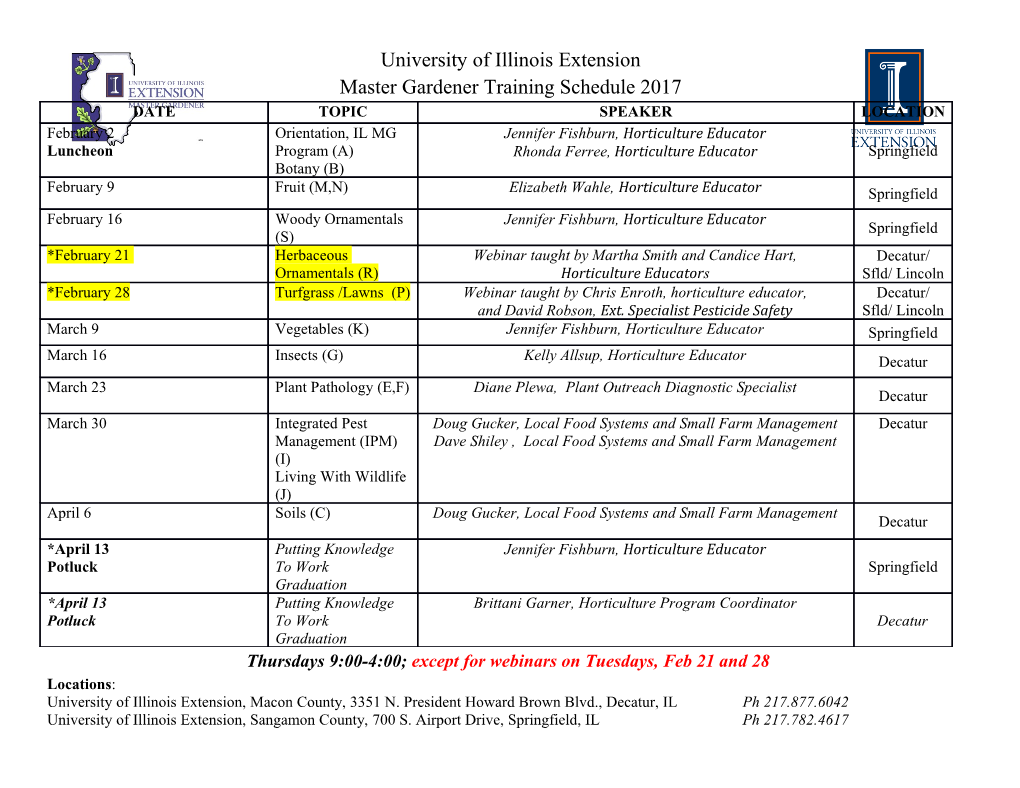
<p>Mgmt 469 Final Exam Spring 2007</p><p>You have 180 minutes to complete this exam. Please answer all questions for a maximum of 35 points. (All point totals are in parentheses.) I am not looking for long responses to the short answer questions. A few words, or at most a few sentences, will suffice. Precision will be rewarded. </p><p>Be sure to put your name on the exam. The exam is due in my office no later than Tuesday 5/29 at 5 pm. You may instead fax the exam to 847-467-1777; be sure to include a cover page. Please do not send the exam via email. </p><p>If you answer the questions by hand, then once you have finished, you may take extra time to retype your answers, provided that you make no changes except to correct spelling.</p><p>This is a closed book exam, but you may refer to one two-sided cheat sheet. Please include your cheat sheet when you return your exam. Name ______Mgmt 469 Final</p><p>(9 pts) 1) Indicate whether the following conclusions are true or false/premature. (By premature, I mean that there is nothing inherent in the set-up that would lead you to the conclusion.) Note that there is no partial credit.</p><p> a) Set-up: The residual in your regression is correlated with an omitted variable. Conclusion: The model is heteroscedastic T F</p><p> b) Set-up: The dependent variable in your OLS regression is measured with noise Conclusion: Your standard errors are biased towards zero T F</p><p> c) Set-up: The magnitude of your residuals is correlated with some predictor Z Conclusion: You can still keep the predictor in your model T F</p><p> d) Set-up: Your dependent variable comes from count data, is never less than 0, and has a median of 1 Conclusion: You can estimate a linear probability model provided that the predicted values do not exceed 1 T F</p><p> e) Set-up: You use ordered probit to study the number of firms in a market and observe that κ1 <2κ2, where κ are the “cut points.” Conclusion: The market size does not need to double before a second firm enters the market. T F</p><p> f) Set-up: You are computing the effects of a change in a significant RHS variable in a Logit regression. Conclusion: The predicted effect of a change in that variable varies as the value of that variable changes T F</p><p> g) Set-up: You run the OLS regression Y = B0 + B1X + B2Z Conclusion: The predicted effect of a change in X depends on the level of Z T F</p><p>2 h) Set-up: You run the OLS regression Y = B0 + B1X + B2X Conclusion: The predicted effect of a change in X depends on the level of X T F</p><p> i) Set-up: Your regression errors are correlated within groups Conclusion: It is okay to run a fixed effects model without “clustering” the standard errors T F j) Set-up: A key predictor in your model is correlated with an omitted variable that is also a key predictor Conclusion: The coefficients on the other included predictors are unbiased T F</p><p> k) Set-up: You run the regression logY = B0 + B1X You obtain B1 = 1.0 Conclusion: A one unit increase in X causes Y to increase by approximately 100 percent. T F</p><p> l) Set-up: You determine that your model suffers from heteroscedasticity Conclusion: Your coefficients are unbiased T F</p><p> m) Set-up: You have grouped data and determine that your “plain vanilla” OLS model generates unbiased estimates of the main predictors Conclusion: You still have to cluster the standard errors. T F</p><p> n) Set-up: You compare the coefficient on one included state in the fixed-state effects version of the ISP project and find that it is statistically significant. Conclusion: All larger coefficients (on other states) are also statistically significant T F</p><p> o) Set-up: You introduce fixed effects into a model and the R2 increases substantially even as key coefficients remain unchanged Conclusion: Your results are robust T F</p><p> p) Set-up: You find that a particular observation is influential, in the sense that one or more key coefficients change substantially when you drop it Conclusion: You should drop that observation T F</p><p> q) Set-up: You are evaluating, at an intuitive level, a potential instrumental variable for two stage least squares Conclusion: A good instrument has no causal relationship with the dependent variable and is correlated with the predictor variable. T F</p><p>(2 pts) 2) A predictor variable is defined to be endogenous if it is correlated with the error term. Use this definition to explain why reverse causality (i.e, “Y causes X”) implies that the relevant predictor X is endogenous. (1 pt) 3) Suppose that Y is a count variable and X is a continuous predictor. You estimate the following Poisson regression equation (standard errors may be ignored for purposes of answering this question): </p><p>Y = 4 + 2X + .3X2</p><p>Suppose that the mean value of X is Xm and the mean value of Y is Ym. What is the predicted effect of a one unit increase in X on the expected value of Y in the average market? </p><p>(6 pts) 4) Suppose you are estimating the determinants of starting salaries for Kellogg graduates. You have data on starting salaries and lots of predictor variables, such as each student's work experience. (To keep things simple, ignore bonuses and other components of earnings, and pretend that there is no harm in doing so.)