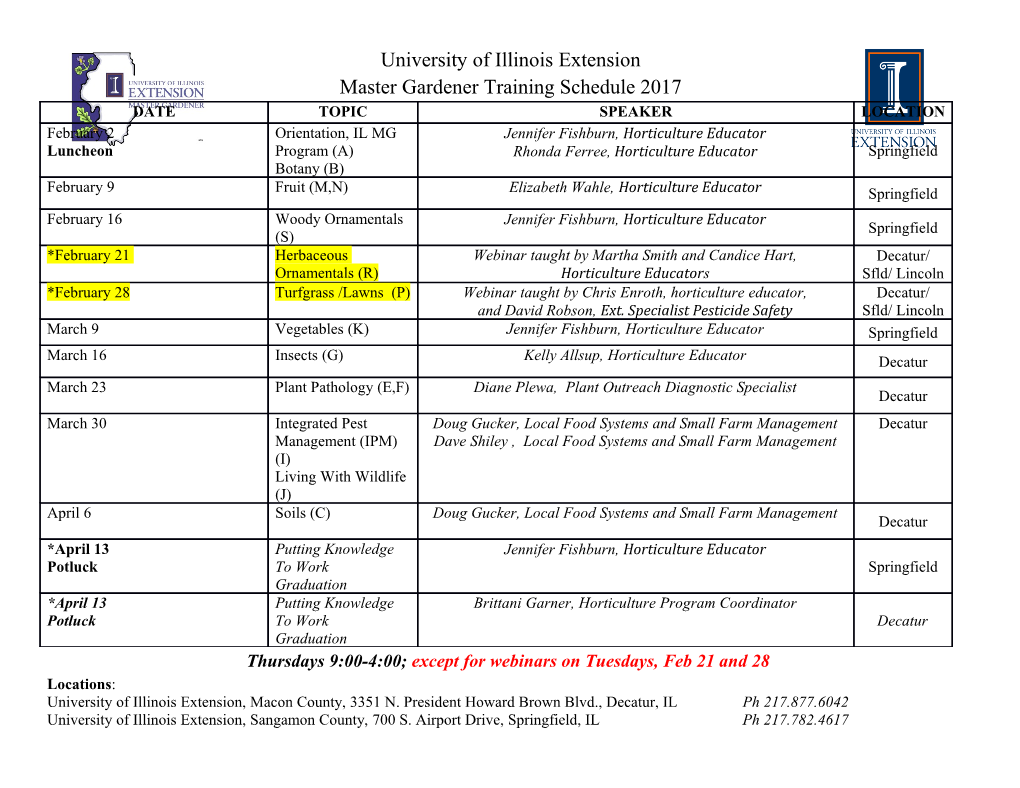
<p> The Standardized ILO October Inquiry 1983-2003</p><p>Remco H. Oostendorp Free University Amsterdam Tinbergen Institute Amsterdam Institute for International development</p><p> [email protected] September 12, 2005 Introduction</p><p>This document describes the standardization procedure for the 1983-2003 ILO October Inquiry data. This procedure is an improved version of the procedure that has been applied to the 1983-1998 ILO October Inquiry as described in Freeman and Oostendorp (2000). The main improvements are twofold, namely an improved cleaning procedure and the use of country-specific data type correction factors. First we discuss the main characteristics of the 1983-2003 ILO October Inquiry database, and next we give a detailed account of each of the steps of the standardization procedure.</p><p>The 1983-2003 ILO October Inquiry data</p><p>Table 1 reports the number of countries that report pay data for each year and all years cumulatively for at least one of the 161 occupations.1 The number of countries that report pay data for at least one occupation varies between 42 and 76 countries in the years 1983-2002. The number of countries reporting for 2003 is lower at 26 because the data are preliminary with some countries expected to report later. Nevertheless the average number of countries reporting for the periods 1983-1989, 1990-1999, and 2000-2002 is respectively 67.7, 65.8 and 47.0 – in other words, reporting compliance appears to be falling.</p><p>Table 1. Number of countries reporting in the 1983-2003 ILO October Inquiry Number of Countries Reporting Year In Given Year Cumulative Reporting 1983 58 58 1984 60 75 1985 71 96 1986 67 105 1987 76 115 1988 73 121 1989 69 124 1990 75 129 1991 69 130 1992 61 132 1993 67 140 1994 62 145 1995 76 151 1996 65 157 1997 67 158 1998 56 158 1999 60 158 2000 56 159 2001 42 160 2002 43 163 2003 26 163 Table 2 gives a detailed description of the information in 1983-2003 ILO October Inquiry. It should be noted that the reported numbers are only for observations from sources that the ILO classifies as of acceptable/good or excellent quality (see Freeman and Oostendorp 2001). Also the numbers reflect only those observations that were retained after extensive cleaning (the data cleaning procedure is described below). </p><p>Panel A gives information on the size of the sample. It shows the maximum conceivable number of observations that the Inquiry would contain if each country reported a single wage statistic for each occupation yearly: over 400,000 pieces of data.2 The actual number of observations is smaller, largely because most countries do not report statistics in many years. On average, countries report wages for 8.5 years out of 21 possible years. Even if we ignore 2003, which has preliminary data for only 26 countries, more than half of country year observations are empty. In addition, countries do not report data for every occupation in the years when they do report. The bottom line is that there are 90,772 country x year x occupation cells with wage data in the 1983-2003 file.</p><p>However, many countries report more than one wage for a single occupation. Some give hourly wage rates and average earnings. Others give wages for men and wages for women. Others give wages for one gender and for both genders. Nearly half of the observations (47.4%) contain multiple wage figures. While this will help us to calibrate the data into a standardized format, it makes the raw data difficult to use in cross-country comparisons, particularly since different countries report pay differently. Including multiple wages, there are 157,021 pieces of data.</p><p>Panel B shows the frequency distribution of countries by the number of occupations they report; and the frequency distribution of occupations by the number of countries that report statistics on them. The distribution of countries by number of occupations shows that in most countries there are sufficient occupations with wage data to get a good measure of the overall wage structure. It also shows, however, that different countries report on different numbers of occupations, which creates problems in comparing wage structures across countries. The distribution of occupations by country shows that many occupations have wage data for large numbers of countries, which will allow us to contrast labor costs and living standards for workers in the same occupation around the world.</p><p>Panel C shows the diverse way in which countries report wages. Most countries report wage rates, presumably from employer surveys or collective bargaining contracts or Table 2. Types of observations contained in the October Inquiry computer files, 1983-2003 Number A. SAMPLE SIZE Maximum conceivable observations 463,197 Observations missing because country did not report in given year 275,954 Observations missing because occupation missing in year country reported 96,471</p><p>Actual year/country/occupation observation 90,772 Observations with multiple figures 42,988 Multiple figures 66,249 Total, including all multiple observations 157,021</p><p>B. COUNTRIES AND OCCUPATIONS WITH AT LEAST ONE REPORTED WAGE STATISTIC Countries with reported wage statistic for different numbers of occupations No. of occupations No. of countries (totaling 137) <30 7 30-59 16 60-79 15 80-99 13 100-119 30 120-139 22 140+ 34 Occupations with one reported wage statistic for different numbers of countries No. of countries reporting on occupations No. of occupations (totaling 161) <59 18 60-79 35 80-99 45 100-119 52 120+ 11</p><p>C. ACTUAL OBSERVATIONS Pay concept Wage rates (142 countries) 99,050 Earnings (95 countries) 57,971 Averaging concept Mean 110298 Minimum 33012 Maximum 5300 Average of min-max 192 Prevailing 4654 Median 2961 Other 15 Missing 589 Period concept Monthly 102202 Hourly1 27756 Weekly 15462 Daily 8386 Annual 2737 Fortnight 457 Other 20 Missing 1 Sex Male workers 63,434 Male and female workers 59,354 Female workers 34,233 Coverage Whole country 131,872 Part of country 25,149 Source: Tabulated from ILO October Inquiry computer files, 1983-2003. The total number of countries is 137 (compare with the 163 countries reporting in table 1) because the reported numbers are only for observations