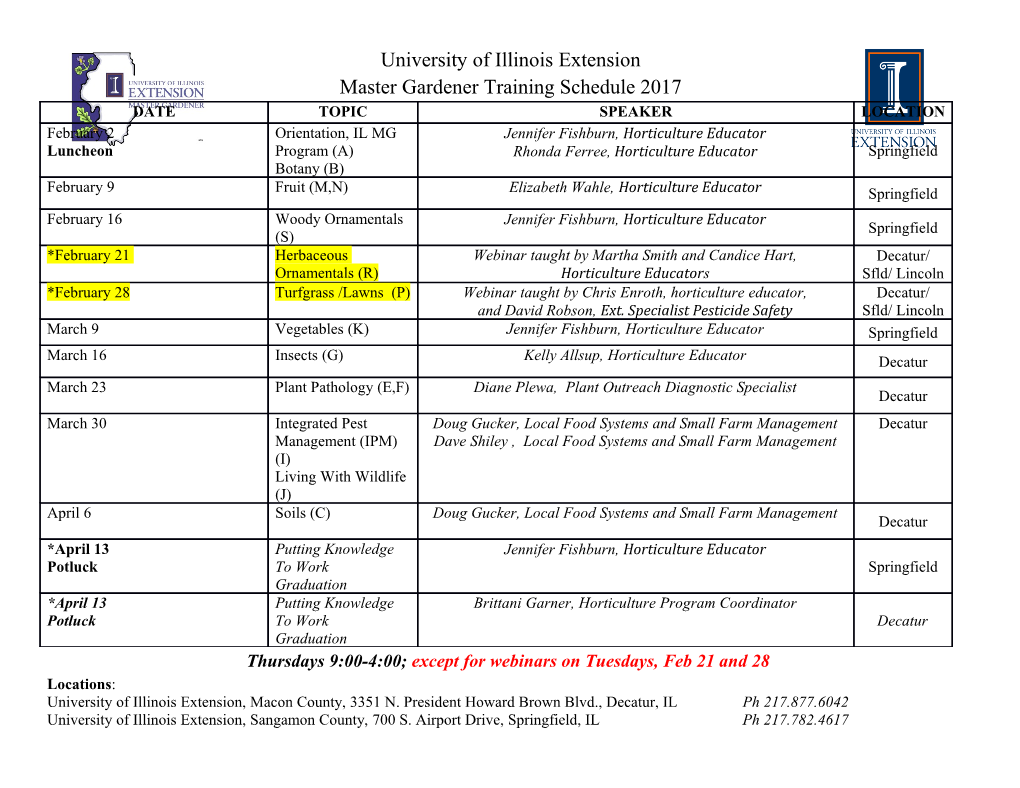
<p> 1</p><p>CHAPTER EIGHT (Complete Rewrite 04/10)</p><p>LEARNING FROM EXPERIENCE</p><p>“We define the art of conjecture, or stochastic art, as the art of evaluating as exactly as possible the probabilities of things, so that in our judgements and actions we can always base ourselves on what has been found to be the best, the most appropriate, the most certain, the best advised; this is the only object of the wisdom of the philosopher and the prudence of the statesman.” Jacques Bernoulli, ‘The Art of Conjecture’ Basle, 1713</p><p>All sane persons, scientists included, learn from experience. Indeed the certainty scientists attach to some idea usually results from the accumulation of experience they have acquired as a group when testing that idea in practise. An idea that has been tested out thousands of times by dozens of independent scientists is eventually accepted as “A Scientific Law”, so that hardly anybody bothers to test it further. Strangely enough, although learning from experience is the main mode of scientific progress, it is not one the community is prone to discuss at length – or agree about when they do. The reason for this diffidence may partly be that learning from experience is so commonplace, so instinctual, that conscious analysis of it seems unnecessary. After all animals do it very successfully all the time without having to read books about it. Your dog knows from experience when you are going for a walk, just as your cat knows when it is her turn to be fed. Nevertheless if Science is to deserve the claim that it is based on observation and experiment then surely it must be able to lay bare the processes by which it moves from such primary data to its final conclusions. And there are two levels of process here. First how do individual scientists or teams move from their observations to their conclusions. Second, how do the experts in a particular scientific field reach a collective decision as to the truth or otherwise of a claim – say global warming. This last collective process will be the fascinating business of Chapter…. Here we concentrate on the lone thinker (or team), scientific or otherwise, who must try to reach wise decisions on the basis of the usually incomplete and imperfect data before them. 2</p><p>The hope must be that if we can find out how we as human beings learn from experience we may possibly learn to do it better and to avoid some serious pitfalls into which even famous thinkers have been prone to tumble. The extraordinary fact is that we are still not entirely sure how scientists do learn from experience. Rather, there are fiercely contending schools on the matter who debate how it is actually done, how it ought to be done, and even whether it can be done at all. Most practicing scientists, it has to be said, just get on and do science without attending to such debates. They apparently use the kind of everyday hunch- thinking that serves the rest of us. That is all very well until they have to explain themselves to their argumentative colleagues, or to the public at large. An atmospheric scientist who got up to plead for a carbon tax, on the grounds that he had a private hunch that global warming was taking place, would be rightly laughed off the stage. He or she must thoroughly understand their own thinking processes before they can expect anyone else intelligent to follow them. The end point of a successful piece of scientific research is a paper published in a reputable scientific journal for all to see. Before he publishes it the editor will want to make sure that the paper is ‘sound’. To that end he will send it to a couple of ‘experts’ in the field called ‘referees’, asking for their opinions. Most often it will have to be modified, sometimes drastically, to meet the referees queries or objections, and even then it may finally be rejected as unsound, or insufficiently interesting. Were a paper to begin “I was lying in my bath playing with my rubber duckie when suddenly the idea of hydrostatic balance leapt into my head. I jumped out of the bath crying ‘Eureka’…..” it would certainly be rejected today – at least in that form. Why? Because the essence of science is that it must be reproducible. Others must be able to affirm that if one carries out an identical observation or experiment, the result will be as claimed in the paper. Playing with a rubber duckie will not necessarily produce the same effect on Doctor X as it did on Archimedes. So it must be left out as extraneous to the science because it is neither persuasive, nor reproducible — even if it is natural and charming. A conventional scientific paper [See example Watson and Crick’s famous DNA paper in Appendix ] generally consists of a careful description of some evidence (say an experiment), and an argument as to why that evidence supports one hypothesis (idea) rather than another, together with some quantitative estimate as to the degree of statistical certainty that the argument implies. Any persuasion beyond that is generally, though not always, frowned upon. 3</p><p>One can hardly argue with a convention that has served science so well, and I certainly do not. At the same time it makes it almost impossible for anyone outside the team of authors to actually decipher what went on in their heads at the time: what their thinking processes actually were, as opposed with what they were allowed to report in their published paper. Almost certainly they went up some blind alleyways, made mistakes, misread the literature, consulted colleagues, had internal arguments etc etc before they settled on the final account that satisfied the referees. One has only to compare Watson and Crick’s terse original paper, with Watson’s racy account in his “Double Helix”, to be convinced of all this. In that sense, as one distinguished practitioner claimed ‘The scientific paper is a fraud’. Wisely so maybe. But that makes it hard for any author to reconstruct how scientific thinking really works. I am therefore going to assume for now, based on working with a lot of fellow scientists, that our learning from experience takes a particular form based, unconsciously, on gambling. This will allow us to get deep into some instructive examples, to learn the terminology, and to spot some of the controversial issues. Near the end, I shall point out some objections to this theory of learning. What we aim to do is tease out the nature of thinking by appealing to some more or less realistic stories about thinking in different contexts, from horse-racing to the Stone-Age, from a marital row to galaxies, from a cancer scare to Flying Saucers. In the process we will discover some surprising, but valuable lessons: that even honest scientists can still disagree, and disagree wildly, about the implications of the same evidence; that piling on more evidence won’t necessarily bring about a convergence of opinion – on the contrary; that one can’t avoid prejudices; indeed one can’t make up one’s mind without them. But there is a price to pay; if your prejudices are wrong, or too strong, you will have to pay with your life; or at least with some of it .</p><p>THINKING LIKE A GAMBLER. Gamblers, at least professional gamblers, have to think straight – otherwise they quickly go out of business. So something might be learned through studying their thinking. My old friend Stella has a very extensive interest and knowledge of horse racing, steeple-chasing in particular. Over the course of a season she places dozens of judicious bets and, more often than not, wins more than she loses – usually enough to pay for an extravagant annual family holiday. And she never misses betting on The Grand National, that Everest of the steeple-chasing calender. With forty or more horses, thirty 4</p><p> fearsome fences and a course over 4 miles long it has everything, not least drama as horses fall, hurling their riders under the hooves of the thundering field. For months in advance Stella studies the form of the declared runners and places</p><p> odds in her head ( and in her notebook) on likely prospects, with O(H j ) being her odds</p><p> on a particular horse j winning the National; i.e. her hypothesis H j is ‘Horse j will win the National’. She will have several such notional bets in her head long before the race</p><p> is run.</p><p>As the season progresses some prospective runners do well and win steeple-chases whilst others fail too often to appear serious National contenders. If she is to bet successfully, all this evidence from previous races has to be incorporated into Stella’s thinking. For instance Wavehunter, one of the horses she is interested in for the National, is to run in The Gold Cup, another marathon steeplechase held some months beforehand. He wins it ! How does she incorporate this new evidence E into her odds on her hypothesis</p><p>HW that Wavehunter will win the National? What she does is go back into her extensive library ofrecord books to find out, historically, what bearing a win in the Gold Cup has had on subsequent success in the</p><p>National. She discovers that National winners have been 5 times more likely than other National runners to win the previous Gold Cup. In the parlance of Probability, where you will remember P(A|B) means ‘the Probability of A given B’, she can therefore say P(E | H ) W = 5 P(E | H W )</p><p> where E is the new ‘Evidence’ i.e. winning the Gold Cup; HW is the hypothesis that</p><p>Wavehunter will win the subsequent National, H W that he is not going to win it</p><p>[remember a bar on top of H for Hypothesis means ‘not-H’ , see p ].</p><p>So given this new evidence Stella’s odds on Wavehunter , ie her O(HW | E),[ i.e.