, we can first compute (A-1U) and (VTA-1) and then multiply the results, which are three (n2) operations. So computing A-1UVTA-1 is a (n2) operation. So that, by using SMF, we can reduce computing load of (A+UVT)-1 from (n3) to (n2) flops. </p><p>Proof of SMF Bear in mind the fact that VTA-1U is a number so that we have following equation: UV T A-1UV T A-1 U (V T A-1U )V T A-1 (V T A-1U )UV T A-1 Using the equation above prove SMF as follows: A1UV T A1 (A UV T )(A1 ) 1V T A1U UV T A1 U (V T A1U )V T A1 I UV T A1 1V T A1U 1V T A1U UV T A1 (1V T A1U )UV T A1 (V T A1U)UV T A1 I 1V T A1U I</p><p>2. Using SMF to Simplex Method</p><p>Given the basis, let N and B denote nonbasic and basic parts of matrix A respectively, then BRmm and NRmn. We need to solve equation system AX=b, ARm(n+m), XRn+m, bRm.</p><p>B i1 ,i1 ,⋯im N j1 , j2 ,⋯ jn </p><p>Here i’s and j’s are index of variables.</p><p>To some extend, the simplex method is to continuously construct new basic variable sets in order to find an optimal one. And the new set is different from the old set in just one variable. If we calculate the new equation system based on the new set without considering the information we have got from calculating the old system, we meet another (n3)-flop operation. Fortunately, we can use SMF in the new system derived from the old one.</p><p>2.1 At iteration 1: At this time, we need to compute B-1. 1 3 First, from BX B b X B B b o(m ) Then, compute yT C T B 1 o(m2 ), because B -1 is known. ~ B B -1 N N o(mn) 2.2 At next iteration:</p><p>Bnew B \ ik js , where ik is the index of leaving variable and js is the index of incoming variable.</p><p>0 ⋯ 0 b1r ⋯ 0 0 ⋯ 0 N1 j ⋯ 0 s B B ⋮ ⋮ ⋮ ⋮ ⋮ ⋮ ⋮ ⋮ new 0 ⋯ 0 b ⋯ 0 0 ⋯ 0 N ⋯ 0 nr njs </p><p> bT (b ⋯ b ) aT (N ⋯ N ) Let r 1r nr , which is the rth column of B, and q 1 js njs , which is the qth column of N. These two vectors will change and only change the values of rth column of B to Bnew. T The second and third parts of right hand side of the equation can be written as –brer and T T aqer , where er 0 0 ⋯ 1 ⋯ 0, and the number “1” appears on the rth column T of er . Then we get</p><p>T Bnew B der d aq b r 1 T 1 Bnew (B der )</p><p>Now we can use SMF and have:</p><p>1 T 1 1 1 B der B Bnew B T 1 1 er B</p><p>-1 It can be shown that all elements of Bnew have been calculated at iteration 1, hence need no further calculation. (1) B-1 is known. 1 1 ~ -1 (2) B d B (aq br ) N q er , where B aq has been calculated at iteration 1 and it is ~ -1 -1 the qth column of N , and B br is the rth column of B B=I. T 1 T ~ ~ ~ (3) 1 er B d 1 er (N q er ) N rq , which is an element of N calculated before.</p><p>From section 2.1 and 2.2, we can see that with SMF solving an LP needs one (n3)-flop operation only at the first iteration and (n2)-flop operations at the following iterations.</p><p>3. LU Factorization </p><p>Sometimes even with SMF it is not economic to compute B-1 at the first iteration, because LP matrices (A) are large (in rows and columns) and sparse (each column and row have only few nonzero numbers, and the others are zeros). With these properties we can use LU factorization to reduce computing load even further. 3.1 Gaussian Elimination Using Gaussian elimination to solve a system of equations is to get an upper triangle matrix. Let’s see an example.</p><p>2 1 0 2 2 1 0 2 0 3 0 0 0 3 0 0 X b r1 (2)r4 r2 (2/ 3)r4 0 0 2 1 0 0 2 1 4 0 4 2 (4) 2 4 2 2 1 0 2 2 1 0 2 0 3 0 0 0 3 0 0 r3 (2)r4 0 0 2 1 0 0 2 1 (4) (2) 4 2 (4) (2) (4) 4</p><p>There is a nice property of the last triangle matrix. This property is: </p><p>2 2 2 1 0 2 0 3 3 3 0 0 A LU 0 0 2 2 2 1 4 2 4 4 4 4</p><p>By decomposing A into L and U, we reduce the computing load of solving AX=b from (n3) to (n2).</p><p>LY b 2 AX b LUX b o(n ) UX Y</p><p>If we have U and L, when b changes we just need (n2) flops instead of (n3) to solve the new problem.</p><p>The procedure of Gaussian elimination to a matrix is the procedure of successively multiplying the matrix by lower diagonal matrices, which are essentially identical but excepts one column. The procedure can be shown as follows:</p><p>AX b L1 AX L1b L2 L1 AX L2 L1b ⋯ (Ln1 ⋯L2 L1 A)X (Ln1 ⋯L2 L1 )b</p><p>3 At last we get an upper triangle matrix: (Ln-1L2L1A). These operations require (n ) flops. It can be proved that the results of lower triangle matrices multiplication is still a lower triangle matrix.