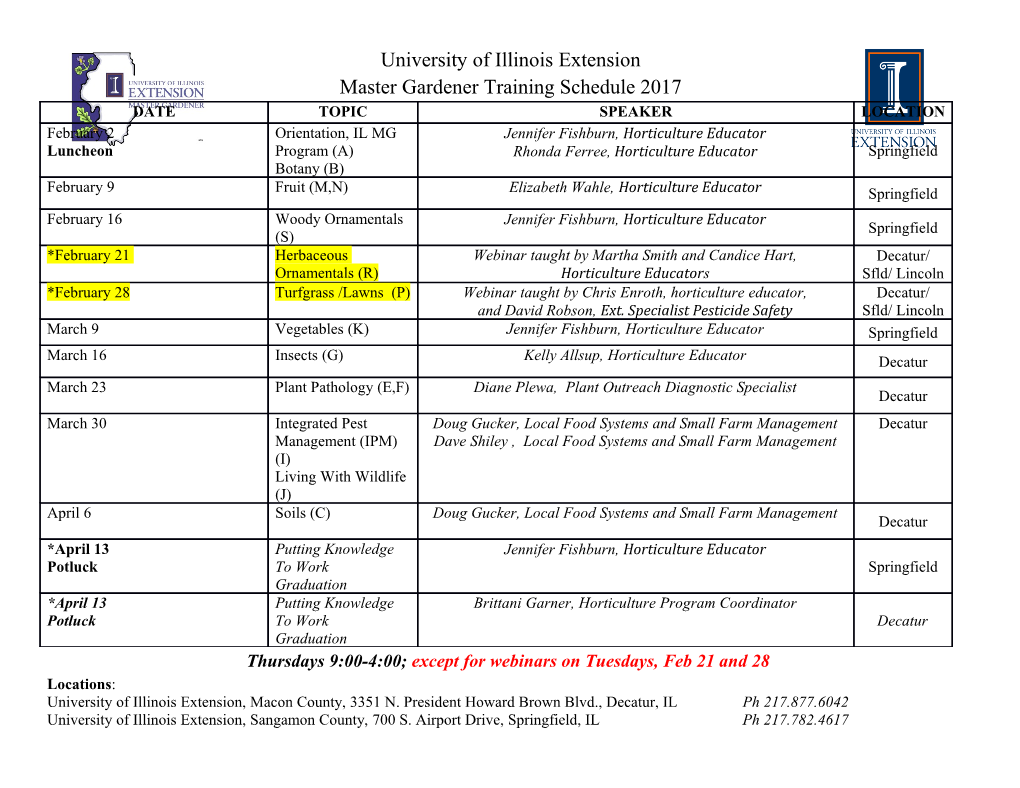
<p>SEM Workshop Handout 1 Page 1 (1/19/2018)</p><p>Structural Equation Modeling And Related Techniques</p><p>Michael Biderman Department of Psychology University of Tennessee at Chattanooga SEM Workshop Handout 1 Page 2 (1/19/2018) Why do this?</p><p>Altruistic Reasons</p><p>To familiarize faculty and students with new techniques.</p><p>Selfish Reasons</p><p>“You don’t really understand something until you’ve taught it.”</p><p>To force myself to address issues that I’ve glossed over in my own reading.</p><p>To get to know faculty and students.</p><p>To assuage loneliness. SEM Workshop Handout 1 Page 3 (1/19/2018) Why learn structural equation modeling techniques?</p><p>The following reasons will be much more meaningful after you’ve had the workshop.</p><p>Provides a single analytic technique for testing a) multiple hypotheses, involving b) multiple variables including c) latent variables and possibly involving d) multiple stages.</p><p>Latent variable: A variable believed to exist but one which is not directly observable.</p><p>Latent variables are typically indicated by several observed variables, each of which gives partial information about the latent variable.</p><p>, SEM Workshop Handout 1 Page 4 (1/19/2018) Written resources</p><p>In order of relevance to this workshop</p><p>Arbuckle, James L., & Wothke, Werner. (1999). Amos 4.0 User’s Guide. Chicago, IL: SmallWaters Corporation. ISBN 1-56827- 264-2. AMOS is at V5, so there may be a V5 User’s Guide.</p><p>Byrne, Barbara M. (2001). Structural equation modeling with AMOS: basic concepts, applications, and programming. Mahwah, NJ: Erlbaum. ISBN 0-8058-3322-6.</p><p>Loehlin, John C. (1998). Latent variable models: an introduction to factor, path, and structural analysis. 3rd Ed. Mahwah, NJ: Erlbaum. ISBN 0-8058-2831-1 (Paperback), 0-8058-2830-3 (Cloth).</p><p>Maruyama, Geoffrey M. (1998). Basics of structural equation modeling. Thousand Oaks, CA: Sage. ISBN 0-8039-7408-6 (Cloth), 0-8039-7409-4 (Pbk.)</p><p>Duncan, Terry E., Duncan, Susan C., Strycker, Lisa A., Fuzhong, Li, & Alpert, Anthony. (1999). An introduction to latent variable growth curve modeling: concepts, issue, and applications. Mahway, NJ: Erlbaum. ISBN 0-8058-3060-X</p><p>Kline, Rex B. (198). Principles and practice of structural equation modeling. New York: Guilford Press. ISGN 1-57230-336-0 (Cloth), 1-57230-337-9 (Pbk). Lots of words, few diagrams.</p><p>Byrne, Barbara M. (1994). Structural equation modeling with EQS and EQS/Windows. 1000 Oaks, CA: Sage. ISBN 0-8039-5092-6.</p><p>Byrne, Barbara M. (1998). Structural equation modeling with LISREL, PRELIS, and SIMPLIS: basic concepts, applications, and programming. Mahway, NJ: Erlbaum. ISGN 0-8058-2924-5. SEM Workshop Handout 1 Page 5 (1/19/2018) Other resources</p><p>Statistical Analysis with Latent Variables by Bengt O. Muthén http://www.ats.ucla.edu/stat/seminars/ed231e/default.htm</p><p>This is the web site of a course being offered in Spring 2004. The course lectures are available as streaming video. It goes quite a bit beyond what will be covered in this workshop.</p><p>Ed Rigdon's Structural Equation Modeling Page http://www.gsu.edu/~mkteer/</p><p>This page contains a compendium of links to other sites dealing with structural equation modeling. (I found it by googling structural equation modeling.)</p><p>Working Group Structural Equation Modeling http://www.uni-muenster.de/SoWi/struktur/</p><p>From the web page: “This is the Web-Page of the Working Group Structural Equation Modeling. This group has now its 10 years anniversary and is going to create information pages for its members and other people who are interested in developments and applications of structural equation models.” Note: The group offers a workshop on Amos. Cost is $190. Two catches: It’s in Germany and it’s in German. SEM Workshop Handout 1 Page 6 (1/19/2018)</p><p>Some computer programs for SEM</p><p>In order of appearance on the scene</p><p>LISREL K.G. Jő reskog</p><p>The gold standard. Reputation for being difficult to use. Typically used via a programming language.