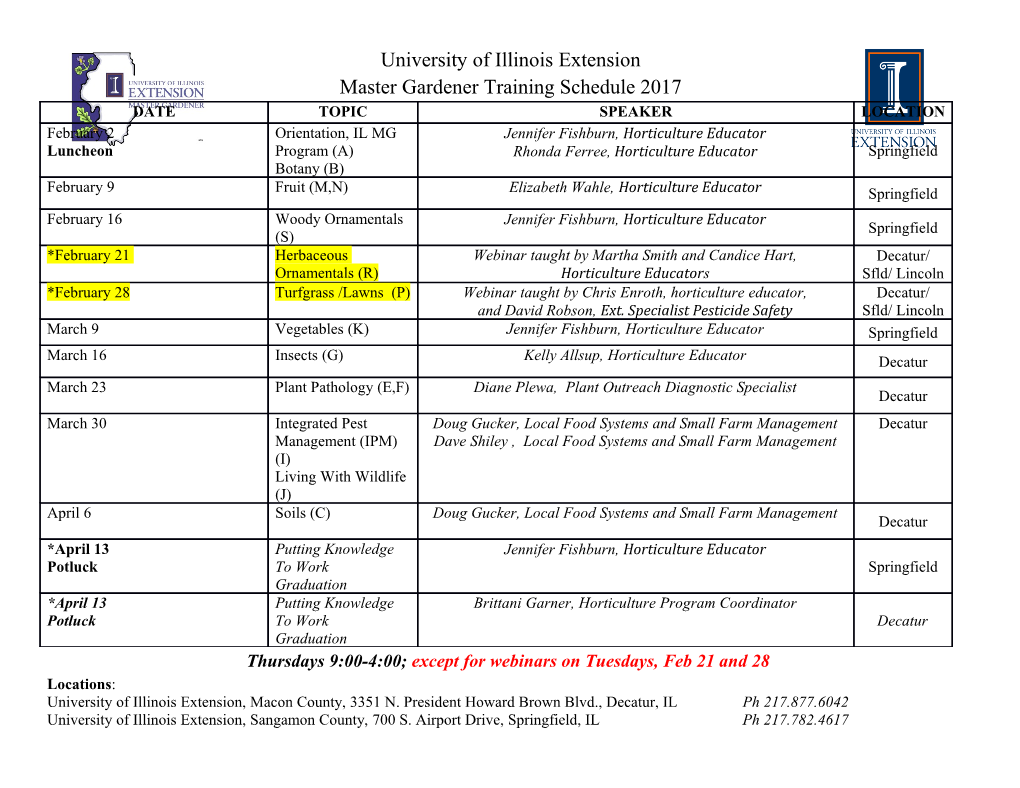
<p> 1</p><p>Wm Ross/MGT 486 Word file: (HP) c:selection~spss~Spring~2015 Data file (SPSS for Windows, version 22): Vt2015.sav Exercise last revised: Feb. 2, 2015 Assessing the Validity of a Selection System Using SPSS</p><p>For this exercise, please assume the following information: 1. As the new HR Manager at Metro Telecom you have gathered performance appraisal data from 70 recently- hired (within the past two years) Call Center Representatives (CCRs). The CCRs must have at least an 8th grade education and be at least 18 years old. Their main duties are to assist customers over the telephone or Internet chat as the customers want to change or upgrade their service. You want to know how these new CCRs are doing and whether you hired good ones (if not, you’ll need to change your selection methods). The appraisal system data for each CCR uses a “360-degree” performance evaluation system. It consists of averages of supervisor's, peers’, and customers’ various ratings on specific dimensions of performance. These included timeliness, courtesy to customers, knowledge of products, absenteeism, and interactions with co-workers and supervisors. Measures of performance were combined into a “composite performance appraisal score” for each rater. The composite scores from the different raters were then averaged. Performance (Y) was measured using seven-point rating scales with “1 = poor performance,” and “7 = excellent performance.”</p><p>2. The Metro system is planning a general hospital expansion. You will be hiring 14 new CCRs. Each will undergo one week of training and orientation when first hired prior to working.</p><p>3. After reviewing the published literature on selecting people for this job, you first tentatively identified three reasonable selection methods (predictors). In your data set (described below), these are labeled X1, X2, and X3. Their actual meaning is as follows:</p><p>(a) X1 “Customer Service Aptitude Profile (CSAP).” This is commercially – available test that measures traits such as diplomacy and cooperation. For a description of this test, see http://www.criteriacorp.com/solution/CSAP.php . It has good reliability and the test publisher claims it has good validity. However, you can’t just take the test salesman’s word on this – you need to see if it is valid for CCRs at your call centers. To facilitate ease of use, this will be administered as either a mobile phone app or computer-based test. The form of administration will be noted elsewhere in your data set – you can see if the scores are comparable as part of this project. [By the way, there is some concern that such tests can be faked (see Richard Frei’s 1998 dissertation entitled “Fake this test!...” in the PsychInfo database if you are curious about that topic); something to mention in your “Discussion” section???]</p><p>(b) X2 A Weighted Application Blank (WAB; also called “Biodata”) measure of a personality trait called “Service Orientation” (SO). This is a percentile score (0 – 99%) that measures whether the CCR helps put the customer at ease and is willing to do other things to help the customer feel that his/her needs and preferences are being considered. This is not a personality test; rather, this personality variable is assessed by information gathered from an application blank (see the Gatewood Field, and Barrick text for general information on WABs). The specific measure used here is described in the following articles. (1) McBride, A., Mendoza, J., & Carraher, S. (1997). Development of a biodata index to measure customer service orientation. Psychological Reports, 81, (3, pt. 2), 1395 – 1407. (2) Chait, H. N., Carraher, S., & Buckley, M. R. (2000). Measuring service orientation with biodata. Journal of Managerial Issues, 12, (1), 109 – 120. 