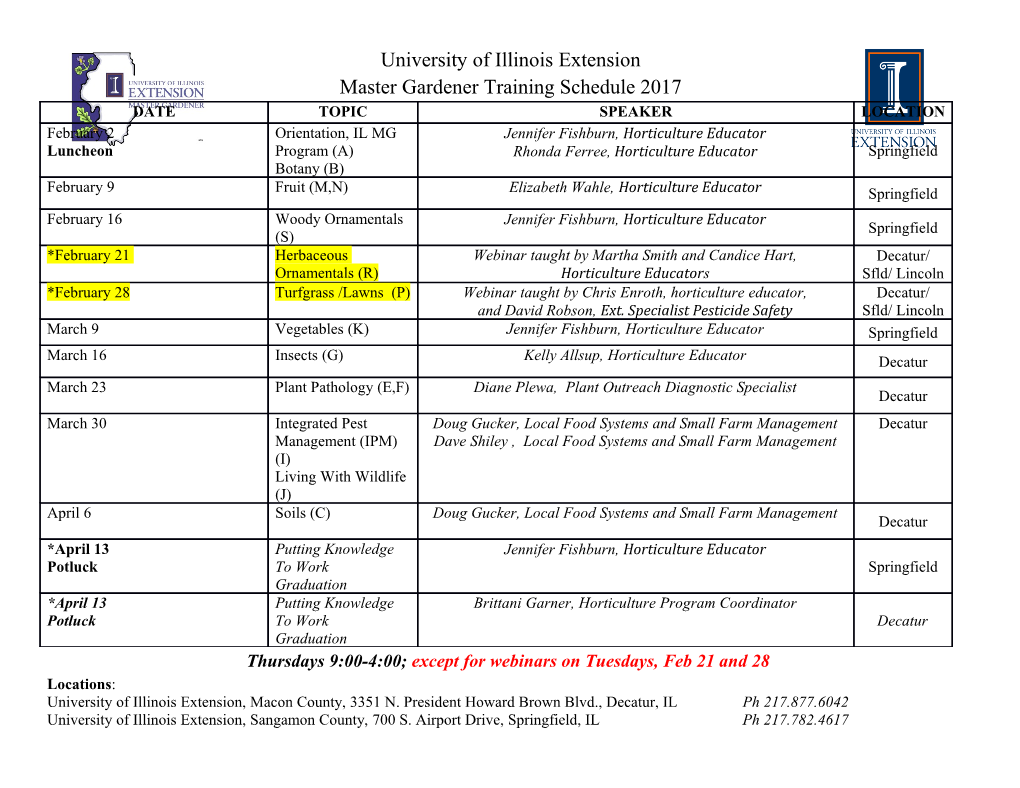
<p> Electronic Supplementary Material</p><p>Tables with comparative results for linear and logistic fixed effect models</p><p>In the following tables (Tables S1 and S2) we provide a comparative analysis of the logistic models (used in this study) and the linear model used by similar studies (as discussed in the ‘Method’ section of the paper). </p><p>As noted in the paper the logistic fixed effects model takes the form:</p><p>In the linear model the response is replaced by . Note the linear model is weighted by the square root of the population.</p><p>1 Table S1 Result of the Aggregate State Level Data Analysis Unemployment rate lag 0 Unemployment rate lag 1 Unemployment rate lag 2 Unemployment rate lag 3 Unemployment rate lag 4 Linear Logistic Linear Logistic Linear Logistic Linear Logistic Linear Logistic Males All ages State 0.480 0.669** 0.630* 0.719** 0.518 0.679** 0.099 0.305 -0.191 -0.116 Unemployment (0.266) (0.239) (0.270) (0.239) (0.270) (0.236) (0.267) (0.240) (0.255) (0.234) State per capita 0.047** 0.037** 0.048** 0.037** 0.045** 0.034** 0.042** 0.027** 0.041** 0.024* GDP ($0,000) (0.009) (0.011) (0.009) (0.010) (0.009) (0.010) (0.009) (0.010) (0.009) (0.010) R-square 0.984 0.979 0.985 0.979 0.984 0.979 0.984 0.978 0.984 0.977 Durbin Watson 2.177 2.087 2.183 2.082 2.192 2.135 2.168 2.086 2.168 2.082 Females All ages State 0.547 0.617 0.797* 0.723* 0.511 0.544 -0.064 -0.005 -0.142 -0.248 Unemployment (0.372) (0.326) (0.360) (0.312) (0.364) (0.316) (0.362) (0.321) (0.354) (0.311) State per capita 0.041** 0.032** 0.042** 0.032** 0.037** 0.027* 0.034** 0.021* 0.035** 0.021* GDP ($0,000) (0.011) (0.012) (0.010) (0.011) (0.010) (0.011) (0.010) (0.011) (0.010) (0.010) R-square 0.982 0.974 0.982 0.975 0.982 0.974 0.982 0.974 0.982 0.974 Durbin Watson 2.146 2.182 2.161 2.198 2.178 2.216 2.167 2.203 2.163 2.205 Combined Sexes All ages State 0.505 0.662** 0.752** 0.759** 0.519 0.651** -0.014 0.196 -0.291 -0.222 Unemployment (0.279) (0.253) (0.273) (0.246) (0.272) (0.244) (0.269) (0.249) (0.256) (0.240) State per capita 0.042** 0.034** 0.044** 0.034** 0.040** 0.030** 0.036** 0.023* 0.036** 0.020* GDP ($0,000) (0.009) (0.010) (0.008) (0.010) (0.008) (0.009) (0.008) (0.009) (0.008) (0.009) R-square 0.987 0.981 0.988 0.981 0.987 0.981 0.987 0.980 0.987 0.980 Durbin Watson 2.139 2.078 2.156 2.089 2.173 2.134 2.150 2.095 2.150 2.104 These are the results of models where each lag of unemployment was fitted separately. The standard errors are provided in parentheses. Statistical significance is marked with stars.* - significant at 5% level of significance and **- significant at 1% level of significance.</p><p>2 Table S2 Result of the Aggregate State Level data with combined Unemployment Rate effects. 