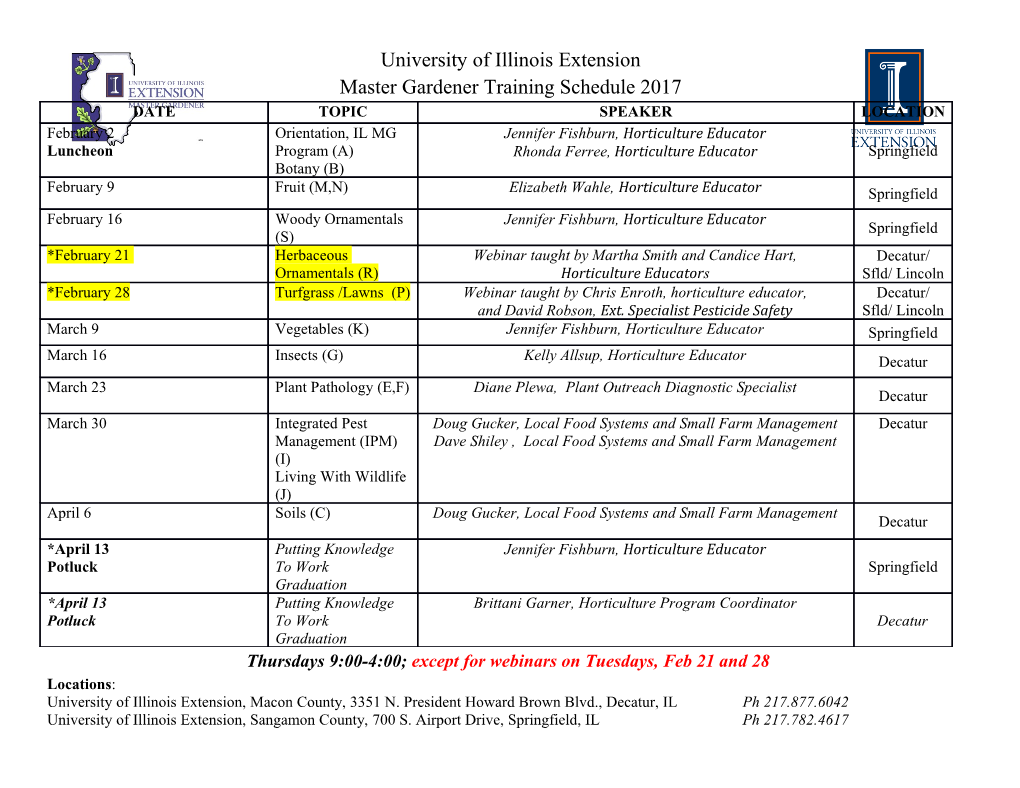
Noname manuscript No. (will be inserted by the editor) Knowledge Distillation: A Survey Jianping Gou1 · Baosheng Yu 1 · Stephen J. Maybank 2 · Dacheng Tao1 Received: date / Accepted: date Abstract In recent years, deep neural networks have Keywords Deep neural networks · Model been successful in both industry and academia, es- compression · Knowledge distillation · Knowledge pecially for computer vision tasks. The great success transfer · Teacher-student architecture. of deep learning is mainly due to its scalability to encode large-scale data and to maneuver billions of model parameters. However, it is a challenge to deploy these cumbersome deep models on devices with limited 1 Introduction resources, e.g., mobile phones and embedded devices, not only because of the high computational complex- During the last few years, deep learning has been ity but also the large storage requirements. To this the basis of many successes in artificial intelligence, end, a variety of model compression and acceleration including a variety of applications in computer vi- techniques have been developed. As a representative sion (Krizhevsky et al., 2012), reinforcement learning type of model compression and acceleration, knowledge (Silver et al., 2016; Ashok et al., 2018; Lai et al., 2020), distillation effectively learns a small student model from and natural language processing (Devlin et al., 2019). a large teacher model. It has received rapid increas- With the help of many recent techniques, including ing attention from the community. This paper pro- residual connections (He et al., 2016, 2020b) and batch vides a comprehensive survey of knowledge distillation normalization (Ioffe and Szegedy, 2015), it is easy to from the perspectives of knowledge categories, train- train very deep models with thousands of layers on ing schemes, teacher-student architecture, distillation powerful GPU or TPU clusters. For example, it takes algorithms, performance comparison and applications. less than ten minutes to train a ResNet model on a Furthermore, challenges in knowledge distillation are popular image recognition benchmark with millions of briefly reviewed and comments on future research are images (Deng et al., 2009; Sun et al., 2019); It takes no arXiv:2006.05525v7 [cs.LG] 20 May 2021 discussed and forwarded. more than one and a half hours to train a powerful BERT model for language understanding (Devlin et al., 2019; You et al., 2019). The large-scale deep models Jianping Gou E-mail: [email protected] have achieved overwhelming successes, however the Baosheng Yu huge computational complexity and massive storage E-mail: [email protected] requirements make it a great challenge to deploy them Stephen J. Maybank in real-time applications, especially on devices with E-mail: [email protected] Dacheng Tao limited resources, such as video surveillance and au- E-mail: [email protected] tonomous driving cars. 1 UBTECH Sydney AI Centre, School of Computer Science, To develop efficient deep models, recent works usu- Faculty of Engineering, The University of Sydney, Darlington, NSW 2008, Australia. ally focus on 1) efficient building blocks for deep models, 2 Department of Computer Science and Information Systems, including depthwise separable convolution, as in Mo- Birkbeck College, University of London, UK. bileNets (Howard et al., 2017; Sandler et al., 2018) and ShuffleNets (Zhang et al., 2018a; Ma et al., 2018); and 2 Jianping Gou1 et al. Teacher Model Knowledge Transfer Student Model Knowledge K n o Transfer Distill w l e d g e Data Fig. 1 The generic teacher-student framework for knowledge distillation. 