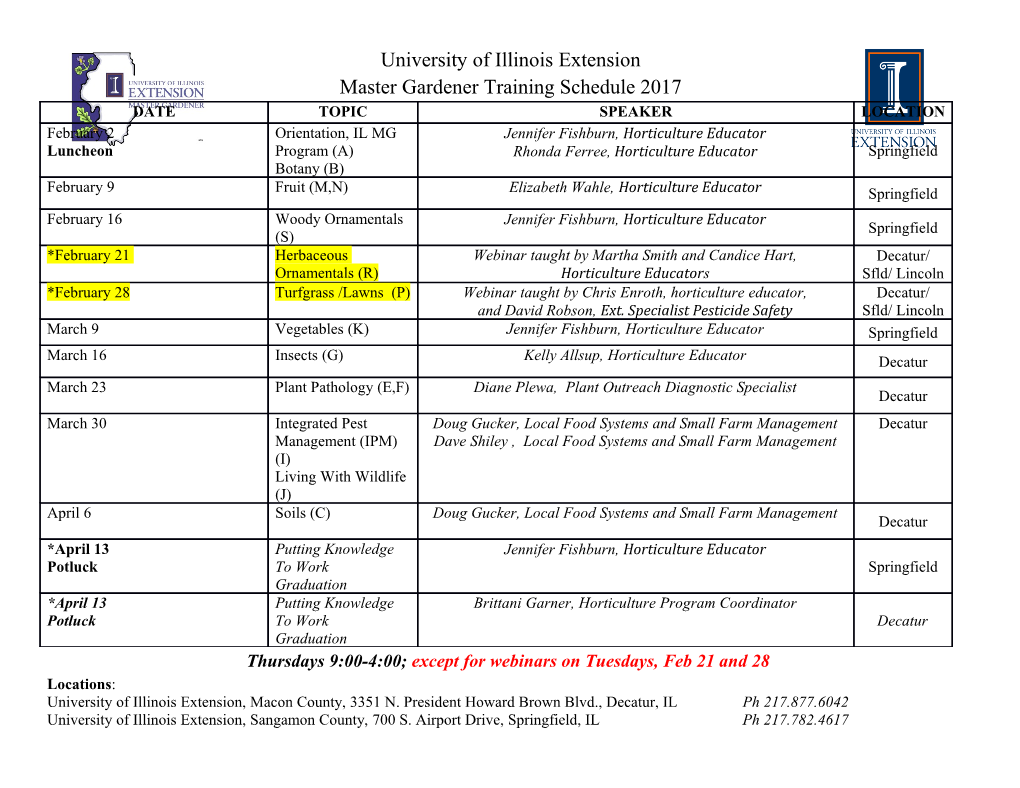
<p> Appendix</p><p>I. Statement regarding the performance of the Good Judgment Project in the ACE tournament. </p><p>June 12, 2015</p><p>Five research teams competed to win a forecasting tournament that was part of the Aggregative Contingent Estimation (ACE) program sponsored by IARPA. The goal was to come up with the most innovative ways possible to elicit, aggregate and communicate geopolitical forecasts. The first two seasons occurred between September 2011 and April 2013. Each forecasting team was asked to submit daily predictions on all active question to the official judges by email. Accuracy was assessed using mean daily Brier score. The Good Judgment Project, one of the five teams in the ACE tournament, achieved the lowest Brier scores among all teams in both seasons and won the tournaments by far exceeding the accuracy goals set by IARPA. In 2013, IARPA decided to fund a single team, the Good Judgment Project, and set benchmarks for success against another control group. Two tournaments were run between June, 2013 and June, 2015. Once again, the Good Judgment Project successfully increased accuracy relative to the benchmark comparison group by over 30%. </p><p>Steven Rieber</p><p>Program Manager Aggregative Contingent Estimation Program Intelligence Advanced Research Projects Activity (IARPA) II. Example Forecasts. </p><p>Question: Will opposition forces in Syria seize control of the Syrian city of Aleppo by 30 April 2013? Outcome: Event did not occur. </p><p>Team polls forecasts are displayed in Figure A1. The grey line follows the predictions for the Unweighted Linear Opinion Pooling (ULinOP) from the start of the question, December 6 to April 30, when the event was judged as “not occurring”. This method does not perform any aggregation adjustments. The red line incorporates decay, so that the aggregate forecast incorporates the latest 20% of individual forecast updates. As can be seen in the figure, this increases sensitivity to new information. Purple line denotes aggregated forecasts with weighting. Individuals with a track record of high accuracy and high updating frequency receive higher weights. The blue line illustrates the forecasts after applying extremizing transformation. The black line shows the full algorithm, incorporating decay, weighting and transformation. Figure A1: Forecast streams for Team Prediction Polls with various statistical aggregation techniques. </p><p>Figure A2 shows the forecast streams for Independent Prediction Polls following the same aggregation techniques as those used in Team Prediction Polls, as described above. Figure A3 describes the forecast stream for Prediction Market, the simple average of the end-of-day prices for the two parallel prediction markets. Figure A2: Forecast streams for Independent Prediction Polls. </p><p>Figure A3: Forecast stream for Prediction Markets.</p><p>2. Optimization Results</p><p>All parameters were optimized sequentially using elastic net regularization in the following sequence: temporal decay, prior performance weighting, belief-updating weighting and recalibration. This order was determined before Study 1 data were available. The rationale for applying decay first was that old forecasts should be discounted, even if they belong to the most accurate forecaster. The rationale for applying recalibration last was that the optimal recalibration parameter depends on the informational value of the underlying forecast. Decay and weighting were utilized to make the aggregate forecasts as informative as possible before extremizing them. Temporal decay was implemented using a moving window that retained 20% of the forecasters in both team and independent prediction polls. The optimal exponent value () for prior accuracy weights was 4, the maximum allowed, for both independent and team prediction polls. At this value, the highest weighted forecaster received approximately 16 times more weight than the median forecaster. For frequency of