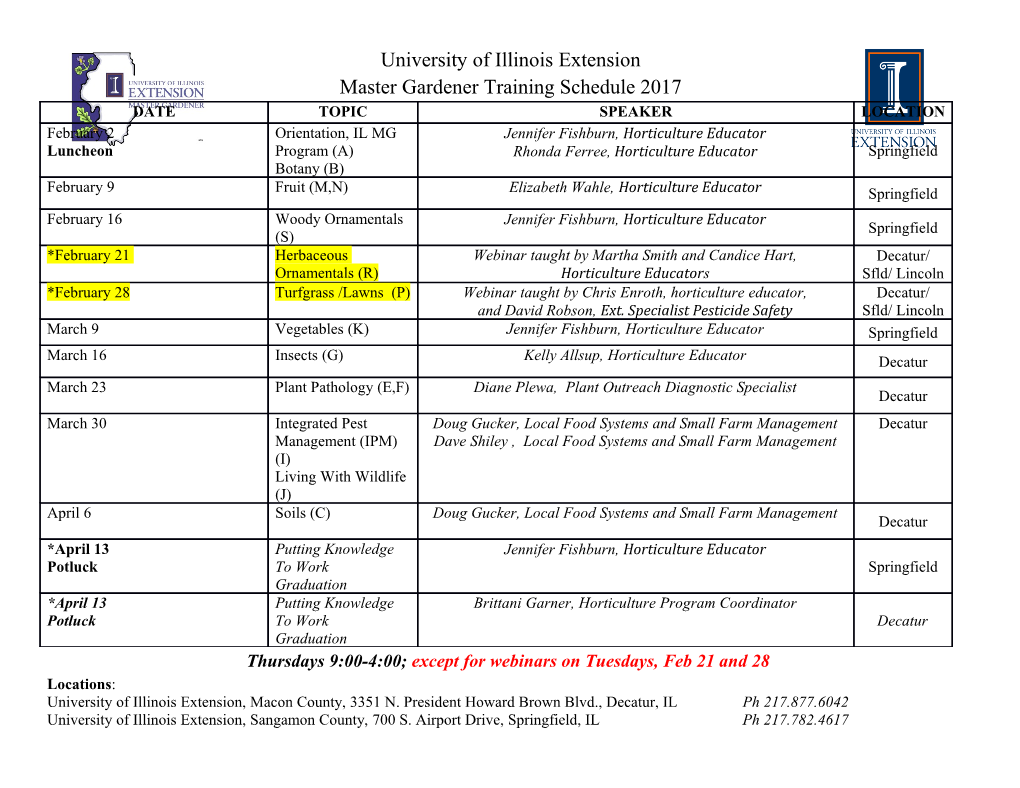
<p> Event History Modeling: A Guide for Social Scientists by Janet M. Box-Steffensmeier and Bradford S. Jones</p><p>Relevant Files for Stata Output Data file: UNFINAL2.DTA (Stata 7.0 Dataset; already stset) “DO” file: UNpeacekeeping1.do (Contains code) Log file: UNpeacekeeping1.doc (Log file in WORD format of output from “do” file; does not contain graphs) UNpeacekeeping1.rtf (Log file in RTF format of output from “do” file; does not contain graphs)</p><p>The following is a Stata log file containing the output generated from the file “Unpeacekeeping1.do”. This Stata DO file will generate Kaplan-Meier estimates, Weibull (and exponential) estimates, and Cox estimates using the U.N. Peacekeeping data. Results from parametric and Cox models are published in Chapters 3 and 4 of Event History Modeling: A Guide for Social Scientists. Graphs generated from the “do” file are not reproduced in this file (though if you execute the file, the graphs will be saved on your computer). </p><p> log: c:\ehbook\UNpeacekeeping.log</p><p>. do c:\ehbook\Unpeacekeeping1</p><p>. * DATA SET USED IS UNFINAL2.DTA* . *Note that all variables that are created in this file are DROPPED; edit as necessary.* . . use c:\data\statadata\UNFINAL2</p><p>. . *Note that directory may be different depending on the location of your data files* . . * 1 Produce Kaplan-Meier Estimates:* . sts list </p><p> failure _d: failed analysis time _t: duration</p><p>Beg. Net Survivor Std. Time Total Fail Lost Function Error [95% Conf. Int.] ------2 54 1 0 0.9815 0.0183 0.8757 0.9974 4 53 1 0 0.9630 0.0257 0.8599 0.9906 5 52 2 0 0.9259 0.0356 0.8146 0.9715 7 50 3 0 0.8704 0.0457 0.7472 0.9360 10 47 1 0 0.8519 0.0483 0.7255 0.9230 11 46 1 0 0.8333 0.0507 0.7042 0.9096 12 45 2 0 0.7963 0.0548 0.6624 0.8816 13 43 1 1 0.7778 0.0566 0.6420 0.8672 15 41 2 0 0.7398 0.0598 0.6005 0.8369 16 39 1 1 0.7209 0.0612 0.5802 0.8214 18 37 1 0 0.7014 0.0626 0.5594 0.8053 19 36 1 0 0.6819 0.0638 0.5389 0.7889 21 35 1 0 0.6624 0.0649 0.5186 0.7723 23 34 2 1 0.6235 0.0667 0.4789 0.7385 25 31 3 1 0.5631 0.0687 0.4185 0.6848 26 27 1 0 0.5423 0.0693 0.3980 0.6660 28 26 1 0 0.5214 0.0697 0.3777 0.6469 29 25 1 1 0.5005 0.0699 0.3577 0.6276 30 23 2 0 0.4570 0.0703 0.3164 0.5870 31 21 1 0 0.4353 0.0702 0.2962 0.5663 34 20 1 0 0.4135 0.0700 0.2764 0.5453 46 19 3 0 0.3482 0.0684 0.2189 0.4807 48 16 2 0 0.3047 0.0664 0.1823 0.4362 49 14 1 0 0.2829 0.0651 0.1645 0.4134 59 13 0 1 0.2829 0.0651 0.1645 0.4134 66 12 1 0 0.2593 0.0638 0.1453 0.3890 70 11 1 0 0.2358 0.0622 0.1266 0.3642 71 10 0 1 0.2358 0.0622 0.1266 0.3642 99 9 0 1 0.2358 0.0622 0.1266 0.3642 127 8 0 2 0.2358 0.0622 0.1266 0.3642 128 6 1 0 0.1965 0.0630 0.0912 0.3310 284 5 0 1 0.1965 0.0630 0.0912 