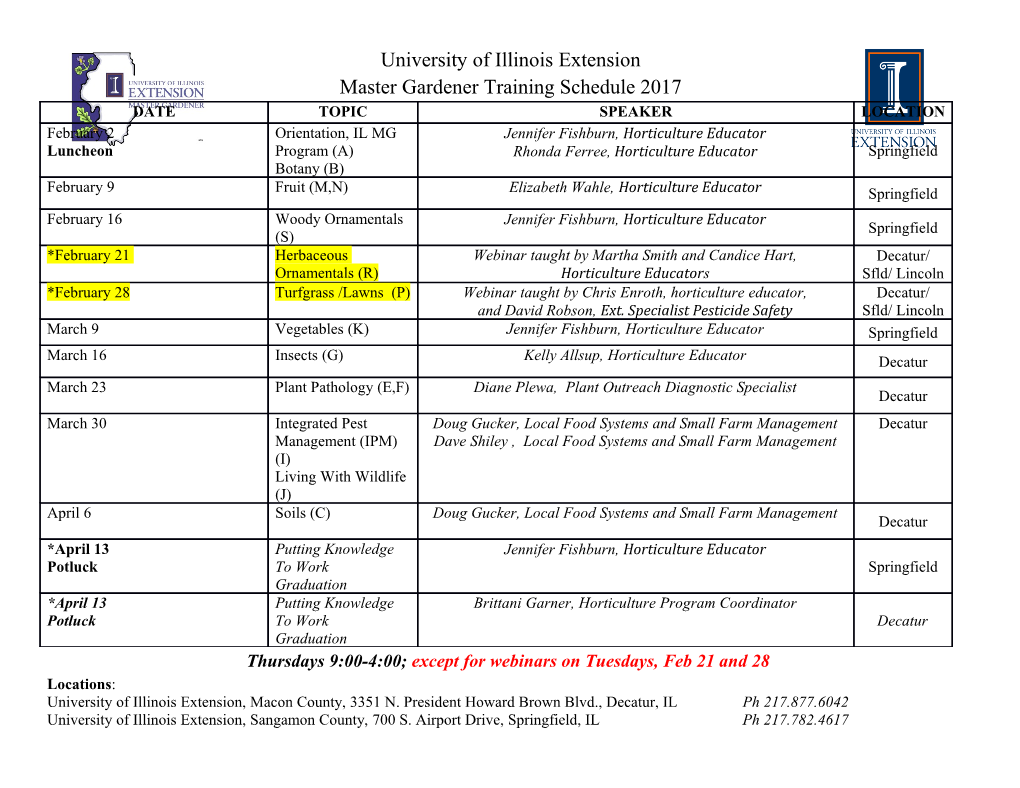
<p>Analysis of a Schizophrenic Patient’s Score on a Test</p><p> of Perceptual Speed: The Effects of a Tranquilizer</p><p>Emily Zitek</p><p>November 26, 2001</p><p>STAT 421 Introduction</p><p>I did a time series analysis on a schizophrenic patient’s daily scores on a test of perceptual speed. There are 120 observations. From the 61st day on, the patient was given a strong tranquilizer that was supposed to decrease perceptual speed. In this project, I will first look at the data set as a whole. I will model the data and test to see whether the tranquilizer had an effect on the patient’s performance. I will then look at the two halves of the data separately. I will compare their correlation structures and spectral densities to examine the effects of the tranquilizer. </p><p>Modeling the data</p><p>First I looked at the plots of the entire data set. The time series plot, the plot of the autocorrelation function (ACF), the plot of the partial autocorrelation function (PACF), and the histogram appear below. </p><p>Scores on a perceptual speed test by a schizophrenic patient 5 2 0 0 8 2 5 1 e r 0 o 6 c s 0 1 0 4 5 0 0 20 40 60 80 100 120 time in days 20 40 60 80 100</p><p>Series : schiz Series : schiz acf pacf I then took a log transformation of the data in order to even out the variance. This series is </p><p> clearly not stationary. </p><p> log transform 4 . 4 0 . 4 6 . 3 2 . 3</p><p>0 20 40 60 80 100 120 Time</p><p>Nonparametric Density Estimate ACF PACF 8 . 0 0 . . 0 1 1 y $ ) 5 7 7 . . 0 0</p><p>=</p><p>5 5 . . h t 0 0 d i w 6 d . n 0 a b</p><p>, " l F F 0 0 a . . C C m 0 0 A A r 5 o . n 0 "</p><p>= l e n r e 5 5 . . 4 k .</p><p>0 0 , 0 - - x ( h t o o m s 3 . k 0 0 0 . . 1 1 - -</p><p>3.2 3.4 3.6 3.8 4.0 4.2 4.4 0 5 10 15 20 0 5 10 15 log transform Lag Lag The slow decay in the ACF of the log transform of the data led me to take the first difference. </p><p>After taking the first difference of the log transform of the data, the data looked much more </p><p> stationary. However, as can be seen in the time series plot, there were a few outliers. </p><p>1st difference 5 . 0 5 . 0 -</p><p>0 20 40 60 80 100 120 Time</p><p>Nonparametric Density Estimate ACF PACF 0 0 . . 1 1 y $ ) 0 . 5 1 7 . 0</p><p>=</p><p>5 5 . . h t 0 0 d i 8 . w 0 d n a b</p><p>, " l F F 0 0 a . . 6 C C . m 0 0 A A r 0 o n "</p><p>= l e 4 n . r 0 e 5 5 . . k</p><p>0 0 , - - x ( h t o 2 o . m 0 s k 0 0 . . 1 1 - -</p><p>-0.5 0.0 0.5 0 5 10 15 20 0 5 10 15 1st difference Lag Lag Because the outliers could cause some problems, I used the acm.smo command in S-Plus to </p><p> smooth the data. After this step, I felt ready to fit a model to the data. </p><p> differenced and smoothed 4 . 0 0 . 0 4 . 0 -</p><p>0 20 40 60 80 100 120</p><p>Time</p><p>Nonparametric Density Estimate ACF PACF 2 0 0 . . . 1 1 1 y $ ) 5 7 . 0</p><p>=</p><p>0 h . 5 5 t . . 