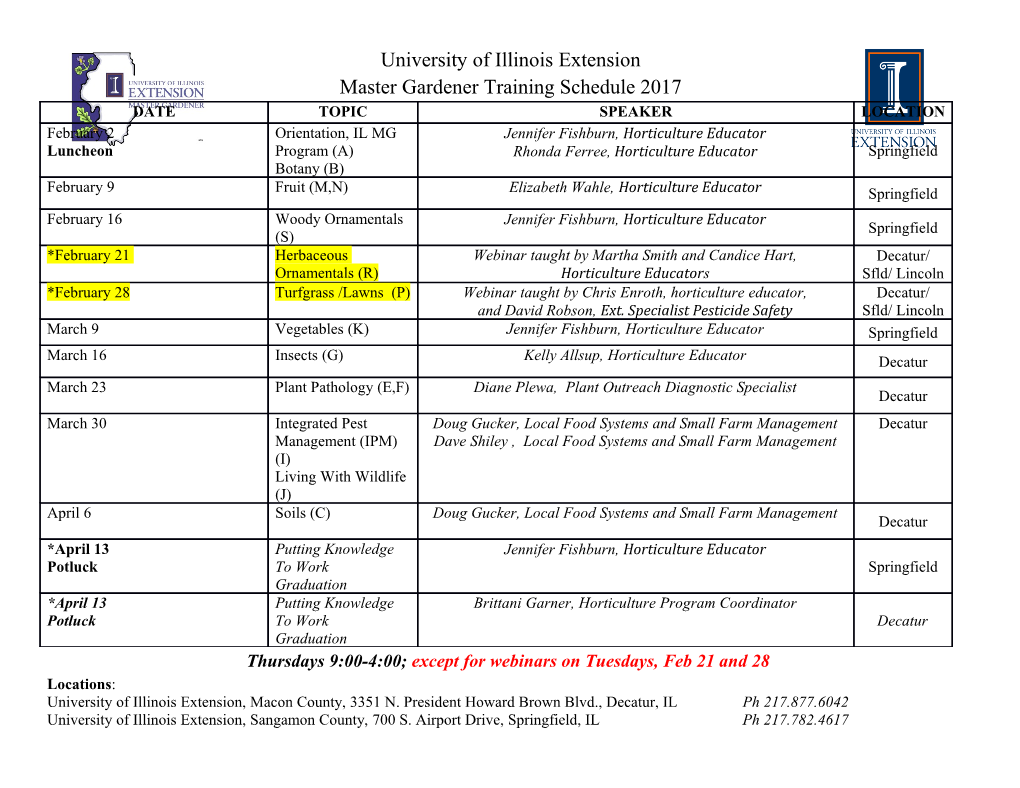
<p> Back to the Future?</p><p>Manuscript title: Back to the Future? Algorithms and equipment vs. simplicity and common sense in physical activity measurement.</p><p>Author: David A. Rowe</p><p>Address for correspondence:</p><p>David A. Rowe</p><p>School of Psychological Sciences and Health, </p><p>University of Strathclyde Jordanhill Campus, </p><p>76 Southbrae Drive, </p><p>Glasgow G13 1PP, </p><p>Scotland, U.K.</p><p>Contact details: </p><p>Email: [email protected]</p><p>Telephone: 44 (0)141 950 3712</p><p>1 Manuscript blind title page</p><p>Manuscript title: Back to the Future? Algorithms and equipment vs. simplicity and common sense in physical activity measurement.</p><p>Key words: Physical activity, technology, validity, statistics Back to the Future?</p><p>Abstract</p><p>This opinion paper establishes the importance of measuring physical activity in kinesiology and public health research, and describes recent developments leading to more technologically advanced instruments yielding more complex types of physical activity data. While this may seem desirable, because physical activity is a complex construct, the purpose of this paper is to warn against pursuing a “Holy Grail” of complexity in measurement tools and data arrays. Excessive complexity carries several disadvantages, which are described in the paper. Perhaps the best approach is that used by Marty McFly, the main character in the Hollywood blockbuster Back to the Future. In the movie, a combination of modern technology and old-fashioned methods allows Marty to travel through time to return to the future. Using this analogy, a case is made for deciding which method or combination of methods is needed for a given research purpose, and for recognising that sometimes, simpler is better.</p><p>“Don't worry. As long as you hit that wire with the connecting hook at precisely 88 </p><p> miles per hour the instant the lightning strikes the tower... everything will be fine” </p><p>- Doc to Marty McFly in the movie Back to the Future</p><p>This paper, written in tandem with a presentation to the 23rd International Sport Science Congress (Rowe,</p><p>2011), comments on the current state of physical activity measurement, its recent direction and offers a perhaps unorthodox suggestion for its future direction. In looking ahead to the future, I will incorporate a look back at the past, hence the title of the paper. For movie fans, the title (and the beginning quote) will be familiar but an explanation of the seemingly contradictory title, and its connection to the movie, will become clear later in the paper.</p><p>Background – the importance of measuring physical activity</p><p>The measurement of physical activity has become extremely important to kinesiology and public health research in recent years, as evidenced by several conferences and symposia dedicated specifically to the topic </p><p>(e.g., Granat, 2011; Mahar & Ainsworth, 2000; Troiano, 2005) and journal special issues (e.g., Montoye, 2000). </p><p>The incidence of morbidity and mortality from inactivity-related diseases has increased over the past century </p><p>(Physical Activity Guidelines Advisory Committee, 2008), and the cost to governments of inactivity-related health problems is considerable (Chenoweth & Leutzinger, 2006). Concern with the public health implications </p><p>3 Back to the Future? of inactivity is reflected in trends in the amount of published physical activity-related research. For example, a </p><p>PubMed search of journal articles including the term “physical activity” in the article title yielded 659 articles published between 1981 and 1990, 2,178 published between 1991 and 2000, and 8,492 published between 2001 and 2010. The latter two figures represent an increase of 230% and a subsequent increase of 289%, respectively.</p><p>Despite the substantial knowledge base linking physical activity to health (Warburton, Nicol, & Bredin, 2006), participation in physical activity has declined, attributed to factors such as an increase in mechanisation, increases in sedentary employment such as office work, and the ubiquity of passive electronic entertainment options such as television, computers, and video games (Vandewater, Shim, & Caplovitz, 2004).</p><p>Research into health-enhancing physical activity incorporates a variety of paradigms. These include a) epidemiological investigations to understand the relationships between physical activity and health outcomes </p><p>(e.g., Owen, Nightingale, et al., 2010); b) large-scale surveys to determine the prevalence of physical activity at the population level (e.g., Troiano, 2007); c) cross-sectional and longitudinal comparisons to determine differences in physical activity between populations or across time (e.g., Ferreira, Twisk, Van Mechelen, </p><p>Kemper, & Stehouwer, 2005); d) controlled trials to determine the efficacy and effectiveness of physical activity interventions for improving health, reducing disease risk, and aiding rehabilitation (e.g., Kirk, Barnett, Leese, & </p><p>Mutrie, 2009); and e) correlation studies to investigate psychological and environmental determinants of current physical activity (e.g., Churangsarit & Chongsuvivatwong, 2011). Parenthetically, increasing evidence points to the independent effect of sedentary behavior (long periods of sitting and lying) on health (Owen, Healy, </p><p>Matthews, & Dunstan, 2010). Although the importance of sedentarism is therefore clearly important, and sedentarism and physical activity are related behaviors, sedentarism is a different construct than physical activity and will not be discussed in this paper.</p><p>The measurement of physical activity poses multiple challenges, mostly stemming from the complexity of the construct itself. Physical activity is most commonly defined as, “any bodily movement produced by skeletal muscles that results in energy expenditure” (Caspersen, Powell, & Christensen, 1989, p. 126). Although accurate, this classical definition belies the complexity of physical activity. It is a somewhat unique construct in kinesiology in that it incorporates behavioral, physiological, and biomechanical principles. To adequately capture physical activity, one must describe all of its multiple components, or dimensions, some of which are shown in Table 1. The first component is frequency (how often it is done in a given time period, or how many </p><p>“bouts” are completed, e.g., five times per week, or four bouts in one day). The second component is intensity </p><p>(the rate of energy expenditure, sometimes expressed in absolute terms, and sometimes expressed relative to a </p><p>4 Back to the Future?