2 (c) X3. The Typing Skill Test (TST). This is a 5-minute test of simple typing skills (no complex tables, for example). The rationale for using this test is that CCRs must enter order information (e.g., a change of address) into a computer while talking with the customer on the telephone. The test is published by the E. F. Wonderlic Personnel Testing Co., Inc. It was created in 1988. It is described in the following Murphy Library database: Mental Measurements Yearbook with Tests in Print (link): https://libweb.uwlax.edu/login?url=http://search.ebscohost.com/login.aspx? direct=true&AuthType=ip,uid&db=mmt&AN=test.1108&site=ehost-live&scope=site The “Net Words Per Minute” scores have been converted to percentiles, based on national norms, ranging from 0 to 99%ile. </p><p>Further, the measure is administered via computer as a timed, but un-proctored Internet-based test. </p><p>If necessary, you may want to change the labels of these three variables in your data set to something more descriptive than X1, X2, and X3 (in a seven-letters or fewer variable name) before conducting data analyses. You’ll also want to read about your predictors in the scientific literature. </p><p>4. Remember that general information about the formatting of your report is found in the first few pages of your syllabus. The literature review portion of your paper should discuss each of the three types of predictors that you are using – both in content and in method – and why you think that such a predictor might be useful for predicting performance on this type of job. While it might be hard to find references for the specific tests, you can probably find some literature for each type of predictor. Therefore, find references for – and discuss – each type of predictor! Your literature review should also discuss #5 – #7 (below).</p><p>5. You are using a Concurrent Validity procedure, with weighting and holdout groups. [Hint: Why are you using this procedure? What are the advantages of this procedure?]</p><p>6. You will be assessing the Decision Accuracy and Utility (return on investment) of your selection system.</p><p>7. Finally, you will be using Multiple Regression. </p><p>3 PREPARING THE DATA FOR ANALYSIS</p><p>Entering the data set. For this exercise, the data set may be sent to your campus e-mail address as an attachment and/or available from the D2L website and/or available on my UW-L website (http://websites.uwlax.edu/wross/mgt486.htm) .</p><p>Here are some additional points to consider: 1. Each line of data represents one employee's data. The meaning of each variable is also summarized below:</p><p>Variable Name What it means (plus scale range) ID Employee Identification Number x1 Your 1st predictor, measured out of 100. x2 Your 2nd predictor, measured out of 100. x3 Your 3rd predictor, measured out of 100. </p><p>To change the variable label, click on the “file folder tab” at the bottom of the screen. This takes you to the “variable” information, where you can click on the appropriate cell and retype the name of the variable and can also type a descriptive label if you wish.</p><p> y Average performance rating, measured on a 7-point rating scale (1=poor, 4=average, 7=excellent performance).</p><p>App Whether x3 was administered via regular computer or mobile device, such as an iPad or iPad Mini with a bluetooth keyboard; the mobile version uses especially-created software for such a device called an “app” (short for “application”). 1 = regular computer 2 = mobile device app</p><p>In addition to the above data, information is included about various demographic groups. You will not compare these groups in this exercise, but will in Part III. Therefore, you should save your data file on a diskette, flash drive, or other device for later use.</p><p>Race/ Ethnic Group This particular hospital is in an area with a lot of older citizens and a large Asian-American population. You wonder whether your new selection system is fair to all applicants. Of course, these are issues for Part III – do NOT analyze these data for Part II of your project.