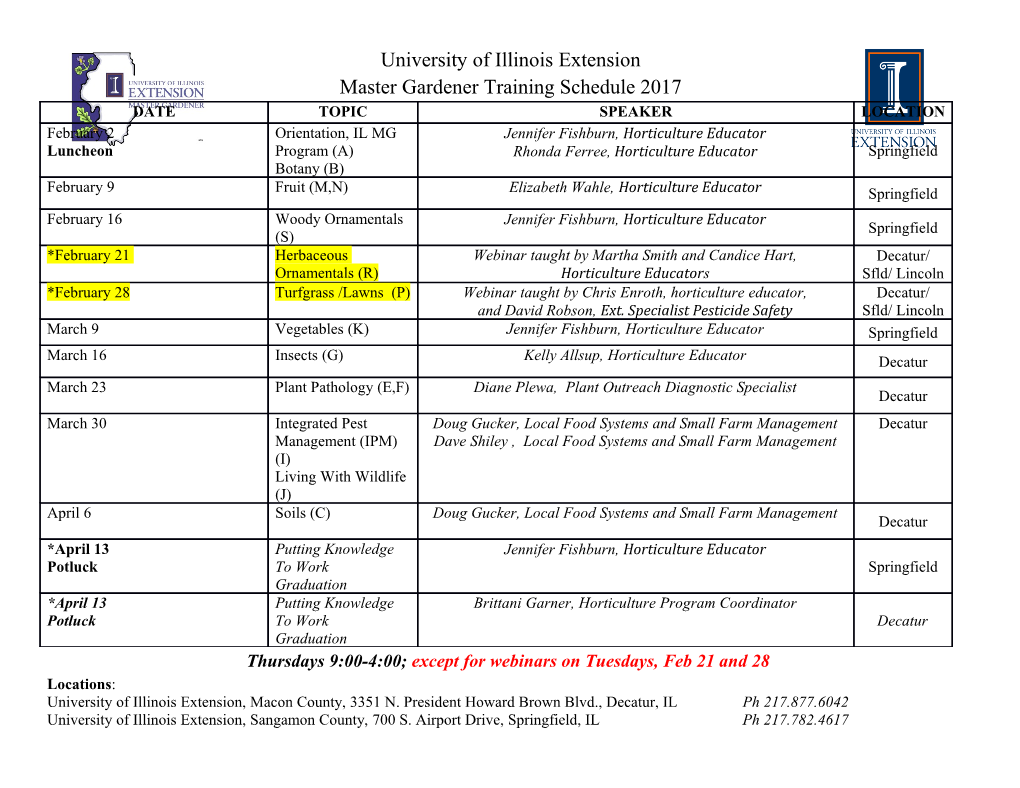
<p>COURSE NOTES - STAT 110 – Fall 2011</p><p>1 – Basic Concepts, Terminology, and Types of Studies</p><p>1.1 - Basic Definitions and the Statistical Process Statistics -</p><p>General Approach to Statistical Process</p><p>1 The Cycle of Statistical Investigation</p><p>R e a l p r o b l e m s D e s i g n m e t h o d o f C u r i o s i t y d a t a c o l l e c t i o n P o s e t h e q u e s t i o n </p><p>A n s w e r t o o r i g i n a l C o l l e c t d a t a q u e s t i o n </p><p>I n t e r p r e t t h e r e s u l t s - S u m m a r y a n d W h a t d o t h e y m e a n ? a n a l y s i s o f d a t a </p><p>More Definitions/Terms Population –</p><p>Parameter –</p><p>Sample –</p><p>Statistic – </p><p>Descriptive Statistics –</p><p>Inferential Statistics –</p><p>2 1.2 - Types of Studies (see Powerpoint on website)</p><p>Two Main Types of Studies Observational – researcher collects info on attributes or measurements of interest, but does not influence results.</p><p>Experimental – researcher deliberately influences events and investigates the effects of the intervention, e.g. clinical trials and laboratory experiments.</p><p>EXPERIMENTAL STUDIES</p><p>In this section we will examine some basic experimental designs and design principles, namely: I. Completely Randomized Designs</p><p>II. Blocking and Block Designs</p><p>III. People as Experimental Units (e.g. clinical trials)</p><p>I. Completely Randomized Design (CRD) The treatments are allocated entirely by chance to the experimental units.</p><p>Example 1: Tomato Plants Which of two varieties of tomatoes (A & B) yield a greater quantity of market quality fruit? Factors that may affect yield: soil fertility; exposure to wind/sun; soil pH levels; soil water content etc. Divide the field into plots and randomly allocate the tomato varieties (treatments) to each plot (unit). Situation 1: 8 plots – 4 get variety A</p><p>Situation 2: What if the field had a slope to it?</p><p>UPHILL 8 plots – 4 get variety A</p><p>3 II. Blocking Situation 2 on the previous page illustrates the use of what is referred in experimental design as blocking. In blocking we group (or block) experimental units by some known factor and then randomize within each block in an attempt to balance out the unknown factors.</p><p>Example 2: Comparing Three Pain Relievers for Headache Sufferers How could we design an experiment? How could blocking be used to increase precision of our experiment? </p><p>Horse Leg Diagram:</p><p>Example 3: Race Horse Leg Wraps (Data Files: Horseboots and Conboots) • 17 “boots” tested, each boot is tested n = 5 times. Why?</p><p>• Because of the time constraints all boots were not tested on the same day. • 8 tested 1st day, 5 tested 2nd day, 4 tested 3rd day. • Leg was placed in freezer and thawed before the 2nd and 3rd days of testing. Questions: What problems do you foresee with this experimental design?</p><p>4 Example 3 – Race Horse Leg Wraps (cont’d) What actually happened? Below is a plot of the force readings when no wrap was used on the leg during the three days of testing.</p><p>What is the implication of the results shown above?</p><p>Final Results of Horse Leg Wrap Study</p><p>Q: What should have been done?</p><p>5 III. Using People as Experimental Units (Medical Studies)</p><p>Example 4: Cholesterol Drug Study</p><p>Suppose we wish to determine whether a drug will help lower the cholesterol level of patients who take it. </p><p>Q: How should we design our study? </p><p>6 Important Concepts for Experiments with Human Subjects</p><p>• control group: – Receive no treatment or an existing treatment</p><p>• blinding: – Subjects don’t know which treatment they receive</p><p>• double blind: – Subjects and administers / diagnosticians are blinded</p><p>• placebo: – Inert dummy treatment</p><p>• placebo effect: – A common response in humans when they believe they have been treated. – Approximately 35% of people respond positively to dummy treatments - the placebo effect</p><p>OBSERVATIONAL STUDIES There are two major types of observational studies: prospective studies and retrospective studies. I. Prospective Studies Choose samples now, measure variables and follow up in the future, e.g. choose a group of smokers and non-smokers now and observe their health in the future.