</p><p> maximal level or resting level, e.g., 3 METs or 50% VO2max). The third components, duration, is the length of time spent being physically active in a given bout, or over a given time period, e.g., 30 minutes each day, or a </p><p>10-minute bout. Mode is the selected type of activity, described either in generic terms (aerobic activity, or team games) or more specifically (jogging, or baseball). These four dimensions are familiar to many kinesiologists as the foundation components of an exercise prescription (FITT - frequency, intensity, time, type; </p><p>American College of Sports Medicine, 2010). </p><p>For physical activity promotion purposes, physical activity context is also critical. Context describes the surrounding environment in which physical activity takes place and can provide key information for understanding why an individual participates in physical activity, thereby informing physical activity promotion.</p><p>Context can relate to the physical environment (geographic location, or characteristics of the built environment such as availability and quality of parks), or to the social environment (time of day, presence of significant others, etc.). Other dimensions of physical activity have been derived, sometimes from combinations of the dimensions described above, such as total volume (e.g., number of steps or activity counts per week, or energy expenditure in kcals per day) and time spent in health-enhancing physical activity (usually accepted as being at a moderate intensity or higher). For specific modes of physical activity, other characteristics may be relevant, such as distance covered (walking), or resistance, repetitions and sets (weightlifting). </p><p>Measurement methods</p><p>Physical activity is therefore a complex construct that seemingly demands the use of sophisticated techniques and instruments. In an attempt to capture the complexities of physical activity, researchers and manufacturers have developed an extensive array of instruments, some of which are presented in Table 1. It is beyond the scope of this article to describe each instrument in detail, but further information is readily available in other sources (e.g., Welk, 2002). Perhaps the most evident message contained in Table 1 is that no single instrument serves as a gold standard instrument, defined as an instrument that accurately measures all components of physical activity. Interestingly, doubly-labeled water, which is frequently cited as the gold standard to which other instruments should be compared, measures only one dimension (total volume or energy expenditure)! </p><p>In general, the accuracy of a measurement instrument is related to its cost, convenience, and complexity. </p><p>For example, questionnaires are convenient, relatively inexpensive methods for large-scale population, but are known to have limitations of reliability and validity (Sallis & Saelens, 2000). Conversely, accelerometers have </p><p>5 Back to the Future? gained acceptance as objective, accurate measures of the movement dimension of physical activity, but are expensive and pose logistical challenges such as participant and researcher burden.</p><p>Not surprisingly, the increasing importance of physical activity measurement has generated an extensive, sometimes confusing array of new instruments of all types. A Google Shopping search for “pedometer” for example, produced 7,870 results. Similarly, the choice of physical activity questionnaires is considerable (see, for example, Kriska & Caspersen, 1997). The exponential growth in new technologies for objective measurement of physical activity belies a long history, however. The most basic of ambulatory physical activities, walking, was measured in ancient Roman times – the distance of one mile derives from the Latin </p><p>“milia passuum”, equivalent to one thousand paces of a legion of Roman soldiers at regular marching speed (a </p><p>Roman mile was in fact 1400 meters). Someone must have been counting those steps somehow! Several distinguished people including Leonardo DaVinci and Thomas Jefferson have been credited with designing early mechanical pedometers based on a ratchet mechanism, and examples of such instruments dating back to the 1800s can be found in museums and antique shops. In the late 20th century, pedometers were developed further to include spring-loaded lever-arm technology and an electrical switch plate to register a contact with each step (the Yamax company was one of the pioneers of mass-producing this design). More recently, piezoelectric strain gauges have enabled the use of accelerometer technology to more accurately measure steps taken during physical activity (Bassett, Mahar, Rowe, & Morrow, 2008).</p><p>Looking back at the science of measuring physical activity</p><p>Paradoxically, while many complex technologies are currently marketed for personal or commercial use, the measurement of physical activity for more scientific purposes such as physical activity epidemiology is rooted in simpler methods. Jeremy Morris, for example, used occupational classification as a relatively crude measure of daily activity in order to demonstrate an association with cardiovascular health in a series of landmark studies conducted in the United Kingdom (Morris, Heady, Raffle, Roberts, & Parks, 1953). Perhaps the most well-known of these studies compared coronary heart disease in bus drivers (who sat all day at work) to bus conductors (who walked up and down the stairs of the bus, collecting fares). Interestingly, almost 60 years later, Morris’s research is recognised as presenting perhaps the first evidence of the detrimental effects of sedentarism (long periods spent sitting) on health.</p><p>At around the same time that Morris was collecting data on some 25,000 bus drivers and conductors, in the United States of America, the National Heart, Lung and Blood Institute and Boston University began an ambitious longitudinal study of heart health in 5,209 inhabitants of Framingham, Massachusetts that continues </p><p>6 Back to the Future?