15-34 35-44 45-54 55-64 65 and above All Ages Linear Logistic Linear Logistic Linear Logistic Linear Logistic Linear Logistic Linear Logistic Males Combined State -0.834 -0.040 1.668 1.743 -0.279 0.385 -0.711 -0.437 0.392 0.213 0.705* 0.918** Unemployment (0.798) (0.731) (1.204) (0.977) (0.911) (0.688) (0.432) (0.347) (0.471) (0.491) (0.319) (0.276) State per capita 0.136** 0.213** 0.077** 0.077** 0.033 0.029 -0.008 -0.009 0.001 -0.021 0.049** 0.040** GDP ($0,000) (0.041) (0.050) (0.028) (0.030) (0.023) (0.023) (0.016) (0.017) (0.009) (0.011) (0.009) (0.011) R-square 0.914 0.930 0.734 0.824 0.910 0.946 0.983 0.990 0.961 0.972 0.985 0.979 Durbin Watson 1.974 2.079 2.112 2.190 1.982 1.982 2.222 2.276 2.325 2.291 2.187 2.114 Females Combined State -0.637 0.352 0.368 0.609 0.468 0.696 -0.727 -1.082 0.012 -0.457 0.791 0.796* Unemployment (1.326) (1.166) (2.032) (1.691) (1.563) (1.188) (1.151) (0.916) (0.315) (0.386) (0.440) (0.375) State per capita 0.232** 0.270** 0.055 0.050 -0.003 0.001 -0.039 -0.030 0.010 -0.005 0.042** 0.034** GDP ($0,000) (0.059) (0.065) (0.033) (0.035) (0.028) (0.028) (0.022) (0.021) (0.009) (0.011) (0.011) (0.012) R-square 0.778 0.829 0.634 0.724 0.849 0.905 0.951 0.971 0.887 0.901 0.982 0.975 Durbin Watson 2.061 2.134 2.150 2.126 2.263 2.313 2.186 2.104 2.266 2.215 2.160 2.194 Combined Sexes Combined State -0.926 0.104 2.171 2.079 0.142 0.652 -1.037 -0.730 0.381 -0.201 0.698* 0.871** Unemployment (0.830) (0.751) (1.232) (1.021) (0.781) (0.626) (0.481) (0.382) (0.456) (0.525) (0.325) (0.287) State per capita 0.165** 0.240** 0.072** 0.078** 0.019 0.020 -0.021 -0.017 0.007 -0.011 0.044** 0.036** GDP ($0,000) (0.039) (0.046) (0.022) (0.025) (0.016) (0.018) (0.014) (0.014) (0.008) (0.010) (0.009) (0.010) R-square 0.914 0.930 0.791 0.858 0.950 0.967 0.985 0.991 0.947 0.957 0.988 0.981 Durbin Watson 1.945 2.063 2.078 2.075 2.034 2.024 2.089 2.046 2.280 2.226 2.158 2.098 The models were fitted with unemployment rates at lags 0, 1 and 2 taken together. The coefficients are the sum of the coefficients of the three lags. The standard errors are provided in parentheses. Statistical significance is marked with stars.* - significant at 5% level of significance and **- significant at 1% level of significance.</p><p>3 Age-Specific Analysis</p><p>The results of the age level analysis are provided in Table S3, where, for brevity, only the results for the unemployment rates are presented. In the analysis, for changes in the current level of unemployment rate, mortality is found to be significantly counter-cyclical in nature for males aged 35-44 and combined sexes in the same age group. Furthermore, using the previous year’s unemployment rate (lag 1); the analysis shows that mortality is pro-cyclical for males and combined sexes aged 55-64. (Note that only four (out of 45) results were significant, hence we have to be careful about drawing any conclusions from this analysis.) </p><p>Table S3 Result of the Aggregate State Level data (Age-Specific) 15-34 35-44 45-54 55-64 65 and above Males Unemployment 0.019 1.892* 0.317 -0.211 0.225 Rate, Lag 0 (0.651) (0.833) (0.595) (0.287) (0.293) Unemployment 0.481 0.876 0.103 -0.795** -0.061 Rate, Lag 1 (0.608) (0.809) (0.573) (0.277) (0.289) Unemployment -0.196 0.564 0.253 0.027 0.082 Rate, Lag 2 (0.576) (0.809) (0.570) (0.274) (0.283) Females