</p><p>II. Retrospective Studies Looks back at the past, e.g. a case-control study Separate samples for cases and controls (non-cases). Why? </p><p>Look back into the past and compare histories. For example, we could choose two groups: lung cancer patients and non-lung cancer patients. Compare their smoking histories.</p><p>7 Important Note: 1. Observational studies should use some form of random sampling to obtain representative samples. 2. Observational studies cannot reliably establish causation. Only well-executed controlled experiments can be used to establish causation.</p><p>Controlling for various factors</p><p>A prospective study carried out over 11 years on a group of smokers and non-smokers showed that there were 7 lung cancer deaths per 100 000 in the non-smoker sample but 166 lung cancer deaths per 100 000 in the smoker sample. This still does not show smoking causes lung cancer because it could be that smokers smoke because of stress and that this stress causes lung cancer. To control for this factor we might divide our samples into different stress categories. We then compare smokers and non-smokers who are in the same stress category. This is called ______for a confounding factor, in this case stress level.</p><p>Example 1 - “Home births give babies a good chance”, NZ Herald, 1990</p><p> An Australian report was stated to have said that babies are twice as likely to die during or soon after a hospital delivery than those from a home birth.</p><p> The report was based upon simple random samples of home births and hospital births. Comments:</p><p>8 Example 2 – Lead Exposure and Tooth Decay (USA Today, Children exposed to lead are more likely to suffer tooth decay, and vitamin C might help lower blood lead levels, say two recent studies. </p><p>In the first of the reports in the Journal of the American Medical Association, researchers estimate that lead exposure could account for tooth decay in 2.7 million children. “Other people may debate that, but that’s our position”, says head researcher Mark Moss of the Univ. of Rochester (NY) School of Medicine and Dentistry. </p><p>Prior studies showed that lead exposure can depress a child’s IQ. “There are a lot worse things that lead can do to you than hurt your teeth”, Moss says. He notes, however, that one of the key questions in dentistry is why low-income people experience more tooth decay than higher-income people. “This study suggests lead might be one of the reasons,” he says.</p><p>The study involved 24,901 children ages 2 and older. It showed that the greater the child’s exposure to lead, the more decayed or missing teeth. “The risk of getting tooth decay increased as the amount of lead went up,” Moss says.</p><p>1. What is the population of interest?</p><p>2. What is the sample?</p><p>3. What are some possible explanations for the results Moss and the other researchers observed?</p><p>In a second study, Joel Simon and his colleagues at the University of California at San Francisco studied 19,578 people who had no history of excess lead exposure. They found that the higher a person’s intake of vitamin C, the lower his or her blood lead level.</p><p>4. Does this prove that increasing one’s vitamin C intake will lower blood levels? Explain.</p><p>9 Example 3 - WSU Student Survey - In order to generate data for use in one of my introductory statistics courses a few years back, I had the class develop a short survey and administer this survey to ten WSU students of their choosing. In the end, survey responses were recorded for a total of 348 WSU students (n=348). </p><p>1. What is the population of interest?</p><p>2. What is the sample?</p><p>3. What are some potential problems with this survey methodology? </p><p>1.3 - Surveys</p><p>Survey</p><p>Errors</p><p>Sampling/ Chance/ Random Nonsampling Errors Errors</p><p>. errors caused by the act of taking a sample . can be much larger than sampling errors</p><p>. have the potential to be bigger in smaller . are always present</p><p> samples than in larger ones . can be virtually impossible to correct for after </p><p>. possible to determine how large they can be the completion of survey</p><p>. unavoidable (price of sampling) . virtually impossible to determine how badly they will affect the result</p><p>. must try to minimize in design of survey (use a pilot survey etc.)