</p><p>‘her new odds on Wavehunter given the Evidence E’)] ought to be 5 times greater. But greater than what? Greater than all the other horses in the race? Hardly, because some</p><p> of the best prospects didn’t even run in the Gold Cup. And hardly because some of the prospective runners are such outsiders that surely Wavehunter was a 5 times better bet than they were even before he won the Gold Cup. What Stella actually does, so she tells me, is multiply by 5 the odds she already had in her head on Wavehunter before the Gold Cup was run; i.e. she writes 5</p><p>P(E | HW ) O(HW | E) = ҙ O(HW ) = 5 ҙ O(HW ) (1) P(E | H W ) That seems sensible to me because the evidence of Wavehunter’s win bears only on him, not on the other prospective runners, many of whom didn’t even run in the Gold</p><p>Cup. Since she had Wavehunter at 25-1 against before the Gold Cup (i.e. 1 to 25 on) she therefore calculates his odds-on afterwards as:</p><p>O(HW | E) = 5 ҙ (1 to 25) = 5 to 25, or 1 to 5 on [or ‘5 to 1 against’ in the usual terms]. What she does then is go round looking for bookies who will offer her odds better than 5-1 against. If she can get 10-1 then, she reckons, she is likely to win twice as often as she will lose. And so her experience has proved over the years. Indeed the last time I rang she was about to take her entire extended family, including grandchildren, for a month’s holiday in The Seychelles. THINKING LIKE STONE-AGERS. After she told me all this, rather diffidently for she is no mathematician, I decided to look into all my books on Probability Theory to see if Stella’s Equation had some pedigree, and to advise her if she was acting in an optimal way. It took some digging but I eventually discovered that Stella’s Equation (1) was known already [we actually proved it at the end of chapter six] and is referred to, but only rarely so, as ‘The Odds form of Bayes Theorem’. This theorem is usually written in another guise (see later), and as such goes back to 1763 when it was discovered at Tunbridge Wells in the posthumous papers of a certain Reverend Thomas Bayes entitled ‘An Essay Towards Solving a Problem in the Doctrine of Chances’. The more I thought about it however the more I realized that it had to be far older than that, for surely it is no more than Common Sense. Even if they weren’t aware of it in a formal sense, and of course they weren’t, Stone-agers must nevertheless have been using it informally to learn from their experiences and act rationally. To see how, let us imagine ourselves back 100,000 years in a small Palaeolithic band. Having inadvertently trespassed and hunted on some other and larger band’s territory we are now being pursued by them with murderous intent. How might Stella’s Equation have helped us to escape? We reach a valley which we desperately need to cross. Unfortunately it is being grazed by mammoths, enormous beasts we have never encountered before. Overawed by their size and their tusks we must estimate whether they are dangerous or not. Since they are obviously herbivorous, and apparently cumbersome, the majority opinion 6 among the 4 seniors of the band is 3 to 1 on that ‘Mammoths are harmless’. That is our working hypothesis as we cautiously set off down the hillside to cross the valley. At first the mammoths are oblivious, but then the giant herd-bull, previously invisible behind some trees, charges out trumpeting with rage. Targeting one unfortunate old lady he chases her so fast through the long grass that she only narrowly makes the safety of the rocks. The tribe assembles to reconsider the situation. We still desperately need to cross the valley for there are enemies in close pursuit, so that making a detour could itself prove fatal. In reconsidering our options the tribe now have some evidence E to go on. One mammoth at least looks vicious, even if the rest appear docile. It is time to re- evaluate our hypothesis H that “Mammoths are Harmless” in the light of the experience E that one of them has charged. We certainly would not have made an explicit “Stella Calculation” but a rational discussion among ourselves would have amounted to much the same thing. In order to arrive at a rational assessment of the new O(H |E) they would need to arrive at estimates for P(E| H) and P(E | H ) . Now P(E| H) is the probability that mammoths will sometimes charge (=E), even though they are harmless (=H). The tribe have encountered such behaviour before among other large beasts such as oxen, which make a tentative charge but then turn tail when challenged boldly enough. Perhaps if the old lady hadn’t run away the bull mammoth would have behaved more politely. Perhaps. It may seem unlikely, but it has to be considered because the tribe simply must make its escape from pursuit. The Stoneagers decide, on the basis of previous experiences with large herbivores, that P(E|H), that is to say the probability of mammoths making mock charges like oxen, is a pessimistic 1 in 20 or 0.05. Finally P(E | H ) is the probability that mammoths will charge, given that they are dangerous. This must be high but is not necessarily certain [ i.e. =1]. Mammoths might have poor vision, or be docile when well fed, or ignore you if you are down wind. A rough guess at P(E | H ) might therefore be 9/10, which amounts to saying that mammoths, if indeed they are dangerous, will nearly always charge if approached, but one time in 10 = 1/10 = 0.10 will leave you alone. We would now be in a position to re-evaluate our hypothesis, initially thought likely, that mammoths are harmless because now: 7</p><p>P(E|H) =1/20; P(E| H ) =9/10 and O(H) was 3 to1 on, or 3. Were we to think like Stella we would then fill in her equation (1 ) as P(E | H) 1/20 .05 O(H | E) = ҙ O(H) = ҙ 3 = ҙ 3 =1/6 P(E | H ) 9/10 0.9 i.e. 1 to 6 on, or 6 to 1 against. This calculation appears to give the kind of answer we might have expected intuitively – after the event E. It seems to embody “Learning from</p><p>Experience” in a sensible way. Unfortunately odds of 6 to 1 against are not decisive. With our children exhausted, and murderous pursuers closing in behind, we cannot afford to ignore the 1 to 6 chance of making our escape across the valley unscathed. A second attempt is therefore made, and a second time the bull charges malevolently. Does that mean that the odds are now 12 to 1 against us crossing safely – in which case it might be worth making a third try – or what?</p><p>With this second piece of evidence (E 2) Stella’s calculation could be updated in the light of it as follows. As before P(E|H) =1/20 and P(E| H ) =9/10 but now O(H) must be amended to 1 to 6 or 1/6 , as previously calculated. In that case Stella’s equation:</p><p>P(E2 | H) 1/20 .05 1 O(H | E2) = ҙ O(H) = ҙ O(H | E1) = ҙ » .01 P(E 2 | H ) 9/10 0.9 6 Because .01 is one one-hundredth, the odds on H have dropped to roughly 1 to 100, or 100 to 1 against.</p><p>The crucial factor in Stella’s equation, or ‘Bayes Theorem’ as we shall refer to it henceforth, is the so called “Bayes’ Factor” P(E | H)/P(E | H ) which multiplies our odds on the hypothesis, i.e. O(H), to bring it up to date in the light of some new evidence E. We Stone-Agers were in no position to make precise estimates of either of </p><p> the Probabilities P(E|H) [that mammoths are basically harmless, although they charge] or P(E| H ) [that they are dangerous and will always charge] but we took a pessimistic view in both cases [1/20 and 9/10 respectively]. Since the Bayes Factor is the former (1/20) divided by the latter (18/20) it reduced our odds on H (“Mammoths are </p><p> harmless’) by no less than 18 each time we were in fact charged. Thus two charges sufficed, in this case, to be decisive. There is something rough and ready about Bayes’ Theorem which allows one to take crucial decisions even when much remains uncertain, or imprecise. But if it is logically based, and Bruno di Finetti’s argument in Chapter Six demonstrates that it is, then it may have been a vital survival mechanism on our prehistoric journey. And as 8 such Darwinian selection may well have hard-wired it into our mental system. Thus scientists could learn from experience (in the form of data, observations or experiments) in the same way as the rest of us, i.e unconsciously, using Bayes’ Theorem, without being able to explain themselves. Perhaps all we survivors (not only humans) think like Stella, think like gamblers, because Life is, and has always been, a gamble. In summary then, there is a way of thinking which would allow us to learn from experience. It is based on The Gamblers formula, otherwise known as Bayes’ Theorem, that Posterior odds-on = Bayes’ Factor times Prior odds-on. (1) Where, you will recall, the ‘Bayes Factor’ was P(E|H)/ P(E| H )</p><p>The terms ‘Prior’ and ‘Posterior’ are very useful, and so we shall use them extensively henceforth. ‘Prior’ refers to the odds-on our hypothesis prior to, or before, we considered some specific evidence E i . Likewise “Posterior’ refers to our odds-on after , or posterior to, us considering that same evidence E i .(Thus ‘Prior’ and ‘Posterior’ can only have meaning relative to some particular piece of evidence.)</p><p>It’s all very well talking about gamblers and Stone-agers, but do we actually use </p><p>Bayes’ Theorem, albeit unwittingly, in our everyday lives? One cannot prove it either way, but the next example appears to show that everyday thinking, and Bayesian thinking, mirror one another rather closely THE CASE OF THE MISSING WIFE. A married couple were having breakfast when a post-card from Australia dropped through the letterbox. An old flame of the wife, returning to Britain for a few days, was inviting her out to dinner that very evening. An almighty row ensued with both parties banging out of the door vowing never to speak to one another again. At 7pm the husband returns from work to find an unusually darkened house. By 9.30,with still no sign of his wife, the jealous husband begins to ponder whether she really has left him. The idea, the hypothesis H that ‘She has run away with X’ begins to form in his mind, but with a low prior odds of O(H) =1/100 =.01 because they have been reasonably happily married for nearly a year.