</p><p>EQS. Peter Bentler. Easier to use than LISREL. Tyypically used via a programming language.</p><p>AMOS James Arbuckle Very easy to use (as a structural equation modeling program can be) Typically programmed using path diagrams. A programming language is available. Ties with SPSS, Inc.</p><p>SAS, PROC CALIS</p><p>I don’t know much about this proc.</p><p>MPLUS. Bengt O. Muthén Latent variable analyses with continuous and discrete variables. Touted as integrating a variety of analyses that go beyond traditional SEM. May be the program of the future. SEM Workshop Handout 1 Page 7 (1/19/2018)</p><p>Relationship of SEM to other types of analyses</p><p>A hierarchy of analytic techniques.</p><p>Each analytic technique can perform all the essential analyses of the prior techniques. t-tests are a special case of the Analysis of Variance. ANOVA does t- tests and more.</p><p>All analysis of variance tests can be performed using multiple regression analyses. MR does ANOVA and more.</p><p>All multiple regression analyses can be performed using structural equation modeling techniques and programs. SEM does multiple regression and more.</p><p>Who know what the next omnibus technique will be.</p><p>?????</p><p>Structural Equation Modeling</p><p>Multiple Regression /General Linear Model ANOVA t-tests SEM Workshop Handout 1 Page 8 (1/19/2018) Basic SEM Concepts</p><p>Observed Variable: </p><p>A variable whose values are directly observable without error.</p><p>The scores in a column of the SPSS data editor. So these are what we work with every day in SPSS.</p><p>UGPA, GREV, GREQ, GREA, IQ scores (but not intelligence), Depression scores (but not depression).</p><p>Most current SEM analyses involve continuous variables.</p><p>All SEM analyses begin and end with observed variables.</p><p>Latent Variable: </p><p>A variable whose values cannot be directly observed without error.</p><p>Values of latent variables are indicated by observed variables. </p><p>The indicators are assumed to imperfectly represent the values of the latent variable.</p><p>Latent Variable Indicators</p><p>Job Satisfaction Score on Job Satisfaction Survey, Score on Job Descriptive Index Score on MN Satisfaction Questionnaire.</p><p>Intelligence Score on Wonderlic, Score on Stanford Binet Score on Wechsler</p><p>Depression Score on Beck Depression Inventory Hamilton Depression Rating Scale Center for Epidemiological Studies-D Scale SEM Workshop Handout 1 Page 9 (1/19/2018) Exogenous Variable: </p><p>A variable whose variation is assumed to exist but is not accounted for in an analysis.</p><p>Equivalent to independent variables in traditional analyses.</p><p>May be observed or latent.</p><p>Endogenous Variable</p><p>A variable whose variation is completely explained by the other variables in an analysis.</p><p>Equivalent to dependent variables in traditional analyses.</p><p>May be observed or latent.</p><p>Path Diagrams</p><p>It is very common to represent the analyses performed using SEM using path diagrams.</p><p>Although all the analyses can be represented as sets of equations, many, perhaps most analysts prefer to represent models with path diagrams.</p><p>Some programs, for example, AMOS, were written to be programmed using path diagrams. (How cool is that?)</p><p>We will make extensive use of path diagrams to represent relationships, models, and analyses in this workshop. SEM Workshop Handout 1 Page 10 (1/19/2018) Basic Path Analytic / SEM Notation</p><p>Observed variables are symbolized by squares or rectangles.