belief-updating weights, the optimal exponent () was 0.4 for independent polls and 1.8 for team polls, denoting that the most frequent updater would receive approximately 1.3 and 3.5 times the weight of the median forecaster, respectively. Finally, the estimated recalibration parameter, a, shown in Equation 3, was 2 for independent prediction polls and 1.5 for team prediction polls. The parameter values were consistent with those obtained by Baron et al. (2014). We experimented with other weighting schemes. Weights based on self-rated expertise did not produce consistent improvements in accuracy. Weights derived from question viewing time produced small improvements in Brier score (less than 1%), when applied on top of frequency and accuracy weights. Mellers et al. (2015) discuss psychometric measures that predict individual performance. These measures could be used as aggregation weights, and may be especially useful in a setting where accuracy information is difficult to collect. Overall, we believe it is possible to improve accuracy by several percentage points by including additional parameters, but the addition is unlikely to overcome the cost of increased complexity. </p><p>III. Prediction Markets: A Closer Look</p><p>1. Bid-ask spread analysis. </p><p>We calculated bid-ask spreads for the two parallel prediction markets in Study 1 and the single market in Study 2. The calculation proceeded in three steps. First, we found the highest bid and lowest ask price on the order book each day at midnight PST. If there were no bids on the book, the highest bid value was imputed as zero cents. If there were no asks on the book, lowest ask values were imputed to 100 cent. Second, we calculated the mean bid-ask spread across days within a question. Finally, the distribution of average bid-ask spreads across questions in the box- plots below. </p><p>Market A tended to yield lower bid-ask spreads (M=31, SD=17) than market B (M=38, SD=18). Figure A4 displays these distributions. Each market had approximately 250 traders. We examined the relationship between bid-ask spread and forecasting accuracy. For this analysis, we used data after September 8, the date when the four markets were merged and become two. First, we calculated bid-ask spread difference between markets A and B, as well as the Brier score difference between A and B. Next, we calculated the Pearson correlation between the differences in bid-ask spreads and Brier scores. The correlation coefficient was in the expected direction, as markets with higher bid-ask spreads on any given day tended to produce prices associated with higher Brier scores (r=0.04, t(10,060)=4.04, p<.001). However, the correlation was weak. The results were replicated using a hierarchical linear model, which accounted for clustering of observations within questions: differences in performance between the two markets were largely unpredictable from differences in bid-ask spreads in these markets. Aggregation methods that placed higher weights on markets/questions with lower bid-asked spreads did not produce reliable accuracy improvements. Figure A4: Bid ask spreads for two parallel prediction markets in Study 1. Bid-ask spreads for the market discussed in Study 2 (M=21, SD=11), displayed in Figure A5, were on average lower than those in Study 1 markets. This was consistent with the idea that larger markets tend to produce lower bid-ask spreads: the number of participants (approximately 540) assigned to the prediction market in Study 2 was greater than the number of participants in each of the two markets in Study 1.</p><p>Figure A5: Bid ask spreads for Single prediction Market in Study 2.