</p><p>(and has grown to include multiple generations of the original sample) to this day. Part of the Framingham Heart</p><p>Study was a basic 24-hour recall of physical activity that was associated with coronary heart disease (Kannel, </p><p>1967). Interestingly, the concluding paragraph stated that “Prospective epidemiologic studies are hampered by inadequate methods for assessing physical activity ...” (p. 811).</p><p>While the work conducted by Morris, and within the Framingham Heart Study, is recognised as providing landmark early evidence of the link between physical activity and disease prevention, the types of measurement methods used in these studies are criticized for their measurement limitations (Sallis & Saelens, </p><p>2000). Consequently, recent years have seen the development of more complex and elegant methods for monitoring physical activity, and a substantial shift towards objective motion sensors (pedometers and accelerometers) as the “method of choice”, rather than subjective methods such as questionnaires and diaries. In the remainder of this paper, I will focus on the wisdom of this move towards technology as the future of physical activity measurement.</p><p>Even in large scale surveys such as the National Health and Nutrition Evaluation Survey and the </p><p>European Youth heart Study, accelerometer protocols are now used (Troiano, 2007). Perhaps the most striking development arising from this relatively recent wave of research is the realisation that previous questionnaire methods may have led to gross overestimates of population levels of physical activity (Troiano et al., 2008). The capabilities of existing technology are also much-improved compared to technologies of only 10 years ago, leading to longer battery life, greater data storage capacity, and reduction in costs due to mass production.</p><p>More recent developments include the use of multiple technologies in combination (Corder, Brage, </p><p>Wareham, & Ekelund, 2005), wearable technology such as smart clothes and portable cameras to capture physical activity context (Bonato, 2005), incorporation of accelerometry and geographical positioning systems into mobile phone technology (Kiukkonen, Blom , Dousse , Gatica-Perez, & Laurila, 2010), the use of branched regression equations (Crouter, Clowers, & Bassett, 2006) and artificial neural networks (Staudenmayer, Pober, </p><p>Crouter, Bassett, & Freedson, 2009). </p><p>A downside of the move towards motion sensors as the method of choice is that they are insensitive to mode of physical activity (they cannot detect what type of physical activity the wearer is engaging in), but paradoxically are dependent on mode (interpretation of pedometer and accelerometer data differs depending on the type of activity). This is due to the different relationship between gross body acceleration and energy expenditure, or physical activity intensity, during rhythmic ambulatory activities (walking, running, dancing, etc.) and other physical activity modes that are arrhythmic, incorporate upper body work, and/or involve </p><p>7 Back to the Future? minimal acceleration at the hip (lifestyle activities such as household tasks and gardening, cycling, kayaking, weightlifting, etc.). This is demonstrated by a series of studies conducted during the late 1990s and early 2000s, testing linear regression equations under conditions of ambulatory physical activity (e.g., Freedson, Melanson, </p><p>& Sirard, 1998), lifestyle activities (e.g., Swartz, et al., 2000) and combinations of both (e.g., Hendelman, </p><p>Miller, Baggett, Debold, & Freedson, 2000). In brief, from this line of research it became clear that equations developed on ambulatory activity differ from equations developed on lifestyle activities, and that equations developed on combinations of these types of activities tend to have low accuracy for predicting physical activity energy expenditure.</p><p>Several unique and innovative solutions to this problem have been developed. Crouter et al. (2006) developed a branched equation based on epoch-to-epoch variability (coefficient of variation) to detect the mode of activity and then employ either an ambulatory activity equation, or a lifestyle equation. More recently, </p><p>Freedson and colleagues have used pattern recognition or “machine learning” approaches to processing accelerometer data in order to more accurately predict energy expenditure across varied modes and, separately, to classify activity mode (Staudenmayer, et al. 2007). Lastly, combined heart rate and accelerometry data have been used in conjunction with a branched regression equation method to improve the accuracy of estimating energy expenditure during combined modes of physical activity (Thompson, Batterham, Bock, Robson, & </p><p>Stokes, 2006).</p><p>These innovative ideas for merging technologies and using complex data processing techniques should be commended for advancing our ability to generate and interpret movement data in ways that are elegant and scientifically astute. They will presumably lead to a “trickle-down effect” of the technologies and signal processing methods to more widely-accessible instruments, as has been seen in the progression from lever-arm, </p><p>“single-day data” pedometers to pedometers that incorporate accelerometer technologies and multiple-day data storage capabilities.</p><p>So are we heading towards a rosy future?</p><p>It would appear from current trends that the future of measuring physical activity lies in gadgetry (or technological complexity) and equations (or statistical sophistication). However, in the rest of this paper I will make a case for going “Back to the Future” (or perhaps “Forward to the Past”?). In the movie of the same name,</p><p>Marty McFly (played memorably by Michael J. Fox) travelled back in time to 1955 with the help of a converted </p><p>DeLorean sports car, a supply of plutonium, and the ingenuity of his friend Dr. Emmett "Doc" Brown. Marty subsequently found himself stuck in the past without the necessary plutonium to return “back to the future” but </p><p>8 Back to the Future? was able to obtain the necessary 1.21 gigawatts of power by harnessing a lightning bolt. In this way, he was able to go back to the future using a mixture of modern technology (the DeLorean and a “flux capacitor”) and 30- year-old technology (a lightning bolt, clock tower, length of wire, and a hook). The future of physical activity measurement may also lie partly in our past, and the gadget- and data-sophistry direction we currently seem to be taking may involve pitfalls that, to my knowledge, have not been recognized in the published literature. The availability of multiple, constantly changing methods and paradigms for improving physical activity measurement present us with a variety of problems that I have highlighted below via three questions.