</p><p>103 84 The values of the observed variable. Observed 121 These are implied by the use of the 76 Variable . . . symbol, although they’re not shown in 97 path diagrams. 81</p><p>Latent Variables are symbolized by Circles or ellipses.</p><p>106 Note that the values of the latent Latent 78 variable are represented as dimmed – 115 Variable 80 because they’re not directly . . . observable. 93 83</p><p>Correlations between variables are represented by double-headed arrows</p><p>"Correlation" "Correlation" Arrow Arrow Observed Observed Latent Latent Variable A Variable B Variable A Variable B</p><p>103 101 84 90 106 121 128 78 104 76 72 115 79 ...... 80 114 97 93 . . . 79 81 80 93 . . . 83 92 81</p><p>"Causal" or "Predictive" relationships between variables are represented by single- headed arrows "Causal" "Causal" Arrow Arrow Latent Observed Observed Latent Variable Variable Variable Variable</p><p>"Causal" "Causal" Arrow Arrow Observed Latent Latent Observed Variable Variable Variable Variable SEM Workshop Handout 1 Page 11 (1/19/2018) Exogenous Observed Variables: Variables whose variation originates outside the setting and is unexplained. "Correlation" Arrow "Causal" Exogenous Arrow Exogenous Observed Observed Variable Variable</p><p>Exogenous observed variable connect to other variables in the model through either a “causal” arrow or a correlation arrow.</p><p>Exogenous Latent Variables: Latent Variables whose variation originates outside the setting. "Correlation" "Causal" Arrow Arrow Exogenous Exogenous Latent Latent Variable Variable As is the case with exogenous observed variables, exogenous latent variables connect to other variables in the model through either a “causal” arrow or a correlation</p><p>Endogenous Observed Variables - Endogenous Latent Variable</p><p>Random "Causal" Random "Causal" error Arrow Endogenous error Arrow Endogenous Observed Latent Variable Variable Endogenous variables connect to other variables in the model by being on the “receiving” end of “causal” arrows. Specifically, endogenous variables are typically represented as being “caused” by 1) other variables in the theory and 2) random error. Thus, 100% of the variation in every endogenous variable is accounted for by either other variables in the model or random error. This means that random error is an exogenous latent variable in SEM diagrams.</p><p>Values associated with symbols Mean, Variance Mean, Variance Observed Latent Variable Variable Our SEM program, AMOS, prints means and variances above and to the right. Typically the mean and variance of latent variables are fixed at 0 and 1 respectively, although there are exceptions to this in advanced applications. "Correlation" "Causal" Arrow Arrow</p><p>Correlation (r) or Covariance B or : A regression coefficient SEM Workshop Handout 1 Page 12 (1/19/2018) New shoes for old feet: Old analyses done using new techniques</p><p>SEM programs can be used to conduct any analysis that can be performed using multiple regression techniques. And this means that they can conduct any analysis that can be done using MR, such as ANOVA, t-tests.</p><p>“Can be used” should not be confused with “Should be used.”</p><p>SEM program output is not designed for regression analyses, so much of the useful stuff printed by regression programs may be difficult or impossible to obtain.</p><p>SEM programs use different statistical tests than most regression programs, so p-values will often not agree with those of SPSS, for example.</p><p>SEM programs have little diagnostic output.</p><p>So don’t throw away your regression procedure.</p><p>The same goes for ANOVA and for t-tests. SEM Workshop Handout 1 Page 13 (1/19/2018) AMOS representation of correlation of two variables</p><p>Data are records of 190 psych graduate students admitted between 1991 and 2002.