from sources that the ILO classifies as of acceptable/good or excellent quality (see Freeman and Oostendorp 2001). Note. The hourly figures include a small number of observations which concern hours paid for, and another small number which concern wages relating to hours worked. legislated pay schedules. However, many report earnings, which may come from household surveys. Most give statistics in the form of averages3 but 21 percent report minimum wages, some from collective bargaining contracts. Some countries report maximum wages. Others give prevailing wages. The US reports median weekly earnings for most occupations (from individual reports on the Current Population Survey). The time period to which the pay refers also varies. The most common period is the month, followed by the hour, but some countries report weekly pay, others give daily rates for some occupations, and so on. There is also variation by gender. Forty percent of the observations relate to male workers, 38 percent to all workers, and 22 percent to female workers. </p><p>Combining all of these different variants, the vast majority of the Inquiry statistics are non-comparable. Just 7.8 percent relate simultaneously to the most common pay concept (wages), use the most common averaging (mean), cover the most common time span (monthly), relate to the most common gender (male) and cover the whole country.4 </p><p>Standardization procedure for the 1983-2003 ILO October Inquiry data</p><p>Because of the nonstandard nature of the database we use a standardization procedure to make the data comparable across occupations, countries and time. This procedure is an improved version of the standardization procedure as developed and described by Freeman and Oostendorp (2000, 2001), with an improved cleaning procedure and the application of country-specific data type correction factors. The remainder of this section provides a detailed account of each of steps in the standardization procedure.</p><p>Step 1. Data cleaning</p><p>Data cleaning was done through the inspection of data plots, with wages (in local currency units) on the vertical axis and year of reporting on the horizontal axis for each country x occupation pair.5 Although we cleaned all data, here we describe the outcome of the cleaning procedure only for observations from sources that the ILO classifies as of acceptable/good or excellent quality. The following figure shows one example of a plot for the occupation “Quarryman” for Belgium. </p><p>The plot clearly shows that the reported wage observation for 1985 is an outlier. Further inspection of the raw data showed that this problem was not caused by variation in the averaging concept (e.g. maximum wage reported instead of minimum wage), (obvious) miscoding in the period concept (e.g. annual wages reported instead of monthly wages), gender wage differences, or differences in location (i.e. regions within the country) from which wages were reported. In this case it was therefore decided to drop the above outlier in order to preserve the obvious time pattern in the data.</p><p> y1=BE y4=19</p><p>71603.6</p><p>39723.4 1983 1985 1987 1989 1991 1993 1995 1997 1999 2001 2003 y0</p><p>The following figure shows a similar plot for the occupation “Deep-sea fisherman” for Suriname:</p><p> y1=SR y4=9</p><p>3683.33</p><p>450 1983 1985 1987 1989 1991 1993 1995 1997 1999 2001 2003 y0</p><p>Because wages were reported with different averaging units, minimum wages were plotted by squares, maximum wages by pluses, and average wages by a triangle. The plot clearly shows that the reported wages for 1990 are outliers, and further inspection of the raw data showed that this was due to an obvious miscoding of the period concept for 1990 (monthly wages were reported instead of weekly wages). Therefore the period concept was recoded to monthly wages for 1990 and the obvious time pattern was restored.</p><p>In total 14,402 country x occupation pairs were inspected. For a few country x occupation pairs it was obvious that there was no logical time pattern in the reported data and all the observations of the country x occupation pair were deleted from the database. The following plot for “Motor bus driver” for Puerto Rico illustrates this case. Here only minimum wages are reported for the period 1985-1995, but both minimum wages (marked by squares) and maximum wages (marked by pluses) are reported for 1996-2002. However, there is no logical time pattern in the reported minimum wage, although one may speculate that the reported wages for the period 1985-1995 are actually maximum wages. This is not obvious, however, and it was therefore decided to drop all the observations from this country x occupation pair.</p><p> y1=PR y4=111</p><p>1875.47</p><p>911.733 1983 1985 1987 1989 1991 1993 1995 1997 1999 2001 2003 y0</p><p>After inspecting wages across years for each country x occupation pair, two further data checks were performed. First, wages were inspected across occupations for each country x year pair. In case occupational wages were a tenfold smaller or a tenfold larger than the average occupational wage within a country x year pair, then this wage observation was further checked and corrected if necessary. Second, the average wage within a country x year pair was compared to GDP per capita for that country and year. In case the ratio of the average wage and GDP per capita was very low or high (in the lower or upper 1% of the distribution) , then these wage observations were further checked and corrected if necessary. In total 1082 wage observations were removed and 6210 wage observations were recoded, or 0.69% and 3.95% of the total respectively. Hence in total 4.64% of all wage observations were affected by the cleaning procedure.</p><p>Step 2. Data construction </p><p>Before estimating the data type correction factors (see Freeman and Oostendorp 2000, 2001 for a discussion of the methodology for estimating data correction factors), a number of steps were taken to standardize the data as much as possible. </p><p>Recoding of footnotes</p><p>Many wage observations are reported with footnotes, such as “Average per hour”, “Auckland”, “Both sexes" or “Large hotels”. Each of these footnotes has been coded as much as possible, using the variables as listed in the following table. The part of the footnote that could not be coded within y0-y10 was retained within the string variable ftn.