</p><p>Three important questions leading us “Back to the Future”</p><p>Is the tail wagging the dog?</p><p>In this question, the tail refers to the manufacturers of physical activity instruments, and the dog refers to the researchers wishing to measure physical activity. In a perfect world, researchers would be able to dictate their needs and manufacturers would respond to those needs. In fairness, some manufacturers do consult with researchers and are responsive to researchers’ requests for improvements and adaptations. However, the tenuous relationship between the commercial needs of the manufacturer and the scientific needs of the researcher is inescapable. An example of this situation is the existence of proprietary (private, unavailable to the consumer) equations built into instruments to convert the raw acceleration signal into arbitrary, or at least instrument- specific, activity counts. Put simply, two accelerometers from different manufacturers will produce very different activity counts when worn by the same person during the same activity.</p><p>A potential conflict of interest subsequently exists when universities or other government-subsidized institutions conduct reliability and validity studies in conjunction with manufacturers (especially if the research is also sponsored by the manufacturers), often at a cost that would likely be much higher if the manufacturer were to conduct the study themselves, or via a commercial research company. Additionally, in these situations, ownership of data may be ambiguous, and the potentially adverse effect on the researcher-manufacturer relationship of publishing adverse results is evident.</p><p>Are we putting the cart before the horse?</p><p>In this question, the cart is the conducting of scientific research, often at great public and personal expense to the researcher and his institution, and the horse is the necessary pre-market development and quality- checking to ensure that the instrument is useable and the data are interpretable. I am concerned at the growing number of new manufacturers, new devices and new device models that are available in the Exhibitor Halls at </p><p>9 Back to the Future? international scientific conferences and the potential effect of this “equipment explosion” on the science of investigating physical activity.</p><p>I am aware of at least three research situations to illustrate the hazards of the “cart before the horse” situation, each associated with a different manufacturer (all of which will remain anonymous). The first involved the purchase of multiple units of a new accelerometer that was based on a previous trustworthy but discontinued model, for use in an externally funded 12-month school-based physical activity intervention. </p><p>Ironically, the validity of the accelerometer was irrelevant, because much of the data was inaccessible due to a problem with the accelerometer-download dock interface. Despite assertions to the contrary by the manufacturer, several colleagues at other institutions have reported similar problems and despite the popularity of the instrument at launch, very few studies have been published in a period of almost 10 years since the unit first appeared on the market.</p><p>In the second situation, a researcher was contracted to investigate the validity of another new accelerometer, marketed by a company with other successful, validated physical activity instruments. During the course of the validation study, several faults appeared in the instrument that were corrected by the manufacturer, but eventually the instrument was discontinued. The validation study was subsequently unpublishable, despite the fact that sales of the unit were reported as high, and similar to the previous example, no published studies have appeared using the instrument in the 5 years since its release to market.</p><p>The final example is of a newly-developed geographic position system, again based on a previously established model. Marketing materials promised higher accuracy, greater sampling frequency, greater storage capacity, and longer battery life than existing models. Twenty of these units were purchased for use in an externally funded 18-month school-based study to investigate the effectiveness of an active commuting intervention. Within a relatively brief period, it became evident that the battery life extended barely beyond 24 hours, and that sampling frequency was considerably below the standard stated in the technical specifications. In a subsequent controlled trial of the instrument’s functionality, these adverse findings were confirmed, as well as the fact that location error was more than twice the stated standard. Perhaps fortunately, route detection was a secondary outcome measure of the intervention study, and the use of GPS was eventually discontinued because of the limitations of the instrument. </p><p>The negative consequences of losing data or obtaining bad data due to the types of problems described above are substantial. The extent to which the “cart before the horse” problem adversely affects research is </p><p>10 Back to the Future? probably underrecognized, by virtue of the fact that poor data quality or significant loss of data render the research unpublishable.</p><p>Are we talking the same language?</p><p>The “language” in the final question refers to the metric with which instruments convey information about physical activity, and the terminology that researchers use to interpret this information. The development of a coherent body of knowledge about physical activity and health is dependent on a) instrument consistency, b) consistency in the algorithms used to convert raw data to meaningful outcome scores, and c) consistency in the terminology used by researchers to interpret those outcome scores.