1 d i 0 0 w d n a b</p><p>, " l 8 a . F F 0 0 0 m . . r C C 0 0 o A A n "</p><p>= l e n r 6 . e 0 k 5 5</p><p>. . , 0 0 x - - ( h t o o m s 4 . k 0 0 0 . . 1 1 - -</p><p>-0.4 -0.2 0.0 0.2 0.4 0 5 10 15 20 0 5 10 15 differenced and smoothed Lag Lag</p><p>I examined the ACF and PACF in order to fit an appropriate model based on the </p><p> techniques described in Table 2.1 of our text book, Time Series Analysis and Its Applications </p><p>(Shumway & Stoffer, 2000). The ACF appeared to cut off after lag=1, and the PACF appeared </p><p> to decay exponentially. Therefore, an MA(1) seemed appropriate. (I tried fitting other models as</p><p> well, but the MA(1) model had the lowest AIC, AICc, and SIC values.) After fitting an MA(1), I examined how well the model fit. The p-values of the Ljung-</p><p>Box statistic were all greater than .05, so I failed to reject the null hypothesis that my residuals were white noise. Additionally, the ACF and PACF of the residuals both looked mostly like white noise. As can been seen from the histogram of the residuals, they were not perfectly normally distributed, but they were close enough. The MA(1) model fit pretty well. </p><p>ARIMA Model Diagnostics: diffschiz Plot of Standardized Residuals 2 1 0 1 - 2 - 3 - 0 20 40 60 80 100 120</p><p>0 ACF Plot of Residuals . 1 5 . 0 F 0 . C 0 A 0 . 1 - 0 5 10 15 20 PACF Plot of Residuals 2 . 0 1 . 0 F C 0 . A 0 P 2 . 0 - 5 10 15 20</p><p>P-values of Ljung-Box Chi-Squared Statistics 8 . 0 e u l a v 4 - . p 0 0 . 0 2 4 6 8 10 12 14 Lag ARIMA(0,0,1) Model with Regression Parameters 0.007285185</p><p>Histogram of the residuals</p><p>30</p><p>25</p><p>20</p><p>15</p><p>10</p><p>5</p><p>0</p><p>-3 -2 -1 0 1 2 3 Testing the null hypothesis</p><p>A question of interest for this project is, “Did the tranquilizer have an effect on the patient’s scores on the test of perceptual speed?” I first tried to test the null hypothesis that the mean of the first 60 days and the mean of the second 60 days were the same using the differenced data. I got a t-value of -0.49, which was not significant at the .05 level. However, after examining the data again, I concluded that I missed the effect of the tranquilizer by using the differenced data. Therefore, I decided to test the difference between the means by using a smoothed version of the log transform of the data. When I did this, I calculated a t-value of </p><p>–15.8. This t-value allowed me to reject the null hypothesis. However, I must point out that the way I did the t-test did not take the trends in the data into consideration. </p><p>A Closer Look at the Two Series</p><p>I then decided to look at the first 60 days and the second 60 days separately. I wanted to know if the correlation structure changed after the patient received the tranquilizer.