</p><p>Table 3. Variables in raw data set Name Description y0 year y1 country code y2 city or region code y3 industry code y4 occupation code y6 pay or hours of work concept code y7 sex code y8 range code y9 period concept code y10 averaging concept code ftn footnote x data</p><p>Range data</p><p>In a few cases the wages are in the form of ranges. We found the midpoint of the range and use it as the wage for the category.</p><p>Minimum-maximum data</p><p>In case a pair of wages have been reported as ‘minimum-maximum wage’, the smallest has been recoded as ‘minimum wage’ and the largest as ‘maximum wage’. Construction of monthly data</p><p>The wage observations have been recalculated on a monthly basis as much as possible, using dimensional analysis6 and the reported hours of work. The number of hours was not always reported for the given occupation (y4), pay concept (y6), sex (y7), year (y0) and city/region (y2). In this case the next best alternative hours of work was assigned. The following table reports the different hours of work data that have been used successively in lexicographic order.</p><p>Table 4. Lexicographic assignment of hours of work Lexicographic Hours of work assigned from order 1 same occupation (y4), pay concept (y6), sex (y7), year (y0) and city/region (y2)</p><p>2 other sex (y7) for given occupation (y4), pay concept (y6), year (y0) and city/region (y2) 3 other city/region (y2) for given occupation (y4), pay concept (y6), sex (y7) and year (y0) 4 other year (closest) (y0) for given occupation (y4), pay concept (y6), sex (y7), year (y0) and city/region (y2) 5 other pay concept (y6) for given occupation (y4), sex (y7), year (y0) and city/region (y2) 6 other occupation (average) (y4) for given pay concept (y6), sex (y7), year (y0) and city/region (y2)</p><p>The above lexicographic ordering has been chosen because the variation in hours of work can be attributed in increasing order of magnitude to variation in sex (y7), city/region (y2), year (y0), pay concept (y6) and occupation (y4). In the few remaining cases where the above lexicographic assignment did not yield an estimate of hours of work, the country- average or, if not available, the world-average of hours of work was used. </p><p>Removing idiosyncratic data types</p><p>A number of wage observations were removed from the data set because their exact data type was unspecified or too idiosyncratic. For the period concept, wage observations with a missing or ‘other’ period concept (such as per shift, per piece) were dropped. For the averaging concept, wage observations with missing or ‘other’ (unspecified) averaging concept were also dropped. We also removed the wage observations that were reported as the average of minimum and maximum wages because there are only few of them (see table 2) and it is not a common averaging concept. We only included wage observations with the median averaging concept for the US in the data set, because most of the reported median wages are from the US (about 75%) and inclusion of median wages for the other countries led to an implausible estimate of the data correction factor for median wages.7</p><p>As a result of the above data construction steps, we have two pay concepts (62.30% wage rates, 37.70% earnings), five averaging concepts (71.71% mean, 20.04% minimum, 3.19% maximum, 3.12% prevailing, 1.94% median), 2 period concepts (94.73% monthly, 5.27% daily), and 3 sex concepts (41.73% male, 36.38% male and female, 21.90% female). In the following section we discuss the procedure to standardize these data with these different concepts.</p><p>Step 3. Estimation of data type correction factors</p><p>The next step is to estimate data correction factors for the 1983-2003 ILO October Inquiry data following the procedure discussed in Freeman and Oostendorp (2000). Note first that because data concepts can occur in combination with each other, this gives potentially 60 data correction factors: 2 types of pay concepts, 5 types of averaging concepts, 2 types of period concepts, and 3 types of sex concepts (2 x 5 x 2 x 3 = 60). Also the impact on wages of each of these (combinations of) data concepts could vary across countries (and even across regions within countries), occupations, and years. Hence, there are a large number of potential correction factors that need to be estimated.</p><p>This problem of heterogeneity of the data correction factors was discussed in Freeman and Oostendorp (2000). It was noted that the variation in the October Inquiry is too ‘thin’ to estimate all potential data correction factors for all data types and that it is necessary to simplify the procedure. Also here we will assume that the different data types affect wages separately rather than interactively (reducing the number of combinations of data concepts from 60 to 12). Also we will not estimate data correction factors that vary across time or occupation, assuming for instance that the gender wage gap is constant across time and occupations within a country.8 The reason we do make these simplifying assumptions is that we think that the largest source of variation in the data correction factors can be found across countries rather than across time or occupations.</p><p>In Freeman and Oostendorp (2000, 2001) data correction factors were estimated that varied by region or income rank of the country but not by country. Here we go one step further and estimate country-specific data correction factors as much as possible. Because data correction factors turn out to be highly variable across countries, the proposed standardization procedure can be seen as an important refinement of the earlier procedures. An additional refinement is that we also use country-specific occupational dummies to estimate the standard wage and hence we no longer assume that the occupational wage structure or ranking is similar across countries.