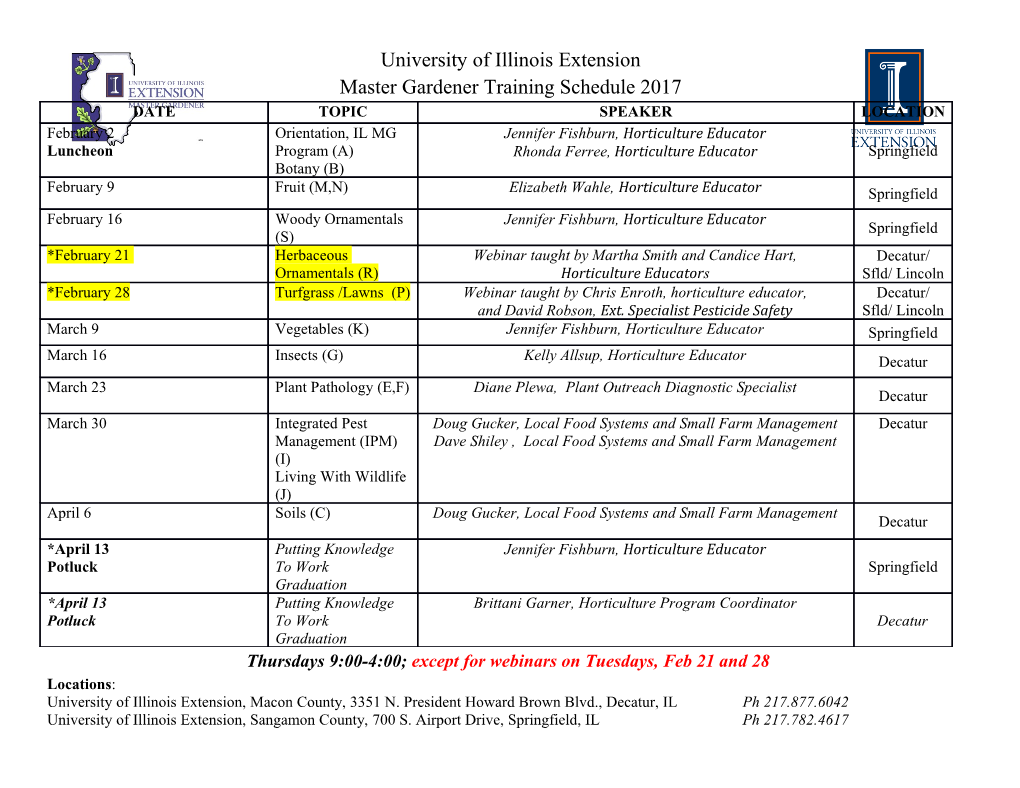
<p> THE DISTRIBUTION OF SAMPLE MEANS </p><p>Inferential statistics:</p><p>Generalize from a sample to a population</p><p>Statistics vs. Parameters</p><p>Why?</p><p>Population not often possible</p><p>Limitation:</p><p>Sample won’t precisely reflect population</p><p>Samples from same population vary</p><p>“sampling variability”</p><p>Sampling error = discrepancy between sample statistic and population parameter</p><p>1 Extend z-scores and normal curve to SAMPLE MEANS rather than individual scores</p><p> How well will a sample describe a population?</p><p> What is probability of selecting a sample that has a certain mean? </p><p> Sample size will be critical</p><p> Larger samples are more representative</p><p> Larger samples = smaller error</p><p>2 THE DISTRIBUTION OF SAMPLE MEANS </p><p>Population of 4 scores: 2 4 6 8 = 5</p><p>4 random samples (n = 2):</p><p>X 1= 4 X 3 = 5</p><p>X 2 = 6 X 4 = 3</p><p> X is rarely exactly </p><p> Most X a little bigger or smaller than </p><p> Most X will cluster around </p><p> Extreme low or high values of X are relatively rare</p><p> With larger n, X s will cluster closer to µ (the DSM will have smaller error, smaller variance) </p><p>3 A Distribution of Sample Means</p><p>X = 4 X = 5 X = 6</p><p>Figure 7-3 (p. 205) The distribution of sample means for n = 2. This distribution shows the 16 sample means obtained by taking all possible random samples of size n=2 that can be drawn from the population of 4 scores (see Table 7.1 in text). The known population mean from which these samples were drawn is µ = 5.</p><p>4 THE DISTRIBUTION OF SAMPLE MEANS</p><p> A distribution of sample means (X ) All possible random samples of size n A distribution of a statistic (not raw scores)</p><p>“Sampling Distribution” of X</p><p> Probability of getting an X , given known and Important properties</p><p>(1) Mean (2) Standard Deviation (3) Shape</p><p>5 PROPERTIES OF THE DSM </p><p> Mean?</p><p>X = </p><p>Called expected value of X</p><p>X is an unbiased estimate of Standard Deviation?</p><p>Any X can be viewed as a deviation from </p><p>X = Standard Error of the Mean </p><p> X = n</p><p>Variability of X around </p><p>Special type of standard deviation, type of “error”</p><p>Average amount by which X deviates from </p><p>6 Less error = better, more reliable, estimate of population parameter</p><p>X influenced by two things:</p><p>(1) Sample size (n)</p><p>Larger n = smaller standard errors</p><p>Note: when n = 1 X = </p><p> as “starting point” for X, </p><p>X gets smaller as n increases</p><p>(2) Variability in population ()</p><p>Larger = larger standard errors</p><p>Note: X = M </p><p>7 Figure 7-7 (p.215) The distribution of sample means for random samples of size (a) n = 1, (b) n = 4, and (c) n = 100 obtained from a normal population with µ = 80 and σ = 20. Notice that the size of the standard error decreases as the sample size increases.</p><p>8 Shape of the DSM?</p><p>Central Limit = DSM will approach a normal dist’n Theorem as n approaches infinity</p><p>Very important! </p><p>True even when raw scores NOT normal! </p><p>True regardless of or </p><p>What about sample size? </p><p>(1) If raw scores ARE normal, any n will do </p><p>(2) If raw scores NOT normal, n must be “sufficiently large” </p><p>For most distributions n 30</p><p>9 Why are Sampling Distributions important?</p><p> Tells us probability of getting X , given & </p><p> Distribution of a STATISTIC rather than raw scores</p><p> Theoretical probability distribution</p><p> Critical for inferential statistics! </p><p> Allows us to estimate likelihood of making an error when generalizing from sample to popl’n</p><p> Standard error = variability due to chance</p><p> Allows us to estimate population parameters</p><p> Allows us to compare differences between sample means – due to chance or to experimental treatment?</p><p> Sampling distribution is the most fundamental concept underlying all statistical tests </p><p>10 WORKING WITH THE </p><p>DISTRIBUTION OF SAMPLE MEANS </p><p>. If we assume DSM is normal . If we know & . We can use Normal Curve & Unit Normal Table! </p><p> z = X x</p><p>Example #1: = 80 = 12</p><p>What is probability of getting X 86 if n = 9?</p><p>11 Example #1b: = 80 = 12</p><p>What if we change n =36</p><p>What is probability of getting X 86 </p><p>12 Example #2:</p><p> = 80 = 12</p><p>What X marks the point beyond which sample means are likely to occur only 5% of the time? (n = 9)</p><p>13 Homework problems: Chapter 7: 3, 10, 11, 17</p><p>14</p>
Details
-
File Typepdf
-
Upload Time-
-
Content LanguagesEnglish
-
Upload UserAnonymous/Not logged-in
-
File Pages14 Page
-
File Size-