</p><p>SPSS Representation</p><p>Correlations</p><p>GREV GREQ GREV Pearson 1 .175* Correlation Sig. (2-tailed) . .015 N 190 190 GREQ Pearson .175* 1 Correlation Sig. (2-tailed) .015 . N 190 190</p><p>*. Correlation is significant at the 0.05 level (2-tailed).</p><p>AMOS Unstandardized Estimates View Covariance</p><p>Mean Variance</p><p>AMOS Standardized Estimates View Correlation Means and variances of observed variables are not represented in Standardized Estimates view because they’re assumed to be 0 and 1 respectively. SEM Workshop Handout 1 Page 14 (1/19/2018) AMOS representation of correlations of many variables. Data are records of 190 psych graduate students admitted between 1991 and 2002.</p><p>SPSS Representation Correlations</p><p>UGPA GREV GREQ GREA UGPA Pearson Correlation 1 .048 -.131 -.099 Sig. (2-tailed) . .512 .071 .176 Sum of Squares and Cross-products 23.170 241.079 -718.929 -591.461 Covariance .123 1.276 -3.804 -3.129 N 190 190 190 190 GREV Pearson Correlation .048 1 .175* .370** Sig. (2-tailed) .512 . .015 .000 Sum of Squares and Cross-products 241.079 1093021.579 208790.000 482132.632 Covariance 1.276 5783.183 1104.709 2550.966 N 190 190 190 190 GREQ Pearson Correlation -.131 .175* 1 .414** Sig. (2-tailed) .071 .015 . .000 Descriptive Statistics Sum of Squares and Cross-products -718.929 208790.000 1295790.000 587180.000 M ean Std. Deviation N Covariance -3.804 1104.709 6856.032 3106.772 UGPA 3.3465 .35013 190 N 190 190 190 190 GREV 472.79 76.047 190 GREA Pearson Correlation -.099 .370** .414** 1 GREQ 557.00 82.801 190 Sig. (2-tailed) GREA 600.32 90.664 190 .176 .000 .000 . Sum of Squares and Cross-products -591.461 482132.632 587180.000 1553581.053 Covariance -3.129 2550.966 3106.772 8220.006 N 190 190 190 190</p><p>*. Correlation is significant at the 0.05 level (2-tailed). **. Correlation is significant at the 0.01 level (2-tailed). AMOS Unstandardized Estimates View Argh!!</p><p>AMOS Standardized Estimates View Much prettier. SEM Workshop Handout 1 Page 15 (1/19/2018) AMOS representation of simple regression analysis Data are records of 190 psych graduate students admitted between 1991 and 2002. P511G is proportion of required points in 1st semester graduate statistics course. Formula is 200*UGPA + (GREV+GREQ)/2</p><p>Model Summary</p><p>SPSS Representation Std. Error of M ode l R R Sq uare Adjusted R Squa re the Estim ate 1 .501a .251 .24 7 .06536</p><p> a. Predi ctors: (Consta nt), FO RM ULA</p><p>Coefficients a</p><p>Standardized Unstandardized Coefficients Coefficients M odel B Std. Error Beta t Sig. 1 (Constant) .369 .065 5.718 .000 FORM ULA .000 .000 .501 7.934 .000</p><p> a. Dependent Variable: P511G Mean and variance of Mean, Variance residual variance are Intercept arbitrarily set = 0 and 1 respectively. (Math B (Reg Coeff) voodoo.) AMOS Unstandardized Estimates View</p><p>All variance of an endogenous variable (P511G) must be accounted for. That which is not related to the observed exogenous variables (UGPA) is assumed to be accounted for by a latent variable representing residual variation (Other). r-squared. AMOS Standardized Estimates View</p><p>The standardized Beta, Standardized regression coefficient. relationship of P511G (Also r in a simple regression.) with other factors. (= √ .1-r-squared) SEM Workshop Handout 1 Page 16 (1/19/2018) AMOS Representation of Multiple Regression Data are records of 190 psych graduate students admitted between 1991 and 2002. P511G is proportion of required points in 1st semester graduate statistics course. SPSS Representation Model Summary Std. Error of M odel R R Square Adjusted R Square the Estim ate 1 .512a .262 .246 .06538</p><p> a. Predictors: (Constant), GREA, UGPA, GREV, GREQ</p><p>Coefficients a</p><p>Standardized Unstandardized Coefficients Coefficients M odel B Std. Error Beta t Sig. 1 (Constant) .367 .065 5.627 .000 UGPA .068 .014 .316 4.940 .000 GREV .000 .000 .116 1.699 .091 GREQ .000 .000 .295 4.225 .000 GREA .000 .000 .164 2.229 .027</p><p> a. Dependent Variable: P511G</p><p>AMOS Unstandardized Representation Messy, messy</p><p>Intercept (Constant in SPSS output)</p><p>Standardized Regression AMOS Standardized Representation weights (Betas)</p><p>1-R2</p><p>Multiple R2 SEM Workshop Handout 1 Page 17 (1/19/2018) Analyses more easily done than in SPSS</p><p>Testing for mediation</p><p>Same data as before – 190 Psychology graduate students.