</p><p>A number of issues need to be addressed when estimating country-specific data correction factors. First, some or none of the data correction factors can be estimated because they are not identified for lack of variation in the data at the country level. If wages in one country are only reported as minimum wages, then it will not be possible to estimate the average wage in this country. Or if average wages are only reported for female workers, and prevailing wages for male workers, then it is not possible to identify the data correction factors for the averaging and sex concept. Second, there might be variation in the data but some of the data types have been sparsely reported. For instance in some countries wages are mostly reported as minimum wages and only in a few instances as average wages. Third, the estimated data correction factor may be implausible. If wages have been reported as daily wages in some instances, and if the estimated data correction factor for the period concept implies that daily wages are forty times less than monthly wages, then this is implausible as the maximum number of working days per month is 31 at most.9</p><p>Taking these issues into account, we therefore distinguish between three types of standard(ized) wages. First, there are wages that are reported in a standard format and that do not need to be standardized. The standard format is here defined as average monthly wage rates for male workers in the whole country because relatively most wages are reported in this format. Second, there are wages that are reported at least partly in non-standard format and for which plausible data country-specific correction factors can be identified on non-sparse data types. The definition of non-sparse data types is arbitrary and we have chosen as cut-off point at least 10 wage observations of the given data type. Third, there are wages that are reported at least partly in non-standard format for which no plausible country-specific estimates on non-sparse data types for all correction factors can be identified. In this case we substitute the estimated data correction factors for all countries for the correction factors that could not be estimated plausibly on non-sparse data for a given country. </p><p>We also distinguish a fourth type of standard(ized) wage namely wages which are corrected using the estimated data correction factors for all countries (hence not country- specific). This type of standardized wages corresponds to the standardization variant 2 in Freeman and Oostendorp (2000, table A.1) and has commonly been used in analyses so far. Hence we include this type of standard(ized) data for comparison.</p><p>It should be noted that for each of the second, third and fourth type of standardized data there is an issue of how to treat multiple wage observations within a given country, occupation, and year. Countries often report wages in different format (for instance male and female wages or for different regions) and therefore we have often multiple estimated standard wages for a given country, occupation and year. Following Freeman and Oostendorp (2000), we use two types of weighting schemes. First, we use uniform weighting which gives an equal weight of the reciprocal of the number of wage observations reported within a country, occupation and year. Second, we use lexicographic weighting, which gives weight equal to one to the wage observation that is reported in standard format and zero to others. If no standard wage is reported, then uniform weights are assigned. It can be shown that lexicographic weighting is most efficient if there is much uncertainty in the data type correction terms and uniform weighting is most efficient if there is much measurement error in the reported wage data.</p><p>The following table summarizes the different standardized data that we have calculated.</p><p>Table 5. Sources of data for different types of standardized data type 1 type 2 type 3 type 4 data reported in standard yes yes yes yes format data corrected with country- no yes yes no specific correction factors data corrected with average no no yes yes correction factors</p><p>Table A.1 in appendix A reports the estimated data correcting factors for the different countries. Naturally no correction factor was estimated for data types that were not reported (indicated by a dot). Correction factors in italics are average data correction factors (not country-specific), either because the country-specific correction factor could not be estimated, the data type was sparsely reported, or because the estimate was implausible. </p><p>We have not attempted to estimate data correction factors for the coverage of the wage data to estimate the difference in wages reported for the whole country and for part of the country. The reason for this omission is that regional coverage varies mostly across time, and therefore its impact on wages is mostly unidentified. Also 84% of the data in the 1983- 2003 October Inquiry has been reported for the whole country so the impact of variation in coverage on predicted wages will be limited. However most of the pre-1983 data have been reported for part of the country (often the capital city) and the issue of how to correct for regional coverage will be pertinent when standardizing the pre-1983 October Inquiry data.</p><p>Because the data correction factors were estimated for log wages, they indicate that the reported wages of the corresponding data type deviate from the standard data type by the factor eestimated data correction factor or by (eestimated data correction factor-1)x100%.</p><p>Table A1 shows that for Antigua and Barbuda (y1=AG) it is estimated that earnings are 7 percent higher than wage rates, that females earn 8 percent less than male workers, that workers of both sexes earn 3 percent less than male workers, that minimum wages are 25% less than average wages, that prevailing wages are the same as average wages, and that maximum wages are 34 percent higher than average wages. A country-specific data correction factor for Antigua and Barbuda for daily wages could not be estimated because of lack of variation in the data and therefore the average data correction factor estimated across all countries for daily wages (-3.33 which implies a factor of 27.9) is reported (indicated in italics). </p><p>It is clear that the estimated correction factors vary widely across countries, underlining the need for estimating country-specific correction factors unlike in Freeman and Oostendorp (2000, 2001).