</p><p>2. Serial Correlation of Market Returns</p><p>Next, we turn to the serial correlations of returns. A positive serial correlation makes it possible to derive above-average returns by using a momentum strategy, i.e. betting that past trends will persist in the near future. Conversely, a negative serial correlation of returns would yield excess returns to a counter-momentum strategy, i.e. betting that recent price changes will reverse in the near future. Either pattern implies market inefficiency, as the predictability of future prices from past price movements implies that the current market prices do not incorporate all publicly available information. </p><p>Serial correlations of returns were estimated using the acf function of the stats package in R. Figure A6 displays the distribution of serial correlation coefficients in markets A and B. The mean AR1 coefficients in both markets were negative in both markets (-0.09 and -0.11, respectively). Negative coefficients were estimated for approximately 75% of questions. These estimates imply that betting to reverse the previous day’s price movements would have resulted in generally higher returns than betting to amplify recent price changes. However, the magnitude of the coefficients and the non-zero bid-ask spreads would have likely limited excess returns associated with a counter-momentum strategy. Higher order serial correlation coefficients approached zero. </p><p>Figure A6. Serial correlation of returns in Study 1 for Market A (left panel) and market B (right panel). </p><p>In Study 2, the single market produced positive first-order serial correlations. The mean AR1 coefficient for the market was 0.08. The distribution of coefficients is displayed in Figure A6. The forecast time series for 70% of questions produced positive serial correlations, unlike the markets in Study 1. A positive serial correlation implies that participants could earn higher returns by betting that prices will continue to move in the direction of last day-to-day change. However, the serial correlation would likely be too weak to produce consistently positive returns across questions. Notably, the signs of serial correlation coefficients were not consistent across the two seasons, implying that that the patterns of returns were largely unpredictable from one season to the next. Figure A6. Serial correlation of returns for single market in Study 2. 3. Stationarity of Price Movements </p><p>We examined the stationarity of time-series predictions. Stationarity is the property of time series that follows a stable trend. Stationary time-series tend to revert to a trend after a temporary movement away from the trend, whereas non-stationary time-series do not follow this pattern. Stationarity in market prices can be seen as a sign of market inefficiency, because the behavior of stationary time-series is predictable from past movements. We performed a series of Augmented Dickey-Fuller tests to test for stationary of market prices over time, separately for each question. The null hypothesis for the test is non-stationarity in price movements, so p-values below 0.05 denote stationarity or inefficiency of price movements. </p><p>We could not compute test statistics for two questions, because their duration was too short to generate reliable patterns price/forecast movements. Prediction market B produced constant prices for two additional questions so no statistics were computed for these instances. In year 2, the non-stationarity null hypotheses was rejected at α=0.05 for 22 out of 112 questions in market A and 22 out of 110 questions in market B. For comparison, time series of team and independent polls yielded Augmented Dickey-Fuller test p-values below p=0.05 for 28/112 questions and for 29/112 questions, respectively. </p><p>In summary, both markets and polls produced non-stationary series more frequently than could be expected by chance. However, violations of non-stationary were infrequent. In prediction markets, we found significant evidence for stationarity in approximately 20% of the questions. These patterns may generate excess returns for traders who systematically bet on trend-reversal after large price movements, but the low frequency of stationary times series suggests that excess profits would be limited. IV. Sample Size and Relative Performance</p><p>1. Sensitivity to