</p><p>Psychology’s formula score: 200*UGPA + (GREV+GREQ)/2.</p><p>PSY 513 is taken immediately following PSY 511.</p><p>How should PSY 513 be predicted?</p><p>Is performance in 1st semester graduate statistics all that is needed to predict performance in the 2nd semester?</p><p>Or, even if we know P511G, will Formula still add to our ability to predict P513G? SEM Workshop Handout 1 Page 18 (1/19/2018) AMOS representation of mediated relationship AMOS unstandardized view</p><p>Intercept</p><p>The coefficients above indicate that there is a positive relationship of P511G to Formula (B=.0004). The coefficient is small because of the difference in variability of the Formula and P511G scores. The formula scores range from 1000-1600 while the P511G (and P513G) scores range from .6 – 1.00. There is a positive relationship (B=.69)of P511G to P513G controlling for Formula. And there is a small, positive relationship (B=.0001) of Formula to P513G controlling for P511G. The output below shows that all coefficients are significantly different from 0. (AMOS prints *** when a p-value is less than .001.) The fact that the Formula-to- P513G coefficient is significantly different from 0 indicated that P511G only partially mediates the relationship between P513G and Formula. Excerpt from Text Output Estimate S.E. C.R. P P511G<--- FallOther .0650 .0033 19.4422 *** P511G<--- FORMULA .0004 .0001 7.9551 *** P513G<--- FORMULA .0001 .0001 2.5154 .0119 P513G<--- P511G .6943 .0662 10.4812 *** P513G<--- SpringOther .0592 .0030 19.4422 *** SEM Workshop Handout 1 Page 19 (1/19/2018)</p><p>AMOS standardized view</p><p>R2</p><p>The Standardized Formula-to-P511G coefficient of .50 means that a difference of one standard deviation in formula score is associated with about a .50 SD increase in P511G score. The .62 means that a 1 SD difference in P511G is associated with a .62 SD increase in P513G, controlling for Formula scores. The .15 means that a 1 SD increase in Formula score is associated with a .15 SD increase in P513G even when controlling for P511G.</p><p>What’s it all mean?</p><p>P511G: Immediate past performance is the best predictor of future performance. Plus the P511G score probably reflects aspects of the graduate school experience not represented in the Formula score – adjustment to UTC, roommates, financial situations, etc. – that carries over to the spring P513 course. Formula: Even among persons equal in performance in the fall course, P513G performance is related to UGPA and GRE scores as embodied in the Formula score. This might reflect difference in content between 511 (review of basic statistics, research methods) and 513 (emphasis on multiple regression), or differential weighting of intellectual and motivational characteristics between fall and spring. SEM Workshop Handout 1 Page 20 (1/19/2018) Regression with a Latent Dependent Variable</p><p>Quionna Caldwell Thesis</p><p>Quionna studied the relationship of perceptions of organizations’ diversity efforts to liking for the organization.</p><p>Sample: 200 African American female managers</p><p>Independent Variables</p><p>IV1. Perception of organization’s inclusion of diverse elements.