[ O(H) can only be a very rough guess – as it is. He might feel O(H) is not as high as 1 to 10 on, nor as low as 1 to a thousand, so an O(H) of 1:100 on, or 1%, is an intermediate compromise.] All the same it is something, though not much of a coincidence that she should be out tonight 9</p><p> because 3 nights out of 4 she will be home before him – the other 1 in 4 being either </p><p> delayed at work, or visiting family or friends. How much should the evidence (=E1) that she is not home tonight worry him? He knows that P(E| H) =1/4 whereas P(E|H) =1 i.e. she would certainly be absent if she had actually run off with X. If he cared to, the husband could update his odds on H (his worry) using a Bayes calculation: </p><p>1 O(H | E1) = ҙ 0.01 = 4 ҙ 0.01 = 0.04 0.25 or 1 to 25 on. In other words the odds are now 25 to 1 against her having run away. When she still isn’t back by 10.30 he rings her best friend, to find that she is not </p><p> there either (=E2). This is worrying because if she was out but hadn’t run away he would have expected, from past experience, to find her there 3 times out of 4, so that </p><p>P(E2| H) =1/4 =0.25. And of course if she’s run away P(E2|H) =1 while his Prior has now been updated to 0.04 So his updated (posterior) odds on her having run are:</p><p>P(E2 | H) 1 O(H | E 2) = ҙ O(H | E1) = ҙ .04 = 4 ҙ .04 = 0.16 » 1/6 P(E 2 | H ) .25</p><p> i.e. the odds against H are now only 6 to 1 Not so reassuring!</p><p>Alarmed now, he begins desperately seeking for new evidence. He finds she is not at</p><p> her mother’s [=E3 with P(E3| H ) =0.25], she is not her sister’s [=E4 with P(E4| H )=0.5] </p><p> and he finds that all her credit cards are apparently missing [=E5 with P(E5| H)=0.1]. Each successive discovery progressively increases his worry, i.e. increases his posterior</p><p> odds that H is true, as follows: After E3 , O(H|E3) =0.64 ; after E4 , O(H|E4) =1.25 (i.e. </p><p>5 to 4 on); after E5 , O(H|E5)=12.5 or about 13 to 1 on. Readers should try to reach these same figures, or roughly similar ones, for themselves by using Bayes’ formula over and over again (a simple calculator helps).You can check your workings against my own arithmetic laid out in Table (8: 1) – later. These calculations seem to track the kind of ‘common-sense reasoning’ that might naturally pass through our minds when we are faced with such an unpleasant situation. With all the credit cards missing suspicion runs high . The odds in his mind are now roughly 13 to 1 on the hypothesis that his wife has, as she threatened, deserted him. Thoroughly alarmed he dashes up to the attic to find if her suitcase is missing. It is </p><p> not [=E6, P(E6| H) =1; P(E6|H)=0.2 because he feels there is a 20 per cent she would </p><p> purchase another], so that his posterior drops to O(H|E6) =2.5 or 5 to 2 on. Then he </p><p>10</p><p> remembers the boyfriend is returning to Australia so he searches and finds (E7) his wife’s passport [P(E7| H)=1; P(E7|H) =0.1] yielding O(H|E7) = 2.5/10=0.25 .Things are looking up again and a more thorough search for the missing credit cards finally locates them in her spare handbag [P(E8|H) =0.1; P(E8| H) =0.9] leading to a reassuring O(H|</p><p>E8) =0.25/9 0.03. In his mind there is now 1/.03 or 33 to 1 against her having left him. At midnight he goes to bed and sleeps soundly.</p><p>A second, Truly Jealous Husband, going through exactly the same set of experiences, will come to very different conclusions. To begin with, his prior – his initial suspicion of infidelity – is much higher; his initial O(H) =0.1 as against a prior of 0.01 for the first husband. Then again he is much less willing to let his wife out alone at night [his P(E1| H) =0.02] and at every stage he is less inclined to give her the benefit of the doubt [e.g. P(E5| H) =0.02 when he cannot find her credit cards]. Indeed [see </p><p>Table (8: 1) ] by the time he discovers she is not at her sister’s (E4) his odds are already </p><p>80 to 1 that she has absconded with her old boyfriend. Even the subsequent discoveries of her suitcase (E6), her passport (E7) and her credit cards (E8) cannot significantly change a mind so swayed by the previous evidence. Far from going to sleep he goes round to the boyfriends hotel and assaults him. At the other extreme the Complaisant Husband is so certain of his wife’s fidelity [prior O(H) = 0.000001, or 1 in a million] that nothing of exactly the same evidence disturbs his equanimity in the slightest [Table(8: 1)] . Indeed it is doubtful, even if her passport and suitcase had proved to be missing, that he would have lost a wink of sleep [you can calculate for yourself that his O(H|E) would never exceed one in a hundred]. There are two conclusions I wish to draw. Firstly that a Bayesian calculation does in fact closely mimic what passes through our minds when confronted by evidence or experience. In so far as we behave rationally we may be making Bayesian calculations somewhere in our psyche all the time. Our prejudices (or ‘Priors’ if you prefer) are naturally strengthened by experiences that are consistent with them, weakened by experiences with which they conflict. Secondly that the conclusions we draw, the judgements we make, and the actions we take, may be as heavily, if not more heavily, affected by the Priors we adopt, than by the evidence or experiences of life. The three husbands, although presented with identical evidence, come to wholly different conclusions in this, admittedly exaggerated, case. And who is to say which of them was right; which of them chose the better Prior, one which presumably rested in each case 11 on an instinctual judgement of his wife’s personality and of the strength of their marriage? One could argue that, in a case of this sort, such instinctual evidence should indeed carry far more weight than circumstantial evidence evaluated by calculation. In so far as it models scientific thinking Bayes’ Theorem would enable scientists to learn from experience or evidence whilst, at the same time, allowing them to incorporate instinctual Priors. Some scientists regard these as virtues, indeed as essentials. Others, including many philosophers, mathematicians and statisticians, throw up their hands in horror. Scientific thinking, they assert, is not to be compared with that employed by gamblers and cuckolds; it must surely float on some higher plane, driven by Reason, accompanied by Dispassion, and sanctified by Mathematics. Perhaps.</p><p>12</p><p>TABLE (8.1) THE CASE OF THE MISSING WIFE; BAYES’ ODDS CALCULATIONS</p><p>HUSBAND Ord Ord Ord Ord Jeal Jeal Jeal Jeal Compl EVIDENCE Prior P(E|H) P(E | H) Post Prior P(E|H) P(E | H) Post Prior -erior -erior</p><p>E1 Not at home .01 1 .25 .04 0.1 1 .02 5 .000001 E Not at friends’ .04 1 0.25 .17 5 1 0.5 10 .000004 2 E 3Not at mothers’ .17 1 0.25 0.64 10 1 0.25 40 .000064</p><p>E 4 Not at Sisters’ 0.64 1 0.5 1.25 40 1 0.5 80 .00026 E 5No credit cards 1.25 1 0.1 12.5 80 1 0.02 4000 .00052 E 6Suitcase found 12.5 0.2 1 2.5 4000 0.5 1 2000 .0052</p><p>E 7Passport found 2.5 0.1 1 0.25 2000 0.5 1 1000 .001</p><p>E 8Cards found 0.25 0.1 0.9 0.028 1000 0.5 0.9 560 .0001</p><p>13</p><p>THE CASE OF THE DREADED CANCER TEST . When Mrs. Jones received the letter below she and her family were naturally devastated. They knew, and this was confirmed by reading the family’s medical encyclopaedia, that cancer of the woozle is an agonising and invariably fatal disease. And now she appeared to be exhibiting the first signs of it. According to the encyclopaedia she had less than a year to live, and there was little or no hope of a successful operation. The only hope that appeared to remain, and it was a very slim one [apparently a 2% one from the letter] was that her test result was wrong. Pathological Analysis Laboratory North East Glamorgan Health Authority, Our Ref CA/ 41/RSD/15/03/05 Abercwmboy, Glamorgan BN23 7EH</p><p>March 15 2005</p><p>Dear Mrs Jones A specimen of your blood was forwarded to us by your medical practitioner on Feb 26 last to test for the presence of carcinoma lymostrophia woozeli.We are writing to inform you that the test has now proved positive. In accordance with Health Authority guidelines [2001/07/1453c] we are obliged to inform you of the reliability of such a test, as established by the UK Medical Statistics Bureau in 1998.They find: Reliability of identifying the disease when it is present : 95% Probability of finding a positive result when the disease is in fact absent : 2%</p><p>You are advised to get in touch with your medical adviser(s) immediately.</p><p>Yours sincerely R.S.Davies BSc., PhD., F.R.I.C., M.P.S., D.C.O.G.</p><p>For The Authority.</p><p>While she was waiting to see her ‘medical adviser’, a process, which can take ages in Britain, the poor woman and her entire family, were traumatised. Fortunately however, one of her nieces was studying medical statistics at university. When she heard about the tragedy the niece did a Bayesian calculation of her own. She realised that the true implication of the letter depended on how common cancer of the woozle actually is in the population. She couldn’t find this out in a hurry but as no one in the family had heard of anybody who was suffering from, or had died of cancer of the 14</p><p> woozle, she guessed that the incidence among the general population was very low, say one in a thousand or less. So her Bayesian calculation looked like this: Hypothesis H = ‘Aunt has disease’. Evidence E = positive test. Prior 0(H) = 0.001 (general incidence in the population). And according to the letter P(E|H) =0.95. Also according to letter P(E| H ) =0.02 (i.e. the test has a 2 percent chance of showing a positive result when in fact the disease is not present). Thus: 0.95 O(H | E) = ҙ .001 = 0.0475 (i.e.less than 5 per cent! 0.02 Overjoyed she rang up her aunt to explain that the odds-on her actually having the disease was less, and probably much less than 1 to 20. Because cancer of the woozle is </p><p> so rare in the population at large there will 20 times as many tests which give false positive results as true positives [for that reason the test would not generally be used – at least not on its own.]