Unemployment 0.268 0.049 0.712 -0.230 -0.383 Rate, Lag 0 (1.021) (1.236) (0.934) (0.613) (0.247) Unemployment 0.296 0.331 -0.086 -0.705 -0.009 Rate, Lag 1 (0.980) (1.258) (0.912) (0.599) (0.240) Unemployment 0.250 0.606 0.540 -0.504 -0.198 Rate, Lag 2 (0.957) (1.257) (0.897) (0.594) (0.233) Combined Sexes Unemployment 0.129 2.175* 0.660 -0.348 -0.071 Rate, Lag 0 (0.675) (0.851) (0.545) (0.310) (0.314) Unemployment 0.307 1.097 0.527 -0.807** -0.102 Rate, Lag 1 (0.635) (0.847) (0.528) (0.300) (0.303) Unemployment 0.042 0.742 0.299 -0.282 -0.106 Rate, Lag 2 (0.606) (0.849) (0.527) (0.294) (0.293) These are the results of models where each lag of unemployment was fitted separately. Only the coefficients of the unemployment rates are provided here (all values are in percentage points). The standard errors are provided in parenthesis. Statistical significance is marked with stars.* - significant at 5% level of significance and **- significant at 1% level of significance.</p><p>In the models with all the lags taken together the study does not find any significant cyclical mortality for the age level analysis. The results are provided in Table S4.</p><p>Table S4 Result of the Aggregate State Level data with combined Unemployment Rate effects (Age-Specific)</p><p>15-34 35-44 45-54 55-64 65 and above Males Combined State -0.040 1.743 0.385 -0.437 0.213</p><p>4 Unemployment (0.731) (0.977) (0.688) (0.347) (0.491) State per capita 0.213** 0.077** 0.029 -0.009 -0.021 GDP ($0,000) (0.050) (0.030) (0.023) (0.017) (0.011) R-square 0.930 0.824 0.946 0.990 0.972 Durbin Watson 2.079 2.190 1.982 2.276 2.291 Females Combined State 0.352 0.609 0.696 -1.082 -0.457 Unemployment (1.166) (1.691) (1.188) (0.916) (0.386) State per capita 0.270** 0.050 0.001 -0.030 -0.005 GDP ($0,000) (0.065) (0.035) (0.028) (0.021) (0.011) R-square 0.829 0.724 0.905 0.971 0.901 Durbin Watson 2.134 2.126 2.313 2.104 2.215 Combined Sexes Combined State 0.104 2.079 0.652 -0.730 -0.201 Unemployment (0.751) (1.021) (0.626) (0.382) (0.525) State per capita 0.240** 0.078** 0.020 -0.017 -0.011 GDP ($0,000) (0.046) (0.025) (0.018) (0.014) (0.010) R-square 0.930 0.858 0.967 0.991 0.957 Durbin Watson 2.063 2.075 2.024 2.046 2.226 The models were fitted with unemployment rates at lags 0, 1 and 2 taken together. The coefficients are the sum of the coefficients of the three lags. The standard errors are provided in parenthesis. Statistical significance is marked with stars.* - significant at 5% level of significance and **- significant at 1% level of significance.</p><p>References (electronic supplement only)</p><p>Guest, R. S. (2005). A Life Cycle Analysis of Housing Affordability Options for First Home Owner-Occupiers in Australia, Economic Record, 81, 237-248. Ruhm, C. J. (2000). Are Recessions Good for Your Health?, Quarterly Journal of Economics, 115, 617-650. Worthington, A. C. (2009). The Usage and Understanding of Australian Household Mortgages, International Journal of Housing Markets and Analysis, 2(4), 347-362.