0.3310 319 4 0 1 0.1965 0.0630 0.0912 0.3310 452 3 0 1 0.1965 0.0630 0.0912 0.3310 634 2 0 1 0.1965 0.0630 0.0912 0.3310 641 1 0 1 0.1965 0.0630 0.0912 0.3310 ------</p><p>. . * 2 Produce Graph of K-M Estimates given from above command* . sts graph, t1("Kaplan-Meier Survival Function Estimates") b2("Duration of U.N. Mission") noborder saving(UN > KMgraph1, replace)</p><p> failure _d: failed analysis time _t: duration</p><p>. . * 3 Produces Kaplan-Meier Estimates for the three conflict types* . sts list, by(contype)</p><p> failure _d: failed analysis time _t: duration</p><p>Beg. Net Survivor Std. Time Total Fail Lost Function Error [95% Conf. Int.] ------contype=1 4 14 1 0 0.9286 0.0688 0.5908 0.9896 5 13 1 0 0.8571 0.0935 0.5394 0.9622 12 12 1 0 0.7857 0.1097 0.4725 0.9254 13 11 1 1 0.7143 0.1207 0.4063 0.8819 15 9 1 0 0.6349 0.1308 0.3312 0.8297 16 8 1 0 0.5556 0.1364 0.2636 0.7717 23 7 1 0 0.4762 0.1381 0.2026 0.7083 25 6 1 1 0.3968 0.1360 0.1478 0.6396 28 4 1 0 0.2976 0.1334 0.0820 0.5559 30 3 1 0 0.1984 0.1203 0.0343 0.4603 34 2 1 0 0.0992 0.0924 0.0061 0.3504 46 1 1 0 0.0000 . . . contype=2 7 10 1 0 0.9000 0.0949 0.4730 0.9853 16 9 0 1 0.9000 0.0949 0.4730 0.9853 26 8 1 0 0.7875 0.1340 0.3809 0.9426 31 7 1 0 0.6750 0.1551 0.2906 0.8825 70 6 1 0 0.5625 0.1651 0.2094 0.8092 127 5 0 1 0.5625 0.1651 0.2094 0.8092 128 4 1 0 0.4219 0.1737 0.1110 0.7126 319 3 0 1 0.4219 0.1737 0.1110 0.7126 634 2 0 1 0.4219 0.1737 0.1110 0.7126 641 1 0 1 0.4219 0.1737 0.1110 0.7126 contype=3 2 30 1 0 0.9667 0.0328 0.7861 0.9952 5 29 1 0 0.9333 0.0455 0.7589 0.9829 7 28 2 0 0.8667 0.0621 0.6828 0.9478 10 26 1 0 0.8333 0.0680 0.6450 0.9270 11 25 1 0 0.8000 0.0730 0.6080 0.9048 12 24 1 0 0.7667 0.0772 0.5720 0.8813 15 23 1 0 0.7333 0.0807 0.5369 0.8567 18 22 1 0 0.7000 0.0837 0.5026 0.8312 19 21 1 0 0.6667 0.0861 0.4692 0.8047 21 20 1 0 0.6333 0.0880 0.4365 0.7775 23 19 1 1 0.6000 0.0894 0.4045 0.7495 25 17 2 0 0.5294 0.0918 0.3378 0.6889 29 15 1 1 0.4941 0.0922 0.3059 0.6573 30 13 1 0 0.4561 0.0926 0.2716 0.6232 46 12 2 0 0.3801 0.0915 0.2070 0.5521 48 10 2 0 0.3041 0.0876 0.1477 0.4766 49 8 1 0 0.2661 0.0845 0.1202 0.4371 59 7 0 1 0.2661 0.0845 0.1202 0.4371 66 6 1 0 0.2217 0.0812 0.0884 0.3924 71 5 0 1 0.2217 0.0812 0.0884 0.3924 99 4 0 1 0.2217 0.0812 0.0884 0.3924 127 3 0 1 0.2217 0.0812 0.0884 0.3924 284 2 0 1 0.2217 0.0812 0.0884 0.3924 452 1 0 1 0.2217 0.0812 0.0884 0.3924 ------</p><p>. . *4 Produce Graph of K-M Estimates by Conflict Type* . sts graph, by(contype) t1("Kaplan-Meier Survival Function Estimates") b2("Duration of U.N. Mission") nobor > der nolabel saving(UNKMgraph2, replace)</p><p> failure _d: failed analysis time _t: duration</p><p>. *Note that graphs were modified in Corel Draw; I added labels to graph lines using Corel Draw; omitting the > nolabel command* . *will allow Stata to apply labels* . . *Using UN data for exponential and weibull models* . . * 5 Estimate Exponential Model using proportional hazards estimates.