</p><p>The consistency with which raw physical activity data are reproduced may be influenced by the use of different devices (“interinstrument”), of different models of the same device (“intermodel”), of different generations of the same model (“intergenerational”), and/or of different units of the same model and same generation (“interunit”). Even among relatively basic instruments such as pedometers, considerable differences in step counts have been found under controlled conditions when using different makes and models, for example</p><p>(Schneider, Crouter, Lukajic, & Bassett, 2003). Among accelerometers also, there is evidence that raw activity counts may differ between “generations” of the same device (e.g., Kozey, Staudenmayer, Troiano, & Freedson, </p><p>2010). The instruments and manufacturers featured in the cited studies have not been selected purposively as being more prone to inconsistency than other instruments and manufacturers; indeed the scrutiny of their consistency in the public domain is to be commended, as this type of evidence allows researchers to conduct a more informed interpretation of their physical activity data. </p><p>Beyond the consistency of raw data, the use of standard algorithms and metrics for converting raw data is uncommon. Except for minor differences in processing parameters (e.g., sampling frequency, filtering criteria), the raw acceleration signal from different instruments should be relatively similar across the normal range of bodily movements involved in physical activity (especially ambulatory physical activities). The methods for processing the raw signal, however, vary considerably among manufacturers. This results in manufacturer- specific “activity counts” that have no immediate relevance to common metrics of movement such as energy cost in kJ, or speed of ambulatory motion. Where instruments do provide output such as kcals, these are usually based on proprietary equations that are unavailable to the research community. Consequently, instrument- specific equations must be developed by researchers in order to translate the arbitrary counts into a meaningful metric such as VO2, METs, kcals, or some other physical activity-related outcome variable. This has led to confusion in the field, as different equations have been developed by different researchers, sometimes under </p><p>11 Back to the Future? different conditions, and studies. Examples of four equations for predicting energy expenditure in children are presented in Table 2. The difference between the lowest and highest MET estimate ranges from 69% to 84%, depending on the intensity expressed in activity counts/min. Similar discrepancies exist in adult equations, as described earlier. </p><p>Fortunately, some manufacturers now make the raw acceleration signal accessible to the user (e.g., the most recent generations of Actigraph; Actigraph LLC, Pensacola FL) which, if standardized across manufacturers, would allow for the development of data-processing methods that would be applicable to all devices. Until this becomes standard practice among instrument manufacturers, and uniformly-accepted algorithms are used, the existence of model-specific activity counts and different cutpoints remains problematic.</p><p>When determining the prevalence of children (or adults) meeting national recommendations for physical activity for example, the cutpoint discrepancies shown in Table 2 would lead to different conclusions regarding the extent to which children meet recommended levels of physical activity. Not only would the results be inconsistent, but currently it is not clear which cutpoints provide valid results (accurate estimates of population prevalence, and accurate determination whether an individual meets recommended physical activity levels). </p><p>Sallis & Saelens (2000) introduced the concept of absolute validity (the degree of exactness with which the scores reflect each person’s true level on the underlying construct). Similarly, Andersen, McCullagh, & Wilson, </p><p>(2007) stressed the dangers of arbitrary metrics – though their point was made with respect to psychological instruments, the same line of reasoning applies to measures of physical activity. These issues notwithstanding, in some circumstances absolute validity may be less important. Correlational designs (e.g., behaviour change model-testing via path analysis) may be dependent on ordinal accuracy (what Sallis and Saelens called relative validity) , but absolute validity, or accurate translation into real-world metrics may not be crucial in these situations.</p><p>Also related to language and terminology, Kelley (1927) identified two fallacies of measurement. The </p><p>“jingle fallacy” describes the misconception that two instruments with similar names measure the same construct, and the “jangle fallacy” describes the converse assumption (that instruments with different names measure different constructs). The important lesson underlying the jingle-jangle fallacies is to take care in using construct labels such as “physical activity,” “intensity,” and “energy expenditure.” When reading and writing about physical activity, we should consider carefully whether the label in question appropriately reflects the construct, and whether the label means the same thing when it is used in the context of different instruments, by different people, or in different studies. </p><p>12 Back to the Future?</p><p>For example, consider the question, “What is ‘health-enhancing’ physical activity?” Most public health recommendations would define this as any physical activity that is performed at moderate intensity. </p><p>Confusingly, the definition of moderate intensity is not consistent across sources. The ACSM guidelines </p><p>(American College of Sports Medicine, 2010) describe moderate intensity as 3-6 METs, but also as 40%-59% </p><p>VO2 reserve (p. 5 and p. 153). Similarly, Norton, Norton, and Sadgrove (2010) described moderate intensity as </p><p>3-6 METs, but also as 55%-69% maximal heart rate (p. 500). Setting these differences aside, the generally consistent definition in most public health recommendations is that moderate intensity corresponds to physical activity of at least 3 METs. However, the translation of this into even the basic metric of steps is not consistent across instruments. For example, the New Lifestyles NL-1000 pedometer (New Lifestyles Inc., Lees Summit, </p><p>MO) separates “MVPA time” where this is defaulted to a walking pace that corresponds to 3.6 METs and above, although the threshold can be adjusted to 2.9 METs. Some models of Omron pedometers (Omron </p><p>Healthcare, Kyoto, Japan) provide output on what they term as “aerobic time” and “aerobic steps”, corresponding to any physical activity conducted at a cadence greater than 60 steps/min. Conversely, the Polar </p><p>Active (Polar Electro Oy, Kempele, Finland) watch tracks time spent ≥ 70 steps/min. Interestingly, a recent series of controlled studies of the metabolic cost of walking indicate that for the general population, a cadence of</p><p>100 steps/min corresponds to moderate intensity (3 METs; Tudor-Locke & Rowe, in press).