</p><p>5 R code used in the paper [Code for paper was run on R version 2.15]</p><p># initial stuff options(contrasts=c(factor="contr.sum", ordered="contr.poly")) #this sets the default contrasts in R to sum contrasts library(MASS) library(car) #need to install the package first library(lmtest) #need to install the package first library (stats)</p><p># the following function creates the coefficients output in a desired format (as presented in the papers). Obtained from Dave Armstrong's website <http://www.quantoid.net/> shuffle <- function(b,se,p, df.res, two.sided=T, digits, sep= ",", apa=T){ nb <- names(b) nse <- sapply(1:length(b), function(x)paste(rep("", each=x), collapse="")) stars <- sapply(1:length(p), function(x)paste(rep("*", x), collapse="")) stars <- c("", stars) sig <- do.call(cbind, lapply(p, function(x) (2^(two.sided)*pt(abs(b/se), df.res, lower.tail=F)) < x)) sig <- apply(sig, 1, sum)+1 b <- sprintf(paste("%.", digits, "f", sep=""), b) se <- sprintf(paste("%.", digits, "f", sep=""), se) if(apa){ newb <- gsub("^-0", "-", gsub("^0", "", b)) } else{ newb <- b } newb <- paste(newb, stars[sig], sep="") if(apa){ newse <- gsub("^-0", "-", gsub("^0\\.", "\\.", se)) } else{ newse <- se } newse <- paste("(", newse, ")", sep="") mat <- matrix(rbind(newb, newse), ncol=1) rownames(mat) <- as.vector(rbind(nb, nse)) mat <- cbind(sep, mat) colnames(mat) <- c("", "") noquote(mat) }</p><p># Table 1 - descriptive statistics (getting the means and standard deviations of the variables used in the study) a<-c("./mdatay/Statewn/mnt6.csv", "./mdatay/Statewn/fnt6.csv" , "./mdatay/Statewn/bnt6.csv")</p><p>6 vmn<-matrix(nrow=8,ncol=3) vsd<-matrix(nrow=8,ncol=3) for( i in 1:3){ mean1<-vector(length=8) sd1<-vector(length=8) ausm1 <- read.csv(file=paste(a[i]),head=TRUE,sep=",") attach(ausm1) mr<-(Deaths/Pop*100000) GDP=GDPPP/1000 MED=MEDPP/100 mean1[1]<-mean(mr) mean1[2]<-mean(U0)*100 mean1[3]<-mean(GDP) mean1[4]<-mean(MED) mean1[5]<-mean(ABP)*100 mean1[6]<-mean(EDUP)*100 mean1[7]<-mean(p5)*100 mean1[8]<-mean(p65)*100 sd1[1]<-sd(mr) sd1[2]<-sd(U0)*100 sd1[3]<-sd(GDP) sd1[4]<-sd(MED) sd1[5]<-sd(ABP)*100 sd1[6]<-sd(EDUP)*100 sd1[7]<-sd(p5)*100 sd1[8]<-sd(p65)*100 vmn[,i]<-mean1 vsd[,i]<-sd1 detach(ausm1) } round(vmn,digits=2) # this gives the means used in the table round(vsd,digits=2) # this gives the standard deviations used in the table</p><p># Table 2 - output of the regressions with separate model for each unemployment rate a<-c("./mdatay/Statewn/mnt6.csv", "./mdatay/Statewn/fnt6.csv" , "./mdatay/Statewn/bnt6.csv") result1<- matrix(nrow=14,ncol=9) rs1<- vector(length=9) rs2<- vector(length=9) for( i in 1:3){ j=(i*3)-2 k=j+1 l=j+2 ma1 <- read.csv(file=paste(a[i]),head=TRUE,sep=",") attach(ma1) GDP=GDPPP/10000 MED=MEDPP/1000 Pop1=round(Pop,digits=0) dr=cbind(Deaths,Pop1) 7 #the model used by other researchers takes the form(not