</p><p>The standardized data</p><p>Appendix B provides the codes for the variables in the standardized data set. The means, standard deviation, minimum and maximum of the standardized data of the four types is reported in the table 6. The reported numbers are for lexicographic weighting but the numbers are virtually the same if uniform weighting is applied.</p><p>The first type of standardized data consists of only those wage observations that have been reported in the standard format. There are 17766 standard observations in 80 countries and the mean monthly wage rate for male workers is 804 US $. The second type of standardized data also includes the wages that could be corrected with country-specific data</p><p>Table 6. Descriptive statistics of standardized data (lexicographic weighting). Number of Mean wage Standard Minimum Maximum observations (in US $) deviation type 1 17766 804 796 5 9008 type 2 64018 732 1012 6 22648 type 3 90132 819 1033 4 26575 type 4 90132 789 994 5 27724 Note: the mean wages in US $ have been calculated on slightly smaller number of observations because of non-available exchange rates. correction factors. This gives 64018 observations in 101 countries and the average wage per month is 732 US $. The third type also includes wage observations that could only be corrected using average (non-country-specific) data correction factors. This gives 90132 wage observations in 137 countries with a mean wage of 819 US $. The fourth type of standardized data is based on average data correction factors with also 90132 observations but a mean wage of 789 US $.</p><p>The following table gives the pairwise correlations of the four types of standardized wages across all countries / occupations / years. </p><p>Table 7. Pairwise correlations of standardized wages. type 1 type 2 –wu type 3 –wu type 4 –wu type 2 –wl type 3 –wl type 4 –wl type 1 1.0000 type 2 –wu 0.9976 1.0000 type 3 –wu 0.9976 1.0000 1.0000 type 4 –wu 0.9974 0.9998 0.9998 1.0000 type 2 –wl 1.0000 0.9976 0.9976 0.9974 1.0000 type 3 –wl 1.0000 0.9976 0.9976 0.9974 1.0000 1.0000 type 4 –wl 1.0000 0.9976 0.9976 0.9974 1.0000 1.0000 1.0000 Note: wu = uniform weighting, wl = lexicographic weighting.</p><p>The above table shows that the different standardization methods give similar results, with correlations above 0.99. The same result holds for the correlations between wages converted to US$. This is reassuring as a high correlation suggests that the choice of the exact standardization procedure has little effect on the outcome. However, table 6 also shows that the average predicted wages between type 3 and type 4 standardization varies between 789 and 819 US $ per month, or by 3.8%.10 This may be a large or small difference depending on the type of analysis. It is therefore recommended to start any analysis conservatively with the type 1 standardized data and to use the higher types of data (with increasing levels of data imputation) only if the basic results using the lower types of data remain unaffected. </p><p>Table C1 in appendix C summarizes the data for each country and year. The reported wages are expressed in US $ and based on the type 3 standardization. In a few instances we were unable to transform the wages in US $ because of missing exchange rates in the IMF IFS (indicated by *). It should be noted that part of the reason why average wages vary across time is that wages have been reported for different occupations (across and within countries over time). References</p><p>Freeman, R.B. and R.H. Oostendorp (2000): “Wages Around the World: Pay Across Occupations and Countries”, NBER Working Paper no. 8058.</p><p>Freeman, R.B. and R.H. Oostendorp (2001): “The Occupational Wages around the World Data File”, ILO Labour Review, 2001, Fall Issue.</p><p>Oostendorp, R.H (2004): “Globalization and the Gender Wage Gap”, World Bank Policy Research Working Paper 3250, March. Appendix A</p><p>Table A1. Estimated data correction factors Country Earnings Females Both sexes Daily Median Minimum Prevailing Maximum AG 0.07 -0.08 -0.03 -3.33 . -0.29 0.00 0.29 AN 0.09 -0.16 -0.01 . . -0.15 -0.02 . AO . . -0.01 . . -0.63 -0.02 . AR 0.30 . -0.01 -3.29 . -0.14 -0.04 . AS -0.11 -0.11 -0.01 . . -0.15 . 0.29 AT 0.20 -0.04 0.02 . . -0.08 0.06 0.24 AU 0.10 -0.16 -0.14 . . -0.10 . . AZ 0.26 -0.13 0.12 . . . . . BB . 0.01 0.01 -3.16 . -0.17 0.04 0.20 BD 0.70 -0.03 -0.04 -2.88 . -0.15 . . BE . . . . . -0.15 . . BF 0.29 0.02 0.12 . . -0.15 -0.02 0.29 BG . . -0.01 . . . . . BI 0.16 ...... BJ 0.16 0.07 -0.04 -3.33 . -0.09 0.09 0.35 BM 0.11 . -0.01 . . -0.15 -0.02 0.25 BN 0.05 -0.09 -0.08 . . . . . BO 0.18 -0.06 -0.01 . . . -0.23 . BR 0.16 -0.22 -0.04 . . . . . BW 0.16 . -0.01 . . . . . BY 0.47 -0.10 0.04 . . . . . BZ . -0.11 -0.01 -3.33 . -0.38 0.02 0.16 CA 0.16 -0.22 -0.08 . . . . . CF -0.04 -0.16 -0.13 -3.33 . -0.37 -0.12 . CI -0.10 . 0.04 -3.33 . -0.14 . . CL 0.16 . -0.01 . . . . . CM . -0.16 -0.01 . . -0.15 -0.02 . CN . -0.12 ...... CO . -0.23 -0.13 -3.33 . -0.58 -0.17 . CR 0.16 -0.08 -0.03 -2.86 . -0.15 -0.02 . CS 0.16 -0.11 -0.10 . . . . . CU 0.24 -0.05 -0.01 . . -0.08 0.02 . CV . -0.07 -0.01 . . . -0.02 . CY 0.06 -0.23 -0.08 . . . . . CZ 0.16 -0.06 -0.01 . . . . . DE . . -0.01 -3.33 . -0.15 . . DJ . -0.16 -0.01 . . -0.15 -0.02 . DK 0.02 -0.08 -0.01 . . . . . DO . . -0.01 . . -0.15 . . DZ 0.16 -0.16 -0.48 . . -1.13 . . EE -0.05 -0.17 -0.01 . . . -0.02 . ET . . 0.17 . . . -0.02 . FI 0.16 -0.10 0.02 -3.33 . . . . FJ 0.00 . -0.11 . . . . . FK 0.00 -0.01 -0.04 . . -0.05 -0.02 0.18 FR 0.16 -0.16 -0.01 . . . . . GA 0.19 -0.13 -0.04 . . -0.15 -0.02 0.29 GB 0.09 -0.18 0.06 . . -0.15 . . GH 0.32 -0.09 ...... GI 0.01 -0.25 -0.03 . . . . . GP . -0.16 -0.01 . . -0.15 -0.02 . GQ . -0.16 -0.01 . . -0.15 . . GU 0.00 -0.16 -0.01 . . . 0.00 . GY 0.03 -0.03 -0.11 -3.20 . -0.21 0.00 . HK 0.20 -0.10 -0.08 -3.21 . -0.15 . 0.29 HN 0.06 -0.11 -0.07 -3.15 . -0.15 . . HR 0.16 . -0.01 . . . . . HU 0.08 -0.17 -0.01 . . . . . IE 0.16 -0.16 -0.01 . . -0.15 -0.02 . IM 0.10 -0.05 0.13 . . -0.16 0.00 0.10 IN 0.06 -0.16 0.06 -3.30 . -0.39 -0.02 0.32 IR . . -0.01 . . . . . IS 0.31 -0.20 -0.07 . . . . . IT . . -0.01 . . -0.04 . . JP 0.16 -0.41 -0.01 -3.33 . . . . KG 0.19 -0.04 0.01 . . . . . KH . . -0.01 . . -0.15 . . KM 0.37 -0.26 -0.18 -3.33 . -0.15 0.07 1.20 KN . 