</p><p>The First 60 Days</p><p>For the first 60 days, I transformed the data in the same way I transformed the entire data set. First, I took the log transform in order to even out the variance. I then took a first difference since there appeared to be a linear trend. After taking the first difference, there were two outliers. I used the acm.smo command in S-Plus to smooth the data and eliminate the outliers. </p><p>After these steps, the data appeared to be stationary. The plots of the data at each of these stages appear on the following two pages. first 60 days</p><p>0 10 20 30 40 50 60 Nonparametric Density Estimate ACF PACF</p><p>40 50 60 70 80 90 0 5 10 15 0 5 10 15</p><p> first 60 days, log transform 4 . 4 0 . 4 6 . 3</p><p>0 10 20 30 40 50 60 Time</p><p>Nonparametric Density Estimate ACF PACF 2 0 0 . . . 1 1 1 y $ ) 5 7 . 0 . 0</p><p>1 =</p><p>5 5 . . h t 0 0 d i w d 8 n . 0 a b</p><p>, " l F F 0 0 a . . C C m 0 0 A A r o 6 . n 0 "</p><p>= l e n r e 5 5 . . k 4</p><p>. 0 0 , - - 0 x ( h t o o m s 2 k . 0 0 0 . . 1 1 - -</p><p>3.6 3.8 4.0 4.2 4.4 0 5 10 15 0 5 10 15 first 60 days, log transform Lag Lag first 60 days, 1st difference 5 . 0 5 . 0 -</p><p>0 10 20 30 40 50 60 Time</p><p>Nonparametric Density Estimate ACF PACF 0 0 2 . . . 1 1 1 y $ ) 5 7 . 0 0 . = 1 h 5 5 t . . d i 0 0 w d n 8 . a 0 b</p><p>, " l a F F 0 0 m . . r C C 0 0 6 o A A . n 0 "</p><p>= l e n r 4 e . k 5 5 0</p><p>. . , 0 0 x - - ( h t o o 2 . m 0 s k 0 0 . . 1 1 - -</p><p>-0.5 0.0 0.5 0 5 10 15 0 5 10 15 first 60 days, 1st difference Lag Lag</p><p>First 60 days, differenced and smoothed 2 . 0 2 . 0 -</p><p>0 10 20 30 40 50 60</p><p>Time</p><p>Nonparametric Density Estimate ACF PACF 0 0 . . 1 1 y $ ) 2 . 5 1 7 . 0</p><p>=</p><p> h 5 5 t . . 1 d . i 0 0 1 w d n a b</p><p>, 0 " . l 1 a F F 0 0 m . . C C r 0 0 o A A n "</p><p>9 = .</p><p> l 0 e n r e k 5 5</p><p>. . , 8 0 0 x . - - ( 0 h t o o m s 7 k . 0 0 0 . . 1 1 - -</p><p>-0.2 0.0 0.2 0 5 10 15 0 5 10 15 First 60 days, differenced and smoothed Lag Lag Based on the ACF and PACF of the differenced and smoothed data, I decided to fit an MA(1) to the data from the first 60 days. This model appeared to fit well. The ACF and PACF of the residuals both looked like white noise, and the plot of the standardized residuals looked close to white noise, too. Also, the p-values of the Ljung-Box statistic were all greater than .05, so I failed to reject the null hypothesis that my residuals were white noise. </p><p>ARIMA Model Diagnostics: diffschiza Plot of Standardized Residuals 2 1 0 1 - 2 -</p><p>0 10 20 30 40 50 60</p><p>0 ACF Plot of Residuals . 1 5 . 0 F 0 . C 0 A 0 . 1 - 0 5 10 15 PACF Plot of Residuals 3 . 0 1 . 0 F C A P 1 . 0 - 3 . 0 - 5 10 15 P-values of Ljung-Box Chi-Squared Statistics 6 . 0 4 e . u 0 l a v - p 2 . 0 0 . 0 2 4 6 8 10 12 14 Lag ARIMA(0,0,1) Model with Mean 0.01073154</p><p>Histogram of the residuals</p><p>14</p><p>12</p><p>10</p><p>8</p><p>6</p><p>4</p><p>2</p><p>0</p><p>-3 -2 -1 0 1 2 The Second 60 Days</p><p>I followed the same general transformation pattern for the second half of the data. First I plotted the data. Then I took the log transform of the data. Then I took the first difference of the log transform of the data because the ACF decayed very slowly. I did not need to use the acm.smo command for the second half of the data, though, because there were no extreme outliers. The plots from each step can be found below.