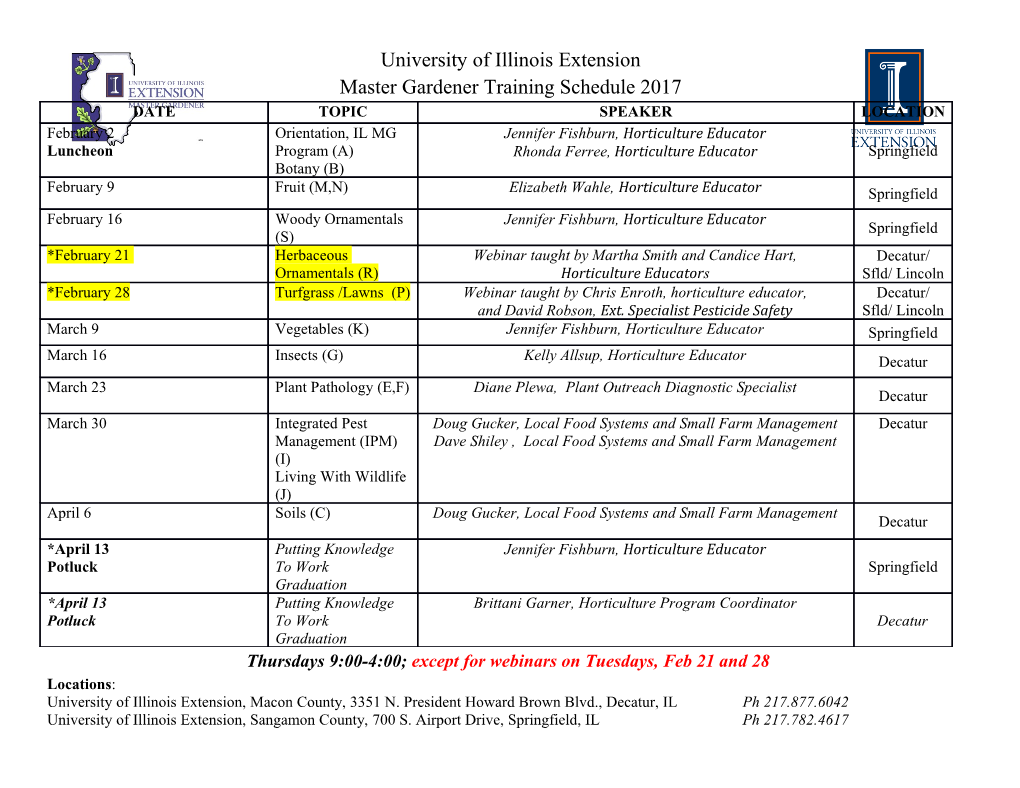
<p> 12/13/2017 CN550 MEMORY MODELS: VISUALIZATION SPRING 2008 1</p><p>CN550 Class Project: Matlab Code for Evaluation and Visualization</p><p>======</p><p>ROC curves and the c-index</p><p>======</p><p>Plot an ROC curve function [tp, fp] = roc(t, y) % % ROC - generate a receiver operating characteristic curve % % [TP,FP] = ROC(T,Y) gives the true-positive rate (TP) and false positive % rate (FP), where Y is a column vector giving the score assigned to each % pattern and T indicates the true class (a value above zero represents % the positive class and anything else represents the negative class). To % plot the ROC curve, % % PLOT(FP,TP); % XLABEL('FALSE POSITIVE RATE'); % YLABEL('TRUE POSITIVE RATE'); % TITLE('RECEIVER OPERATING CHARACTERISTIC (ROC)'); %</p><p>% process targets t = t > 0;</p><p>% sort by classifier output</p><p>[Y,idx] = sort(-y); t = t(idx);</p><p>% compute true positive and false positive rates tp = cumsum(t)/sum(t); fp = cumsum(~t)/sum(~t);</p><p>% add trivial end-points tp = [0 ; tp ; 1]; fp = [0 ; fp ; 1];</p><p>======12/13/2017 CN550 MEMORY MODELS: VISUALIZATION SPRING 2008 2</p><p>Convex hull of an ROC curve function [tp, fp] = rocch(t, y) % % ROCCH - generate a receiver operating characteristic convex hull % % [TP,FP] = ROCCH(T,Y) gives the true-positive rate (TP) and false positive % rate (FP), corresponding to the convex hull of the receiver operating % characteristic, where Y is a column vector giving the score assigned to % each pattern and T indicates the true class (a value above zero % represents the positive class and anything else represents the negative % class). To plot the ROC convex hull, % % PLOT(FP,TP); % XLABEL('FALSE POSITIVE RATE'); % YLABEL('TRUE POSITIVE RATE'); % TITLE('RECEIVER OPERATING CHARACTERISTIC CONVEX HULL (ROCCH)'); %</p><p>% generate the ROC curve</p><p>[tp,fp] = roc(t,y); tp = [tp ; 0]; fp = [fp ; 1];</p><p>% we are really interested in the convex hull idx = unique(convhull(fp, tp)); fp = fp(idx(1:end-1)); tp = tp(idx(1:end-1));</p><p>% bye bye...</p><p>======</p><p>Compute the c-index = the area under the ROC curve function A = auroc(tp, fp) % % AUROC - area under ROC curve % % An ROC (receiver operator characteristic) curve is a plot of the true % positive rate as a function of the false positive rate of a classifier % system. The area under the ROC curve is a reasonable performance 12/13/2017 CN550 MEMORY MODELS: VISUALIZATION SPRING 2008 3</p><p>% statistic for classifier systems assuming no knowledge of the true ratio % of misclassification costs. % % A = AUROC(TP, FP) computes the area under the ROC curve, where TP and FP % are column vectors defining the ROC or ROCCH curve of a classifier % system. % % [1] Fawcett, T., "ROC graphs : Notes and practical % considerations for researchers", Technical report, HP % Laboratories, MS 1143, 1501 Page Mill Road, Palo Alto % CA 94304, USA, April 2004. % % See also : ROC, ROCCH</p><p>% n = size(tp, 1); A = sum((fp(2:n) - fp(1:n-1)).*(tp(2:n)+tp(1:n-1)))/2;</p><p>% bye bye...