</p><p>Management here encourages the formation of employee network support groups. There is a mentoring program in use here that identifies and prepares all minority and female employees for promotion. The “old boys’ network” is alive and well here. ® The company spends enough money and time on diversity awareness and related training.</p><p>IV2. Perception of organization’s fairness to diverse elements.</p><p>I feel I have been treated differently here because of my race, sex, religion, or age. ® Managers here have a track record of hiring and promoting employees objectively, regardless of their race, sex, religion, or age. Managers here give feedback and evaluate employees fairly, regardless of the employee’s ethnicity, gender, age, or social background. Manager’s here make layoff decisions fairly, regardless of factors such as employees’ race, sex, age, or social background. Managers interpret human resource policies (such as sick leave) fairly for all employees. Managers here give assignments based on the skills and abilities of employees.</p><p>Participants responded to each item indicating among of agreement with the item on a six-level scale, from 1=Strongly Disagree to 6 = Strongly agree.</p><p>Scale scores were computed by averaging responses to the questions within each scale. ® indicates that an item was reverse scored before averaging. SEM Workshop Handout 1 Page 21 (1/19/2018) Caldwell Thesis Dependent Variables</p><p>DV1. Job Satisfaction Scale.</p><p>How satisfied do you feel with your chances for getting ahead in this organization? All in all, how satisfied are you with the persons on your work group? All in all, how satisfied are you with your supervisor? All in all, how satisfied are you with this organization, compared to most others? How satisfied do you feel with the progress you have made in this organization up to now? Considering your skills and the effort you put into the work, how satisfied are you with your pay? All in all, how satisfied are you with your job?</p><p>DV2: Affective Commitment Scale</p><p>I would be very happy to spend the rest of my career with this organization. I really feel as if this organization’s problems are my own. I do not feel like “part of the family” at my organization. ® I do not feel “emotionally attached” to this organization. ® This organization has great deal of personal meaning for me. I do not feel a strong sense of “belonging” to my organization. ®</p><p>DV3: Turnover intention Scale</p><p>I think a lot about leaving the organization. I am actively searching for a substitute for the organization. As soon as it is possible I will leave the organization.</p><p>Scale scores for the three dependent variables were computed in a fashion analogous to those for the independent variables. SEM Workshop Handout 1 Page 22 (1/19/2018) Caldwell Thesis: Analysis of Observed Dependent Variables Separately</p><p>Job Satisfaction</p><p>Affective Commitment</p><p>Turnover Intent</p><p>We could leave the analysis at this: Perception of org diversity efforts are related to Job Satisfaction, Affective Commitment, and Turnover Intentions. SEM Workshop Handout 1 Page 23 (1/19/2018) Caldwell Thesis: Analysis of Liking for Organization as a latent Dependent Variable</p><p>An alternative to the above analyses is one that treats the three DVs in the above as indicators of a more general “Liking for the Organization” construct.</p><p>The advantage of this representation is that is unifies the separate variables, Job Satisfaction, Affective Commitment, and Turnover Intention under a single latent variable representing the participants’ attitude toward their organization.</p><p>Notes: </p><p>35% of the variance in org liking was related to the diversity perceptions.</p><p>The three outcome variables – JS, AC, and TI, were all strongly related to the org liking variable.</p>
Details
-
File Typepdf
-
Upload Time-
-
Content LanguagesEnglish
-
Upload UserAnonymous/Not logged-in
-
File Pages23 Page
-
File Size-