. What this case illustrates is the danger of leaving out prior information, in this example the information that the disease is rare. While prior information may be contentious or uncertain (as it was with the missing wife) leaving it out can betray one just as well. Notice that the niece didn’t have to know an exact Prior: it sufficed to know that the Prior O(H) was very small, i.e. less than 0.001. In this instance, to be absolutely sure, the family decided to have a second test </p><p> carried out immediately at a private clinic in London. Unfortunately it (E2) turned out to be positive too and when the niece did her calculation this time, and with the new Prior arising from the Posterior in the first test i.e. with O(H) =0.045 she found: </p><p>P(E 2 | H) .95 O(H | E2) = ҙ O(H) = ҙ .045 = 2.1 P(E 2 | H) .02 In other words the odds were now 2 to 1 on her aunt being mortally sick. To be absolutely certain they rushed her to another private clinic in Cardiff where </p><p> alas too the test (E3) proved positive, resulting in a Bayesian probability this time of: 0.95 O(H | E 3) = ҙ O(H | E 2 ) = 47.5 ҙ 2.1 » 100 0.02 which amounted to a virtual sentence of death. When the desperate woman finally got to see her family doctor he had had time to </p><p> do his homework. He knew that the first test was far from conclusive while the second 15 and third might be worthless. Worthless because it was known that a woman in menopause, who additionally suffered from low blood sugar, would always give a false positive test for cancer of the woozle. He tested her blood sugar on the spot, found that it was indeed very low, and sent her home reassured once again. An alternative type of test carried out later showed that in fact she didn’t have cancer. The problem with the second and third Bayes calculations here was not that they were invalid per se but that they incorporated an unconscious natural Prior that was in fact wrong, ie that ‘false positive tests arise at hazard, in a random manner’ when in fact they may be certain to arise in particular patients (i.e. those in the menopause with low blood sugar). So Priors can be extremely significant, easy to neglect, and easier still to slip in unconsciously. One of the great virtues of doing a Bayesian calculation is that it forces one to be clear about one’s Priors, and to bring them out into the open. Many an argument can be cleared up, if not settled, by recognising exactly why the two sides differ. Unknown to each other, unknown even to themselves, protagonists may have been arguing on the basis of different Priors. If they can reconcile their Priors they might come to agree; or if they still cannot then they can agree to differ – and know exactly why. The argument has shifted ground to a discussion of Priors –which can be very constructive. THE CASE OF THE MIRACULOUS CURE. Young Mr Smith has developed a most unfortunate condition, let’s call it Tiptuppititis, which his doctor can do nothing about. It causes acute suffering, painful embarrassment and a general lowering of self- confidence that affects his entire life. Desperate for relief he searches high and low for a remedy. Many are advertised and after discrete inquiries he settles for the Organocalm treatment that, although very expensive, sounds plausible and claims a 90 per cent success rate. Impressed by many ecstatic testimonials from grateful ex-patients to be found on the Organocalm website Smith takes out a loan and starts on a course of treatment. Sure enough it is entirely successful. A few treatments are enough to demonstrate the efficacy of Organocalm and by the end of the course Smith is restored to perfect health and jubilant self-confidence. He posts his own paean of praise on the Organocalm website and becomes a rabid proselyte for the Organocalm system among his acquaintances. And why not? Organocalm has worked for him and turns out to be just as successful amongst his fellow sufferers. The founder of the Organocalm method 16</p><p> eventually becomes rich and famous. His annual books sell in millions; television hosts seek his views on everything from slimming to salvation. Let us examine Smith’s thinking in Bayesian terms. The hypothesis under examination is: H = ‘Organocalm is a reliably successful treatment for Tiptuppititis’. While the evidence in favour of it is: E = ‘Jones has recovered completely.’ He started with a completely open mind, granting even odds on both alternatives i.e. that H and its antithesis H (that it is not a reliable treatment) have odds O(H)=O( H )=1 because he assumes P(H) = 0.5 and P( H )= 0.5. We can now write Bayes’ Theorem as: </p><p>P(E | H) O(H|E)= ҙ 1 P(E | H)</p><p>If H is true, that is to say if Organocalm is a reliably successful treatment, then the </p><p> probability that Smith would recover i.e. P(E|H), must be close to 1.Thus,to an excellent approximation: 1 O(H|E) » ҙ 1 P(E | H)</p><p>What is the remaining unquantified P(E| H)? It is the probability that Smith would have recovered as promptly even if H were true i.e. even if Organocalm is not an </p><p> effective treatment. The problem for Smith is that he cannot know P(E| H) because he </p><p> does not know whether or not he would have recovered spontaneously even without </p><p> taking Organocalm. For him the experiment can be done only once, and it has proved </p><p> totally successful. Granted his prompt recovery he feels it most unlikely that the treatment and the recovery are unrelated. If he has to choose between the extremes of setting P(E| H) =0 and P(E| H) =1 he might reasonably select the former, in which case, according to Bayes: 1 O(H|E) » which is infinite! 0 i.e. the odds on H given E, are so high that H must be almost certain! He has concluded that Organocalm definitely is a reliably successful treatment for Tiptuppititis. [Even a </p><p>P(E| H) = 0.1, i.e. a 10 percent chance of spontaneous recovery, yields odds-on the efficacy of Organocalm of 10 to 1.] </p><p>17</p><p>We have now encountered one of the most plausible, the most confusing, the most treacherous and the most damaging fallacies in human thought. Starting with an open mind, unquestionable evidence, and the best of intentions Smith has arrived at certainty, or virtual certainty, where in fact none whatever was justified. Thus reputations have been made, fortunes amassed, patients slaughtered, economies wrecked, innocents punished, witches hunted down, parties and religions founded, wars declared, minorities persecuted, uncounted numbers tortured and killed, all on account of what I shall call, for want of a better name “The Snake Oil Delusion” or SNOD for short. The fallacy in SNOD lies in assigning a plausible value for P(E| H) when in fact no such value is justified. Smith has no idea whether he would or would not have recovered without Organocalm, or indeed any treatment at all. To assume as he did that</p><p>P(E| H) was zero, or was indeed any less than P(E|H), is to argue in a circle. The only such assumption warranted by the evidence, or rather the lack of evidence, is that P(E| H) is no less than P(E|H) ,in which case </p><p>P(E | H) O(H|E) = ҙ O(H) =1ҙ O(H) P(E | H)</p><p>In other words the experiment (successful treatment with Organocalm) should not have increased his confidence in Organocalm by one iota above his declared prior belief that </p><p> the odds on it were evens. The Snake Oil Delusion [SNOD] is so pernicious precisely because its’ circularity is implicit or concealed. Nothing wrong is explicitly stated: the fallacy slips in by the back door. The patient is cured, and since no other medicine was given, the medicine must be efficacious. The policy was implemented, the economy crashed, the policy must have been responsible. Children in the village have mysteriously died; no other cause but witchcraft can be imagined; a witch must be found and burned. The fallacy of SNOD lies not so much in lack of rationality as in lack of imagination, an inability to imagine one or more hypotheses alternative to H that will explain the evidence as well. The blind man recovers his sight, no one can explain why: a miracle must have occurred. SNOD underlies conjuring as well as a host of other more pretentious, more profitable and potentially far more harmful forms of mumbo-jumbo and black magic ranging from much of medicine to financial counselling [think of medicinal leeches]. In its most transparent form SNOD was known to the ancients as the fallacy of “Post hoc ergo propter hoc” which translates as “ After that, therefore because of that” 18</p><p> and as such was relatively easy to spot. When it comes in a more elaborate, or apparently more respectable disguise, it can fool all but the most alert of us – including scientists. For instance scientists at The Stanford Research Institute were comprehensively gulled by Yuri Geller the ‘spoon bending’ magician. Because they couldn’t explain rationally what he had shown them, they were willing to entertain an irrational explanation. At the heart of SNOD lies the often very real need to come to a conclusion about an hypothesis, for instance in order to reach a decision, and the frequent impossibility of assigning a rational value to P(E| H ), i.e. the probability that the evidence could be explained by some hypothesis H, other than the one under discussion. When decisions cannot be deferred, more or less arbitrary choices must be made which leave any </p><p> number of doors for SNOD to creep in. For instance it may not have been possible, or </p><p> in the commercial interest, to carry out the control experiments needed to truly establish the efficacy of Organocalm. Or we may have to trust ‘experts’ whose expertise is more self-delusional or self-serving (all too frequently the case). Then again we cannot afford to evaluate the potentially infinite number of alternatives to H [ e.g. juries] . And if we want