</p><p> second 60 days 0 7 0 6 0 5 0 4 0 3</p><p>0 10 20 30 40 50 60 Nonparametric Density Estimate ACF PACF 0 0 . . 1 1 6 0 . 5 5 . . 0 0 0 4 0 . 0 0 . . 0 0 0 2 5 5 0 . . . 0 0 0 - - 0 0 0 . . . 1 1 0 - -</p><p>30 40 50 60 70 0 5 10 15 0 5 10 15 second 60 days, log transform 2 . 4 0 . 4 8 . 3 6 . 3 4 . 3 2 . 3</p><p>0 10 20 30 40 50 60 Nonparametric Density Estimate ACF PACF 0 0 . . 1 1 8 . 0 5 5 . . 0 0 0 0 6 . . . 0 0 0 5 5 . . 0 0 - - 4 . 0 0 0 . . 1 1 - -</p><p>3.2 3.4 3.6 3.8 4.0 4.2 0 5 10 15 0 5 10 15</p><p> second 60 days, 1st difference 5 . 0 0 . 0 5 . 0 -</p><p>0 10 20 30 40 50 60 Nonparametric Density Estimate ACF PACF 0 0 . . 1 1 0 . 1 5 5 . . 0 0 8 . 0 6 0 0 . . . 0 0 0 4 . 0 5 5 . . 0 0 - - 2 . 0 0 0 . . 1 1 - -</p><p>-0.5 0.0 0.5 0 5 10 15 0 5 10 15 Based on the ACF and PACF of the first difference of the log transform of the second half of the data, I decided to try to fit an AR(5). This model appeared to fit very well. The p- values of the Ljung-Box statistic were all greater than .05, and the ACF and PACF of the residuals looked like white noise. </p><p>ARIMA Model Diagnostics: diffschizb Plot of Standardized Residuals 2 1 0 1 - 2 - 3 - 0 10 20 30 40 50 60</p><p>0 ACF Plot of Residuals . 1 5 . 0 F 0 . C 0 A 0 . 1 - 0 5 10 15 20 PACF Plot of Residuals 3 . 0 1 . 0 F C A P 1 . 0 - 3 . 0 - 5 10 15 20</p><p>P-values of Ljung-Box Chi-Squared Statistics 8 . 0 e u l a v 4 - . p 0 0 . 0 6 8 10 12 14 Lag ARIMA(5,0,0) Model with Mean -0.007389551</p><p>Histogram of the residuals</p><p>20</p><p>15</p><p>10</p><p>5</p><p>0</p><p>-3 -2 -1 0 1 2 3 Because I fit an MA(1) to the first half of the data, I also tried to fit this model to the second half of the data. This model also appeared to be a good fit. The p-values of the Ljung-Box </p><p>Statistic were all greater than .05. However, the AIC, AICc, and SIC values of the AR(5) model were lower than they were for the MA(1) model, so the AR(5) model was a slightly better fit.</p><p>The Spectral Densities</p><p>The plots of the spectral densities of each half of the data are located below. I used the maximum likelihood estimator (MLE) parameter estimates for the first 60 days, and I used the </p><p>Yule-Walker parameter estimates for the second 60 days.