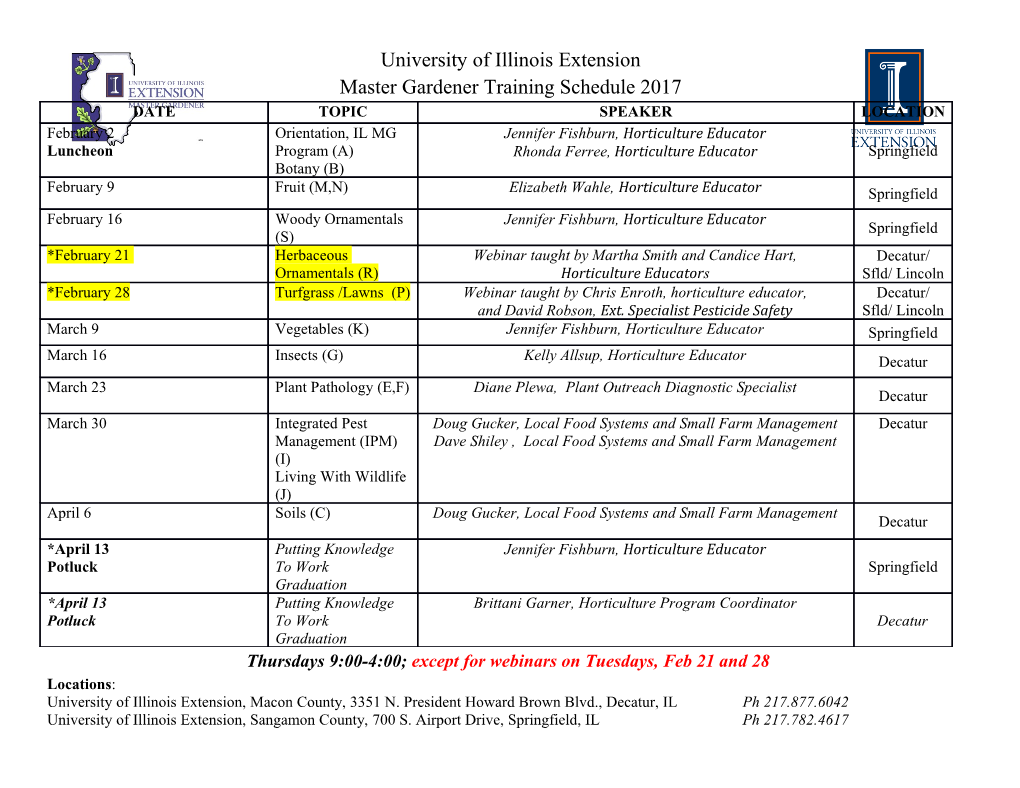
<p> 1</p><p>4.3 Sampling From A Multivariate Normal Distribution And Maximum Likelihood Estimation (a) The Multivariate Normal Likelihood</p><p>Let X1, X 2 ,, X n ~ N, be a random sample from a multivariate normal population. Then, the joint density function of</p><p>X1, X 2 ,, X n</p><p> n t 1 1 x j x j f x ,, x exp 1 n p 1 2 2 j1 2 2 n t 1 . x j x j 1 exp j1 np n 2 2 2 2 </p><p>The following results will be used to obtain the maximum likelihood estimate of and . </p><p>Result: Let A be a p p symmetric matrix and x be a p 1 vector. Then, </p><p> t t t (a) x Ax trx Ax trAxx , where A aij and</p><p> p trA aii . i1</p><p> p (b) trA i , where i are the eigenvalues of A. i1</p><p>Based on the result, we have 2</p><p> n n t 1 t 1 x j x j trx j x j j1 j1 n 1 t tr x j x j j1 n 2A.12, p. 98 tr 1 x x t j j j1 Then, n n t t t x j x j x j x x x j x x j1 j1 n n t t x j xx j x x x j1 j1 n t t x j xx j x nx x j1</p><p> n where xi . Further, x i1 n</p><p> n tr 1 x x x x t n x x t j j j1 n tr 1 x x x x t ntr 1 x x t j j j1 n tr 1 x x x x t n x t 1 x j j j1 </p><p>Therefore, the likelihood function of X1, X 2 ,, X n can be simplified to 3</p><p> n t 1 x j x j 1 j 1 L , f x ,, x exp 1 n np n 2 2 2 2 . n tr 1 x x x x t n x t 1 x j j 1 j1 exp np n 2 2 2 2 (b) Maximum Likelihood Estimation of and To obtain the maximum likelihood estimate, the following result will be used. Result: Given a p p symmetric positive definite matrix B and a scalar b 0 , it follows that 1 tr 1B 1 exp 2b pb exp bp b b 2 B</p><p> 1 B for all positive definite , with equality holding only for 2b . </p><p>Import Result (MLE of and )</p><p>Let X1, X 2 ,, X n ~ N, be a random sample from a multivariate normal population. Then, n t X j X X j X ˆ X and j1 n 1S ˆ n n are the maximum likelihood estimators of and , respectively, where n t X j X X j X S j1 n 1 4</p><p> is a unbiased estimate of . Their observed values, x and</p><p> n 1 x x x x t n j j , are called the maximum likelihood j1 estimates of and . [proof:] ˆ maximizing the function </p><p> n tr 1 x x x x t n x t 1 x j