a decision at all we may feel we cannot afford to set P(E| H) equal to P(E|H), when perhaps we ought. Choices are necessary, but choices are difficult and potentially treacherous with SNOD waiting in the wings. And SNOD thrives on wish fulfilment. We want to get rich quick so we want to believe in the self-</p><p> proclaimed financial wizard who urges us to buy those shares. We want to get well again so we want to believe in the wisdom and integrity of Dr X in the white coat. Regrettably there are no universal nostrums for avoiding SNOD. However, thinking out important decisions in terms of Bayes’ Theorem will always alert one to the component assumptions i.e. O(H), P(E|H) and P(E| H) which must, in one way or another, go to make it up. For instance had Smith known about Bayes it might have prompted him to look into the spontaneous recovery rate P(E| H), and so he might have</p><p> saved himself a great deal of money, and worry. One of the manifold children of SNOD is or was Psycho-analysis, though that is </p><p> disputed by proponents, especially those who still make a living out of it. Devised by Sigmund Freud and his disciples in and around Vienna in the early twentieth century it spread around the globe and into every interstice of western culture. Its’ plausible mumbo-jumbo included ‘inferiority-complex’, ‘ego’, ‘id’, and ‘Oedipus-complex’ which became household words and generated handsome incomes for any number of 19</p><p>“therapists” trained in the fashionable Viennese techniques. Its’ reputation rested on claimed or supposed ‘cures’. Its disgrace and downfall, still not complete, only began in the 1960s with long overdue control experiments which revealed that untreated patients recovered just as often as those unfortunates who had paid for five or more years ‘treatment’ on the psycho-analyst’s couch. To what extent Psycho-analysis started as deliberate fraud, and to what extent as self-delusion, is still a matter of controversy, but there can be little doubt that it caused a great deal of suffering and abuse. For instance little girls who claimed to have been interfered with by male relatives were labelled by Freud and his followers as suffering from. hysterical wish- fulfilment, and left to further abuse, even punishment, for the same. As Peter Medawar, then President of the Royal Society said : “Considered in its entirety, psycho-analysis won’t do. It is an end product, like a dinosaur or a zeppelin; no other theory can ever be erected on its ruins, which will ever remain one of the saddest and strangest landmarks in the history of twentieth century thought.” [in ‘Hope of Progress’,1972] From our point of view, as thinkers about thinking, psychoanalysts, and the long but undeserved hold they gained on western culture, should serve as a continual warning. We are today, in an increasingly complex world, surrounded by, and often forced to rely on, an army of ‘experts’: IT consultants, psycho-therapists, doctors of various kinds and specialities, priests, cosmologists, lawyers, plumbers, financial advisers, astrologers, dieticians, pharmacists, teachers, professors, councillors on everything from child-rearing to post-traumatic-stress disorder, beauty consultants, accountants, stock-market gurus, estate-agents, mechanics, psycho-surgeons…..the list goes on and on. The only thing we know about them for sure is that in one way or another they’re all after our money or our votes, or our souls. How many of them rely on SNOD to get it? </p><p>THE CASE OF THE MISSING GALAXIES is drawn from my own experience as an astronomical scientist. Many years ago I was lucky enough to stumble over an exciting idea relating to galaxies – titanic whirlpools of stars which seem to be the building bricks of the cosmos at large .We live at the edge of one such, called The Milky Way. What I noticed was that practically all the known galaxies had exactly the same brightness-per- unit-area or ‘surface brightness’ as we say. More to the point the precise value of their surface brightness was exactly such as to draw the maximum attention to themselves when observed against the night sky as seen from the Earth. 20</p><p>Either this was the most felicitous and unlikely coincidence, or else there were vastly more galaxies out there crouching below the brightness of our night sky. The analogy here is to looking out through the window from a lighted room at night. You see nothing of the exterior – not the houses, mountains or trees –unless they too are lighted up like your room. And, in astronomical terms, our sky on Earth is very bright indeed. Even the naked eye can detect as many as 50,000 light particles a second coming from the darkest area of sky the size of a full Moon. So I was on to a really exciting idea, one implying that most of the galaxies, most of the universe, was lurking out there, hidden beneath the sky, waiting to be discovered. I straightway decided to devote my working life to the search. My colleagues and I searched all over the sky using telescopes, satellites and radio antennae. Finding new galaxies turned out to be much harder than I had naively supposed because you have got to stare very hard indeed out of a lighted window to see anything more than other lights. Nevertheless, after twenty years hard work we, and other colleagues abroad, had amassed enough evidence to suggest, if not prove, that there are indeed significant numbers of hidden galaxies out there. Then came the dramatic blow. At a large international conference called specifically to discuss hidden galaxies, a young Dutch astronomer, let us call him Dr X, announced that there weren’t any. He and a team of eminent colleagues had pointed the world’s largest radio telescope at a narrow strip of sky for several months. Independently of their light output they had found fifty radio objects in the strip, and confirmed their existence as radio emitters with another powerful radio telescope. They had at last detected the hydrogen signals that I had predicted would be the signatures of otherwise invisible galaxies. However, they had then gone on to look at all of their radio sources with an optical telescope – and in every single case found it to be an easily visible galaxy. Not one of the 50 was invisible. Not one! Here was the decisive, the crucial test advocated by philosophers such as Karl Popper. And my beloved hypothesis had failed it miserably. I blustered and fumed but there seemed no honest way of denying a test so ingeniously designed, so thoroughly checked and so numerically decisive. Nobody was fooled by my blustering, least of all myself. As a then disciple of Popper it was inconsistent to deny that a theory, no matter how beautiful and beloved, can be murdered by a single ugly fact. And yet my instinct rebelled. What of all the other positive evidence, admittedly indirect, amassed over the years? Was all that now to be set at naught? Here was a 21 typical messy conflict of evidence: one clearly negative piece set against an agglomeration of separately weaker positive pieces. I retired to the library to read and to think. Here I belatedly came upon the Reverend Thomas Bayes, the gentleman who died in 1761 and is buried at Bunhill Fields in central London. I read that his Theorem had become the rallying cry for a group of dissident statisticians and scientists who used it as a method of weighing disputed evidence. In the hidden galaxy case Dr X and I could both do Bayesian calculations regarding the same piece of evidence E i.e. his failure to find any hidden galaxies, and arrive at quite different conclusions about the probability, in consequence, that my hypothesis H [that ‘Hidden galaxies do exist’] was wrong. We could arrive at these inconsistent conclusions quite honestly because we might start from very different Priors, and also because we might entertain rather different opinions [ i.e. P(E|H)’s] about the reliability of his observations, or his interpretation of them . We could display our parallel Bayesian calculations as in table (8: 2):</p><p>TABLE (8.2) ARE THERE ANY HIDDEN GALAXIES? Hypothesis H : “There are Hidden galaxies” Evidence E : “X’s failure to find any in his search.”</p><p>Person Prior O(H) P(E|H) P(E | H) O (H | E) 1Believer(me) 0.91 0.53 0.86 6 2Sceptic (X) 0.22 0.14 0.955 0.02</p><p>See Note 7 0.2 0.5 0.8 0.1</p><p>See Note 8 10 0.1 0.95 »1</p><p>1 My Prior is high because I am persuaded by previous evidence. 2 X’s Prior is low because he is unaware of, or unpersuaded by, my previous evidence. 3 I feel there is a 50% chance that X’s evidence is flawed. 4 X feels there is only a 10% chance his evidence is flawed. 5 X is 95% sure that if H is wrong he would get his observed result. 6 I am not so sure that if H is wrong he would get his observed result. 7 What happens if I accept X’s Prior but retain my data assessment P(E|H)? 8 What happens if I keep my Prior but accept X’s data assessment? 