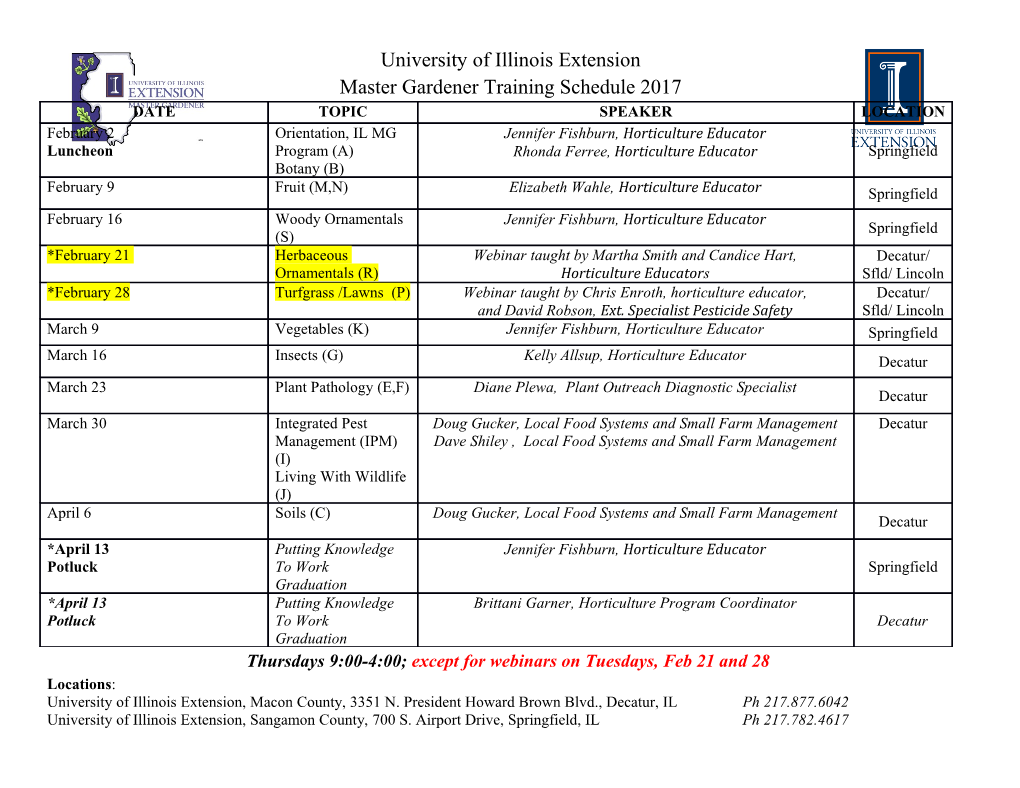
Journal of Mathematical Finance, 2021, 11, 246-254 https://www.scirp.org/journal/jmf ISSN Online: 2162-2442 ISSN Print: 2162-2434 Empirical Research on the Impact of Real Estate on Economic Development Shichang Shen School of Mathematics and Statistics, Qinghai Nationalities University, Xining, China How to cite this paper: Shen, S.C. (2021) Abstract Empirical Research on the Impact of Real Estate on Economic Development. Journal Real estate development investment has a significant impact on economic of Mathematical Finance, 11, 246-254. development. Based on data from 17 prefecture-level cities and prefectures in https://doi.org/10.4236/jmf.2021.112014 Hubei Province from 2002 to 2018, the relationship between real estate de- Received: March 5, 2021 velopment investment and economic growth is analyzed with the help of Accepted: May 14, 2021 panel data models. The study found that there is a long-term equilibrium re- Published: May 17, 2021 lationship between the two, and there are regional differences in the impact of real estate development investment on economic growth in Hubei Province. Copyright © 2021 by author(s) and Scientific Research Publishing Inc. Among them, Wuhan has the largest impact and Shennongjia forest area has This work is licensed under the Creative the smallest impact. The impact of real estate development investment on Commons Attribution International economic growth depends on the level of regional economic development. License (CC BY 4.0). http://creativecommons.org/licenses/by/4.0/ Keywords Open Access Real Estate, Economic Growth, Panel Data Model 1. Introduction The real estate industry has been on the rise for many years. In recent years, de- spite the slowdown in the development of the real estate industry across the country, various provinces and cities have introduced various new housing poli- cies in order to attract talent. It can be seen that, whether it is the direct impact of the real estate industry on economic growth in the past few decades or the in- direct impact of the real estate industry on economic growth in recent years, it shows that the real estate industry is an important long-term effective factor for economic growth. Therefore, many scholars [1] [2] [3] [4] [5] discussed the rela- tionship between real estate development investment and economic growth, mainly using the following two methods: the first is to discuss the cause and ef- fect of real estate development investment and economic growth based on Granger causality test [6] relationship; the second is to use time series data to DOI: 10.4236/jmf.2021.112014 May 17, 2021 246 Journal of Mathematical Finance S. C. Shen establish a vector autoregressive (VAR) model [6] to study the interactive rela- tionship between real estate development investment and economic growth. This paper uses panel data to establish a fixed-effect variable coefficient model [7] to conduct an empirical analysis of the relationship between real estate de- velopment investment and economic growth in 17 prefecture-level cities and prefectures in Hubei Province from 2002 to 2018. 