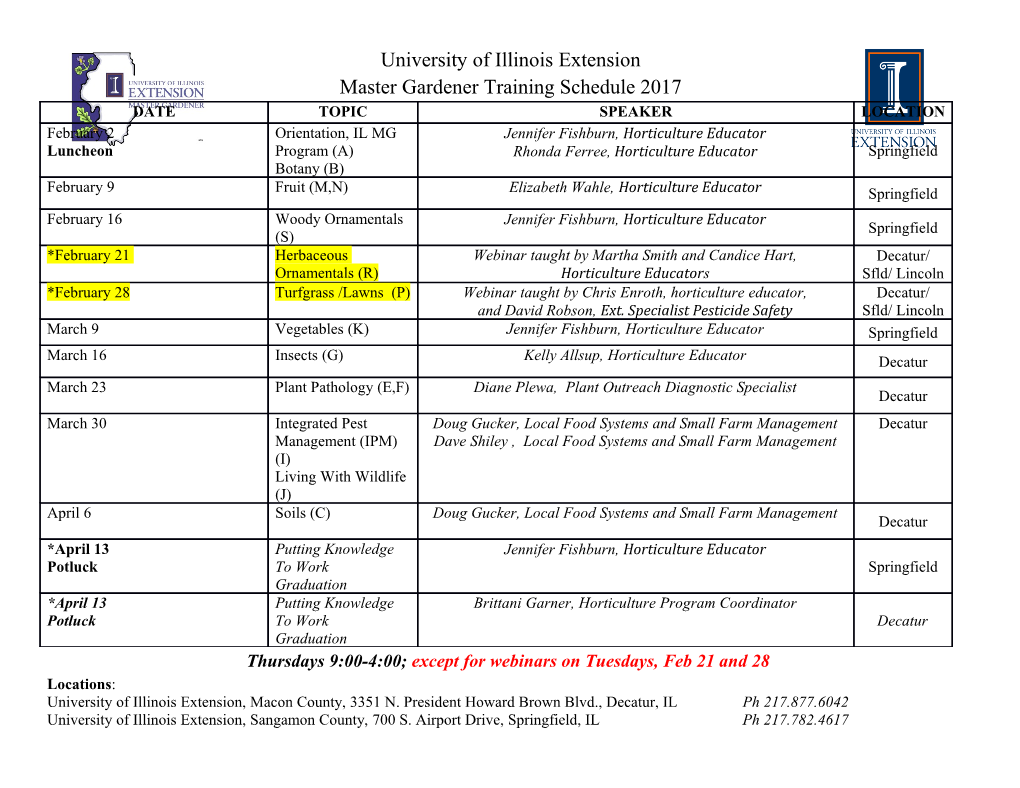
<p> Data Management in Natural Resources</p><p>(FOR 603/608 lecture notes)</p><p>Fikret Isik Research Assistant Professor of Quantitative Forest Genetics [email protected] </p><p>Department of Forestry and Environmental Resources, NCSU 1019 Biltmore Hall</p><p>Lecture Outline</p><p>1. Statistical Components of a Research 2. Some Definitions in the Experiment and Survey Data 3. Design of Experiments (design tools, sample size) 4. Data Management Using a Software 5. Data cleaning (outliers, typo errors, odd values) 6. Descriptive Statistics 7. Data Summary (plots, charts) 8. ANOVA examples</p><p>1. Statistical components of a research*</p><p> A plan to conduct the experiment/s to collect the right type of data, and enough of it. Talk to a statistician before designing an experiment</p><p> Prepare specific questions and construct hypothesis. Planning an experiment begins with carefully considering what the goals are. </p><p> Prioritize objectives. That helps you decide which direction to go with regard to the selection of the factors, responses and the particular design.</p><p> Have a timetable and follow it, write activities in detail (research diary). </p><p>Page 1 of 13, April 2007, FOR 603 Lecture Notes – Fikret Isik Example 1. (From a WPS student project)</p><p>The paper industry is constantly looking for ways to operate more efficiently. One way to increase the efficiency of papermaking is using additives. The effects of starch, PAE, and latex on paper strength and elasticity were tested in an experiment. The possible benefits could be greater paper strength values, reduced additives and fiber usage. Possible questions: 1. Which additive is the most efficient to increase the paper strength and elasticity? 2. If we add different levels of an additive, how does the fiber usage change? Does it decrease? 3. At which stage of the paper making is the additive more efficient? </p><p>Consider the following points: 1. What are the factors? How many levels are needed? 2. Is the measuring process simple? 3. Does the study produce reliable data? 4. Is the cost reasonable? 5. Can the study be completed in timely manner? 6. Are there extraneous variable effecting measurements? 7. How to summarize data? Appropriate statistical methods to answer questions? 8. What kind of inferences can be made from the results? We use results from statistical analysis based on the sample to make inference about the population.</p><p>2. Some Definitions in the Experiment and Survey Data</p><p> Factor: A factor of an experiment is a controlled independent variable whose levels are set by the experimenter. Irrigation or fertilizers are factors. Treatment: A treatment is a level (amount) of factor applied to the experimental units. For example, a field experiment is divided into four units; each part is 'treated' with 10mg, 20mg, 30mg and 40mg of the same fertilizer N. Each amount of N is a treatment. Variable: All the factors, their levels and all the measured traits (responses) are variables Independent or class variables: Factors applied to the experimental units are independent variables Response or dependent variables: Measured or observed traits in the experiment. Height of seedlings measured after ‘treated’ with irrigation is a response (or dependent) variable. Main effects: This is the simple effect of a factor on a dependent variable. It is the effect of the factor alone averaged across the levels of other factors. Example: In the fertilization experiment, it was found that on average, the N increased the growth (main effect of