</p><p>From this brief set of examples, it is clear that researchers do not necessarily speak the same language when using physical activity terminology. From this and previous sections, it is also evident that different manufacturers (and even different instruments from the same manufacturers) use different metrics (the output is expressed in different arbitrary activity counts, that then must be validated by researchers) and different mechanisms and signal processing across different generations of the same instrument (potentially resulting in different outputs from the same physical activity). Even when the same basic metric is used (steps), different pedometers generate different outputs, especially at slower and/or faster speeds, in overweight or obese populations, and in clinical populations with asymmetric or shuffling gait patterns.</p><p>One could also ask whether researchers speak the same physical activity language as “real” (non- research) people. Does the general population understand, for example, how to interpret accelerometer counts? </p><p>Are terms like ‘moderate’ and ‘vigorous’ understood by the general population? Recent physical activity recommendations more explicitly recognize that both moderate and vigorous physical activity are beneficial to health, that fewer minutes of vigorous activity have a similar health benefit to longer duration, moderate- intensity physical activity, and that moderate and vigorous physical activity can be combined in order to </p><p>13 Back to the Future? enhance health, in various permutations. For example, cardiovascular health benefits can be obtained from 30 minutes of moderate intensity on 5 days per week, from 20 minutes of vigorous physical activity on 3 days per week, or from an appropriate combination (e.g., moderate intensity for 30 minutes twice during a week plus vigorous activity for 20 min on two other days; Haskell et al. 2007). While scientifically sound, the interpretation of these guidelines may not constitute a simple (easily followed or monitored) public health message.</p><p>The case for going “Back to the Future”</p><p>In summary, the past 10-20 years have seen an exponential rise in the number of available instruments, the instrument capabilities, and the complexity of interpreting “basic output” physical activity data – steps, raw acceleration, or activity counts. The signs are that this trend will continue. Certainly, there are some exciting developments in terms of post-processing accelerometer data, and the scientific quality of this research is indisputable. However, I believe we should be cautious about pursuing greater technological and statistical complexity as a “Holy Grail” for valid physical activity data collection and analysis. Consider the early research by Morris, and of the Framingham Study described earlier, for example. Both showed convincing evidence of a link between physical activity and various health outcomes, thus demonstrating criterion-related validity evidence for even very crude measures of physical activity (Rowe & Mahar, 2004). As we head into the future, we should be wary of dispatching existing measures and methods in favour of the latest devices. A potential side-effect could be the creation of a research underclass, without the resources to keep up with the perceived need to purchase the most recent equipment, for example. </p><p>Recommendations</p><p>In order to capitalize on recent developments but avoid the pitfalls described in this paper, the following recommendations are offered for physical activity researchers:</p><p> Apply Occam’s Razor when measuring physical activity (Blumer, Ehrenfeucht, Haussler, & Warmuth, </p><p>1987). Use the minimal level of complexity in measuring and analysing the data that can be done </p><p> without losing important information. Potential advantages of this principle are:</p><p> o Simpler, less expensive equipment enables the use of larger sample sizes and potentially leads </p><p> to more studies, by more research groups, therefore contributing to the critical mass of </p><p> knowledge;</p><p> o Simpler data leads to more understandable metrics (and thus a more accessible public health </p><p> message), and simpler and less ambiguous data processing; and</p><p>14 Back to the Future?</p><p> o Standardization of metrics enables comparability of data and results across studies.</p><p> The research community-manufacturer relationship should be more transparent and co-operative, for </p><p> example in the following ways:</p><p> o Researchers should appeal to manufacturers to enable downloading of raw acceleration data;</p><p> o Where proprietary (instrument-specific) algorithms are inbuilt for post-processing raw </p><p> acceleration data, manufacturers should make the algorithm available to researchers for </p><p> adaptation where necessary;</p><p> o Any research and development conducted on behalf of (or partially funded by) manufacturers </p><p> should be preceded by a clear agreement that the researcher owns the right to publish the data </p><p> regardless of the outcome; </p><p> o To avoid the appearance of impropriety, it may be advisable to have data analysis conducted </p><p> by an impartial third party; </p><p> o The research community should lobby manufacturers to only change mechanisms and </p><p> algorithms where absolutely necessary; and </p><p> o Sufficient research and development should be conducted independently prior to bringing new</p><p> instruments to market.