0.00 0.02 -1.86 . -0.15 -0.20 0.32 KR 0.32 -0.42 ...... KZ . -0.15 -0.06 . . . . . LC 0.00 -0.07 0.02 -3.03 . -0.30 -0.02 0.18 LK 0.16 -0.07 -0.04 . . . . . LR 0.01 -0.16 -0.01 -3.33 . . . . LT 0.04 -0.13 -0.06 . . . . . LU . -0.18 ...... LV 0.16 -0.14 -0.05 . . . . . MD 0.29 -0.05 ...... MG . 0.01 0.06 . . -0.15 . 0.29 ML 0.12 0.05 -0.17 . . -0.05 -0.12 0.29 MM . . 0.03 . . -0.15 0.13 . MN 0.16 -0.16 -0.01 . . . . . MO 0.17 -0.15 -0.01 -3.33 . . . . MU 0.09 -0.12 0.00 -3.33 . -0.29 . 0.22 MW 0.23 -0.05 0.08 . . -0.15 . . MX 0.04 -0.16 -0.01 -3.33 . -0.15 . . MZ . -0.16 . . . -0.15 0.07 . NC 0.16 -0.16 -0.01 . . -0.15 . . NE . -0.16 -0.01 . . -0.15 . 0.29 NG 0.06 -0.13 0.06 -3.33 . -0.19 -0.02 . NI . -0.16 -0.18 . . . . . NL . -0.16 -0.01 . . -0.15 0.00 0.22 NO -0.02 -0.05 -0.08 . . . . . NZ . . -0.01 -3.33 . -0.15 -0.02 0.29 PE 0.10 -0.13 -0.01 -3.33 . -0.05 -0.16 . PF . . -0.19 . . -0.27 . . PG 0.05 0.15 0.02 . . -0.08 -0.02 . PH . -0.16 -0.01 -3.33 . -0.15 . 0.29 PL 0.16 -0.14 ...... PM 0.01 . -0.01 . . -0.02 -0.02 . PR 0.07 -0.06 -0.05 . . -0.13 . 0.10 PT 0.19 -0.11 -0.01 -3.33 . . . . RO 0.03 -0.08 -0.05 . . . -0.02 . RU 0.49 -0.15 -0.12 . . . -0.02 . RW . . -0.01 . . -0.15 . . SC -0.01 -0.01 -0.01 . . -0.04 -0.01 . SD 0.20 . 0.21 . . -0.42 -0.01 0.10 SE 0.06 -0.08 -0.01 . . -0.34 -0.02 0.29 SG . -0.15 -0.06 . . . . . SH . -0.07 -0.01 -3.33 . -0.15 . 0.29 SI 0.16 -0.07 -0.04 . . . . . SK -0.06 -0.16 -0.05 . . . . . SL . -0.01 -0.09 -3.17 . -0.22 0.01 . SM . . -0.01 . . . -0.02 . SN . . -0.01 . . -0.15 . . SR . -0.01 -0.01 -3.33 . -0.41 -0.19 0.23 SY . . . -3.33 . . . . SZ . -0.05 -0.01 -3.33 . -0.15 . . TD 0.10 0.17 0.21 -3.33 . -0.09 -0.02 0.29 TG . -0.16 0.05 . . -0.65 -0.24 . TH 0.19 -0.12 -0.01 . . . . . TN 0.17 . -0.01 -3.33 . -0.15 . 0.60 TR 0.40 -0.31 0.21 . . . . . TT . 0.04 -0.01 -3.33 . -0.15 -0.02 . TW 0.16 . -0.01 . . . . . UA . . -0.01 . . . . . UG . . -0.01 -3.33 . -0.15 -0.02 0.29 US -0.07 -0.16 -0.11 . -0.06 . . . UY 0.19 . -0.01 . . . . . VC . . -0.01 -2.89 . -0.17 . 0.26 VE -0.08 -0.16 -0.03 -3.33 . -0.33 -0.16 . VG . -0.16 -0.01 . . -0.15 -0.02 . VI . -0.12 ...... YU 0.00 . -0.01 . . . . . ZA 0.16 . -0.01 . . . . . ZM . -0.04 -0.06 -3.19 . -0.03 0.01 0.30 ZR 0.16 0.06 -0.01 . . -0.15 . . Appendix B. Codes for standardized ILO October Inquiry Database 1983-2003 y0: year y1: country</p><p>AG Antigua and Barbuda AN Netherlands Antilles AO Angola AR Argentina AS American Samoa AT Austria AU Australia AZ Azerbaijan BB Barbados BD Bangladesh BE Belgium BF Burkina Faso BG Bulgaria BI Burundi BJ Benin BM Bermuda BN Brunei BO Bolivia BR Brazil BW Botswana BY Belarus BZ Belize CA Canada CF Central African Republic CI Ivory Coast CL Chile CM Cameroon CN China CO Colombia CR Costa Rica CS Czechoslovakia CU Cuba CV Cape Verde CY Cyprus CZ Czech Republic DE Germany DJ Djibouti DK Denmark DO Dominican Republic DZ Algeria EE Estonia ET Ethiopia FI Finland FJ Fiji FK Falkland Islands FR France GA Gabon GB United Kingdom GH Ghana GI Gibraltar GP Guadeloupe GQ Guinea Ecuatorial GU Guam GY Guyana HK Hong Kong HN Honduras HR Croatia HU Hungary IE Ireland IM Isle of Man IN India IR Iran, Islamic Republic of IS Iceland IT Italy JP Japan KG Kyrgyzstan KH Cambodia KM Comoros KN St Kitts and Nevis KR Korea, Republic of KZ Kazachstan LC St Lucia LK Sri Lanka LR Liberia LT Lithuania LU Luxembourg LV Latvia MD Moldova MG Madagascar ML Mali MM Myanmar MN Mongolia MO Macau, China MU Mauritius MW Malawi MX Mexico MZ Mozambique NC New Caledonia NE Niger NG Nigeria NI Nicaragua NL Netherlands NO Norway NZ New Zealand PE Peru PF French Polynesia PG Papua New Guinea PH Philippines PL Poland PM St Pierre and Miquelon PR Puerto Rico PT Portugal RO Romania RU Russian Federation (before 9.91: USSR) RW Rwanda SC Seychelles SD Sudan SE Sweden SG Singapore SH Saint Helena SI Slovenia SK Slovakia SL Sierra Leone SM Samoa SN Senegal SR Surinam SY Syria (United Arab Republic) SZ Swaziland TD Chad TG Togo TH Thailand TN Tunisia TR Turkey TT Trinidad and Tobago TW Taiwan UA Ukraine UG Uganda US United States UY Uruguay VC St Vincent and the Grenadines VE Venezuela VG Virgin Islands (British) VI Virgin Islands (US) YU Yugoslavia ZA South Africa ZM Zambia ZR Zaire y3: industry code AA Agricultural production (field crops) AB Plantations AC Forestry AD Logging AE Deep-sea and coastal fishing BA Coalmining BB Crude petroleum and natural gas production BC Other mining and quarrying CA Slaughtering, preparing and preserving meat CB Manufacture of dairy products CG Grain mill products CH Manufacture of bakery products DA Spinning, weaving and finishing textiles DB Manufacture of wearing apparel (except footwear) DC Manufacture of leather and leather products (except footwear) DD Manufacture of footwear EA Sawmills, planing and other wood mills EB Manufacture of wooden furniture and fixtures FA Manufacture of pulp, paper and paperboard FB Printing, publishing and allied industries GA Manufacture of industrial chemicals GB Manufacture of other chemical products GC Petroleum refineries IA Iron and steel basic industries JA Manufacture of metal products (except machinery and equipment) JB Manufacture of machinery (except electrical) JC Manufacture of electronic equipment, machinery and supplies JD Shipbuilding and repairing KA Electric light and power LA Construction MA Wholesale trade (grocery) MB Retail trade (grocery) MC Restaurants and hotels NA Railway transport NB Passenger transport by road NC Freight transport by road ND Maritime transport NE Supporting services to maritime transport NF Air transport NG Supporting services to air transport NH Communication OA Banks OB Insurance OC Engineering and architectural services PA Public administration PB Sanitary services PC Education services PD Medical and dental services PF Repair of motor vehicles y4: occupation code</p><p>1 Farm supervisor 2 Field crop farm worker 3 Plantation supervisor 4 Plantation worker 5 Forest supervisor 6 Forestry worker 7 Logger 8 Tree feller and bucker 9 Deep-sea fisherman 10 Inshore (coastal) maritime fisherman 11 Coalmining engineer 12 Miner 13 Underground helper, loader 14 Petroleum and natural gas engineer 15 Petroleum and natural gas extraction technician 16 Supervisor or general foreman 17 Derrickman 18 