</p><p>10 MORE ON ERRORS IN SURVEYS The sampling process introduces two types of error: • Sampling / Chance / Random Errors • Nonsampling Errors</p><p>Sources of Nonsampling Errors</p><p>Selection bias Population sampled is not exactly the population of interest. e.g.</p><p>Nonresponse bias People who have been targeted to be surveyed do not respond.</p><p>Self-selection bias People decide themselves whether to be surveyed or not.</p><p>Question effects Subtle variations in wording can have an effect on responses.</p><p>Interviewer effects Different interviewers asking the same question can obtain different results.</p><p>Behavioural considerations People tend to answer questions in a way they consider to be socially desirable.</p><p>Transferring findings Taking the data from one population and transferring the results to another.</p><p>Survey-format effects</p><p>11 2 - Data/Variable Types</p><p>There are two main variable types:</p><p>Example - WSU Student Survey The following items comprised the survey. Classify each item (variable) as being either numeric, ordinal, or categorical. (Data File: WSU Student Survey) Survey Item/Variable Variable Type Gender Age Did student have a declared major? Major, if declared College major program is in, e.g. College of Liberal Arts (CLA) Class (Fr, So, Jr, or Sr) Hours spent studying per day GPA Is student involved in extra-curricular activity, e.g. intramural sports or biology club? Is student living on- or off-campus? Hours of sleep per night Number of credits student is currently taking Does student have a “significant other”? Does student skip at least on class per week? If they do skip, what is the most common reason for skipping? Does student drink alcohol? If they do drink, what would be a typical number of “drinks” they would have per night that they drink? Does student smoke cigarettes? If student is a smoker, how many cigarettes do they smoke per day? Should President Clinton be impeached for his sexual relations with </p><p>12 Monica Lewinsky? Is student of legal drinking age (21 yrs. old)? How much did student spend on textbooks this semester? Does student think the WSU Laptop Program is a good idea?</p><p>POTENTIAL QUESTIONS OF INTEREST FROM THE STAT 110 SURVEY</p><p>13 3 – Descriptive Statistics</p><p>3.1 - Describing a Single Categorical/Qualitative Variable Frequency Distribution Table</p><p>Entering Data JMP</p><p>From the JMP Starter window click the New Data Table button. </p><p>A new spreadsheet will appear with only one column (labeled Column 1). For this example we need two columns in our spreadsheet to enter this table, one for the gas price </p><p>14 opinion of the respondents and one for the number of respondents in each opinion category. To add columns to a spreadsheet simply double click to the right of the first column. Each time you double click to the right of the rightmost column another column will be added. Here we only need one additional column so we will double click once to the right of the first column. You can change the name at the top of the column by clicking at the top of the column so that field becomes highlighted for editing. We will name the first column “Opinion” and the second “# of Respondents”. We may want to force the values in the # of Respondents column to be interpreted as frequencies. To do this we will use the role assignment pop-up menu to change this column’s role to that of a frequency count. The Preselect Role menu is accessed by right-clicking at the top of a column. From this pull-out menu select Freq to change this column’s role to frequency/count.</p><p>The numbers in the # of Respondents column will now be interpreted as frequencies associated with the gas price opinions.</p><p>Use the mouse, the return key, and/or the arrow keys to move about the spreadsheet and enter the data. Rows will automatically be added each time you hit enter when you are entering data in the last row so you do not necessarily have designate the number of rows in advance. </p><p>When you are finished your spreadsheet should look like:</p><p>Variables in the dataset are listed here. The data type for each variable in JMP is denoted as follows: = continuous = ordinal = nominal </p><p>When we select, exclude, hide, or label certain cases in JMP graphs or spreadsheets information about the number of each of those types is presented here. </p><p>15 In general, we are usually entering raw data rather than pre-tabulated data as we have here. A typical dataset