2. Empirical Analysis 2.1. Data Description This article selects the annual data of cities and states in Hubei Province from 2002 to 2018. The gross product of each city and state is taken as the explanatory variable and expressed by GDP. The investment scale of real estate development, infrastructure investment scale and technological transformation investment scale of each city and state are taken as the explanatory variable and expressed by REI, INF and TEC respectively. Before establishing the model, we perform a natural logarithmic transformation on each data, which can both eliminate he- teroscedasticity and make the data series more stable. The data comes from Hu- bei Statistical Yearbook. 2.2. Unit Root Test To prevent false regression or false regression, we use LLC, IPS, ADF, PP-Fisher four test methods to analyze the stability of the data, as shown in Table 1. Table 1. Unit root test of panel data. Variable LLC test IPS test ADF test PP-Fisher test −0.34424 5.07864 8.37431 6.93169 LnGDP (0.3653)** (1.0000)* * (1.0000)** (1.0000)** −25.3064 −12.5603 125.554 134.834 ΔLnGDP (0.0000)** (0.0000)** (0.0000)** (0.0000)** −5.09708 0.18295 28.8456 49.1685 LnREI (0.0000)** (0.5726)** (0.6270)** (0.0268)** −8.79931 −7.50299 114.362 130.928 ΔLnREI (0.0000)** (0.0000)** (0.0000)** (0.0000)** −2.96376− 2.69346 19.6971 13.7760 LnINF (0.0015)** (0.9965)** (0.9761)** (0.9992)** −7.48415 −5.89356 96.1887 98.6457 ΔLnINF (0.0000)** (0.0000)** (0.0000)** (0.0000)** −1.46581 1.69675 17.0436 18.4390 LnTEC (0.0713)** (0.9551)** (0.9932)** (0.9863)** −8.53638 −8.28401 133.847 125.490 ΔLnTEC (0.0000)** (0.0000)** (0.0000)** (0.0000)** Note: Δ means first-order difference; **means significance level of 5%. DOI: 10.4236/jmf.2021.112014 247 Journal of Mathematical Finance S. C. Shen As can be seen from the table above, the horizontal sequence of LnGDP, LnREI, LnINF and LnTEC cannot reject the null hypothesis that unit roots exist at a significant level of 5%. However, the P values of the ΔLnGDP, ΔLnREI, ΔLnINF and ΔLnTEC sequences after the first-order difference are all 0, that is, the null hypothesis is rejected, indicating that the first-order difference sequence of the sequence is stable. 2.3. Panel Data Cointegration Test Because the sequence ΔLnGDP, ΔLnREI, ΔLnINF and ΔLnTEC are all I(1) se- quences, the conditions of the cointegration test are satisfied. And the first-order difference sequences of the four variables are all stable, meeting the premise of the co-integration test. In order to ensure the accuracy, the author uses the Pe- droni test, Kao test, and Combined Individual test to verify at the same time. The original hypothesis of these three tests is that there is no co-integration. The test results are shown in Table 2. The results in Table 2 indicate that at the significance level of 5%, the P statis- tics of Panel PP-Statistic, Panel ADF-Statistic, Group PP-Statistic, and Group ADF-Statistic of the Pedroni test are all 0, and most of the test results are re- jected. The null hypothesis indicates the existence of cointegration. However, the other three statistics of the Pedroni test showed no co-integration. Therefore, in order to ensure