22</p><p>Because I had strong faith in my hypothesis beforehand [i.e. a high Prior O(H) = 10], based on twenty years of earlier work, and a degree of scepticism about the reliability of X’s observations [i.e. my P(E|H) =0.5] my posterior odds remained high [O(E|H) = 6] in agreement with my instinctual reaction at the time – despite the damning appearance of X’s result. X on the other hand came fairly sceptically to my hypothesis [OX(H) =0.2], as he was entitled to do, felt his results were reliable [see his </p><p>PX(E|H) and PX(E| H) ] and concluded that there was now only a 2 per cent chance [i.e. the odds were 50 to 1 against] of my hypothesis being correct. In other words we could agree to differ, without coming to blows. I was admitting [see my P(E|H) and P(E| H) ] that his observations were possibly, though not conclusively, right, while he was conceding [his OX(H|E) ] that there was still a chance, albeit a small one, that my hidden galaxies might yet exist. We could also explore how our respective conclusions might change if we were to accept different parts of our opponents’ argument. For instance in rows 3 and 4 of the Table I have calculated what would happen to my own conclusion P(H|E) were I first to accept X’s Prior, but not his data assessment, or second his data but not his Prior. Comparison of rows 2,3 and 4 makes it clear that in this case it is the contrast between our Priors which has made the real difference between our Posteriors. Investigating thus how sensitively ones conclusions depend on ones Priors, and their different component assumptions is called ‘Sensitivity Analysis’ and should form part of any Bayesian program. As a result of X’s work both his odds on H, and mine, had changed in the same direction i.e. we were both more sceptical. His odds had gone from 5 to 1 against to 50 to 1, while mine had weakened from 10 to 1 on down to 6 to 1 on. And that surely is as it should be. Our difference arose from a different Prior. I had included all the earlier evidence in mine. He had either ignored or discounted it. The debate could fruitfully move on from the assessment of X’s observations to the reassessment of our Priors. This case highlights both the strengths and weaknesses of the Bayesian approach to evidence – which amount to the same thing in the end: the weight it attaches to Priors as well as to evidence. Those philosophers who would like to see in science an exemplar of objective truth hate to admit that the interpretation of a scientific experiment or observation is to still to some extent a plaything of prior opinion. Plumbers on the other hand, who are used to making mistakes themselves, and to 23 uncovering them in other peoples’ handiwork, are relieved to find there is still room to weigh the evidence. Harlow Shapley the famous American astronomer once remarked “Nobody believes a theory but the man who proposed it. Everybody believes an observation – except the man who made it.” The setting of Priors is of course open to serious abuse. One who wants either to affirm or deny some hypothesis in the face of all the evidence is empowered to do so, as long as he declares a strong enough Prior. But that is his business and his lookout. He will have to take the consequences. For instance by declaring a prior of 10 to 1 I could have fooled myself, and so wasted several precious years of my scientific life digging for fool’s gold. Conversely, by adopting a weaker prior, I might have been persuaded to abandon a really good idea, and decades of previous hard work, by evidence of a doubtful nature. The art of science, it would appear, no less than the art of life itself, is getting one’s Priors right. [Incidentally, in the case of the missing galaxies, X’s data proved to be right, but his telescope was nowhere near as sensitive as he thought, and so his interpretation of it, which was what we both needed, was mostly wrong. My beautiful hypothesis survived to fight another day. But, of course, it could still very well be wrong.]</p><p>THE CASE OF THE FLYING SAUCER. One might have hoped that the accumulation of more and more evidence would, in the end , lead to a convergence of opinions, no matter how far apart the initial Priors, or if you like the initial prejudices, of those in debate. Sometimes it does and sometimes it does not. It turns out that convergence will only occur if both parties are willing to grant the evidence the same degree of belief. If they cannot do that then the same evidence put before them may actually lead to an even greater divergence of opinion. Let us look at an example. Weird lights are seen in the sky by dozens of inhabitants of the lonely island of Jura. Two ‘expert’ journalists , a believer B in flying saucers, and a sceptic S, are sent to investigate. B has a prior belief OB(H) =10 to 1 on the hypothesis H that ‘Flying </p><p>Saucers exist.” while for the sceptic S OS(H) = 0.1 only. After listening to the transparently sincere evidence (E) of the islanders each must reconsider his position. Table (8: 3) summarises the outcome from a Bayesian perspective: </p><p>TABLE (8.3) WERE THERE ANY FLYING SAUCERS ON JURA? 24</p><p>Hypothesis H : “Yes there were.” Evidence E : “Islanders saw lights in the sky”.</p><p>Prior P(E|H) P(E | H) Posterior 1 Believer 10 1 0.2 50 2 Sceptic (A) 0.1 1 0.2 0.5 3 Sceptic (B) 0.1 0.9 0.9 0.1</p><p>Before going to Jura the ratio of their prior beliefs OB(H) / OS(H) was 10 / 0.1 =100 to 1. Afterwards that same ratio depends on their evaluation of the evidence. Were the sceptic to take the same enthusiastic view of the evidence as the believer [see (A) in Table (8.3] – that is to say adopt the same P(E|H) = 1 and P(E| H) = 0.2 – then his posterior would increase to 0.5 and the ratio of their posteriors would still be 100 to 1. In fact the sceptic [see (B) in table] took the view that the reports could as easily be </p><p> explained by some other phenomenon as by flying saucers – so that his P(E|H) = 0.9 and P(E| H) =0.9. In that case his posterior cannot rise above his prior and he remains </p><p> as sceptical as ever, i.e. OS(H|E) =0.1. But because The Believer’s posterior has risen to</p><p>PB(H|E) = 50 the ratio OB(H|E) / OS(H|E) is 50/0.1= 500, or even higher than before. </p><p>They have diverged. So the naïve supposition that ‘Looking at the evidence’ must necessarily lead to agreement, or even to some convergence of views, is by no means guaranteed. It all depends on one’s views of the credibility and relevance of that evidence. Whereas the recounting of every new miracle may strengthen a believer in his belief, the disbeliever can legitimately remain unmoved. It is all, according to Bayes, a question of P(E|H) as compared to P(E| H ). If in your view an alternative explanation H can as well or better explain the evidence as H, you will not be moved.</p><p>OBJECTIONS TO BAYESIAN INFERENCE. Science has been so successful that many commentators have refused to believe that its main mode of thought could be anything as crude as Bayesian Inference. And scientists themselves haven’t helped much. Most of them refuse to discuss The Scientific method, and those that do often make manifestly incorrect assertions. For instance Peter Medawar one President of the Royal Society who we quoted on p… went on to advocate Karl Popper’s “Falsification” program – which had already been discredited by William Quine, while 25 another, Sir Isaac Newton no less, the greatest brain in scientific history, once asserted that “In this experimental philosophy(‘science’ to us) propositions are deduced from phenomena, and made general by induction.” It is now generally accepted, following the Scottish philosopher David Hume, that Induction, that is to say drawing general inferences from specific instances, is impossible; no matter how many white swans you encounter you can never be certain that ‘All swans are white’. For that reason the rock hard certainty that some have sought in science – usually not scientists themselves – has had to be abandoned in favour of provisional statements based on Probability. Some philosophers though won’t even accept probabilistic arguments. Probabilistic estimates, they say, have to be based on past experience, and there’s no guarantee that the future will be like the past. Thus they would criticise Stella for using evidence from past Gold Cup winners to update her odds on Wavehunter winning a future Grand National. In a strictly logical sense they must be right; we have no guarantees as to the future. But in a practical sense aren’t they talking nonsense? We could not live rationally, and would be paralysed, if we couldn’t assume some degree of continuity between past, present and future, even if that continuity isn’t perfect. Why would we trust our lives to an aeroplane if we believed that any one of the hundreds of laws of nature and engineering which went into its design, were capricious? Even those who believe that probabilistic inference is the way to go have fierce and continuing wars about the way to do it “properly”. To begin with there are arguments, fuelled by history, about the very definition of Probability itself (Chapter 6). Mathematicians point out that it was they who first developed Probability Theory back in the sixteenth century in connection with games of chance. Their Laws of Probability are based on ‘relative frequencies’; thus the Probability of drawing an Ace (1 in 13) is, by definition, equal to the relative number of times you commonly draw an Ace, compared to the other cards[ the ‘relative frequency’] in a well shuffled pack. Unfortunately such a definition of Probability is far too limited to be of use to scientists, and indeed to anyone else who isn’t playing cards. An astronomer who is trying to measure the mass of the Sun can’t shuffle anything. What he and his colleagues mean by some proposition such as “The Sun probably weighs about 4 billion billion billion tonnes’ is “the degree of assent that a rational person can give to such a statement”. It is an altogether vaguer but much more useful definition of Probability than the mathematical kind. It may have nothing to do with frequencies but is a numerical measure of our confidence in such and such a statement. In that case, 26 retort the mathematicians, you cannot use all our laws and theorems concerning Probability, which were derived in a far more limited and far less ambiguous context. They’ve got a point. What has ‘degree of assent’ got to do with games of chance? Nothing. And yet in 1812 ‘the French Newton’ Pierre Simon de Laplace, who first laid down the rules for thinking of scientific data in probabilistic terms, sneakily, or perhaps unconsciously, assumed that the two very different kinds of Probability obey the same fundamental rules; that Bayes’ Theorem, for instance, applies to both. He said “It is remarkable that a science which began with games of chance should have become the most important object of human knowledge”. For a century peace reigned as scientists analysed their data according to Laplace’s ten rules., i.e