</p><p> Researchers should use common metrics to report physical activity intensity (kcal, kJ, METs) or at </p><p> least provide the means to convert the reported data into a common metric. </p><p>References</p><p>American College of Sports Medicine. (2010). ACSM's guidelines for exercise testing and prescription (8th ed.).</p><p>New York: Williams & Wilkins.</p><p>Andersen, M. B., McCullagh, P., & Wilson, G. J. (2007). But what do the numbers really tell us? Arbitrary </p><p> metrics and effect size reporting in sport psychology research. Journal of Sport & Exercise Psychology, 29, </p><p>664-672.</p><p>Bassett, D. R., Jr., Mahar, M. T., Rowe, D. A., & Morrow, J. R., Jr. (2008). Walking and measurement. </p><p>Medicine and Science in Sports and Exercise, 40, S529-S536.</p><p>Blumer, A., Ehrenfeucht, A., Haussler, D. & Warmuth, M. K. (1987). Occam’s razor. Information Processing </p><p>Letters, 24, 377-380. </p><p>Bonato, P. (2005). Advances in wearable technology and applications in physical medicine and rehabilitation. </p><p>Journal of NeuroEngineering and Rehabilitation, 2, 2.</p><p>15 Back to the Future?</p><p>Burton, F., Brage, S., Chastin, S., Penpraze, V., & Rowe, D. A. (in review). The British Association of Sport </p><p> and Exercise Sciences expert statement on objective measurement of physical activity. (submitted to the </p><p>Sport and Exercise Scientist).</p><p>Caspersen, C. J., Powell, K. E., & Christenson, G. M. (1985). Physical activity, exercise, and physical fitness: </p><p>Definitions and distinctions for health-related research. Public Health Reports, 100, 126-131.</p><p>Chenoweth, D., & Leutzinger, J. The economic cost of physical inactivity and excess weight in American </p><p> adults. Journal of Physical Activity and Health, 3,148-163</p><p>Churangsarit, S., & Chongsuvivatwong, V. (2011). Spatial and social factors associated with transportation and </p><p> recreational physical activity among adults in Hat Yai City, Songkhla, Thailand. Journal of Physical Activity</p><p> and Health, 8, 758 -765</p><p>Corder, K., Brage, S., Wareham, N. J., & Ekelund, U. (2005). Comparison of PAEE from combined and </p><p> separate heart rate and movement models in children. Medicine and Science in Sports and Exercise, 37, </p><p>1761–1767.</p><p>Crouter, S. E., Clowers, K. G., & Bassett, D. R., Jr. (2006). A novel method for using accelerometer data to </p><p> predict energy expenditure. Journal of Applied Physiology, 100, 1324-1331.</p><p>Ferreira, I., Twisk, J. W. R., Van Mechelen, W., Kemper, H. C. G., & Stehouwer, C. D. A. (2005). Development</p><p> of fatness, fitness, and lifestyle from adolescence to the age of 36 years: Determinants of the metabolic </p><p> syndrome in young adults. The Amsterdam Growth and Health Longitudinal Study. Archives of Internal </p><p>Medicine, 165, 42-48.</p><p>Freedson, P. S., Melanson, E., & Sirard, J. (1998). Calibration of the Computer Science and Applications, Inc. </p><p> accelerometer. Medicine and Science in Sports and Exercise, 30, 777–781.</p><p>Granat, M. H. (Ed.). (2011). Scientific program for the 2nd International Conference on Ambulatory Monitoring </p><p> of Physical Activity and Movement, Glasgow, U.K.</p><p>Haskell, W. L., Lee, I-M., Pate, R. R., Powell, K. E., Blair, S. N., Franklin, B. A., et al. (2007). Physical activity</p><p> and public health: Updated recommendation for adults from the American College of Sports Medicine and </p><p> the American Heart Association. Circulation, 116, 1081-1093.</p><p>Hendelman, D., Miller, K., Baggett, C., Debold, E., & Freedson, P. (2000). Validity of accelerometry for the </p><p> assessment of moderate intensity physical activity in the field. Medicine and Science in Sports and Exercise,</p><p>32(9, Suppl.), S442–S449. </p><p>16 Back to the Future?</p><p>Kannel, W. B. (1967). Habitual level of physical activity and risk of coronary heart disease: The Framingham </p><p>Study. Canadian Medical Association Journal, 96, 811-812.</p><p>Kelley, T.L. (1927). Interpretation of educational measurements. Yonkers-on-Hudson, NY: World Books. </p><p>Kirk, A., Barnett, J., Leese, G., & Mutrie, N. (2009). A randomised trial investigating the 12 month changes in </p><p> physical activity and health outcomes following a physical activity consultation delivered by a person or in </p><p> written form in Type 2 diabetes: TIME2ACT. Diabetic Medicine, 26, 293-301 </p><p>Kiukkonen, N., Blom, J., Dousse, O., Gatica-Perez, D., & Laurila, J. (2010). Towards rich mobile phone </p><p> datasets: Lausanne data collection campaign. In: Proceedings of the ACM International Conference on </p><p>Pervasive Services, Berlin. Retrieved 08-03-2011 from: </p><p> http://publications.idiap.ch/downloads/papers/2010/Kiukkonen_ICPS,BERLIN,2010_2010.pdf. </p><p>Kozey, S. L., Staudenmayer, J. W., Troiano, R. P., & Freedson, P. S. (2010). Comparison of the ActiGraph 7164</p><p> and the ActiGraph GT1M during self-paced locomotion. Medicine and Science in Sports and Exercise. 42, </p><p>971-976.</p><p>Kriska, A. M., & Caspersen, C. J. (Eds.). (1997). A collection of physical activity questionnaires for health-</p><p> related research (MSSE Special Issue). Medicine and Science in Sports and Exercise. 29(6, Suppl.), S3-</p><p>S205.</p><p>Mahar, M. T., & Ainsworth, B. E. (Eds.). (2000). Measurement of physical activity: The Cooper Institute </p><p>Conference Series. Research Quarterly for Exercise and Sport, 71(Suppl. 2), S1-S158.</p><p>Mattocks, C., Leary, S., Ness, A., Deere, K., Saunders, J., Tilling, K., et al. (2007). Calibration of an </p><p> accelerometer during free-living activities in children. International Journal of Pediatric Obesity, 2, 218-226</p><p>Montoye, H. (2000). Introduction: Evaluation of some measurements of physical activity and energy </p><p> expenditure (MSSE Special Issue). Medicine and Science in Sports and Exercise. 32(9, Suppl.), S439-S516.</p><p>Morris, J. M., Heady, J. A., Raffle, P. A. B., Roberts, C. G., & Parks, J. W. (1953). Coronary heart disease and </p><p> physical activity of work. Lancet, 262(6795), 1053-1057.</p><p>Norton, K., Norton, L., & Sadgrove, D. Position statement on physical activity and exercise intensity </p><p> terminology. Journal of Science and Medicine in Sport, 13, 496-502.</p><p>Owen, C. G., Nightingale, C. M., Rudnicka, A. R., Sattar, N., Cook, D. G. Ekelund, U., et al. (2010). Physical </p><p> activity, obesity and cardiometabolic risk factors in 9- to 10-year-old UK children of white European, South </p><p>Asian and black African-Caribbean origin: The Child Heart And health Study in England (CHASE). </p><p>Diabetologia, 53, 1620–1630.