</p><p>First 60 days, MA(1) 5 1 - 0 2 - 5 2 - 0 3 -</p><p>0.0 0.1 0.2 0.3 0.4 0.5 Second 60 days, AR(5) 0 1 - 5 1 - 0 2 -</p><p>0.0 0.1 0.2 0.3 0.4 0.5</p><p>The spectral densities were not exactly the same, but they were similar. Both halves of the data had few low frequencies and more high frequencies. Conclusion</p><p>It appears that the tranquilizer caused the schizophrenic patient to get lower scores on the test of perceptual speed during the second 60 days, but the tranquilizer probably did not have much more of an effect than that. Although I did fit two different models to the two halves of the data, the fact that the MA(1) was a reasonable fit (although not the best fit) for both halves of the data leads me to believe that the tranquilizer probably did not have a large effect on the correlation structure of the data. My belief that the tranquilizer only caused the patient to get lower scores is also supported by the fact that the spectral densities are similar for the two halves of the data.</p><p>More research can be done to determine the effects of the tranquilizer. Future data analyses could bootstrap each half of the data and then fit a model in order to see if the model is the same or not. If it is determined that the tranquilizer actually does have an effect on the correlation structure, then psychological research would need to determine why. Reference</p><p>Shumway, Robert H., and David S. Stoffer (2000). Time Series Analysis and Its </p><p>Applications. New York: Springer-Verlag. Appendix A: S-Plus Code</p><p># Part 1-modeling the entire data set </p><p># schizophrenia data</p><p># from http://www-personal.buseco.monash.edu.au/~hyndman/TSDL/ # " Daily observations of the score achieved by a schizophrenic patient on a test # of perceptual speed. From the 61st day, the patient began receiving a powerful # tranquilizer(chlorpromazine) that could be expected to reduce perceptual speed. # Source: Pankratz. Original source: Glass (1975). Also in McCleary & Hay (1980)."</p><p># schizo is the data set with all 120 observations schiz<-schizo[,1] tsplot(schiz) title(main="Scores on a perceptual speed test by a schizophrenic patient", xlab=" time in days", ylab="score") acf(schiz) title(" acf") acf(schiz, type="partial") title(" pacf") hist(schiz)</p><p># I take the ln transform to try to even out the variability lnschiz<-log(schiz) kdescripb(lnschiz, "log transform") # linear trend so I take the first difference dschiz<-diff(lnschiz, 1) kdescripb(dschiz, "1st difference") # there are extreme outliers # My attempt at using acm.smo argm<-ar.gm(dschiz) acmsmos<-acm.smo(dschiz, argm) diffschiz<-acmsmos$smo kdescripb(diffschiz, "differenced and smoothed")</p><p># prettyplot(2,1) # acf(diffschiz) # title(main=" acf") # acf(diffschiz, type="partial") # title(main=" pacf") karima.diag<-function(stresid,varest,p,q,n){ par(mfrow=c(2,1)) qqnorm(stresid) hist(stresid) par(mfrow=c(1,1))</p><p> k<-p+q loglik<-log(varest) aic<-loglik+ 2*k/n aicc<-loglik+(n+k)/(n-k-2) sic<-loglik+ k*log(n)/n return(aic,aicc,sic) }</p><p># fit a model</p><p># could be MA(1) regime<-rep(0,119) for (i in 61:119) regime[i]<-1 ones<-rep(1,119) Z<-cbind(ones,regime) # the matrix of xreg in arima.mle