fitted) #mr<-(Deaths/Pop*100000) #weight=sqrt(Pop) #fixed1<-lm(log(mr)~U0+GDP+MED+EDUP+ABP+p5+p65+factor(Time) +factor(STATE),weights=weight) #fixed2<-lm(log(mr)~U1+GDP+MED+EDUP+ABP+p5+p65+factor(Time) +factor(STATE),weights=weight) #fixed3<-lm(log(mr)~U2+GDP+MED+EDUP+ABP+p5+p65+factor(Time) +factor(STATE),weights=weight) log1<-glm(dr~U0+GDP+MED+EDUP+ABP+p5+p65+factor(Time) +factor(STATE),family=quasibinomial(logit)) log2<-glm(dr~U1+GDP+MED+EDUP+ABP+p5+p65+factor(Time) +factor(STATE),family=quasibinomial(logit)) log3<-glm(dr~U2+GDP+MED+EDUP+ABP+p5+p65+factor(Time) +factor(STATE),family=quasibinomial(logit)) d1<-shuffle(log1$coef,sqrt(diag(vcov(log1))), c(0.1,.05,.01),log1$df.residual, two.sided=T, digits=3, apa=F) d2<-shuffle(log2$coef,sqrt(diag(vcov(log2))), c(0.1,.05,.01),log2$df.residual, two.sided=T, digits=3, apa=F) d3<-shuffle(log3$coef,sqrt(diag(vcov(log3))), c(0.1,.05,.01),log3$df.residual, two.sided=T, digits=3, apa=F) result1[,j]<-d1[3:16,2] result1[,k]<-d2[3:16,2] result1[,l]<-d3[3:16,2] rs1[j]<-1-(log1$deviance/log1$null.deviance) rs2[j]<-durbinWatsonTest(log1)$dw rs1[k]<-1-(log2$deviance/log2$null.deviance) rs2[k]<-durbinWatsonTest(log2)$dw rs1[l]<-1-(log3$deviance/log3$null.deviance) rs2[l]<-durbinWatsonTest(log2)$dw detach(ma1) } result1 # Coefficients, first 3 are males, next 3 are females and last 3 are individuals round(rs1,digits=3) #rsquared , first 3 are males, next 3 are females and last 3 are individuals round(rs2,digits=3) #dwstatistic, first 3 are males, next 3 are females and last 3 are individuals</p><p>#Table 3 - estimating the effect of combined lags of unemployment rate on mortality using a distributed lag approach (in a fixed effects context) a<-c("./mdatay/Statewn/mnt6.csv", "./mdatay/Statewn/fnt6.csv" , "./mdatay/Statewn/bnt6.csv") est2<- vector(length=3) se2<- vector(length=3) pt2<- vector(length=3) rs2<-vector(length=3) dw2<-vector(length=3) result2<- matrix(nrow=12,ncol=3)</p><p>8 for( i in 1:3){ ma1 <- read.csv(file=paste(a[i]),head=TRUE,sep=",") attach(ma1) GDP=GDPPP/10000 MED=MEDPP/1000 Pop1=round(Pop,digits=0) dr=cbind(Deaths,Pop1) logi<-glm(dr~U0+U1+U2+GDP+MED+EDUP+ABP+p5+p65+factor(Time) +factor(STATE),family=quasibinomial(logit)) d1<-shuffle(logi$coef,sqrt(diag(vcov(logi))), c(.1,.05,.01),logi$df.residual, two.sided=T, digits=3, apa=F) result2[,i]<-d1[9:20,2] est2[i]<-sum(logi$coef[2:4]) af<-subset(vcov(logi), select = U0:U2) af2<-af[2:4,] se2[i]<-sqrt(sum(af2)) pt2[i]<-2*(1-pnorm(abs(est2[i]/se2[i]),mean=0,sd=1)) rs2[i]<-1-(logi$deviance/logi$null.deviance) dw2[i]<-durbinWatsonTest(logi)$dw detach(ma1) } round(est2,digits=3) # provides the estimate for unemployment round(se2,digits=3) # provides the standard error for unemployment round(pt2,digits=3) # provides the probability value for unemployment coefficient (used for finding significance) round(rs2,digits=3) # R-squared round(dw2,digits=3) # Dw Statistic result2 # other coeffients - GDP, Medical EXP, Education, ABP, P5 and P65</p><p># Table 5 - output of the regressions with separate model for each unemployment rate ### Expansion### a<-c("./mdatay/Statewn/mnt6c.csv", "./mdatay/Statewn/fnt6c.csv" , "./mdatay/Statewn/bnt6c.csv") result1<- matrix(nrow=14,ncol=9) rs1<- vector(length=9) rs2<- vector(length=9) for( i