using Bayesian Inference. Then a rebellion broke out when the newly founded profession of ‘Statistics’, led by its high priest R.A.Fisher, pointed to the appalling abyss, the illegitimate assumption, underlying Laplace’s thinking. Bayesian Inference withered away in the cauterising fire of their criticism, to be replaced by the whole new paradigm of ‘Statistics”. So all-destructive was this revolution that undergraduates of my day, the 1960s, were protected from all knowledge of the dastardly Bayes and brought up to pray instead at the alter of ‘Statistics’. It was very hard, it was very mathematical, but it was also very Holy. There is no room here to describe all the bloody battles in this war between Statisticians and Bayesians, a holy war which still rages today. But it is important to understand the main campaigns. Firstly Laplace’s confusion of everyday Probability with its rarified mathematical counterpart has been cleared up, at least in the following sense. It turns out the The Laws of Probability can be derived from much broader considerations than relative frequencies in games of chance, and thus they have far wider application, including to inference in general, and especially to science. So Laplace’s cavalier assumption turned out to be right. For instance in chapter five we derived the Sum and Product Rules, and Bayes’ Theorem, from di Finetti’s idea of the ‘Coherent Bet’. And if you don’t like such a gambling analogy there are other starting points as well. The point is you can’t prove something from nothing; you have to start from some fundamental, unproveable axioms or desiderata. And that leaves room for argument. Thus Harold Jeffreys and his school claim to have proved the vital Rules from little more than common sense and self consistency. But the proof is not easy, and some stubborn Statisticians decline to be persuaded. 27</p><p>The second causus belli is the use of Priors. One school claims you should never use them because they are ‘unscientific’ . Mathematicians in particular don’t like quantifying ‘hunches’ and mixing them up in their otherwise rigorous equations. A second school permits the use of Priors – provided they have been rigorously calculated from ‘objective considerations’, preferably by ‘a robot’. A third school is much more relaxed: you can use any Prior you like – as long as you realize the price you will have to pay to pay when your Prior turns out to be wrong – or inaccurate. That price must be paid in your wasted time, even a lifetime, barking up the wrong tree. How could one do without Priors – as some fanatical statisticians claim that we should, and they do? Given some evidence E, they say, generate a number of hypotheses H1,H2,H3...... then calculate for each Hn ‘The Likelyhood’ i.e P(E| Hn ), then favour that hypothesis with the ‘Maximum Likelyhood’ .To my mind there are two glaring faults with this prescription. First it coyly refuses to give us the odds-on our Hypothesis, given the evidence i.e. to give us O(H|E) – which is what we actually want. And second not all imaginable hypotheses are equally plausible – even if they give the same Likelyhood P(E|H). A Moon made of basalt and a Moon made of green cheese might reflect some specific radiations in the same way (E) -- but I know which hypothesis my money would be on. The call of the second school for ‘Objective Priors’ seems to me altogether more reasonable. After all if each of us have idiosyncratic Priors how can we collectively agree about anything? We return to this vital question in Chapter… Unfortunately it is often very hard, if not impossible, to set objective values on some Priors – my Prior on green cheese for instance – but that doesn’t mean to say they are worthless. In our Cancer scare case a very crude idea (Prior) on the rarity of the disease sufficed to rescue the thinking . Perhaps, as a scientist, it is fine to have a non-objective Prior to set one off on a new voyage of research. Thus Columbus came upon tropical beans washed up on an Irish beach. On their own the beans convinced nobody else of a great continent on the opposite shore. But the beans got Columbus going on a voyage which did return evidence of the incontrovertible kind.</p><p>Einstein asserted that “ The whole of science is nothing more than a refinement of everyday thinking.” In this chapter I have tried to demonstrate that learning from experience, a large part of science, may be inference modelled on Bayes’ Theorem. Given that most scientists have never heard of Bayes, any more than the rest of us, this 28 demonstration cannot be conclusive. However Bayesian Inference is based on Laws of Probability which have a very wide range of application; it enables one to draw the kind of conclusions one needs to live by – even in the face of disputed or imperfect evidence; and it does appear to track everyday thinking. So it would be perverse of scientists not to use it sometimes – unless they have discovered a method far better. Anyway here are some quotes from eminent scientists to suggest that we might be on the right track: “The most important questions of life are, for the most part, really only problems of probability.” Pierre Simon de Laplace, 1812. “The actual science of logic is conversant at present only with things either certain, impossible, or entirely doubtful, none of which (fortunately) we have to reason on. Therefore the true logic of this world is the calculus of Probabilities, which take account of the magnitude of the probability which is, or ought to be, in a reasonable man’s mind.” James Clerk Maxwell, the great Scottish physicist who predicted radio waves and was the fore-runner of Relativity. “ One must have the mentality of a gambler or fisherman. As for me I am only interested in big fish.” Luc Montaignier, discoverer of the AIDS virus.</p><p>If it is so crude why does Bayesian Inference appear to work so well? First, I suspect, because it is accretive; second because it is multiplicative. Each accretion of </p><p>Evidence E1,E2,E3.....multiplies one’s previous prior by a Bayes Factor which can be large (or small), even if it is imprecisely known. Multiplying several such Factors together – the result of acquiring further evidences, can quickly drive a weak Prior towards a decisive conclusion (In either sense) – which is what any animal needs to preserve itself in a fast-moving and unforgiving world. It suggests that more evidence is the way to go, rather than a tighter measure on what we already know.</p><p>REFINEMENTS. What about the ‘refinement’ or refinements of everyday thinking referred to by Einstein? Can we identify any of those? Yes we can.</p><p>So far we have dealt with discrete hypotheses H1,H2,H3...... each one distinct from the others. We can extend our ideas to a virtual infinity of hypotheses where each is only minutely different from its neighbours. This can be very useful when one wants to pick a best value for some quantity X – for instance the mass of the Sun, or the value of Inflation in China. As before we feed in any Evidences, independent measurements for 29 instance, and Priors -- as for example notions of their relative reliability, then we crank the Bayesian machinery, but using elementary calculus in place of arithmetic. Out will come a range of values for X, each with a Probability as to its soundness. One might for instance conclude that the mass of the Sun, with 90 percent Probability, lies in the </p><p>27 range between 3.5 and 4.5 ҙ10 tonnes. A second refinement is the use of STATISTICS. Scientific papers these days are usually peppered with Statistics of various kinds, often ones with frightening-sounding names. How could one disagree with a paper which has withstood the fire of a “Kolmogorov-Smirnov Test”, or been subjected to a “Markov-Chain-Monte-Carlo- Analysis”? Indeed the Statistics is often so complicated that most of the scientific authors on the paper can’t understand it. They have to invite a professional statistician onto their team to do their statistics for them. What is the purpose of all this Statistics? Mostly it is to demonstrate the “Significance” of the paper’s conclusions where ‘Significance’ is used in a strictly limited sense. It is a measure of how unlikely it is that the claimed results can be merely the outcome of pure chance. For instance of 27 patients given a new drug 19 recover, whereas in a control group of 24 given a placebo, only 10 do. If the authors are to claim that the drug is effective they must demonstrate that the difference in recovery rate between the two groups is unlikely to be the mere result of chance. Like Bayesian Inference Statistics is based on those Laws of Probability which we have discussed. It forces us to quantify the significance of our claims and conclusions. This is a very powerful discipline which pseudo-sciences will generally fail. Had the early claims of phrenology and psycho-analysis been tested for significance they would never have gained the footholds which they did. Is there anything more to Statistics than Bayesian Inference? That is a good question, guaranteed to raise the blood pressures of cognoscenti, and to which we will return in Chapter 8 because it could have momentous consequences for us as both thinkers and citizens If, like me, you lost a lot of savings in the recent banking crisis, you might be interested to know that a main reason you did so is wrapped up in this very question. But trying to be uncontroversial for now, the main factor which distinguishes Statistics from plain Inference is ‘Simplification’. Statistics grew up in a climate with loads of numbers, but no computers to handle them. So statisticians were the men and women who invented ingenious tricks to deal with them; for instance replacing whole populations of data with averages, and the dispersions about those 30</p><p> averages; for instance replacing messy looking distributions of numbers with smooth distributions such as ‘The Normal’. That enabled them to