schma<-arima.mle(diffschiz,model=list(order=c(0,0,1)),xreg=Z) schmad<-arima.diag(schma) schmadk<-karima.diag(schmad$std.resid,schma$sigma2,0,1,60) betastderr<-schma$sigma2*solve(crossprod(Z,Z))</p><p># look to see if the residuals are normally distributed</p><p># now get the info for the t-test using the log transform # there are 120 observations since there is no differencing argmln<-ar.gm(lnschiz) acmsmoln<-acm.smo(lnschiz, argmln) schizln<-acmsmoln$smo regimeln<-rep(0,120) for (i in 61:120) regimeln[i]<-1 onesln<-rep(1,120) Zln<-cbind(onesln,regimeln) # the matrix of xreg in arima.mle schmaln<-arima.mle(schizln,model=list(order=c(0,0,1)),xreg=Zln) betastderrln<-schmaln$sigma2*solve(crossprod(Zln,Zln))</p><p># Part 2 # Now I will look at the two halves of the data more closely # Two different models of the data</p><p># first 60 days</p><p># schizo60 is the data set with observations 1-60 schiza<-schiz60[,1] kdescripb(schiza, "first 60 days") # I take the ln transform to try to even out the variability lnschiza<-log(schiza) kdescripb(lnschiza, "first 60 days, log transform") # linear trend so I take the first difference dschiza<-diff(lnschiza, 1) kdescripb(dschiza, "first 60 days, 1st difference") # there are extreme outliers # My attempt at using acm.smo argm<-ar.gm(dschiza) acmsmo<-acm.smo(dschiza, argm) diffschiza<-acmsmo$smo kdescripb(diffschiza, "First 60 days, differenced and smoothed")</p><p># I had a few problems with labeling the plots prettyplot(2,1) acf(diffschiza) title(main=" acf") acf(diffschiza, type="partial") title(main=" pacf") karima.diag<-function(stresid,varest,p,q,n){ par(mfrow=c(2,1)) qqnorm(stresid) hist(stresid) par(mfrow=c(1,1))</p><p> k<-p+q loglik<-log(varest) aic<-loglik+ 2*k/n aicc<-loglik+(n+k)/(n-k-2) sic<-loglik+ k*log(n)/n return(aic,aicc,sic) }</p><p># fit a model</p><p># could be MA(1) schma<-arima.mle(diffschiza,model=list(order=c(0,0,1)),xreg=1) schmad<-arima.diag(schma) schmadk<-karima.diag(schmad$std.resid,schma$sigma2,0,1,60) # look to see if the residuals are normally distributed</p><p># see commands window to check which model to use</p><p># second 60 days, patient was given a tranquilizer</p><p># schiz61 is the data set with observations 61-120 schizb<-schiz61[,1] kdescripb(schizb, "second 60 days") lnschizb<-log(schizb) kdescripb(lnschizb, "second 60 days, log transform") diffschizb<-diff(lnschizb, 1) kdescripb(diffschizb, "second 60 days, 1st difference") prettyplot(2,1) acf(diffschizb) title(main=" acf") acf(diffschizb, type="partial") title(main=" pacf")</p><p># AR(5) scharb<-arima.mle(diffschizb,model=list(order=c(5,0,0)),xreg=1) schardb<-arima.diag(scharb) schardkb<-karima.diag(schardb$std.resid,scharb$sigma2,5,0,60)</p><p># I tried various ARIMA models in the commands window and ARIMA(5,0,0) had the lowest AIC, AICc, and SIC</p><p># examine the spectral densities for the two halves of the data prettyplot(2,1) spec.ma(.785,1,schma$sigma2,60) title("First 60 days, MA(1)") ardiffs<-ar(diffschizb) specar<-spec.ar(ardiffs,n.freq=60) plot(specar$freq,specar$spec,type="l") title("Second 