accuracy, Kao test and Combined Individual test are needed to verify. As can be seen from the above table, the P statistic of the Kao test is less than 5%, the null hypothesis of no co-integration is rejected, and the three hy- potheses of the joint individual test are rejected. This shows that from 2000 to Table 2. Co-integration test results of 17 prefecture-level cities and prefectures in Hubei Province. Statistical methods Statistics Pedroni Test Panel v-Statistic −25.85242 (1.0000) Panel rho-Statistic −1.318870 (0.0936) Panel PP-Statistic −7.216089 (0.0000) Panel ADF-Statistic −7.980418 (0.0000) Group rho-Statistic 2.161981 (0.9847) Group PP-Statistic −4.032946 (0.0000) Group ADF-Statistic −5.359391 (0.0000) Kao Test ADF −2.899656 (0.0019) Fisher Stat.* Hypothesized No. of CE(s) Fisher Stat.* (from trace test) (from max-eigen test) None 395.9 (0.0000) 256.5 (0.0000) At most 1 193.8 (0.0000) 128.9 (0.0000) At most 2 104.6 (0.0000) 69.87 (0.0001) At most 3 98.31 (0.0000) 98.31 (0.0000) DOI: 10.4236/jmf.2021.112014 248 Journal of Mathematical Finance S. C. Shen 2016, there was a long-term stable and balanced relationship between invest- ment in housing development, investment in infrastructure construction, and investment in technological transformation and GDP in Hubei Province. 2.4. Panel Model Selection 2.4.1. Random Effect and Fixed Effect Test Likelihood ratio (LR) test is a test of the model's fixed effect. The null hypothesis of this test is that the fixed effect is redundant. From the results in Table 3, it can be clearly seen that the null hypothesis is rejected under the 5% confidence level That is, the fixed effects introduced are appropriate. 2.4.2. F-Test of Panel Model Through calculation, we can get F2 = 21.77706 , F2 = 8.35356 . Given the significance level of α = 5% , we can know that F2α (60,208) = 1.7 , Fα (45,208) = 1.39 . Because F2 > 1.7 , H2 is rejected; and F1 > 1.39 , H1 is rejected. There- fore, a fixed effect variable coefficient model should be established. 2.4.3. Regression Estimation of Panel Model Based on the panel model’s F-test and likelihood ratio (LR) test results, we estab- lish a fixed-effect variable coefficient model for panel data from 17 cities and states in Hubei Province: LnGDP=+++αβ123 LnREI β LnINF β LnTEC . Eviews7.2 was used to estimate the model, and the results are as follows: Table 4 and Table 5 show the results of applying fixed-effect variable coeffi- cient models to the data of 16 prefecture-level cities in Hubei Province, as well as the results of goodness of fit, statistics, and statistics of regression equations. As can be seen from the table, the estimated value of the explanatory variable at a significant level of 1% is valid, the goodness of fit R2 is above 99%, and the P value of the F statistic is 0. The value of D.W. is near 2, which indicates that cross-section SUR is used to weight the explanatory variables to effectively re- duce the effect of cross-section data on heteroscedasticity and correlation over the same period.
Details
-
File Typepdf
-
Upload Time-
-
Content LanguagesEnglish
-
Upload UserAnonymous/Not logged-in
-
File Pages9 Page
-
File Size-