draw conclusions, and to make estimates of confidence in them, when doing the job ‘properly’ i.e. taking into account all of the available information, was wholly impractical. In a nutshell then Statistics is Inference plus Simplification. But don’t get the idea that Statistics itself is simple. On the contrary. Each cohort of data may require a new set of simplifications, and thus a new sub-branch of Statistics. That is why many, and perhaps most, scientists today can’t afford to do their own Statistics any more. It has become too proliferous, too hair-splitting, too smothered in jargon. And that is very bad news for the rest of us. How can we any longer hope to understand what is going on at the scientific coal face? How can we be reasonably sure that the claims of Global Warming have to be attended to? However, and this is the vital point we return to in Chapt…, Statistics itself may now have become obsolete! Why bother with its multifarious and risky Simplifications when computers can bash out the inferences and the estimates of confidence we need, without them? If true that would be very good news! Another useful refinement is to rewrite Bayes’ Theorem in an alternative way. Recall that the Odds-on some hypothesis H is, by definition the Probability ratio (see p ): O(H) ә P(H)/P(H ). That being so we can replace the Odds terms O(H|E) and O(H) in Bayes’ Theorem (1) with their equivalent Probability ratios as above, in which case we easily find that</p><p>P(E | H) ҙ P(H) P(H | E) = (1A) P(E | H) ҙ P(H) + P(E | H ) ҙ P(H ) a formula which is extremely clumsy by comparison with (1) and actually contains no more information. Nevertheless it is the formula which appears in most textbooks so </p><p> it’s useful if you need to consult them. And its not as bad as it looks because all the stuff bottom left is just a repeat of the stuff on top. Because it was derived from the Odds form, with nothing new added, we obviously can’t learn anything from the new formula that we couldn’t learn from the old. However it sometimes helps to see matters from a slightly different angle. For instance take the Snake Oil Delusion (SNOD) discussed earlier. It arises when we cannot imagine an hypothesis H to explain evidence E alternative to the one H under consideration. Inadvertently we then suppose that P(E| H ) is zero. But look at (1A) for the implication. The top and bottom of the formula become identical and hence the Left</p><p>31</p><p> hand side, the Probability of H given E, becomes exactly 1, making H certain. Because germs were unimaginable phenomena back in 1400 we went out and burned witches.</p><p>Bayes’ Theorem demonstrates the provisionality not only of science, but of inference in general. As such it is, or ought to be a foundation and support for all liberal i.e. tolerant thinking! Unless one can state categorically that no other hypothesis but the one in mind is capable of explaining the evidence, then because of the extra term P(E| H) ҙ P( H) in the denominator – i.e. the bottom of the theorem – then P(H|E) can never amount to absolute certainty, that is to say to one. And how can anybody honest deny that there might be an alternative to H floating out there in the limitless ocean of </p><p> presently unimagined ideas, ideas which one day may fit as well, or better? The search for absolute certainty sounds all very well – until you realise the implication: that there is not, and never can be, another way of looking at the matter. If that is so, and it seems that it is, then certainty is perhaps something we were better to do without. The tendency for many philosophers and mathematicians to project onto science their own cravings for absolute certainty is, or so it seems to me, misplaced. For instance, in his autobiography, Bertrand Russel admitted: “ I wanted certainty in the kind of way that people wanted religious faith.” . Science is rooted in the dirty earth of reality. When we dig scientific knowledge from the earth we cannot expect shining gems, only rough stones, barely distinguishable at first from the trash in which they lie. Only after skilled cutting and polishing in human hands do they gleam with the brilliance we lust after. This same craving for certainty may also explain the attraction of ‘Falsification’ to philosophers of the Popper school. If an hypothesis H is entirely unable to explain some</p><p> evidence E n (i.e. if P( E n |H) =0) then in accord with Bayes (1) P(H| E n ) =0 also; i.e. that hypothesis is certainly ruled out. This should be obvious but is made explicit in </p><p> formula (1A) where the upstairs (unlike the down) has nothing to compensate if P( E n |</p><p>H) is set to zero. In practice though it is very hard to be certain that a P(E|H) is truly zero, and not just small. E itself may not be as unequivocal as we would like. For </p><p> instance in the Galaxy case above the E was “Dr. X found no invisible galaxies in his deep radio search.” What that really means though is that “X found a visible galaxy to correspond with every one of his 50 radio detections.” But were all his correspondences actually correct – or were some of them coincidental? Galaxies cluster close to one another on the sky so that an invisible radio source could easily be misidentified with a visible galaxy nearby. It happens all the time. Then again some hypotheses can be, 32 legitimately or not, protected from absolute falsification, by erecting ‘Auxiliary Hypotheses’ around them. Thus Newton’s Law of Gravitation, which never seems to work on the scale of galaxies or larger (E), is generally protected by assuming the auxiliary hypothesis that “Dark Matter must be present”. It seems that even Falsification cannot avoid messy Probabilistic Inference.</p><p>Bayesian Inference may well be the backbone of everyday thinking, but is it, with the refinements added above, the way that scientists actually think? Fig(8.1 ) shows a LUM( London Underground Map) of the process. I have shown it to many scientists and almost all of them deny that they think like that. Their typical comments include: ‘Never heard of Bayesian Inference’; ‘We use Statistics a lot’; ‘What’s Bayes’ Theorem?’; ‘I don’t use Priors’; ‘What do you mean by ‘Coherent bet’? Science is logic, it isn’t gambling’; ‘I mostly use mathematics and deduction’. So then I show them the LUM B in Fig 8.2 to which they invariably respond ‘Yes that’s much more like it’. On that basis it would appear that scientists, in disagreement with Einstein, have indeed invented a whole new mode of thinking. Actually though, if my scientists can be made to concede the following 4 arguments the two LUMs can be brought into exact coincidence: (A) The kind of Probability we scientists need cannot be the narrow kind based on relative frequencies. We need a much broader version, and much broader foundations for our Laws of Probability based on something like (but not necessarily) The Coherent Bet. (B) Unless we concede that all hypotheses are equally likely we cannot do without Priors, if only to restrict the hypotheses we will in practice entertain. (C) Bayes’ Theorem, however it is disguised , is the only systematic way we presently know of to weigh the odds-on or -against an hypothesis in the light of new evidence. Since it derives from only the universal Sum and Product Laws of Probability, it is hard to see how one could avoid using it, formally or otherwise. (D) Statistics is nothing more than Bayesian Inference plus some risky Priors involving Simplification. There appear to be so many varieties of Statistics only because there are so many alternative Simplifications one can invoke. Anyway, thanks to computers, we don’t need Statistics any more. We can do our inferences properly, using all the data. Of the scientific colleagues who will debate these matters (most won’t) the great majority eventually concede points A to C. They’re much more stubborn about D, 33 about Statistics – which is odd considering it is the subject they, by admission, least understand. The younger ones in particular can become quite emotional, as if one is questioning their capacity to think at all. Because of its controversial nature we return to Statistics in Chapter… So all we claim at this stage is that scientists may learn from experience in the same messy way that the rest of us seem to do – using Bayesian Inference. It is a rather crude, possibly a sub-human method of progress, using as it does Priors that may sometimes be little more than informal, and imprecisely calculable ‘hunches’. But it does our induction, our generalisation for us , albeit in a probabilistic and only provisional way. As Isaac Newton put it “…although the arguing from Experiments and Observations be no Demonstrations of general Conclusions, it is the best way of arguing which the Nature of Things admits of.”</p><p>If you haven’t heard of Bayes’ Theorem before, you should certainly think hard about using it hereafter. It can be a compass in the mists of thought, a steadying guide through perilous reefs of contradictory evidence. If nothing else it will force you to own up to your prior prejudices and admit the reliability you attach to any evidence you might use. We can all benefit from knowing more precisely why we believe what we do, and why we disagree with our opponents.</p><p>If there is no escaping from Priors, even in science, perhaps especially in science, then what should they be? What has emerged so far is that prejudices that are too strong are very hard to overthrow. If you want to learn from experience you must be careful to keep ‘ a reasonably open mind’. Four hundred years ago Francis Bacon wrote “He that shall start in certainties shall end in doubt; but he that shall start in doubt shall come to certainty.” But what is ‘a reasonably open mind’? That will be the business of the next chapter. 34</p><p>35</p>
Details
-
File Typepdf
-
Upload Time-
-
Content LanguagesEnglish
-
Upload UserAnonymous/Not logged-in
-
File Pages35 Page
-
File Size-