2) model compression and acceleration techniques, in due to the limited computational capacity and memory the following categories (Cheng et al., 2018). of the devices. To address this issue, Bucilua et al. • Parameter pruning and sharing: These methods fo- (2006) first proposed model compression to transfer cus on removing inessential parameters from deep the information from a large model or an ensem- neural networks without any significant effect on the ble of models into training a small model without a performance. This category is further divided into significant drop in accuracy. The knowledge transfer model quantization (Wu et al., 2016), model bina- between a fully-supervised teacher model and a stu- rization (Courbariaux et al., 2015), structural matri- dent model using the unlabeled data is also intro- ces (Sindhwani et al., 2015) and parameter sharing duced for semi-supervised learning (Urner et al., 2011). (Han et al., 2015; Wang et al., 2019f). The learning of a small model from a large model • Low-rank factorization: These methods identify re- is later formally popularized as knowledge distilla- dundant parameters of deep neural networks by em- tion (Hinton et al., 2015). In knowledge distillation, a ploying the matrix and tensor decomposition (Yu et al., small student model is generally supervised by a large 2017; Denton et al., 2014). teacher model (Bucilua et al., 2006; Ba and Caruana, • Transferred compact convolutional filters: These meth- 2014; Hinton et al., 2015; Urban et al., 2017). The main ods remove inessential parameters by transferring idea is that the student model mimics the teacher model or compressing the convolutional filters (Zhai et al., in order to obtain a competitive or even a superior 2016). performance. The key problem is how to transfer the • Knowledge distillation (KD): These methods distill knowledge from a large teacher model to a small student the knowledge from a larger deep neural network into model. Basically, a knowledge distillation system is a small network (Hinton et al., 2015). composed of three key components: knowledge, dis- tillation algorithm, and teacher-student architecture. A comprehensive review on model compression and A general teacher-student framework for knowledge acceleration is outside the scope of this paper. The distillation is shown in Fig. 1. focus of this paper is knowledge distillation, which has received increasing attention from the research Although the great success in practice, there are not community in recent years. Large deep neural networks too many works on either the theoretical or empirical have achieved remarkable success with good perfor- understanding of knowledge distillation (Urner et al., mance, especially in the real-world scenarios with large- 2011; Cheng et al., 2020; Phuong and Lampert, 2019a; scale data, because the over parameterization improves Cho and Hariharan, 2019). Specifically, Urner et al. (2011) the generalization performance when new data is con- proved that the knowledge transfer from a teacher sidered (Zhang et al., 2018; Brutzkus and Globerson, model to a student model using unlabeled data is 2019; Allen-Zhu et al., 2019; Arora et al., 2018; Tu et al., PAC learnable. To understand the working mecha- 2020). However, the deployment