* . streg civil interst, dist(exp) nohr</p><p> failure _d: failed analysis time _t: duration Iteration 0: log likelihood = -103.03289 Iteration 1: log likelihood = -90.211473 Iteration 2: log likelihood = -86.44131 Iteration 3: log likelihood = -86.354656 Iteration 4: log likelihood = -86.354481 Iteration 5: log likelihood = -86.354481 </p><p>Exponential regression -- log relative-hazard form </p><p>No. of subjects = 54 Number of obs = 54 No. of failures = 39 Time at risk = 3994 LR chi2(2) = 33.36 Log likelihood = -86.354481 Prob > chi2 = 0.0000</p><p>------_t | Coef. Std. Err. z P>|z| [95% Conf. Interval] ------+------civil | 1.169344 .3588703 3.26 0.001 .4659714 1.872717 interst | -1.6401 .4954337 -3.31 0.001 -2.611132 -.6690679 _cons | -4.350864 .2132007 -20.41 0.000 -4.76873 -3.932999 ------</p><p>. . * 6 Estimate Exponential Model using the accelerated failure time estimates.* . streg civil interst, dist(exp) time</p><p> failure _d: failed analysis time _t: duration</p><p>Iteration 0: log likelihood = -103.03289 Iteration 1: log likelihood = -90.211473 Iteration 2: log likelihood = -86.44131 Iteration 3: log likelihood = -86.354656 Iteration 4: log likelihood = -86.354481 Iteration 5: log likelihood = -86.354481 </p><p>Exponential regression -- accelerated failure-time form </p><p>No. of subjects = 54 Number of obs = 54 No. of failures = 39 Time at risk = 3994 LR chi2(2) = 33.36 Log likelihood = -86.354481 Prob > chi2 = 0.0000</p><p>------_t | Coef. Std. Err. z P>|z| [95% Conf. Interval] ------+------civil | -1.169344 .3588703 -3.26 0.001 -1.872717 -.4659714 interst | 1.6401 .4954337 3.31 0.001 .6690679 2.611132 _cons | 4.350864 .2132007 20.41 0.000 3.932999 4.76873 ------</p><p>. . . *7 Using generate to create variable storing hazard rate for each conflict type (we're referencing coeffic > ients from AFT model)* . . gen haz_civil=exp(-(_b[_cons]+_b[civil])) . gen haz_interstate=exp(-(_b[_cons]+_b[interst]))</p><p>. gen haz_icw=exp(-(_b[_cons]))</p><p>. . *8 Using display to compute h(t) for each conflict type* . display exp(-(_b[_cons]+_b[civil])) .04152249</p><p>. display exp(-(_b[_cons]+_b[interst])) .00250125</p><p>. display exp(-(_b[_cons])) .01289566</p><p>. . *9 We could also use predict option* . predict hazardrate, hazard (4 missing values generated)</p><p>. *Note that the predict option will compute h(t) for each observation.