N) compared to the control (no fertilization). Interaction effect: An interaction is the variation among the differences between means for different levels of one factor over different levels of another factor. Example: Lets say watering and fertilization both significantly increased the growth (main effect of N and irrigation). However, when N and </p><p>Page 2 of 13, April 2007, FOR 603 Lecture Notes – Fikret Isik irrigation applied to the same experimental unit, it was found that the increase in growth was even higher. The plants got the benefits of both factors, plus a bonus. Experimental (or Sampling) Unit: A unit is a person, animal, plant or thing, which is studied by a researcher; the basic objects upon which the study or experiment is carried out. For example, a person; a sample of soil; a pot of seedlings are units. Population: A population is any entire collection of people, animals, plants from which we may collect data. It is the entire group we are interested in, which we wish to describe or draw conclusions about. Example, we are interested in average GPA values of all CNR students. All the students registered in CNR is a population. Sample: A sample is a group of units selected from a larger group (the population). By studying the sample it is hoped to draw valid conclusions about the larger group. A sample is generally selected for study because the population is too large to study in its entirety. Randomization: The sample should be representative of the general population. This is often best achieved by random sampling. For each population there are many possible samples. Parameter: A parameter is a value, usually unknown (and which therefore has to be estimated). It is used to represent a certain population characteristic. For example, the population mean is a parameter that is often used to indicate the average value of a quantity. Parameters are often assigned Greek letters (e.g. sigma). Statistic: A statistic is a quantity that is calculated from a sample of data. For example, the mean, a variance and a standard deviation calculated from a sample are statistics. Statistics are assigned Roman letters (e.g. s for standard deviation). The value of a statistic will vary from sample to sample. Sampling Distribution: The sampling distribution is the probability distribution or probability density function of the statistic. Derivation of the sampling distribution is the first step in calculating a confidence interval or carrying out a hypothesis test for a parameter. Example, suppose that x1, ...... , xn are a simple random sample from a normally distributed population with expected value µ and known variance sigma^2. Then the sample mean x_bar is normally distributed with expected value µ and variance sigma^2/n Estimate: An estimate is an indication of the value of an unknown quantity based on observed data. Example, suppose we want to know the average height of CNR students (population). We can use an estimate of this population mean by calculating the mean of a sample of students. Estimators of population parameters are sometimes distinguished from the true value by using the symbol 'hat'. For example, σ = true population standard deviation. ˆ = estimated (from a sample) population standard deviation. Exercise: What are the factors, treatments and independent variables in the paper strength experiment?