</p><p>======</p><p>Another way to compute the c-index</p><p>% c_index code: % % ci=c_index_2(pred,des) % pred - predicted output % des - desired output % function ci=c_index_2(pred,des); neg=pred(find(des==0)); pos=pred(find(des==1)); total=0; for j=1:length(neg) s=length(find(pos>neg(j))); total=total+s; end ci=total/(length(neg)*length(pos)); ======12/13/2017 CN550 MEMORY MODELS: VISUALIZATION SPRING 2008 4</p><p>ROC tools demo</p><p>% % ROCDEMO - demonstrate use of ROC tools % % An ROC (receiver operator characteristic) curve is a plot of the true % positive rate as a function of the false positive rate of a classifier % system. The area under the ROC curve is a reasonable performance % statistic for classifier systems assuming no knowledge of the true ratio % of misclassification costs.</p><p>% start from a clean slate clear all</p><p>% generate test data from Fawcett [1] (fig 3) fprintf(1, 'generating test data...\n'); t = [1 1 0 1 1 1 0 0 1 0 1 0 1 0 0 0 1 0 1 0]'; y = [.9 .8 .7 .6 .55 .54 .53 .52 .51 .505 ... .4 .39 .38 .37 .36 .35 .34 .33 .3 .1]';</p><p>% generate an ROC curve and plot it fprintf(1, 'plotting ROC curve...\n');</p><p>[tp,fp] = roc(t,y); figure(1); clf; plot(fp,tp); xlabel('false positive rate'); ylabel('true positive rate'); title('ROC curve');</p><p>% compute the area under the ROC fprintf(1, 'AUROC = %f\n', auroc(tp,fp));</p><p>% compute the ROC convex hull (ROCCH) curve if 1</p><p> fprintf(1, 'plotting ROCCH curve...\n'); 12/13/2017 CN550 MEMORY MODELS: VISUALIZATION SPRING 2008 5</p><p>[tp,fp] = rocch(t,y);</p><p> hold on plot(fp, tp, 'r--'); hold off xlabel('false positive rate'); ylabel('true positive rate'); title('ROC and ROCCH curve');</p><p>% compute the area under the ROCCH</p><p> fprintf(1, 'AUROCCH = %f\n', auroc(tp,fp)); end</p><p>======</p><p>Financial dataset: January 2006 profit (or loss) from Investment Strategy 1 function cost = fin_cost_function(preds);</p><p>% assuming preds is a vector of 1x21 of 1s (predict UP) and % -1s (predict DOWN)</p><p>% NOTE: You might have to edit the prediction vector since % the dataset has 0s and 1s for class labels! % in other words, make 0s as -1s before you call this function! % Prices are from 21 trading days in January 2006. price_each_day = [0.41,0.14,0.64,0.46,0.1,0.35,-0.66,-0.23,-0.53,-0.41,0.13,- 2.26,0.39,0.08,0.21,0.86,1.1,-0.2,-0.64,1.3,-1.27]; cost = sum (price_each_day .* preds);</p><p>======12/13/2017 CN550 MEMORY MODELS: VISUALIZATION SPRING 2008 6</p><p>Circle-in-the Square (CIS) visualization</p><p>======</p><p>CIS visualization in black & white</p><p>% Routine for CIS results visualization % x is a vector of 1s and 0s, % 0 stands for square, 1 - for circle (as in the specs) % Usage: cis_vis(x) function cis_vis(x) warning off % Check for the right format, if not, then transpose x d = size(x); if(d(2)==max(d)) x=x'; end</p><p>% Construct the matrix for plotting dim = sqrt(max(d)); T = x(1:dim); for n=2:dim T=[T, x(1+dim*(n-1):n*dim)]; end</p><p>% Plot the result clf hold on g=[0 0 0; 1 1 1]; colormap(g); X=0:1/(length(T)-1):1; Y=0:1/(length(T)-1):1; pcolor(X,Y,T); shading flat</p><p>% Plot the desired class boundary warning off; x1=(.5-1/sqrt(2*pi)); x2=(.5+1/sqrt(2*pi)); x=x1:(x2-x1)/1000:(x2+(x2-x1)/1000); y1=sqrt(1/(2*pi)-(x-.5).^2)+.5; y2=-sqrt(1/(2*pi)-(x-.5).