* . . *10 Graphing the hazard rates* . gr haz_civil haz_interstate haz_icw duration, c(lll) s(iii) xlab ylab t1("Hazard Rates") b2("Duration of U. > N. Peacekeeping Missions") saving(UNexp, replace)</p><p>. . *Again, as with all graphs, I label them in Corel. To get the legend attached to the above graph (so you* . * can figure out which line corresponds to which conflict type, omit the s(iii) and t1( ) options).* . . *11 Dropping newly created variables from dataset* . drop haz_* hazardrate</p><p>. . *12 Using display to compute the expected survival time for each conflict type* . display exp(_b[_cons]+_b[civil]) 24.083333</p><p>. display exp(_b[_cons]+_b[interst]) 399.8</p><p>. display exp(_b[_cons]) 77.545455</p><p>. . *13 We could also use predict option* . predict ETexp, mean (option mean time assumed; predicted mean time) (4 missing values generated)</p><p>. *Note that the predict option will compute E(T) for each observation.* . . *14 Using display to compute the median survival time for each conflict type* . display exp(_b[_cons]+_b[civil])*log(2) 16.693295</p><p>. display exp(_b[_cons]+_b[interst])*log(2) 277.12024 . display exp(_b[_cons])*log(2) 53.750413</p><p>. . *15 We could also use predict option* . predict MTexp, median (option median time assumed; predicted median time) (4 missing values generated)</p><p>. . *16 Dropping newly created variables from dataset* . drop ETexp MTexp</p><p>. . *17 Estimate Weibull Model with Proportional Hazards Parameterization* . streg civil interst, dist(weib) nohr</p><p> failure _d: failed analysis time _t: duration</p><p>Fitting constant-only model:</p><p>Iteration 0: log likelihood = -103.03289 Iteration 1: log likelihood = -93.501426 Iteration 2: log likelihood = -93.488663 Iteration 3: log likelihood = -93.488663</p><p>Fitting full model:</p><p>Iteration 0: log likelihood = -93.488663 Iteration 1: log likelihood = -86.548564 Iteration 2: log likelihood = -84.667898 Iteration 3: log likelihood = -84.655162 Iteration 4: log likelihood = -84.655157 </p><p>Weibull regression -- log relative-hazard form </p><p>No. of subjects = 54 Number of obs = 54 No. of failures = 39 Time at risk = 3994 LR chi2(2) = 17.67 Log likelihood = -84.655157 Prob > chi2 = 0.0001</p><p>------_t | Coef. Std. Err. z P>|z| [95% Conf. Interval] ------+------civil | .8879245 .3832017 2.32 0.020 .1368629 1.638986 interst | -1.401441 .5117817 -2.74 0.006 -2.404515 -.3983673 _cons | -3.459909 .4952858 -6.99 0.000 -4.430652 -2.489167 ------+------/ln_p | -.2145617 .1237889 -1.73 0.083 -.4571834 .02806 ------+------p | .806895 .0998846 .6330642 1.028457 1/p | 1.239319 .1534138 .97233 1.579619 ------</p><p>. . *18 Estimate Weibull Model with Accelerated Failure Time Parameterization* . streg civil interst, dist(weib) time</p><p> failure _d: failed analysis time _t: duration</p><p>Fitting constant-only model:</p><p>Iteration 0: log likelihood = -103.03289 Iteration 