will have one row for each subject in the study. The columns will then contain the different variables we are measuring on each subject in the study. If these data entered in this format our spreadsheet would have n = 1,006 rows (one for each respondent) along with the measured opinion. Other variables would probably be measured as well in a study such this, like for example the respondents gender, age, marital status, occupation, ethnicity, etc. This would allow us to explore difference in opinion across levels of these other variables. </p><p>To the left is a portion of a spreadsheet with each row corresponding to the respondent’s opinion on gas prices. Notice that this spreadsheet has n = 1,006 rows.</p><p>Additional variables could certainly be added in the form of additional columns.</p><p>Mosaic Plots and Frequency Tables To obtain a mosaic plot and frequency distribution table for these data select Distribution from the Analyze pull down menu and place Gas Price Opinion a in the right-hand box by double-clicking on it. Below is the resulting bar graph and mosaic plot for these data.</p><p>A relative frequency or probability axis has been added by selecting Prob Axis from the Histogram Options pull-out menu. The Histogram Options menu is accessed from the </p><p>16 pull down menu located next to the variable name, in this case Gas Price Opinion (see below). The Show Percents option has been selected from the Histogram Options menu as well. Also a mosaic plot (rectangular pie chart) has been added by selecting the Mosaic Plot option from the Gas Price Opinion pull-down menu. Below is the resulting frequency table for these data:</p><p>The Count column contains the frequencies and the Prob column contains the relative frequency or proportion of respondents in each opinion category. Selecting the Confidence Interval (.95) option gives the following:</p><p>Example 2: WSU College of Enrollment (Data File: WSU Student Survey) College By Gender</p><p>Comments:</p><p>17 3.2 - Exploring the Relationship Between Two Categorical Variables</p><p>To examine the relationship between two categorical variables we have can use comparative bar graphs, contingency tables (or cross-tabulations), 2-D mosaic plots (or stacked bar graph), and conditional probabilities/proportions/percentages.</p><p>Comparative Bar Graphs are separate bar graphs for the variable of interest constructed for each level of second factor. Typically the levels of this second factor denote distinct populations we wish to compare in terms of the variable of interest. To do this in JMP, first select Analyze > Distribution then put the variable of interest in the Y, Columns box and the “conditioning” or “grouping” variable in the By box. </p><p>Example: Gender and the Laptop Program Suppose that we wish to examine the relationship between a student’s opinion of the WSU Laptop Program and their gender.</p><p>Opinions of Females Opinions of Males</p><p>Why can’t we directly compare the 142 females who do not think the laptop program is a good idea to the 78 males who feel the same way? </p><p>Contingency Tables A contingency table or cross-tabulation is a table whose rows and columns are defined by the levels of two categorical/ordinal variables. The counts found in the “cells” of the table represent the number of observations found in each possible combination of levels of the row and column variables. </p><p>Example: Gender and the Laptop Program </p><p>Opinion of Laptop Program Gender No Undecide Ye Row Totals d s Female 142 7 53 202 Male 78 1 67 146 Column Totals 220 8 120 348</p><p>1. What percentage of females surveyed have a favorable opinion of the laptop program?</p><p>2. What percentage of those students who had a favorable opinion of the laptop program were female? </p><p>18 3. Why are the answers to (1) and (2) above different? Why is (2) of little interest?</p><p>4. What percentage of males surveyed had an unfavorable opinion of laptop program?</p><p>Mosaic Plot of Laptop Program Opinion and Gender</p><p>To display percentages in the segments of a mosaic plot right- click on a cell in the mosaic plot and select Show Percents from the Cell Labeling pull-out menu.