</p><p>17 Back to the Future?</p><p>Owen,N., Healy, G. N., Matthews, C. E., & Dunstan, D. W. (2010). Too much sitting: The population health </p><p> science of sedentary behavior. Exercise and Sport Science Reviews, 38, 105-113.</p><p>Physical Activity Guidelines Advisory Committee. (2008). Physical Activity Guidelines Advisory Committee </p><p>Report, 2008. Washington, DC: U.S. Department of Health and Human Services.</p><p>Puyau, M. R., Adolph, A. L., Vohra, F. A., & Butte, N. F. (2002). Validation and calibration of physical activity</p><p> monitors in children. Obesity Research, 10, 150-157.</p><p>Rowe, D. A. (2006). Measuring physical activity. In T. A. Baumgartner, A. S. Jackson, M. T. Mahar, & D. A. </p><p>Rowe. Measurement for evaluation in physical education and exercise science (8th ed., pp. 178-221). </p><p>Boston, MA: McGraw-Hill.</p><p>Rowe, D. A. (2011, August). Back to the Future? Algorithms and equipment vs. simplicity and common sense in</p><p> physical activity assessment. Presented at the 23rd International Sport Science Congress, Daegu, Korea.</p><p>Rowe, D. A., & Mahar, M. T. (2006). Construct validity. In T. Wood & W. Zhu (Eds.), Measurement theory </p><p> and practice in kinesiology (pp. 9-26). Champaign, IL: Human Kinetics.</p><p>Sallis, J. F., & Saelens, B. E. (2000). Assessment of physical activity by self-report: Status, limitations, and </p><p> future directions. Research Quarterly for Exercise and Sport, 71(2, Suppl,), S1-14.</p><p>Schneider, P. L., Crouter, S. E., Lukajic, O. & Bassett, D. R., Jr. (2003). Accuracy and reliability of 10 </p><p> pedometers for measuring steps over a 400-m walk. Medicine and Science in Sports and Exercise, 35, 1779-</p><p>1784.</p><p>Staudenmayer, J., Pober, D., Crouter, S., Bassett, D. R., Jr. & Freedson, P. (2009). An artificial neural network </p><p> to estimate physical activity energy expenditure and identify physical activity type from an accelerometer. </p><p>Journal of Applied Physiology, 107, 1300-1307.</p><p>Swartz, A. M., Strath, S. J., Bassett, D. R., Jr., O’Brien, W. L., King, G. A., & Ainsworth, B. E. (2000). </p><p>Estimation of energy expenditure using CSA accelerometers at hip and wrist sites. Medicine and Science in </p><p>Sports and Exercise, 32(9, Suppl.), S450–S456.</p><p>Thompson, D., Batterham, A. M., Bock, S., Robson, C., & Stokes, K. (2006). Assessment of low-to-moderate </p><p> intensity physical activity thermogenesis in young adults using synchronized heart rate and accelerometry </p><p> with branched-equation modeling. Journal of Nutrition, 136, 1037-1042.</p><p>Treuth, M. S., Schmitz, K., Catellier, D. J., McMurray, R. G., Murray, D. M., Almeida, M. J., et al. (2004). </p><p>Defining accelerometer thresholds for activity intensities in adolescent girls. Medicine and Science in Sports</p><p> and Exercise, 36, 1259-1266.</p><p>18 Back to the Future?</p><p>Troiano, R. P. (2005). A timely meeting: Objective measurement of physical activity. (MSSE Special Issue of </p><p> conference papers from “Objective monitoring of physical activity: Closing the gaps in the science of </p><p> accelerometry”, Chapel Hill, NC, December 2004). Medicine & Science in Sports & Exercise. 37(11, </p><p>Suppl.), S487-S588).</p><p>Troiano, R. P. (2007). Large-scale applications of accelerometers: New frontiers and new questions </p><p>(Commentary). Medicine & Science in Sports & Exercise. 39, 1501.</p><p>Troiano, R. P., Berrigan, D., Dodd, K. W., Mâsse, L. C., Tilert, T., & McDowell, M. (2008). Physical activity in</p><p> the United States measured by accelerometer. Medicine and Science in Sports and Exercise, 40, 181–188.</p><p>Trost, S. G., Way, R., & Okely, A. D. (2006). Predictive validity of three Actigraph energy expenditure </p><p> equations for children. Medicine and Science in Sports and Exercise, 38, 380-387.</p><p>Tudor-Locke, C., & Rowe, D. A. (in press). Using cadence to study free-living ambulatory activity. Sports </p><p>Medicine.</p><p>Vandewater, E. A., Shim, M-S., & Caplovitz, A. G. (2004). Linking obesity and activity level with children’s </p><p> television and video game use. Journal of Adolescence, 27, 71–85.</p><p>Warburton, D. E. R., Nicol, C. W., & Bredin, S. S. D. (2006). Health benefits of physical activity: The evidence.</p><p>Canadian Medical Association Journal, 174, 801-809.</p><p>Welk, G. (Ed). (2002). Physical activity assessments for health related research. Champaign, IL: Human </p><p>Kinetics.</p><p>19 Back to the Future?</p><p>Table 1. Accuracy of different instruments for estimating physical activity dimensions</p><p>Dimension DLW HRM Accelerometer Pedometer GPS Questionnaire Direct observation Frequency - + ++ - + + + Intensity - ++ ++ - + + + Time - +++ +++ - ++ + ++ Mode - - + + + ++ +++ Context - - - - ++ +++ +++ Volume +++ ++ ++ ++ + + + Energy expenditure +++ ++ ++ + - + + Adapted from Rowe (2000) and Burton, Brage, Chastin, Penpraze, & Rowe (in review) Key: Not possible to estimate (-), possible to estimate but with large error (+), possible to estimate with medium level of error (++), possible to estimate with low degree of error (+++). DLW = doubly labeled water; HRM = heart rate monitor; GPS = global positioning system. </p><p>20 Back to the Future?</p><p>Table 2. Estimates of children’s METs from Actigraph accelerometer counts using four published equations</p><p>METs (equation) Cts/min Freedson1 Puyau2 Treuth3 Mattocks4 % difference5 500 2.10 2.40 2.44 3.68 76% 1000 2.60 2.70 2.87 4.38 69% 1500 3.10 3.00 3.29 5.08 70% 2000 3.60 3.30 3.72 5.79 75% 2500 4.11 3.60 4.15 6.49 80% 3000 4.61 3.90 4.58 7.19 84% Notes: 1Freedson et al. (1997), as reported by Trost et al. (2006); 2Puyau, Adolph, Vohra, & Butte (2002); 3Treuth et al. (2004); 4Mattocks, et al. (2007); 5 Difference between lowest and highest estimate, expressed as a percentage of lowest estimate. Freedson and Puyau equations were calculated based on a 13-year old girl, and conversion factors of 1 MET = 1 kcal/kg/hr and 1 kJ = 4.1868 kcal were used for the Puyau and Mattocks equations.</p><p>21</p>
Details
-
File Typepdf
-
Upload Time-
-
Content LanguagesEnglish
-
Upload UserAnonymous/Not logged-in
-
File Pages21 Page
-
File Size-