1: log likelihood = -93.501426 Iteration 2: log likelihood = -93.488663 Iteration 3: log likelihood = -93.488663</p><p>Fitting full model:</p><p>Iteration 0: log likelihood = -93.488663 Iteration 1: log likelihood = -86.548564 Iteration 2: log likelihood = -84.667898 Iteration 3: log likelihood = -84.655162 Iteration 4: log likelihood = -84.655157 </p><p>Weibull regression -- accelerated failure-time form </p><p>No. of subjects = 54 Number of obs = 54 No. of failures = 39 Time at risk = 3994 LR chi2(2) = 17.67 Log likelihood = -84.655157 Prob > chi2 = 0.0001</p><p>------_t | Coef. Std. Err. z P>|z| [95% Conf. Interval] ------+------civil | -1.100421 .4457861 -2.47 0.014 -1.974146 -.2266966 interst | 1.736832 .6165459 2.82 0.005 .5284242 2.94524 _cons | 4.28793 .2652436 16.17 0.000 3.768062 4.807798 ------+------/ln_p | -.2145617 .1237889 -1.73 0.083 -.4571834 .02806 ------+------p | .806895 .0998846 .6330642 1.028457 1/p | 1.239319 .1534138 .97233 1.579619 ------</p><p>. . . *19 Computing estimated hazard rates for each conflict type (we're referencing AFT estimates)* . . *20 First, note that lambda=exp(-beta'x). We can compute lambda for* . *each confict type:* . gen lambda_civil=exp(-(_b[_cons]+_b[civil]))</p><p>. gen lambda_interstate=exp(-(_b[_cons]+_b[interst]))</p><p>. gen lambda_icw=exp(-(_b[_cons]))</p><p>. . *21 Second, note that h(t)=lambda*p*(lambda*t)^(p-1). We can generate the* . *hazard rate for each covariate profile:* . gen haz_civil=lambda_civil*e(aux_p)*(lambda_civil*duration)^(e(aux_p)-1) (4 missing values generated)</p><p>. gen haz_interstate=lambda_interstate*e(aux_p)*(lambda_interstate*duration)^(e(aux_p )-1) (4 missing values generated) . gen haz_icw=lambda_icw*e(aux_p)*(lambda_icw*duration)^(e(aux_p)-1) (4 missing values generated)</p><p>. . *22 Now we can graph the hazard rates* . gr haz_civil haz_interstate haz_icw duration, c(lll) s(iii) ylab xlab t1("Hazard Rates") b2("Duration of U. > N. Peacekeeping Missions") saving(UNweibhaz, replace)</p><p>. . *23 We could also use the predict option to compute h(t) for every observation* . predict ht, hazard (4 missing values generated)</p><p>. . drop lambda_* haz_* ht</p><p>. . *24 Displaying the expected survival times for each conflict type* . display exp(lngamma(1+(1/e(aux_p))))*exp(_b[_cons]+_b[civil]) 27.283894</p><p>. display exp(lngamma(1+(1/e(aux_p))))*exp(_b[_cons]+_b[interst]) 465.70383</p><p>. display exp(lngamma(1+(1/e(aux_p))))*exp(_b[_cons]) 81.999892</p><p>. . *25 We could also use the predict option to compute E(T) for every observation* . predict ETweibull, mean (option mean time assumed; predicted mean time) (4 missing values generated)</p><p>. . *26 Displaying the median survival times for each conflict type* . display