j 1 j1 exp np n 2 2 2 2 also minimizes the function </p><p> n 1 t t 1 tr x x x x n x x . j j j1 </p><p>Since 1 is positive definite, so that nx t 1 x 0 . However, as</p><p> x , the function </p><p> n tr 1 x x x x t n x t 1 x j j j1 n tr 1 x x x x t j j j1 achieves its minimum. It remains to find ˆ maximizing 5</p><p> n tr 1 x x x x t j j 1 j1 L ˆ, exp np n 2 2 2 2 </p><p> n t b n By the previous result with 2 and B x j xx j x , the j1</p><p> n x xx xt maximum occurs at j j . ˆ j1 n</p><p>Note: Maximum likelihood estimators possess an invariance property. Let ˆ be the maximum likelihood estimator of , and consider estimating the parameter h , which is a function of , the maximum likelihood estimate of h is given by hˆ. For examples, </p><p>1. The maximum likelihood estimator of t 1 is ˆ t ˆ 1ˆ . </p><p>2. The maximum likelihood estimator of ii is ˆ ii , where </p><p> n 2 X X ij i . ˆ j1 ii n Note: </p><p>Let X1, X 2 ,, X n ~ N, be a random sample from a multivariate normal population. Then, n t X j X X j X ˆ X and j1 ˆ n are sufficient statistics. 6</p><p>4.4 The Sampling Distribution of X and S Definition of the Wishart Distribution: </p><p>Let Z1,Z2 ,,Zn ~ N p 0, be independently distributed. Then,</p><p> n t the random matrix M Z j Z j is distributed as a Wishart j1 distribution with n d.f., Wn, . The density of a Wishart distribution with n d.f. is (n p1) trm 1 m 2 exp 2 . f m | p pn p( p1) n 2 4 2 1 2 n 1 i i1 2 </p><p>Properties of the Wishart Distribution: 1. If M ~ W and M ~ W , then 1 n1 , 2 n2 ,</p><p>M M ~ W . 1 2 n1 n2 ,</p><p> t 2. If M ~ W , then CM1C ~ W t . 1 n1 , n1 ,CC</p><p>Import Result: 1. X ~ N p , . n n t 2. n 1S X j X X j X ~ Wn1, . j 1</p><p>3. X and n 1S are independent. </p><p>4.5 Large-Sample Behavior of X and S Import Result: 7</p><p>Let X1, X 2 ,, X n be a random sample from a population with mean and finite (nonsingular) covariance . Then, </p><p> nX N p 0, and </p><p> t 1 2 nX S X p for n p large. </p>
Details
-
File Typepdf
-
Upload Time-
-
Content LanguagesEnglish
-
Upload UserAnonymous/Not logged-in
-
File Pages7 Page
-
File Size-