Miner 19 Quarryman 20 Butcher 21 Packer 22 Dairy product processor 23 Grain miller 24 Baker (ovenman) 25 Thread and yarn spinner 26 Loom fixer, tuner 27 Cloth weaver (machine) 28 Labourer 29 Garment cutter 30 Sewing-machine operator 31 Tanner 32 Leather goods maker 33 Clicker cutter (machine) 34 Laster 35 Shoe sewer (machine) 36 Sawmill sawyer 37 Veneer cutter 38 Plywood press operator 39 Furniture upholsterer 40 Cabinetmaker 41 Wooden furniture finisher 42 Wood grinder 43 Paper-making-machine operator (wet end) 44 Journalist 45 Stenographer-typist 46 Office clerk 47 Hand compositor 48 Machine compositor 49 Printing pressman 50 Bookbinder (machine) 51 Labourer 52 Chemical engineer 53 Chemistry technician 54 Supervisor or general foreman 55 Mixing- and blending-machine operator 56 Labourer 57 Mixing- and blending-machine operator 58 Packer 59 Labourer 60 Controlman 61 Occupational health nurse 62 Blast furnaceman (ore smelting) 63 Hot-roller (steel) 64 Metal melter 65 Labourer 66 Metalworking machine setter 67 Welder 68 Bench moulder (metal) 69 Machinery fitter-assembler 70 Labourer 71 Electronics draughtsman 72 Electronics engineering technician 73 Electronics fitter 74 Electronic equipment assembler 75 Ship plater 76 Power distribution and transmission engineer 77 Office clerk 78 Electric power lineman 79 Power-generating machinery operator 80 Labourer 81 Building electrician 82 Plumber 83 Constructional steel erector 84 Building painter 85 Bricklayer (construction) 86 Reinforced concreter 87 Cement finisher 88 Construction carpenter 89 Plasterer 90 Labourer 91 Stenographer-typist 92 Stock records clerk 93 Salesperson 94 Book-keeper 95 Cash desk cashier 96 Salesperson 97 Hotel receptionist 98 Cook 99 Waiter 100 Room attendant or chambermaid 101 Ticket seller (cash desk cashier) 102 Railway services supervisor 103 Railway passenger train guard 104 Railway vehicle loader 105 Railway engine-driver 106 Railway steam-engine fireman 107 Railway signalman 108 Road transport services supervisor 109 Bus conductor 110 Automobile mechanic 111 Motor bus driver 112 Urban motor truck driver 113 Long-distance motor truck driver 114 Ship's chief engineer 115 Ship's steward (passenger) 116 Able seaman 117 Dock worker 118 Air transport pilot 119 Flight operations officer 120 Airline ground receptionist 121 Aircraft cabin attendant 122 Aircraft engine mechanic 123 Aircraft loader 124 Air traffic controller 125 Aircraft accident fire-fighter 126 Post office counter clerk 127 Postman 128 Telephone switchboard operator 129 Accountant 130 Stenographer-typist 131 Bank teller 132 Book-keeping machine operator 133 Computer programmer 134 Stenographer-typist 135 Card- and tape-punching- machine operator 136 Insurance agent 137 Clerk of works 138 Computer programmer 139 Government executive official: 140 Stenographer-typist 141 Card- and tape-punching- machine operator 142 Office clerk 143 Fire-fighter 144 Refuse collector 145 Mathematics teacher (third level) 146 Teacher in languages and literature (third level) 147 Teacher in languages and literature (second level) 148 Mathematics teacher (second level) 149 Technical education teacher (second level) 150 First-level education teacher 151 Kindergarten teacher 152 General physician 153 Dentist (general) 154 Professional nurse (general) 155 Auxiliary nurse 156 Physiotherapist 157 Medical X-ray technician 158 Ambulance driver 159 Automobile mechanic 300 Pattern makers (wood) 301 Permanent way labourers 302 Labourers (unskilled, public parks and gardens)</p><p>Wages in local currency units: x1 wage reported in standard format in raw data (type 1) x2wu wage with country-specific calibration (type 2, uniform weighting) x2wl wage with country-specific calibration (type 2, lex weighting) x3wu wage with country-specific and uniform calibration (type 3, uniform weighting) x3wl wage with country-specific and uniform calibration (type 3, lex weighting) x4wu wage with uniform calibration (type 4, uniform weighting) x4wl wage with uniform calibration (type 4, lex weighting)</p><p>Wages in US $: x1us wage reported in standard format in raw data (type 1) x2wuus wage with country-specific calibration (type 2, uniform weighting) x2wlus wage with country-specific calibration (type 2, lex weighting) x3wuus wage with country-specific and uniform calibration (type 3, uniform weighting) x3wlus wage with country-specific and uniform calibration (type 3, lex weighting) x4wuus wage with uniform calibration (type 4, uniform weighting) x4wlus wage with uniform calibration (type 4, lex weighting) Appendix C</p><p>Table C1. Average monthly wage rates for male workers in US $ ------| y0 y1 | 1983 1984 1985 1986 1987 1988 1989 1990 1991 1992 1993 1994 1995 1996 1997 1998 1999 2000 2001 2002 2003 ------+------AG | 653 568 637 713 745 855 816 AN | 1259 1067 1108 1124 877 AO | 86 262 147 AR | 740 246 348 414 356 322 293 404 417 428 390 423 AS | 637 476 736 AT | 681 639 641 903 1146 1196 1158 1411 1435 1618 1582 1677 1982 1890 1662 1690 1649 1446 1448 1457 AU | 1570 1627 1284 1302 1424 1710 1779 1854 1964 1842 1760 2010 2111 2348 2045 2039 2052 AZ | 37 58 66 60 59 60 89 BB | 513 646 638 688 736 796 796 791 842 856 881 882 889 BD | 38 14 40 39 38 47 47 49 87 84 106 99 BE | 830 806 1068 1303 1347 1315 1625 1669 1844 1768 1875 2146 2080 1842 1838 1791 1600 1617 1749 1670 BF | 171 218 251 218 203 228 172 84 83 88 76 BG | 181 BI | 100 137 139 153 149 134 127 120 103 BJ | 145 128 136 188 123 192 236 BM | 1559 1436 1545 2311 2643 2970 2952 3452 3340 2828 3236 3238 3251 3527 2924 3512 BN | 8 7 BO | 146 156 147 124 193 198 222 224 240 228 247 281 BR | 276 495 420 BW | 209 178 BY | 500 554 851 205 767 80 82 108 126 BZ | 362 359 385 403 447 454 478 493 495 505 604 657 718 CA | 1516 1465 1573 1739 1667 1744 1810 1731 1688 1982 CF | 229 232 216 244 261 399 266 269 264 CI | 133 419 247 128 205 185 150 146 CL | 432 304 307 CM | 336 300 246 349 322 129 151 146 155 CN | 41 40 53 70 61 71 89 105 106 92 102 CO | 234 251 197 CR | 119 139 146 282 243 336 388 453 CS | 211 172 172 201 223 214 204 176 122 160 CU | * * * * * * CV | 164 187 CY | 751 640 654 811 944 1037 1058 1245 1325 1406 1348 1472 1671 1680 1610 1689 1580 1569 1748 CZ | 190 222 278 315 301 317 317 304 333 410 503 DE | 1296 1197 1155 1624 2026 2125 