</p><p>Source http://www.stats.gla.ac.uk/steps/glossary/anova.html#maineff</p><p>Page 3 of 13, April 2007, FOR 603 Lecture Notes – Fikret Isik 3. Design of Experiments Tools for developing experimental designs</p><p>Randomization</p><p>Treatments should be allocated to the experimental units randomly. In the paper strength experiment, we would like to treat 10 samples per additive. The additives should be assigned to 10 paper samples randomly. Why is it important? </p><p>1. First, randomization makes sure that the sample is a representative of the population (whole paper produced in the mill). </p><p>2. Secondly, randomization eliminates the effect of systematic biases. Let’s say the humidity in the mill increases during the day. If we do not randomly assign additives to the sample papers during the day, the humidity may have a confounding effect on the paper strength.</p><p>Replication</p><p>This is the number of experimental units (paper samples) measured for each treatment. Increasing the number of replications means collecting more information about the treatments. In the paper strength example, we tested 10 paper samples for each additive. Thus the number of replications per treatment is 10. Replication provides the variability in the response variable that is not associated with the treatment effects. Increasing the number of replications increases the reliability of the outcome. Let Yˆ is the mean, S is the variance and n is the number of observations of the response variable. The standard S error of the paper strength mean (SE) would be SE ( ˆ ) = . Y n SE of the mean decreases as n (number of observations) increases.</p><p>Blocking</p><p>Blocking is a technique used to eliminate the effect of a confounding factor. Blocks are group of experimental units sharing a common level of a confounding variable. For example, in the paper strength experiment, we assume that humidity is an external factor and affecting the paper strength. If we treat all 10 samples with starch in the morning, and treat another 10 random samples with latex in the afternoon, we cannot eliminate the affect of humidity. The effect of treatments will be confounded. There are different blocking techniques. The most commonly blocking is the Randomized Complete Blocks. It is random, because the experimental units are randomly assigned to each block. It is complete, because each treatment is included in every block. Assignment of experimental units to the blocks and to the treatments must be random. </p><p>Page 4 of 13, April 2007, FOR 603 Lecture Notes – Fikret Isik Variance: Distribution of individual observations around the means (x x)2 s2= i n 1</p><p>Standard deviation: Square root of variance. Distribution of individual observations around the mean (x x)2 s = i n 1</p><p>Standard error of the mean: Distribution of sample means around the population mean se s n</p><p>Confidence interval: How confident are we about the sample mean x representing the population mean ? For 95% CI, ( x -1.96*se x +1.96*se) where 1.96 is the Z value for 95% level</p><p>Sample size The decision between the sample size and the cost is a compromise. More measurements increase the reliability of the results but also increase the cost. In general, studying whole population is prohibitively expensive. We take a sample from the population to make inference.</p><p>How many observations do I need (sample size)? The answer varies depending on the accuracy sought. Let us say the true population standard deviation of paper strength is σ = 0.12. If we are satisfied with a d=0.05 margin of error, then for a 95% confidence level we need to have at least 2 n=(tα/2,n-1*σ/d) where α/2 is the error rate (typically .025) =(1.96*0.12/0.05) 2 = 22 as sample size, where 1.96 is the Z value for 95% level. For the same margin of error, what is the number of samples required for a population standard deviation 0.17? If d=0.10 is an acceptable margin of error, what is the sample size needed for σ = 0.17?