</p><p> a) You are unsure whether to estimate earnings or log of earnings on the left hand side of your model. What is the economic distinction between the two? </p><p> b) You are unsure whether to include years of work experience or log of years of work experience on the right hand side. How would you determine which fits the data better?</p><p> c) You estimate a model without industry fixed effects and find that the coefficient on experience is positive, large, and significant. You include industry fixed effects and the coefficient on experience is zero. What can you infer about the industry choices of more experienced students?</p><p> d) You believe that work experience has a greater effect on earnings for those students who choose a job in the same industry in which they had previously worked. How you would test this claim? </p><p>(5 pts) 5) A recent study of the effects of advertising intensity on sales used time-series/ cross-section data from 100 U.S. firms. </p><p> a) What does “time series/cross-section” mean?</p><p> b) Describe in an intuitive manner why it would be desirable to include firm- fixed effects in this study.</p><p> c) Under what circumstances might it be impractical to include firm-fixed effects? (4 pts) 6) Consider the following regression model of executive compensation:</p><p>EXECPAY = B0 + B1PROFITS + B2MALE + B3(PROFITS*MALE)</p><p>EXECPAY = total compensation (in $millions) received by the CEO </p><p>PROFITS = the firm’s annual profits (in $billions)</p><p>MALE = a 0/1 dummy variable indicating sex (1=male). </p><p>The interaction term equals the product of PROFITS and MALE.</p><p>You obtain the following precisely estimated coefficients: B0 = 1, B1= 3, B2 = 1 and B3 = -1. </p><p>Draw two lines on one graph to fully display these results. (Hint: The axes are EXECPAY and PROFITS.) </p><p>(8 pts) 7) Please read the set-up to this problem carefully:</p><p>Consider the following study examining how firms set prices relative to their competitors. The unit of observation is the firm/year. The data cover three years (1994-1996) and the firms belong to three industries: Chemicals, Machinery, and Glass Products. All told, there are 600 observations divided evenly over time and across industries.</p><p>The dependent variable is RELATIVE PRICE. This is the firm’s price relative to its competitors, in percentage terms. (E.g., if RELATIVE PRICE = .10, then the firm’s price is ten percent higher than its competitors.) </p><p>The predictors include the following:</p><p>RELATIVE COST = The firm cost relative to its competitors. Like RELATIVE PRICE, this is measured in percentage terms. </p><p>COMPETITION = a measure of the level of market competition, ranging from 0 to 1, with a mean of .50. (For this measure, higher values imply more competition.) </p><p>The variable COMPETITION is included directly on the RHS. It is also interacted with RELATIVE COST. </p><p>CUSTOM: A measure of the degree to which the products are customized Here is the main regression output: </p><p>Predictor Coefficient Sign. Level RELATIVE COST .50 .001 COMPETITION -.20 .01 COMPETITION x RELCOST -.20 .01 CHEMICALS -.10 .12 MACHINERY .10 .12 CUSTOM .20 .03 1995 .07 .03 1996 .03 .08 a) Use your best judgment: Is there a linear time trend in RELATIVE PRICE, all else equal? b) Use your best judgment: Does it appear that there are significant industry effects? What additional test would you perform to confirm your judgment? c) What is the average effect of a one unit change in RELATIVE COST on the dependant variable? d) As a final step, you add the variable DIFFERENTIATED, which is a measure of the degree to which the firm’s products are differentiated from its competitors. You observe that DIFFERENTIATED is positively correlated with CUSTOM in the raw data. </p><p>∙ Does this imply that your regression will suffer from multicollinearity? ∙ If not, how would you confirm the presence of multicollinearity? </p>
Details
-
File Typepdf
-
Upload Time-
-
Content LanguagesEnglish
-
Upload UserAnonymous/Not logged-in
-
File Pages6 Page
-
File Size-