</p><p>In JMP select Analyze > Fit Y by X and put one of the categorical variables in the Y, Response box and the other variable in the X, Factor box. </p><p>Important Point: Which variable should be Y and which should take the role of X? It depends. Usually X denotes a group or population that wish to compare the variable Y across. </p><p>In the previous example we wished to compare opinions about the WSU Laptop Program across gender, thus the student’s laptop opinion is the Y variable and the student’s gender is the X (see below).</p><p>19 Example 2: Education Level and Opinion on the Invasion of Iraq (Data File: Education-Iraq) Is there a relationship between highest level of education and opinion regarding the invasion of Iraq? If so, what is the nature of the relationship?</p><p>Invasion of Iraq? EDUCATION LEVEL a - For b - c - N/A Row Totals Against 1 - HS Dipl 865 528 73 1466 2 - Some Coll 672 489 61 1222 3 - Coll Grad 458 348 42 848 4 - Post Grad 373 522 38 933 Columns Totals 2368 1887 214 4469</p><p>1. What percent of those whose highest education was a high school diploma are for the invasion of Iraq? </p><p>2. What percent of those who are in favor of the invasion of Iraq had a high school diploma as their highest education level? Why is this percentage deceiving and therefore should not be considered?</p><p>3. Summarize the relationship between education level attained and opinion on the invasion of Iraq.</p><p>20 3.3 – Graphically Summarizing a Single Numeric Variable In this section we examine two graphical displays that are used to summarize numeric variables: the histogram and stem-and-leaf plot.</p><p>Below is a histogram of the semester book costs of a sample of WSU students.</p><p>How is a histogram constructed?</p><p>Book Costs ($)</p><p>Three key features of a histogram</p><p>21 Features to Look for in Histograms and Stem-and-Leaf Displays</p><p>22 (a) Unimodal (b) Bimodal (c) Trimodal</p><p>(d) Symmetric (e) Positively skewed (f) Negatively skewed (long upper tail) (long lower tail)</p><p>(g) Symmetric (h) Bimodal with gap (i) Exponential shape</p><p> spike</p><p>(j) Spike in pattern</p><p> outlier outlier</p><p>(k) Outliers (l) Truncation plus outlier</p><p>Figure 2.3.10 Features to look for in histograms and stem-and-leaf plots.</p><p>From Chance Encounters by C.J. Wild and G.A.F. Seber, © John Wiley & Sons, 2000.</p><p>Example 1: Book Costs Per Semester (Data File: WSU Student Survey)</p><p>23 Book Costs ($)</p><p>1. What would be the typical amount a WSU student would spend on books?</p><p>2. Most students would have textbook costs in what range?</p><p>3. How much “variation” in book costs do we see?</p><p>Stem-and-Leaf Plot of Book Costs</p><p>4. What advantage if any does the stem-and-leaf display of these data provide when compared to the histogram? </p><p>Example 2 - Hours Spent Studying Per Day (Data File: WSU Student Survey)</p><p>24 Hours Spent Studying (hrs/day)</p><p>1. Discuss what is learned about studying time of WSU students from the histogram.</p><p>2. What interesting feature(s) does this particular histogram have?</p><p>Example 3: GPA and Gender of WSU Students (Data File: WSU Student Survey) As long as we are careful to use uniform scaling we can use histograms to compare two or more populations in terms of their values on some numeric variable of interest.</p><p>GPA’s of Female WSU Students Use the histograms to the left to compare and contrast the GPA’s of female and male WSU students.</p><p>GPA’s of Male WSU Students</p><p>Example 4: Hours Studying Per Day and Gender (Data File: WSU Student Survey)</p><p>25 What are the differences between males and Females females in terms of the hours they spend studying per day?</p><p>Males</p><p>Histograms and Stem-and-Leaf Displays in JMP To obtain a histogram and outlier boxplot for numeric variable(s) select Distribution from the Analyze pull down menu and place the variable(s) that you wish to examine in the right-hand box. To obtain a stem-and-leaf plot select the option from the pull-down menu next to the variable name. The Horizontal Layout, Prob Axis, Normal Curve & Smooth Curve options have can been used in constructing the histogram for book costs shown below. The locations of these options are illustrated in the graphics below:</p><p>The density or distribution curves are added by selecting the options shown below.