of deep models in mo- nisms of knowledge distillation, Phuong & Lampert bile devices and embedded systems is a great challenge, obtained a theoretical justification for a generalization Knowledge Distillation: A Survey 3 Sec. 2.1 KD Algorithms Performance Comparison Response-Based Knowledge Sec. 5.1 Sec. 5.2 Sec. 5.3 Multi-Teacher Adversarial KD Cross-Modal KD Sec. 2.2 Sec. 6 KD Feature-Based Knowledge Sec. 5.4 Sec. 5.5 Sec. 5.6 Knowledge Distillation Sec. 5 Graph-Based Attention-Based Sec. 2 Data-Free KD Sec. 2.3 KD KD Relation-Based Knowledge Knowledge Sec. 5.7 Sec. 5.8 Sec. 5.9 Quantized KD Lifelong KD NAS-Based KD Sec. 4 Sec. 3.1 Teacher-Student Offline Distillation Architecture KD Applications Sec. 3 Sec. 3.2 Sec. 7 Sec. 7.1 Sec. 7.2 Natural Language Online Distillation Distillation Visual Recognition Processing Sec. 3.3 Sec. 7.3 Sec. 7.4 Sec. 8 Self-Distillation Speech Recognition Other Applications Sec. 8.1 Sec. 8.2 Challenges Directions Fig. 2 The schematic structure of knowledge distillation and the relationship between the adjacent sections. The body of this survey mainly contains the fundamentals of knowledge distillation, knowledge types, distillation schemes, teacher-student architecture, distillation algorithms, performance comparison, applications, discussions, challenges, and future directions. Note that ‘Section’ is abbreviated as ‘Sec.’ in this figure. bound with fast convergence of learning distilled stu- by this, recent knowledge distillation methods have dent networks in the scenario of deep linear classi- extended to teacher-student learning (Hinton et al., fiers (Phuong and Lampert, 2019a). This justification 2015), mutual learning (Zhang et al., 2018b), assis- answers what and how fast the student learns and tant teaching (Mirzadeh et al., 2020), lifelong learn- reveals the factors of determining the success of dis- ing (Zhai et al., 2019), and self-learning (Yuan et al., tillation. Successful distillation relies on data geometry, 2020). Most of the extensions of knowledge distilla- optimization bias of distillation objective and strong tion concentrate on compressing deep neural networks. monotonicity of the student classifier. Cheng et al. The resulting lightweight student networks can be quantified the extraction of visual concepts from the easily deployed in applications such as visual recog- intermediate layers of a deep neural network, to explain nition, speech recognition, and natural language pro- knowledge distillation (Cheng et al., 2020). Ji & Zhu cessing (NLP). Furthermore, the knowledge transfer theoretically explained knowledge distillation on a wide from one model to another in knowledge distillation neural network from the respective of risk bound, data can be extended to other tasks, such as adversar- efficiency and imperfect teacher (Ji and Zhu., 2020). ial attacks (Papernot et al., 2016), data augmenta- Cho & Hariharan empirically analyzed in detail the tion (Lee et al., 2019a; Gordon and Duh, 2019), data efficacy of knowledge distillation (Cho and Hariharan, privacy and security (Wang et al., 2019a). Motivated 2019). Empirical results show that a larger model may by knowledge distillation for model compression, the not be a better teacher because of model capacity gap idea of knowledge transfer has been further applied in (Mirzadeh et al., 2020).
Details
-
File Typepdf
-
Upload Time-
-
Content LanguagesEnglish
-
Upload UserAnonymous/Not logged-in
-
File Pages36 Page
-
File Size-