60 days, AR(5)") Appendix B: Commands Window Output</p><p>Output from Program 1</p><p>> schma</p><p>Call: arima.mle(x = diffschiz, model = list(order = c(0, 0, 1)), xreg = </p><p>Z) </p><p>Method: Maximum Likelihood </p><p>Model : 0 0 1 </p><p>Coefficients:</p><p>MA : 0.78053</p><p>Variance-Covariance Matrix:</p><p> ma(1) ma(1) 0.003283842</p><p>Coeffficients for regressor(s): Z </p><p>[1] 0.00729 -0.01366</p><p>Optimizer has converged</p><p>Convergence Type: relative function convergence</p><p>AIC: -105.93384</p><p>> betastderr</p><p> ones regime </p><p> ones 0.000377954 -0.000377954 regime -0.000377954 0.000762314</p><p>> -.01366/sqrt(.000762314)</p><p>[1] -0.4947477</p><p>> schmaln</p><p>Call: arima.mle(x = schizln, model = list(order = c(0, 0, 1)), xreg = Zl n) </p><p>Method: Maximum Likelihood </p><p>Model : 0 0 1 </p><p>Coefficients:</p><p>MA : -0.50538</p><p>Variance-Covariance Matrix:</p><p> ma(1) ma(1) 0.006204921</p><p>Coeffficients for regressor(s): Zln </p><p>[1] 4.26129 -0.57978</p><p>Optimizer has converged</p><p>Convergence Type: relative function convergence</p><p>AIC: -38.28649</p><p>> betastderrln</p><p> onesln regimeln onesln 0.0006730214 -0.0006730214 regimeln -0.0006730214 0.0013460428</p><p>> -.57978/sqrt(.0013460428)</p><p>[1] -15.80279</p><p>Output from Program 2</p><p>> schma</p><p>Call: arima.mle(x = diffschiza, model = list(order = c(0, 0, 1)), xreg =</p><p>1) </p><p>Method: Maximum Likelihood </p><p>Model : 0 0 1 </p><p>Coefficients:</p><p>MA : 0.78538</p><p>Variance-Covariance Matrix:</p><p> ma(1) ma(1) 0.006494673</p><p>Coeffficients for regressor(s): intercept </p><p>[1] 0.01073</p><p>Optimizer has converged</p><p>Convergence Type: relative function convergence AIC: -84.15047</p><p>> schmadk</p><p>$aic:</p><p>[1] -4.314878</p><p>$aicc:</p><p>[1] -3.278036</p><p>$sic:</p><p>[1] -4.279972</p><p>> scharb</p><p>Call: arima.mle(x = diffschizb, model = list(order = c(5, 0, 0)), xreg =</p><p>1) </p><p>Method: Maximum Likelihood </p><p>Model : 5 0 0 </p><p>Coefficients:</p><p>AR : -0.59593 -0.31261 -0.36977 -0.36987 -0.29883</p><p>Variance-Covariance Matrix:</p><p> ar(1) ar(2) ar(3) ar(4) ar(5) ar(1) 0.016864857 0.008988919 0.003742838 0.005117587 0.003551737 ar(2) 0.008988919 0.020907929 0.009906077 0.005682256 0.005117587 ar(3) 0.003742838 0.009906077 0.020185666 0.009906077 0.003742838 ar(4) 0.005117587 0.005682256 0.009906077 0.020907929 0.008988919 ar(5) 0.003551737 0.005117587 0.003742838 0.008988919 0.016864857</p><p>Coeffficients for regressor(s): intercept </p><p>[1] -0.00739</p><p>Optimizer has NOT converged</p><p>Due to: iteration limit</p><p>AIC: -21.18131</p><p>> schardkb</p><p>$aic:</p><p>[1] -3.285679</p><p>$aicc:</p><p>[1] -2.225931</p><p>$sic:</p><p>[1] -3.11115</p>
Details
-
File Typepdf
-
Upload Time-
-
Content LanguagesEnglish
-
Upload UserAnonymous/Not logged-in
-
File Pages24 Page
-
File Size-