in 1:3){ j=(i*3)-2 k=j+1 l=j+2 maa1 <- read.csv(file=paste(a[i]),head=TRUE,sep=",") ma1<-subset(maa1,Growth==1) attach(ma1) GDP=GDPPP/10000 MED=MEDPP/1000 Pop1=round(Pop,digits=0) dr=cbind(Deaths,Pop1)</p><p>9 #the model used by other researchers takes the form(not fitted) #mr<-(Deaths/Pop*100000) #weight=sqrt(Pop) #fixed1<-lm(log(mr)~U0+GDP+MED+EDUP+ABP+p5+p65+factor(Time) +factor(STATE),weights=weight) #fixed2<-lm(log(mr)~U1+GDP+MED+EDUP+ABP+p5+p65+factor(Time) +factor(STATE),weights=weight) #fixed3<-lm(log(mr)~U2+GDP+MED+EDUP+ABP+p5+p65+factor(Time) +factor(STATE),weights=weight) log1<-glm(dr~U0+GDP+MED+EDUP+ABP+p5+p65+factor(Time) +factor(STATE),family=quasibinomial(logit)) log2<-glm(dr~U1+GDP+MED+EDUP+ABP+p5+p65+factor(Time) +factor(STATE),family=quasibinomial(logit)) log3<-glm(dr~U2+GDP+MED+EDUP+ABP+p5+p65+factor(Time) +factor(STATE),family=quasibinomial(logit)) d1<-shuffle(log1$coef,sqrt(diag(vcov(log1))), c(0.1,.05,.01),log1$df.residual, two.sided=T, digits=3, apa=F) d2<-shuffle(log2$coef,sqrt(diag(vcov(log2))), c(0.1,.05,.01),log2$df.residual, two.sided=T, digits=3, apa=F) d3<-shuffle(log3$coef,sqrt(diag(vcov(log3))), c(0.1,.05,.01),log3$df.residual, two.sided=T, digits=3, apa=F) result1[,j]<-d1[3:16,2] result1[,k]<-d2[3:16,2] result1[,l]<-d3[3:16,2] rs1[j]<-1-(log1$deviance/log1$null.deviance) rs2[j]<-durbinWatsonTest(log1)$dw rs1[k]<-1-(log2$deviance/log2$null.deviance) rs2[k]<-durbinWatsonTest(log2)$dw rs1[l]<-1-(log3$deviance/log3$null.deviance) rs2[l]<-durbinWatsonTest(log2)$dw detach(ma1) } result1 # Coefficients, first 3 are males, next 3 are females and last 3 are individuals round(rs1,digits=3) #rsquared , first 3 are males, next 3 are females and last 3 are individuals round(rs2,digits=3) #dwstatistic, first 3 are males, next 3 are females and last 3 are individuals</p><p>### Contaction ### a<-c("./mdatay/Statewn/mnt6c.csv", "./mdatay/Statewn/fnt6c.csv" , "./mdatay/Statewn/bnt6c.csv") result1<- matrix(nrow=14,ncol=9) rs1<- vector(length=9) rs2<- vector(length=9) for( i in 1:3){ j=(i*3)-2 k=j+1 l=j+2 10 maa1 <- read.csv(file=paste(a[i]),head=TRUE,sep=",") ma1<-subset(maa1,Growth==0) attach(ma1) GDP=GDPPP/10000 MED=MEDPP/1000 Pop1=round(Pop,digits=0) dr=cbind(Deaths,Pop1)</p><p>#the model used by other researchers takes the form(not fitted) #mr<-(Deaths/Pop*100000) #weight=sqrt(Pop) #fixed1<-lm(log(mr)~U0+GDP+MED+EDUP+ABP+p5+p65+factor(Time) +factor(STATE),weights=weight) #fixed2<-lm(log(mr)~U1+GDP+MED+EDUP+ABP+p5+p65+factor(Time) +factor(STATE),weights=weight) #fixed3<-lm(log(mr)~U2+GDP+MED+EDUP+ABP+p5+p65+factor(Time) +factor(STATE),weights=weight) log1<-glm(dr~U0+GDP+MED+EDUP+ABP+p5+p65+factor(Time) +factor(STATE),family=quasibinomial(logit)) log2<-glm(dr~U1+GDP+MED+EDUP+ABP+p5+p65+factor(Time) +factor(STATE),family=quasibinomial(logit)) log3<-glm(dr~U2+GDP+MED+EDUP+ABP+p5+p65+factor(Time) +factor(STATE),family=quasibinomial(logit)) d1<-shuffle(log1$coef,sqrt(diag(vcov(log1))), c(0.1,.05,.01),log1$df.residual, two.sided=T, digits=3, apa=F) d2<-shuffle(log2$coef,sqrt(diag(vcov(log2))), c(0.1,.05,.01),log2$df.residual, two.sided=T, digits=3, apa=F) d3<-shuffle(log3$coef,sqrt(diag(vcov(log3))), c(0.1,.05,.01),log3$df.residual, two.sided=T, digits=3, apa=F) result1[,j]<-d1[3:16,2] result1[,k]<-d2[3:16,2] result1[,l]<-d3[3:16,2] rs1[j]<-1-(log1$deviance/log1$null.deviance) rs2[j]<-durbinWatsonTest(log1)$dw