</p><p>* The above notes were summarized from a textbook: Statistical Research Methods in the life Sciences. By P.V. Rao. Duxbury Press.</p><p>Page 5 of 13, April 2007, FOR 603 Lecture Notes – Fikret Isik 4. Data Management Using a Software Statistical analysis software packages are essential tools to analyze data. With some practice, it will be simple to use a package. There are many packages in the market, such as SAS, Minitab, SPSS, and S-Plus. SAS is one of the most powerful and commonly used packages used for data summary and analysis. The software is freely available to students in all unity labs at NCSU. </p><p>A brief intro to the SAS for research data summary: SAS windows, data entry, import wizards, file management.</p><p>5. Data cleaning (outliers, typo errors, odd values) Example 30.1: (Source: http://v8doc.sas.com/sashtml/) The following example investigates how snapdragons grow in various soil types. To eliminate the effect of local fertility variations, the experiment is run in blocks, with each soil type sampled in each block. </p><p>Type Block StemLength Clinton 1 32.1 Clinton 2 29.7 Clinton 3 29.1 Knox 1 35.7 Knox 2 35.9 Knox 3 33.1 Compost 1 31.8 Compost 2 28.0 Compost 3 29.2 Webster 1 32.5 Webster 2 31.1 Webster 3 29.7 </p><p>/* Typing data in the SAS Editor Window */</p><p> data plants; input Type $ Block StemLength ; datalines; Clinton 1 32.1 Knox 1 37.7 Compost 1 33.8 Webster 1 32.5 Clinton 2 29.7 Knox 2 35.9 Compost 2 32.0 Webster 2 31.1 Clinton 3 29.1 Knox 3 36.1 Compost 3 29.2 Webster 3 29.7 Page 6 of 13, April 2007, FOR 603 Lecture Notes – Fikret Isik ; proc print ; run;</p><p>/* Checking errors in the data */ proc univariate data=plants plot; var StemLength; run;</p><p>* By treatments; proc univariate data=plants plot; by block ; var stemLength; run;</p><p>6. Descriptive Statistics Mean, Variance, Standard deviation, Standard error, Coefficient of variation. Explanatory data analysis will give you an overall picture. You will get to know data. When analyzing data, do not skip this step.</p><p>/* Descriptive statistics */ proc means mean std stderr ; class type ; var StemLength; run;</p><p>7. Data Summary (plots, charts)</p><p>/* Using SAS-GRAPH to Summarize Data */ title 'VBAR charts with Means and 95% CL'; proc gchart ; vbar type / TYPE=mean SUMVAR=StemLength coutline=black width=8 errorbar=bars ; Page 7 of 13, April 2007, FOR 603 Lecture Notes – Fikret Isik run;</p><p>8. ANOVA example</p><p>8a. Balanced ANOVA and comparisons of Means</p><p>/* Contrasts */</p><p> proc glm order=data; class Block Type; model StemLength = Block Type ; means Type / waller regwq; /*------cltn-knox-cpst-wbsh */ contrast 'Clinton vs. others' Type -3 1 1 1 ; contrast "Knox vs. webster" Type 0 -1 0 1 ; run; quit;</p><p>The GLM Procedure</p><p>Class Level Information</p><p>Class Levels Values</p><p>Block 3 1 2 3</p><p>Type 4 Clinton Knox Compost Webster</p><p>Number of Observations Read 12</p><p>Number of Observations Used 12</p><p>Dependent Variable: StemLength </p><p>Source DF Sum of Squares Mean Square F Value Pr > F</p><p>Model 5 88.48916667 17.69783333 32.57 0.0003</p><p>Error 6 3.26000000 0.54333333 </p><p>Corrected Total 11 91.74916667 </p><p>Page 8 of 13, April 2007, FOR 603 Lecture Notes – Fikret Isik R-Square Coeff Var Root MSE StemLength Mean</p><p>0.964468 2.286208 0.737111 32.24167</p><p>Source DF Type I SS Mean Square F Value Pr > F</p><p>Block 2 12.52666667 6.26333333 11.53 