</p><p>The normal curve and smooth curve density estimate are added by selecting these options from the Fit Distribution pull- out menu,</p><p>3.4 – Summary Statistics for Numeric Data </p><p>26 I) Measures of Central Tendency, Typical, or “Average” Value</p><p>II) Measures of Spread/Variability</p><p>III) Measures of Location/Relative Standing</p><p>I) Measures of Central Tendency (mean, median, and mode)</p><p>Notation for Observations or Data th x1 , x2 ,..., xn where xi i observed value of the variable x and n = sample size</p><p>Mean Sample Mean (x) Population Mean() Why use the median rather than n N the mean to measure typical? xi xi x i1 i1 n N</p><p>Example:</p><p>Median Middle value when observations are ranked from smallest to largest.</p><p>Sample Median (M) Population Median (Med)</p><p>Example:</p><p>Mode</p><p>27 Most frequently observed value or for data with no or few repeated values we can think of the mode as being the midpoint of the modal class in a histogram.</p><p>Examples:</p><p>Example 1 - Hours Spent Studying Per Day (Data File: WSU Student Survey)</p><p>What are the mean, median, and mode for the time spent studying per day by the WSU students sampled?</p><p>28 Example 2 – Hours Spent Studying Per Day and Gender (Data File: WSU Student Survey)</p><p>Hours Spent Studying (WSU Females)</p><p>Hours Spent Studying (WSU Males)</p><p>Use the measures of central tendency to compare and contrast the hours spent studying for male and female students.</p><p>29 II) Measures of Variability Range Variance and Standard Deviation Interquartile Range (IQR) – range of the middle 50% of the data Coefficient of Variation (CV)</p><p>Range Range = Maximum Value – Minimum Value </p><p>Example:</p><p>Variance and Standard Deviation Sample Variance ( s 2 ) Population Variance ( 2 ) n N 2 2 xi x xi s 2 i1 2 i1 n 1 N</p><p>Sample Standard Deviation ( s ) Population Standard Deviation ( ) s s 2 2</p><p>Example:</p><p>30 Interpreting the Standard Deviation: Chebyshev’s Theorem & Empirical Rule Chebyshev’s Theorem and the Empirical Rule are used to determine the percentage of observations that lie within in certain intervals centered about the mean. The intervals have the form:</p><p> mean k standard deviation where k is a positive integer. </p><p>Chebyshev’s applies for any non-normal distribution while the empirical rule applies only for distributions which are approximately normal. The table below gives the percentages associated with the intervals defined by taking the mean plus or minus 1, 2, and 3 standard deviations.</p><p>Interval Chebyshev’s Thm Empirical Rule</p><p>Example 1: Gestational Age of Infants at the Time of Birth</p><p>Gestational Age (days)</p><p>31 Example 1 – Gestational Age (cont’d):</p><p>Application to Decision Making: In 1949, a divorce case was heard where the husband filed for divorce on the grounds of his wife’s adultery. The only evidence he had was the fact she gave birth to a child 50 weeks (350 days) after he had gone abroad on military service. The judge hearing the case agreed that though it was improbable a woman would carry a baby 350 days, it was scientifically possible and the child could have been his. Thus the judge did not grant him a divorce. What do these rules say about the likelihood of a gestation age > 350?</p><p>Example 2: GPA of WSU Students (Data File: WSU Student Survey)</p><p>1. What is the range of the GPA’s?</p><p>2. What are the variance and standard deviation?</p><p>3. Approximately 68% of the students will have GPAs in what range?</p><p>Approximately 95% of the students will have GPAs in what range?</p><p>Approximately 99.73% of the students will have GPAs in what range?</p><p>32 Example 3: Cost of Textbooks for WSU Students (Data File: WSU Student Survey)</p><p>1. Approximately what percent of WSU students spend between $178.09 and $357.19?</p><p>2. Approximately what percent of WSU students spend between $88.52 and $446.76?</p><p>Coefficient of Variation (CV) - Another measure of spread</p><p>Measures spread relative to the size of the mean. s CV 100% x</p><p>Ex: Which has more variation: GPAs of WSU students or their textbook costs? Explain. GPA Book Costs</p><p>33 III) Numerical Measures of Relative Standing</p><p> Percentiles/Quantiles and Quartiles Interquartile Range (IQR) – another measures of variability/spread Outlier boxplot – another plot used to summarize a numeric variable z-scores</p><p>Percentiles/Quantiles</p><p>Quartiles</p><p>Interquartile Range (IQR) (another measure of variability)</p><p>Outlier Boxplots Ex: Number of cigarettes per day for WSU students who smoke</p><p>Outliers: Any observations lying more </p><p> than 1.5 IQR belowQ1 or more than</p><p>1.5 IQR above Q3 are classified as outliers.