rs1[k]<-1-(log2$deviance/log2$null.deviance) rs2[k]<-durbinWatsonTest(log2)$dw rs1[l]<-1-(log3$deviance/log3$null.deviance) rs2[l]<-durbinWatsonTest(log2)$dw detach(ma1) } result1 # Coefficients, first 3 are males, next 3 are females and last 3 are individuals round(rs1,digits=3) #rsquared , first 3 are males, next 3 are females and last 3 are individuals round(rs2,digits=3) #dwstatistic, first 3 are males, next 3 are females and last 3 are individuals</p><p>### Distributed lag models ###</p><p>11 #Table 5 - estimating the effect of combined lags of unemployment rate on mortality using a distributed lag approach (in a fixed effects context) ### Expansion ### a<-c("./mdatay/Statewn/mnt6c.csv", "./mdatay/Statewn/fnt6c.csv" , "./mdatay/Statewn/bnt6c.csv") est2<- vector(length=3) se2<- vector(length=3) pt2<- vector(length=3) rs2<-vector(length=3) dw2<-vector(length=3) result2<- matrix(nrow=12,ncol=3) for( i in 1:3){ maa1 <- read.csv(file=paste(a[i]),head=TRUE,sep=",") ma1<-subset(maa1,Growth==1) attach(ma1) GDP=GDPPP/10000 MED=MEDPP/1000 Pop1=round(Pop,digits=0) dr=cbind(Deaths,Pop1) logi<-glm(dr~U0+U1+U2+GDP+MED+EDUP+ABP+p5+p65+factor(Time) +factor(STATE),family=quasibinomial(logit)) d1<-shuffle(logi$coef,sqrt(diag(vcov(logi))), c(.1,.05,.01),logi$df.residual, two.sided=T, digits=3, apa=F) result2[,i]<-d1[9:20,2] est2[i]<-sum(logi$coef[2:4]) af<-subset(vcov(logi), select = U0:U2) af2<-af[2:4,] se2[i]<-sqrt(sum(af2)) pt2[i]<-2*(1-pnorm(abs(est2[i]/se2[i]),mean=0,sd=1)) rs2[i]<-1-(logi$deviance/logi$null.deviance) dw2[i]<-durbinWatsonTest(logi)$dw detach(ma1) } round(est2,digits=3) # provides the estimate for unemployment round(se2,digits=3) # provides the standard error for unemployment round(pt2,digits=3) # provides the probability value for unemployment coefficient (used for finding significance) round(rs2,digits=3) # R-squared round(dw2,digits=3) # Dw Statistic result2 # other coeffients - GDP, Medical EXP, Education, ABP, P5 and P65</p><p>### Contraction ### a<-c("./mdatay/Statewn/mnt6c.csv", "./mdatay/Statewn/fnt6c.csv" , "./mdatay/Statewn/bnt6c.csv") est2<- vector(length=3) se2<- vector(length=3) pt2<- vector(length=3) rs2<-vector(length=3) dw2<-vector(length=3) 12 result2<- matrix(nrow=12,ncol=3) for( i in 1:3){ maa1 <- read.csv(file=paste(a[i]),head=TRUE,sep=",") ma1<-subset(maa1,Growth==0) attach(ma1) GDP=GDPPP/10000 MED=MEDPP/1000 Pop1=round(Pop,digits=0) dr=cbind(Deaths,Pop1) logi<-glm(dr~U0+U1+U2+GDP+MED+EDUP+ABP+p5+p65+factor(Time) +factor(STATE),family=quasibinomial(logit)) d1<-shuffle(logi$coef,sqrt(diag(vcov(logi))), c(.1,.05,.01),logi$df.residual, two.sided=T, digits=3, apa=F) result2[,i]<-d1[9:20,2] est2[i]<-sum(logi$coef[2:4]) af<-subset(vcov(logi), select = U0:U2) af2<-af[2:4,] se2[i]<-sqrt(sum(af2)) pt2[i]<-2*(1-pnorm(abs(est2[i]/se2[i]),mean=0,sd=1)) rs2[i]<-1-(logi$deviance/logi$null.deviance) dw2[i]<-durbinWatsonTest(logi)$dw detach(ma1) } round(est2,digits=3) # provides the estimate for unemployment round(se2,digits=3) # provides the standard error for unemployment round(pt2,digits=3) # provides the probability value for unemployment coefficient (used for finding significance) round(rs2,digits=3) # R-squared round(dw2,digits=3) # Dw Statistic result2 # other coeffients - GDP, Medical EXP, Education, ABP, P5 and P65</p><p>#Figures 1a and 1b win.metafile(file="C:/Plots/Fig_%d.wmf") par(mfrow=c(2,1)) ausm1 <- read.csv(file="C:/mdatay/National/ausb6.csv",head=TRUE,sep=",") attach(ausm1) mar<- Deaths/Pop</p><p>#the following creates a scatterplot (with a lowess smoother) of mortality rate vs unemployment rate plot(U0,mar, ylab="Mortality Rate", xlab="Unemployment Rate",main="Mortality Rate vs Unemployment Rate", sub="Raw Series",pch="*") lines(lowess(U0,mar,f=2/3)) cor.test(mar, U0)</p><p>13 #the following set of commands uses a cubic spline detrending on unemployment rate and log of mortality rates series and plot them togester in a time series plot z<-ts(log(mar),start=1985,frequency=1) x <- time(z)-1997 fit <- rlm(cbind(1,x,x^2,x^3),z) summary(fit) z2<-fit$residuals z<-ts(U0,start=1985,frequency=1) x <- time(z)-1997 fit <- rlm(cbind(1,x,x^2,x^3),z) summary(fit) z1<-fit$residuals ts.plot(z1,z2/2,ylab="Standard Deviations from Mean",main="Log(Mortality Rate) vs Unemployment Rate",lty=1:2,pch="*") legend("bottomright",legend=c("Unemployment rate","Log(Mortality Rate)"),lty=1:2,bty="n") detach(ausm1)</p><p>#Figure 2 - mortality rate at different levels of unemployment rate using median values of other covariates par(mfrow=c(1,1)) a<-c("C:/mdatay/Statewn/mnt6.csv", "C:/mdatay/Statewn/fnt6.csv" , "C:/mdatay/Statewn/bnt6.csv") pop<-matrix(nrow=3,ncol=11) for( i in 1:3){ ma1 <- read.csv(file=paste(a[i]),head=TRUE,sep=",") attach(ma1) GDP=GDPPP/10000 MED=MEDPP/1000 Pop1=round(Pop,digits=0) dr=cbind(Deaths,Pop1) logi<-glm(dr~U0+GDP+MED+EDUP+ABP+p5+p65+factor(Time) +factor(STATE),family=quasibinomial(logit)) g1<-median(GDP) m1<-median(MED) e1<-median(EDUP) ab1<-median(ABP) pl1<-median(p5) ph1<-median(p65) a2<-logi$coef[1:8] for( j in 1:11){ k=(j-1)/100 a1<-cbind(1,k,g1,m1,e1,ab1,pl1,ph1) b1<-a1%*%a2 pop[i,j]<-exp(b1)/(1+exp(b1)) } 14 detach(ma1) } ym<-pop[1,] yf<-pop[2,] yb<-pop[3,] xnew<-0:10 plot(xnew, ym, ylim=c(0.0057,0.0069), sub="Lag 0", xlab="Unemployment Rate (%)", ylab="Mortality Rate",pch="*") lines(xnew, ym,lty=1) points(xnew,yf,pch="*") lines(xnew, yf,lty=2) points(xnew,yb,pch="*") lines(xnew, yb,lty=3) legend("topleft",legend=c("Males","Females","Combined"),lty=1:3,bty= "n")</p><p>## The calculation below gives the value reported in the discussion section mean(diff(yb,lag=1))*100000 dev.off()</p><p>15</p>
Details
-
File Typepdf
-
Upload Time-
-
Content LanguagesEnglish
-
Upload UserAnonymous/Not logged-in
-
File Pages15 Page
-
File Size-