0.0088</p><p>Type 3 75.96250000 25.32083333 46.60 0.0002</p><p>Source DF Type III SS Mean Square F Value Pr > F</p><p>Block 2 12.52666667 6.26333333 11.53 0.0088</p><p>Type 3 75.96250000 25.32083333 46.60 0.0002</p><p>Contrast DF Contrast SS Mean Square F Value Pr > F</p><p>Clinton vs. others 1 15.08027778 15.08027778 27.76 0.0019</p><p>Knox vs. webster 1 44.82666667 44.82666667 82.50 <.0001</p><p>Waller-Duncan K-ratio t Test for StemLength</p><p>Kratio 100</p><p>Error Degrees of Freedom 6</p><p>Error Mean Square 0.543333</p><p>F Value 46.60</p><p>Critical Value of t 2.37051</p><p>Minimum Significant Difference 1.4267</p><p>Page 9 of 13, April 2007, FOR 603 Lecture Notes – Fikret Isik Means with the same letter are not significantly different.</p><p>Waller Grouping Mean N Type</p><p>A 36.5667 3 Knox</p><p>B 31.1000 3 Webster</p><p>B 31.0000 3 Compost</p><p>B 30.3000 3 Clinton</p><p>8b. Unbalanced two-way ANOVA</p><p>/* UnBalanced two-way ANOVA */</p><p> proc glm data=twoway; class drug disease; model y=drug disease drug*disease / ss1 ss2 ss3 ss4; run;</p><p>Unbalanced Two-Way Analysis of Variance The GLM Procedure</p><p>Class Level Information</p><p>Class Levels Values</p><p> drug 4 1 2 3 4 disease 3 1 2 3</p><p>Number of Observations Read 72 Number of Observations Used 58 Dependent Variable: y Sum of Source DF Squares Mean Square F Value Pr > F Model 11 4259.338506 387.212591 3.51 0.0013 Error 46 5080.816667 110.452536 Corrected Total 57 9340.155172</p><p>R-Square Coeff Var Root MSE y Mean 0.456024 55.66750 10.50964 18.87931</p><p>Source DF Type I SS Mean Square F Value Pr > F Page 10 of 13, April 2007, FOR 603 Lecture Notes – Fikret Isik drug 3 3133.238506 1044.412835 9.46 <.0001 disease 2 418.833741 209.416870 1.90 0.1617 drug*disease 6 707.266259 117.877710 1.07 0.3958</p><p>Source DF Type II SS Mean Square F Value Pr > F drug 3 3063.432863 1021.144288 9.25 <.0001 disease 2 418.833741 209.416870 1.90 0.1617 drug*disease 6 707.266259 117.877710 1.07 0.3958</p><p>Source DF Type III SS Mean Square F Value Pr > F drug 3 2997.471860 999.157287 9.05 <.0001 disease 2 415.873046 207.936523 1.88 0.1637 drug*disease 6 707.266259 117.877710 1.07 0.3958</p><p>Source DF Type IV SS Mean Square F Value Pr > F drug 3 2997.471860 999.157287 9.05 <.0001 disease 2 415.873046 207.936523 1.88 0.1637 drug*disease 6 707.266259 117.877710 1.07 0.3958</p><p>Note the differences between the four types of sums of squares. The Type I SS for drug essentially tests for differences between the expected values of the arithmetic mean response for different drugs, unadjusted for the effect of disease. </p><p> By contrast, the Type II SS for drug measure the differences between arithmetic means for each drug after adjusting for disease. </p><p> The Type III SS measures the differences between predicted drug means over a balanced drug×disease population - that is, between the LS-means for drug. </p><p> Finally, the Type IV SS is the same as the Type III SS in this case, since there is data for every drug-by- disease combination. </p><p>No matter which sum of squares you prefer to use, this analysis shows a significant difference among the four drugs, while the disease effect and the drug-by-disease interaction are not significant. </p><p>Type III SS correspond to differences between LS-means, so you can follow up the Type III tests with a multiple comparisons analysis of the drug LS-means. </p><p>Since the GLM procedure is interactive, you can accomplish this by submitting the following statements after the previous ones that performed the ANOVA. </p><p> lsmeans drug / pdiff=all