</p><p>Lemma: If L1, L2 are lower triangle matrices, then L1L2 is also a lower triangle matrices. Proof: ~ ~ L11 0 L21 0 Let L1 ~ ~ L2 ~ ~ , then we multiply L1 and L2: B1 L12 B2 L22 ~ ~ L11L21 0 L1L2 ~ ~ ~ ~ ~ ~ B2 L12 B1L21 L12 L22 </p><p>By generalization it is easy to prove that this matrix is a triangle matrix.</p><p>3.2 Permutation Matrix It is always the case that the number of zeros in the LU matrices after LU decomposition is decreased. We know that we should keep as many zeros as possible in order to make further computation efficient. Thus we need matrix permutation. The other reason we need permutation is to make Gaussian Elimination possible and accurate. From last section, a Gaussian elimination operation is to pivot a diagonal element, as show below:</p><p>1 a12 a13 a14 a15 1 a a a 23 24 25 </p><p> a33 a34 a35 a43 a44 a45 a53 a54 a55 </p><p>To this matrix we need pivot element a33. If a33 is zero, we have to rearrange row or/ column to put a nonzero element in this position. If a33 is small, pivoting it means divided by a small number, which will result in a big number hence losing accuracy. So we need to pivot say a44 by exchange row 3 and 4 and column 3 and 4. Switching row and/or column can be done by pre- and/or post-multiplying a permutation matrix.</p><p>Permutation matrix is a matrix of which each row and column has only one nonzero element 1. Pre-multiplying a permutation matrix changes the position of rows, while post-multiplying a permutation matrix changes the position of columns</p><p>We can rearrange rows and columns before operating Gaussian elimination. At this time the Gaussian elimination procedure can be shown as:</p><p>AX b P1 AX P1b L1P1 AX L1P1b ⋯ (Ln1Pn1 ⋯L2 P2 L1P1 A)X (Ln1Pn1 ⋯L1P1 )b How to choose a pivoting element is a complicated problem, which is even more complex than LP itself. However, there is a heuristic rule we can follow.</p><p>Minimum-degree heuristic (quoted from textbook): Before eliminating the nonzeros below a diagonal pivot element, scan all uneliminated rows and select the sparsest row, i.e., that row having the fewest nonzeros in its uneliminated part. Swap this row with pivot row. Then scan the uneliminated nonzeros in this row and select that one whose column has the fewest nonzeros in its uneliminated part. Swap this column with the pivot column so that this nonzero becomes the pivot element. (Of course, provision should be made to reject such a pivot element if its value is close to zero.)</p><p>4. Updating Factorization </p><p>From section 3, using LU factorization we reduce computing load of the first iteration by three times. Now we will address how to use information we get from LU factorization at the first iteration to decrease computation at the following iterations.</p><p>With BXB=b and B=LU from the first iteration, at the next iteration we construct a new B and will get LU factorization of this new B. If we work with another factorization, it needs (n3) flops to compute. Fortunately we can construct new L and U without actually doing factorization again.</p><p>We know from section 2.2 that:</p><p>T Bnew B (aq b r )er 1 1 T L Bnew U L (aq b r )er ~ T U (aq U r )er In above equation:</p><p>~ 1 aq L aq 1 U r L b r ~ T ~ (aq U r )er 0 ⋯ aq U r ⋯ 0</p><p>-1 The nonzero column vector on the rth column of L Bnew so that we have:</p><p>1 ~ L Bnew U1 ⋯ U r1 aq U r1 ⋯ U m </p><p>By permutation we change the rth column to the mth column, and we get:</p><p>1 ~ L Bnew U1 ⋯ U r1 U r1 ⋯ U m aq </p><p>Now this matrix looks like: 1 X X X X X X 1 X X X X X X X X X X 1 X X X X 1 X X X 1 X X 1 X</p><p>In order to obtain new L and U from this matrix, we need to pre-multiply a special lower triangle matrix. This special matrix essentially is an identity matrix except one column and looks like:</p><p>1 1 ⋱ ⋱ 1 Er Er er ⋱ er ⋱ 1 1</p><p>The nonzero element er is on the rth column. By continuously multiplying Er matrix, we have:</p><p>1 ~ Er L Bnew P Er B 1 ~ En1 ⋯Er1Er L Bnew P En1 ⋯Er1Er B</p><p>At last we get an upper triangle matrix. From this triangle matrix we can derive new L and U.</p><p>1 1 2 ~ 2 En1 ⋯Er1Er L Lnew is a (r ) operation. And, En1 ⋯Er1Er B U new is another (r ) 2 operation. As a whole, getting Bnew P LnewU new needs (n )-flop computation.</p>
Details
-
File Typepdf
-
Upload Time-
-
Content LanguagesEnglish
-
Upload UserAnonymous/Not logged-in
-
File Pages7 Page
-
File Size-