^2)+.5; plot(x,y1,'r','LineWidth',2) 12/13/2017 CN550 MEMORY MODELS: VISUALIZATION SPRING 2008 7 plot(x,y2,'r','LineWidth',2) axis square; axis([-.05 1.05 -.05 1.05]) box on warning on; hold off</p><p>======</p><p>CIS visualization in color function [ plot_fig ] = cis_vis_color( test_data, predictions, stitle ) % cis_vis_color Plots the performance of a circle-in-square classification test. % % plot_fig ~ figure handle ~ handle to produced plot figure % test_data ~ [number of testing inputs x 3] ~ numeric % ~ testing data in the form [xcoord,ycoord,expected_class] % where expected_class in { 0, 1 } % predictions ~ [number of testing inputs x 1] % ~ numeric ~ classification predictions in { 0, 1 } % stitle ~ string ~ figure title (assumes Interpreter=Tex) %</p><p>% plot predictions plot_2D_test_class(test_data,predictions,stitle);</p><p>% draw circle %warning off; x1=(.5-1/sqrt(2*pi)); x2=(.5+1/sqrt(2*pi)); x=x1:(x2-x1)/1000:(x2+(x2-x1)/1000); y1=sqrt(1/(2*pi)-(x-.5).^2)+.5; y2=-sqrt(1/(2*pi)-(x-.5).^2)+.5; hold on; plot(x,y1,'k','LineWidth',2) plot(x,y2,'k','LineWidth',2) axis square; axis([-.05 1.05 -.05 1.05]); hold off;</p><p>======12/13/2017 CN550 MEMORY MODELS: VISUALIZATION SPRING 2008 8</p><p>Visualizing results for 2D inputs and 2 output classes (e.g., CIS) function [ plot_fig ] = plot_2D_test_class( test_data, predictions, stitle ) % plot_2D_test_class Plots the performance of a classification test with % two input dimensions and two output classes. % % plot_fig ~ figure handle ~ handle to produced plot figure % test_data ~ [number of testing inputs x 3] ~ numeric % ~ testing data in the form [xcoord,ycoord,expected_class] % where expected_class in { 0, 1 } % predictions ~ [number of testing inputs x 1] % ~ numeric ~ classification predictions in { 0, 1 } % stitle ~ string ~ figure title (assumes Interpreter=Tex)</p><p>% check inputs if ~exist('test_data') error('"test_data" cannot be null.'); end; if size(test_data,2)~=3 error('plot_2D_test_class only handles 2D inputs.'); end; if ~exist('predictions') error('"predictions" cannot be null.'); end; if size(predictions,2)~=1 error('"predictions" must be an Nx1 column vector.'); end; if size(test_data,1)~=size(predictions,1) error('Length of "test_data" and "predictions" must be equal.'); end; markersize = 6;</p><p>%%%% % Use these if you want to color incorrect predictions differently from % correct one. % test_x = test_data(predictions==1 & test_data(:,3)==1,1:2); % test_o = test_data(predictions==0 & test_data(:,3)==0,1:2); % wrong_x = test_data(predictions==1 & test_data(:,3)==0,1:2); % wrong_o = test_data(predictions==0 & test_data(:,3)==1,1:2); %%% % Use these if you want to color all predictions of the same class the % same. test_x = test_data(predictions==1,1:2); 12/13/2017 CN550 MEMORY MODELS: VISUALIZATION SPRING 2008 9 test_o = test_data(predictions==0,1:2); wrong_x = []; wrong_o = []; %%%% plot_fig = figure; set(gca,'FontSize',12); set(gca,'XTick',[]); set(gca,'YTick',[]); hold on; plot(test_x(:,1),test_x(:,2),'b.','MarkerSize',markersize); plot(test_o(:,1),test_o(:,2),'r.','MarkerSize',markersize); %plot(wrong_x(:,1),wrong_x(:,2),'k.','MarkerSize',markersize); %plot(wrong_o(:,1),wrong_o(:,2),'g.','MarkerSize',markersize);</p><p>%xlabel('x','FontSize',14); %ylabel('y','FontSize',14); if (exist('stitle')) title(stitle, 'FontSize',14); end; legendstr = {'Class 1','Class 0', 'False 1','False 0'}; legendlogic = [ length(test_x)>0, length(test_o)>0, length(wrong_x)>0, length(wrong_o)>0 ]; legend(legendstr(legendlogic),'FontSize',8, 'Location','best' ); hold off;</p>
Details
-
File Typepdf
-
Upload Time-
-
Content LanguagesEnglish
-
Upload UserAnonymous/Not logged-in
-
File Pages9 Page
-
File Size-