adjust=tukey; run;</p><p>Least Squares Means Adjustment for Multiple Comparisons: Tukey-Kramer</p><p>LSMEAN drug y LSMEAN Number</p><p>1 25.9944444 1 Page 11 of 13, April 2007, FOR 603 Lecture Notes – Fikret Isik 2 26.5555556 2 3 9.7444444 3 4 13.5444444 4</p><p>Least Squares Means for effect drug Pr > |t| for H0: LSMean(i)=LSMean(j)</p><p>Dependent Variable: y i/j 1 2 3 4</p><p>1 0.9989 0.0016 0.0107 2 0.9989 0.0011 0.0071 3 0.0016 0.0011 0.7870 4 0.0107 0.0071 0.7870 </p><p>Page 12 of 13, April 2007, FOR 603 Lecture Notes – Fikret Isik Homework </p><p>Height, weight and age of school children were measured for boys and girls separately. The objectives are 1) to determine the average height and weight in the school, 2) to investigate differences between two genders, and 3) examine the relationships between age and weight.</p><p>1. What are the independent, and response variables in the data? 2. Write specific questions according to the objectives of the study. 3. Copy the following lines and paste into a SAS Editor. Using the SAS procedures;</p><p>*------Data on Age, Weight, and Height of Children------* | Age (months), height (inches), and weight (pounds) were | | recorded for a group of school children. | | From Lewis and Taylor (1967). | *------*; </p><p> data htwt; input gender $ age :3.1 height weight @@; datalines; f 143 88.9 85.0 f 155 62.3 105.0 f 153 63.3 108.0 f 161 59.0 92.0 f 191 62.5 112.5 f 171 62.5 112.0 f 185 59.0 104.0 f 142 56.5 69.0 f 160 62.0 94.5 f 140 53.8 68.5 f 139 61.5 104.0 f 178 61.5 103.5 f 157 64.5 123.5 f 149 58.3 93.0 f 143 51.3 50.5 f 145 58.8 89.0 f 191 65.3 107.0 f 150 59.5 78.5 f 147 61.3 115.0 f 180 63.3 114.0 f 141 61.8 85.0 f 140 53.5 81.0 f 164 58.0 83.5 f 176 61.3 112.0 f 185 63.3 101.0 f 166 61.5 103.5 f 175 60.8 93.5 f 180 59.0 112.0 f 210 65.5 140.0 f 146 56.3 83.5 f 170 64.3 90.0 f 162 58.0 84.0 f 149 64.3 110.5 f 139 57.5 96.0 f 186 57.8 95.0 f 197 61.5 121.0 f 169 62.3 99.5 f 177 61.8 142.5 f 185 65.3 118.0 f 182 58.3 104.5 f 173 62.8 102.5 f 166 59.3 89.5 f 168 61.5 95.0 f 169 62.0 98.5 f 150 61.3 94.0 f 184 62.3 108.0 f 139 52.8 63.5 f 147 59.8 84.5 f 144 59.5 93.5 f 177 61.3 112.0 f 178 63.5 148.5 f 197 64.8 112.0 f 146 60.0 109.0 f 145 59.0 91.5 f 147 55.8 75.0 f 145 57.8 84.0 f 155 61.3 107.0 f 167 62.3 92.5 f 183 64.3 109.5 f 143 55.5 84.0 f 183 64.5 102.5 f 185 60.0 106.0 f 148 56.3 77.0 f 147 58.3 111.5 f 154 60.0 114.0 f 156 54.5 75.0 f 144 55.8 73.5 f 154 62.8 93.5 f 152 60.5 105.0 f 191 63.3 113.5 f 190 66.8 140.0 f 140 60.0 77.0 f 148 60.5 84.5 f 189 64.3 113.5 f 143 58.3 77.5 f 178 66.5 117.5 f 164 65.3 98.0 f 157 60.5 112.0 f 147 59.5 101.0 f 148 59.0 95.0 f 177 61.3 81.0 f 171 61.5 91.0 f 172 64.8 142.0 f 190 56.8 98.5 f 183 66.5 112.0 f 143 61.5 116.5 f 179 63.0 98.5 f 186 57.0 83.5 f 171 63.0 84.0 f 167 61.0 93.5 f 182 64.0 111.5 f 144 61.0 92.0 f 193 59.8 115.0 f 141 61.3 85.0 f 164 63.3 108.0 f 186 63.5 108.0 ; run;</p><p>4. Using the UNIVARIATE procedure, check for errors in the data. 5. Plot the height *weight, and age*weight using the Proc GPLOT 6. Produce bar charts for boys and girls separately using the height with error bars (Use Proc GCHART). 7. Calculate overall descriptive statistics (mean, std, variance, stderror, N) using the MEANS procedure and descriptive statistics for boys and girls separately. Put the outputs in a Word Document and send the homework to Dr. Abt</p><p>Page 13 of 13, April 2007, FOR 603 Lecture Notes – Fikret Isik</p>
Details
-
File Typepdf
-
Upload Time-
-
Content LanguagesEnglish
-
Upload UserAnonymous/Not logged-in
-
File Pages13 Page
-
File Size-