exp(_b[_cons]+_b[civil])*log(2)^(1/e(aux_p)) 15.383298</p><p>. display exp(_b[_cons]+_b[interst])*log(2)^(1/e(aux_p)) 262.57471</p><p>. display exp(_b[_cons])*log(2)^(1/e(aux_p)) 46.233457</p><p>. . *27 We could also use the predict option to compute M(T) for every observation* . predict MTweibull, median (option median time assumed; predicted median time) (4 missing values generated)</p><p>. . drop ETweibull MTweibull</p><p>. . *28 Computing the extimated survivor functions for each conflict type* . *First, note that lambda=exp(-beta'x). We can compute lambda for* . *each confict type:* . gen lambda_civil=exp(-(_b[_cons]+_b[civil])) . gen lambda_interstate=exp(-(_b[_cons]+_b[interst]))</p><p>. gen lambda_icw=exp(-(_b[_cons]))</p><p>. . *29 Second, note that S(t)=exp^-(lambda*t)^p. We can generate the* . *survivor functions for each covariate profile:* . gen surv_civil=exp(-(lambda_civil*duration)^e(aux_p)) (4 missing values generated)</p><p>. gen surv_interstate=exp(-(lambda_interstate*duration)^e(aux_p)) (4 missing values generated)</p><p>. gen surv_icw=exp(-(lambda_icw*duration)^e(aux_p)) (4 missing values generated)</p><p>. . *30 Now we can graph the survivor functions* . gr surv_civil surv_interstate surv_icw duration, c(sss) s(iii) ylab xlab t1("Survivor Function") b2("Durati > on of U.N. Peacekeeping Missions") saving(UNweibsurv, replace) </p><p>. . *31 Note we can also use predict to generate the survivor probability for each observation:* . predict survival_time, surv (4 missing values generated)</p><p>. . drop lambda_* surv_* survival_time</p><p>. . *32 Estimating Gompertz Model* . . streg civil interst, dist(gompertz) nohr</p><p> failure _d: failed analysis time _t: duration</p><p>Fitting constant-only model:</p><p>Iteration 0: log likelihood = -103.03818 Iteration 1: log likelihood = -88.564878 Iteration 2: log likelihood = -80.451626 Iteration 3: log likelihood = -79.833846 Iteration 4: log likelihood = -79.827491 Iteration 5: log likelihood = -79.827491 </p><p>Fitting full model:</p><p>Iteration 0: log likelihood = -79.827491 Iteration 1: log likelihood = -76.219033 Iteration 2: log likelihood = -75.749534 Iteration 3: log likelihood = -75.749466 Iteration 4: log likelihood = -75.749466 </p><p>Gompertz regression -- log relative-hazard form </p><p>No. of subjects = 54 Number of obs = 54 No. of failures = 39 Time at risk = 3994 LR chi2(2) = 8.16 Log likelihood = -75.749466 Prob > chi2 = 0.0169</p><p>------_t | Coef. Std. Err. z P>|z| [95% Conf. Interval] ------+------civil | .630388 .3680023 1.71 0.087 -.0908832 1.351659 interst | -.8770961 .5050953 -1.74 0.082 -1.867065 .1128726 _cons | -3.640878 .252668 -14.41 0.000 -4.136099 -3.145658 ------+------gamma | -.0134251 .0044672 -3.01 0.003 -.0221806 -.0046696 ------</p><p>. . *33 We could use predict to generate the hazard rates for each conflict type* . . predict haz_civil, haz, if civil==1 (44 missing values generated)</p><p>. predict haz_icw, haz, if icw==1 (28 missing values generated)</p><p>. predict haz_interst, haz, if interst==1 (48 missing values generated)</p><p>. . *34 And then graph them* . . gr haz_civil haz_icw haz_interst duration, s(iii) c(sss) ylab xlab t1("Hazard Rates")b2("Duration of U.N. P > eacekeeping Missions") </p><p>. . drop haz_*</p><p>. . *Or we can generate them ourselves using the formula for the Gompertz h(t)* . . *35 First we create lambda, noting that lambda=xb* . gen lambda_civil=_b[_cons]+_b[civil]</p><p>. gen lambda_interstate=_b[_cons]+_b[interst]</p><p>. gen lambda_icw=_b[_cons]</p><p>. . *36 Second, we create h(t):* . gen haz_civil=exp(e(gamma)*duration)*exp(lambda_civil) (4 missing values generated)</p><p>. gen haz_interst=exp(e(gamma)*duration)*exp(lambda_interst) (4 missing values generated)</p><p>. gen haz_icw=exp(e(gamma)*duration)*exp(lambda_icw) (4 missing values generated)</p><p>. . gr haz_civil haz_icw haz_interst duration, s(iii) c(sss) ylab xlab t1("Hazard Rates")b2("Duration of U.N. P > eacekeeping Missions") saving(UNgomphaz, replace) </p><p>. . drop haz_* . . *37 We can compute the survivor function for the Gompertz model* . . gen surv_civil=exp((-(exp(lambda_civil))/e(gamma))*(exp(e(gamma)*duration)-1)) (4 missing values generated)</p><p>. gen surv_interst=exp((- (exp(lambda_interst))/e(gamma))*(exp(e(gamma)*duration)-1)) (4 missing values generated)</p><p>. gen surv_icw=exp((-(exp(lambda_icw))/e(gamma))*(exp(e(gamma)*duration)-1)) (4 missing values generated)</p><p>. . *38 Now we graph them:* . gr surv_civil surv_interst surv_icw duration, c(sss) s(iii) ylab xlab t1("Survivor Function") b2("Duration > of U.N. Peacekeeping Missions") saving(UNgompsurv, replace) </p><p>. . drop surv_* lambda_*</p><p>. . *++++++++++++++++++++++++++++++++++++++++++++++++++++++++++++++++++++++++++++ ++++++++++++* . . *Cox Model of U.N. Peacekeeping Missions* . . *39 Estimating Cox PH model using nohr option* . . stcox civil interst, nohr </p><p> failure _d: failed analysis time _t: duration</p><p>Iteration 0: log likelihood = -131.62249 Iteration 1: log likelihood = -127.43384 Iteration 2: log likelihood = -127.15768 Iteration 3: log likelihood = -127.15763 Refining estimates: Iteration 0: log likelihood = -127.15763</p><p>Cox regression -- Breslow method for ties</p><p>No. of subjects = 54 Number of obs = 54 No. of failures = 39 Time at risk = 3994 LR chi2(2) = 8.93 Log likelihood = -127.15763 Prob > chi2 = 0.0115</p><p>------_t | _d | Coef. Std. Err. z P>|z| [95% Conf. Interval] ------+------civil | .7348046 .3781278 1.94 0.052 -.0063122 1.475921 interst | -.8556111 .5042314 -1.70 0.090 -1.843886 .1326643 ------</p><p>. . *40 This gives coefficients estimates and not hazard ratios. To see the hazard ratios, we can redisplay the > results by typing:* . . stcox, hr</p><p>Cox regression -- Breslow method for ties</p><p>No. of subjects = 54 Number of obs = 54 No. of failures = 39 Time at risk = 3994 LR chi2(2) = 8.93 Log likelihood = -127.15763 Prob > chi2 = 0.0115</p><p>------_t | _d | Haz. Ratio Std. Err. z P>|z| [95% Conf. Interval] ------+------civil | 2.085075 .7884246 1.94 0.052 .9937077 4.375065 interst | .4250234 .2143101 -1.70 0.090 .1582014 1.141867 ------</p><p>. . *The results from the first model are the log of the estimates from this model* . . * Retreiving Baseline Functions* . . *41 Estimate Cox Model* . . stcox civil interst, nohr basech(inthaz) basesurv(surv) basehc(haz)</p><p> failure _d: failed analysis time _t: duration</p><p>Iteration 0: log likelihood = -131.62249 Iteration 1: log likelihood = -127.43384 Iteration 2: log likelihood = -127.15768 Iteration 3: log likelihood = -127.15763 Refining estimates: Iteration 0: log likelihood = -127.15763</p><p>Cox regression -- Breslow method for ties</p><p>No. of subjects = 54 Number of obs = 54 No. of failures = 39 Time at risk = 3994 LR chi2(2) = 8.93 Log likelihood = -127.15763 Prob > chi2 = 0.0115</p><p>------_t | _d | Coef. Std. Err. z P>|z| [95% Conf. Interval] ------+------civil | .7348046 .3781278 1.94 0.052 -.0063122 1.475921 interst | -.8556111 .5042314 -1.70 0.090 -1.843886 .1326643 ------</p><p>. . *42 Now obtain hazard ratios:* . . predict hr, hr</p><p>. . *which is exp(xb)* . . *43 The last three commands produce the estimates of the baseline functions,* . *which we can graph:* . . gr surv durat, ylab xlab c(J) t1("Baseline Survivor Function") b2("Duration of U.N. Peacekeeping Missions") > s(ii) saving(UNcoxst, replace)</p><p>. . *44 To compute the survivor function for each observation we generate new variable:* . . gen surv_i=surv^hr (4 missing values generated)</p><p>. . *45 From this, we can compute the conflict-specific survivor function:* . . gen surv_civil=surv_i if civil==1 (44 missing values generated)</p><p>. gen surv_icw=surv_i if icw==1 (28 missing values generated)</p><p>. gen surv_ic=surv_i if interst==1 (48 missing values generated)</p><p>. . *46 Now we can graph them* . . gr surv_civil surv_icw surv_ic durat, c(JJJ) s(iii) ylab xlab t1("Survivor Functions") b2("Duration of U.N. > Peacekeeping Missions") saving(UNcoxst2, replace)</p><p>. . drop surv_civil surv_icw surv_ic surv_i hr haz inthaz surv</p><p>. log close</p>
Details
-
File Typepdf
-
Upload Time-
-
Content LanguagesEnglish
-
Upload UserAnonymous/Not logged-in
-
File Pages13 Page
-
File Size-