</p><p>34 Example 2 – GPA’s of WSU Students (Data File: WSU Student Survey)</p><p>Using these data we estimate that… 1. 25 percent of WSU students have GPAs below ______2. 75 percent of WSU students have GPAs below ______3. ______percent of WSU students have GPAs below 3.706. 4. 90% of WSU students have GPAs above ______</p><p>Standardized Variables ( z-scores )</p><p> xi x xi The z-score for an observation xi is z (sample) or z (population). i s i It tells us…</p><p>Example 1: Lengths of Fish (Data File: Catfish) Which is more extreme a catfish 24 inches in length or a smallmouth buffalo 13.5 inches in length?</p><p>35 Example 2: GPAs of WSU Students</p><p>1. What is the z-score associated with a GPA = 3.75? ______</p><p>2. What is the z-score associated with a GPA = 2.25? ______</p><p>3. What is the z-score associated with a GPA = 3.90? ______</p><p>Standardizing Variables in JMP (z-scores)</p><p>To obtain z-scores associated with each observation select Standardized from the Save menu which is located within the main pull-down menu for the variable, Books$ in this example. </p><p>A new column labeled Std Books$ will appear in the original spreadsheet containing the z-scores. You could examine the distribution of the z-scores themselves by using the Distribution command. Any observations with z-scores exceeding 3 in absolute value could be classified as potential outliers.</p><p>The histogram below is for book costs standardized using z-scores.</p><p>Histogram of standardized book costs.</p><p>Notice that several of the outliers have z-scores beyond the -3 to 3 range.</p><p>36 Comparative Displays In many situations we are interested in comparing the values of numeric variable across two or more groups/populations. For example, we may be interested in comparing…</p><p>A useful graphical tool for making such comparisons is to use comparative boxplots and histograms. As an example, consider plot below which compares the number of cigarettes smoked per day by male and female WSU students who smoke.</p><p>Example 1 - Cigarettes/Day vs. Gender Comments:</p><p>To obtain this type of display in JMP select Fit Y by X from the Analyze menu and put the grouping variable or population identifier in the X, Factor box and the numeric variable of interest (i.e. response) in the Y, Response box, shown below for cigarettes/day vs. gender.</p><p>37 The resulting display will show the numeric variable plotted versus the levels of the grouping variable. To add boxplots and other enhancements to this plot use the Display Options menu located within the main One-way Analysis of … pull-down menu. </p><p>Quantiles – gives quantile summary statistics and adds boxplots to the display.</p><p>Means and Std Dev – gives means and standard deviations by location and adds mean/SD lines to the plot.</p><p>The options and their effects are summarized below... Box Plots - adds quantile boxplots to the display Mean Diamonds - adds mean diamonds to the plot Mean Lines – adds a horizontal showing the mean for each group/population. Mean CI Lines – adds lines depicting the 95% confidence interval for the population mean of each group to the plot. Mean Error Bars - adds the means and standard errors (Ch. 6) to the plot Std Dev Lines - add lines one standard deviation above and below the mean. Connect Means - adds line segments connecting the individual means. X-Axis Proportion - if checked the space allocated to the groups will proportional to the sample size for that group. Points Spread – staggers the points much more than jittering. Points Jittered – “jitters” the points so individual observations are more easily seen. Histograms – adds histograms</p><p>Example 2 – GPA vs. Gender Comments:</p><p>38 Example 3 – GPA vs. Skipping Class (Data File: WSU Student Survey) How do the GPAs of students who skip at least one class per week compare to those who do not?</p><p>Example 4 - Education Level Attained and Age at 1st Birth (Data File: Current Pop Survey) How does the age at which a woman had her first child differ across the different education levels?</p><p>39</p>
Details
-
File Typepdf
-
Upload Time-
-
Content LanguagesEnglish
-
Upload UserAnonymous/Not logged-in
-
File Pages39 Page
-
File Size-