Forecaster Sample Size </p><p>Does the relative performance of markets and polls vary with sample size? This question is important because tournament designers may have access to fewer than 600 participants, the approximate sample size in each condition for Study 1. The operation of four parallel prediction markets in the first three months of Study 1 allows us to examine whether markets with smaller numbers of forecasters are prone to higher errors than prediction polls. Market participants were randomly assigned to four prediction markets, while individual and team poll participants were sampled based on random forecaster and team identification numbers. </p><p>For this comparison, forecast data were subset to the periods in which the four markets operated separately, June 19 to September 8, 2012. These dates were early in time course of most questions, which made forecasts more difficult and less accurate across the board. Approximately 125 participants were included in each group, with equal numbers across conditions. </p><p>As seen in Table A1, the average price across the four markets registered a mean Brier score of 0.316, while aggregations of all data from team and independent polls clocked in at 0.300 and 0.315, respectively. The mean Brier scores of each separate market varied in a narrow range (from 0.335 to 0.371), as did the performance of team subsets (from 0.328 to 0.359). The last row of Table 1 shows the loss in accuracy (increase in Brier score), resulting from the use smaller subsets rather than a combination of all available data. For example, the average Brier score across the market was 0.350, while the average price of the four markets would resulted in a 0.316 Brier score. The decrease in accuracy was similar across prediction markets, individual and team polls. </p><p>In summary, prediction markets of approximately 125 individuals performed on par with statistically aggregated polls of similar sample size. Thus, market size, as measured by the number of forecasters assigned to each market, did not appear to be a serious constraint on market performance in the current study. </p><p>Table A1. Mean Brier scores for separate markets and random subsets of teams and independent individuals in prediction polls, based on 56 questions open between June and September 2012. Data Subset Individual Team Prediction Prediction Poll Prediction Market Poll A. Combined 0.315 0.300 0.316 Group 1 0.372 0.347 0.335 Group 2 0.355 0.359 0.335 Group 3 0.316 0.328 0.357 Group 4 0.351 0.328 0.371 B. Mean of Groups 1-4 0.349 0.341 0.350 Loss: B – A 0.034 0.041 0.034</p><p>2. Activity </p><p>In Study 1, we found that participants in markets attempted fewer questions than those assigned to prediction polls. We performed down-sampling analyses to estimate the extent to which the relatively strong performance of polls could be traced to higher activity levels. Because independent forecasters have no way of communicating with one another, it is reasonable to down-sample at the forecaster level, without concerns about information spillovers. Team members can help each other to the right answer, so down-sampling simulations were performed at the team level rather than the individual level. Mean Brier scores for randomly selected independent prediction polls using random half- samples ranged from 0.203 to 0.208, compared to 0.195 for the full sample. Random half samples of teams produced average scores ranging from 0.195 to 0.201, relative to 0.186 for the full sample. In summary, reducing sample sizes in prediction polls by half produced only a small accuracy decrement (5%). Thus, the propensity of prediction polls to attract larger crowds to each question—out of a similarly sized poll—partially accounted for team polls’ accuracy advantage over markets, which tended to attract smaller crowds with more lopsided patterns of activity. </p>
Details
-
File Typepdf
-
Upload Time-
-
Content LanguagesEnglish
-
Upload UserAnonymous/Not logged-in
-
File Pages9 Page
-
File Size-