2029 2460 2550 2842 2760 2862 3350 3231 2838 2850 2640 4866 4804 5180 4947 DJ | 533 DK | 1570 1455 1467 2031 2587 2618 2525 3046 3027 3291 DO | 201 199 432 DZ | 2484 2725 2642 2164 1706 1632 932 1021 310 321 358 575 479 EE | 110 157 242 261 288 ET | 106 104 FI | 983 1030 1346 1661 1918 1993 2443 2442 2191 1753 1955 2567 2423 2171 2182 FJ | 430 414 369 325 FK | 518 1738 2013 FR | 2870 GA | 177 476 515 526 760 458 GB | 596 720 1275 1505 1530 1805 1956 2083 1840 1880 2035 2069 2267 2398 2420 2341 2355 2541 2841 GH | 26 91 GI | 913 842 705 948 1074 1038 1218 1286 GP | 1281 798 1295 1269 GQ | 58 GU | 1409 1518 1539 GY | 210 212 204 139 88 101 118 183 186 213 HK | 505 366 392 572 656 761 753 857 967 1075 1468 1586 1733 1927 2163 2045 2088 2094 2029 2057 HN | 281 297 289 291 330 354 180 146 171 127 108 125 212 HR | 360 HU | 160 282 290 270 284 313 299 330 412 554 IE | 937 827 IM | 717 662 972 1320 1570 IN | 226 44 49 51 51 58 66 53 169 45 57 67 48 81 77 79 85 IR | 813 IS | 702 707 681 856 1281 1575 1337 1410 1458 1567 1351 1279 1463 1489 1970 1992 1889 1464 1908 2580 IT | 740 993 1225 1290 1328 1651 1738 1876 1536 1529 1593 1747 1636 1646 1513 1412 1384 1494 1432 JP | 1402 1046 1070 1590 1876 2068 2146 2176 2437 2610 2947 3576 3106 2786 2625 2986 3171 KG | 31 45 27 30 31 KH | 19 21 22 21 89 70 69 68 67 KM | 133 106 130 163 169 241 213 233 184 211 227 KN | 378 308 663 698 KR | 347 336 329 348 399 523 671 740 822 872 888 978 1214 1196 1100 767 945 1091 1016 KZ | 160 185 LC | 410 425 381 419 440 445 482 528 544 LK | 40 43 51 35 47 65 41 46 56 61 60 74 82 75 80 81 92 90 LR | 335 284 LT | 165 279 LU | 3322 LV | 197 208 223 230 239 253 298 MD | 24 30 37 46 43 31 31 35 49 62 MG | 102 44 34 36 38 36 36 ML | 90 120 136 138 129 149 MM | 36 43 50 60 117 124 129 133 162 165 178 171 166 163 168 705 677 761 MN | 23 32 MO | 530 612 441 503 907 734 1036 982 999 946 955 971 * MU | 218 181 150 191 257 255 242 275 279 294 320 351 393 411 391 378 386 367 356 358 430 MW | 566 104 263 76 49 81 117 126 110 87 107 45 64 MX | 169 261 175 198 200 213 210 122 124 141 140 262 339 391 413 392 MZ | 61 72 93 NC | 1014 NE | 163 116 134 270 NG | 474 304 90 104 134 194 NI | 203 192 200 212 197 209 216 223 227 NL | 890 791 780 1088 1322 1385 1317 1582 NO | 1449 1388 1463 1834 2032 2323 2269 2604 2599 2763 2442 2497 2935 2994 2834 2910 2845 2709 3028 3417 3899 NZ | 777 741 932 1273 1496 1420 1501 1475 PE | 100 316 139 323 260 278 471 501 507 468 413 401 417 475 PF | 1885 2120 2083 2536 PG | 308 330 633 437 429 PH | 91 75 39 92 91 112 121 116 120 139 142 160 183 309 276 PL | 282 355 423 467 480 PM | 745 1160 1226 1546 1880 1897 1669 2112 PR | 745 880 1155 1047 1112 1420 1164 1246 1301 1389 1375 1503 1509 1614 1471 1572 1649 1638 1928 1904 PT | 155 209 259 286 299 557 438 529 470 472 819 731 755 754 670 RO | 161 171 * 145 168 126 176 163 179 186 161 191 167 174 175 193 232 RU | 609 545 41 105 136 145 73 94 237 RW | 184 166 211 221 211 204 135 SC | 345 340 317 357 385 405 SD | 902 1741 1579 1573 366 SE | 1068 1120 1055 1426 1747 1935 2026 2496 2479 2729 3433 2231 2470 SG | 437 492 514 554 630 712 727 808 845 921 1065 1132 1145 1124 1034 1035 985 952 SH | 277 549 211 222 289 SI | 580 619 807 806 SK | 249 299 249 269 244 243 295 333 SL | 27 23 41 38 42 47 56 52 SM | 500 587 SN | 279 SR | 533 619 676 713 722 764 591 634 714 85 182 272 346 SY | 703 745 944 984 SZ | 154 78 92 307 TD | 142 165 157 163 175 272 256 223 221 212 TG | 313 176 196 192 190 TH | 118 110 144 185 247 255 287 TN | 150 146 176 185 212 198 209 541 489 461 TR | 504 361 400 398 TT | 1154 820 791 741 666 492 618 541 554 TW | 964 965 UA | 103 129 64 UG | 75 US | 1949 2180 1831 1895 1986 2030 2124 2238 2293 2375 2448 2431 2542 2582 2938 3076 3189 3167 3589 3810 UY | 188 227 271 298 334 347 418 466 576 664 750 VC | 464 465 471 504 512 535 335 334 VE | 933 571 529 184 197 245 312 352 346 347 VG | 958 1136 VI | 1529 1454 1494 YU | 190 165 157 230 243 183 190 415 * * ZA | 554 553 ZM | 77 46 75 81 73 80 100 100 ZR | 101 ------Note: reported wages are based on type 3 standardization (lexicographic weighting). * = exchange rate not available in IMF IFS</p><p>1 The ILO actually asks for information on 159 occupations but it differentiates occupation 139, executives in the government into three sectors; national, regional or provincial, and local governments. 2 The maximum is the multiplicand of the number of countries (137) times the number of occupations (161) times the number of years (21). 3 In a few cases the wages are in the form of ranges. We found the midpoint of the range and report it as the wage for the category. 4 The situation is not quite as dire as this statistic indicates because we can obtain some greater comparability by taking account of the natural time dimensions, such as turning yearly earnings into monthly figures by dividing by 12, turning weekly wages into monthly by multiplying by 4.3, or by multiplying hourly pay by hours worked reported on the survey. But even if we standardize using these procedures, only 11.3 percent of the reported figures are directly comparable. 5 In case the wage data were reported as hourly or daily wages, then these wages were made (roughly) comparable with monthly wages by multiplication with 160 and 20 respectively. 6 By dimensional analysis we mean simply changing the time units in well-determined ways, such as obtaining weekly pay by dividing annual pay by 52. 7 If median wage observations from all countries were included, then the estimated data correction factor implied that median wages were higher than average wages. Only for the US the expected result was found, namely that median wages were lower than average wages. 8 See Oostendorp (2004) on the variation of the gender wage gap across occupations and time. 9 The following rules have been applied to identify implausible estimates: (1) number of working days per month more than 31, (2) minimum wage more than average wage, (3) prevailing wage deviates from average wage by more than 25%, and (4) maximum wage less than average wage. 10 The averages for the other types are non-comparable because of different numbers of observations.</p>
Details
-
File Typepdf
-
Upload Time-
-
Content LanguagesEnglish
-
Upload UserAnonymous/Not logged-in
-
File Pages31 Page
-
File Size-