</p><p>Code % in surrounding 3-county area: Age 1= Under 40 70% 2= 40 or Older 30%</p><p>Sex 1= Ladies 50% 2= Gentlemen 50%</p><p>Race 1= Asian-American 35% 2= Mainstream (White) 65% 4</p><p>"Housekeeping" matters (some of these tips work with some versions of SPSS but not all versions):</p><p>Adding titles to your output: In order to identify your printouts, when viewing output, please click on the utilities menu: File Edit View Insert Format Analyze Statistics Graphs Utilities Windows Help</p><p>Select New Title from the following Menu: Page Break... Clear Page Break... New Heading... New Title... New Text…</p><p>You will see an empty title box: Type your name. You should type something like this (and then click somewhere else on the page): [ Prepared by John Doe and Rip Van Winkle (type YOUR names) ]</p><p>If you desire, you can type explanatory comments, clarifying labels, etc. in your output file and these comments will appear on your printout. Thus, for example, imagine that you just selected younger workers, performed some analyses, and then selected older workers and did the same analyses on this second sub-sample. You might find it valuable to type some labels in your output file so you won't forget which part of the printout goes with each group--especially if they are the same sample size! It is easy to do but SPSS keeps changing the way to do it. Try one of the following: </p><p>(1) When in your output window, just put the cursor where you want the label or comment, click, and then type.</p><p>(2) You can usually double-click a label in your output (e.g. Regression) and then add text to the right of the heading. For example, you can turn it into Regression – Younger Workers Only.</p><p>(3) You can click on Insert... New Text and type a text box (with a smaller font than a new title).</p><p>Avoiding printing unnecessary pages: Note that the output from each new analysis gets added to the end of the output file. If you print the entire file after each specific analysis, you will get that analysis' results but you will also get all of the output from the analyses that preceded it. For example, if you do three analyses and print the entire file after each analysis, you will wind up with three printouts of the first analysis, two printouts of the second analysis, and one printout of the third analysis. </p><p>How do you avoid this problem? (1) If you plan to complete the entire exercise at one sitting, you may wish to wait to print your output until you have completed the entire exercise. (2) When printing, the computer asks if you want to print the entire file or a designated portion. The default option is the entire file. However, if you click on the designated portion box, you can specify exactly what you want to print. Simply drag the mouse over the block of output text that you want to print. (3) Perform an exercise, print your results, exit the data file, reopen the file, and continue with the next analysis. Repeat after each printing. 5 SECTION A: FINDING THE SINGLE BEST PREDICTOR </p><p>Selecting your "Weighting" Sample: For this part of the exercise, you will select the first 50 cases of the sample of 70. These 50 will serve as the "weighting group." You will use these to develop your selection weights for your regression equation. </p><p>Please click on the Data menu:</p><p>File Edit View Data Transform Analyze/Statistics Graphs Utilities Add-ons Window Help</p><p>From this menu, click on Select Cases to see the "Select Cases" Dialogue Box. This box consists of a list of variables in the left column and a list of selection options in the right column. </p><p>Click on the circle to the left of the If condition is satisfied option. </p><p>Next, click on the [If...] box.</p><p>This brings you to the "Select Cases: If" Dialogue Box, containing your list of variables in the left column and room for your "select if" equation in the right column. </p><p>Use the down-arrow key or mouse to highlight the ID variable, then click on the [>] box to put ID in the equation box. Next click on the [<=] key. You should type this equation: ID <= 50</p><p>What does this mean? It means select the first 50 subjects as your "weighting sample!" {Quick, ask yourself: how would you write an equation to select subjects 51-70? Don't write such an equation now; I just want you to think about how you would do it.}</p><p>Make sure the Filter out unselected cases option is chosen.</p><p>Click [ continue ]</p><p>Click [ OK ] 6 Performing Regression Analysis Now, you will perform three regression analyses -- one for each of your three chosen predictors. You will use the Linear Regression statistic. This will give you both the correlation coefficient and the equation for the line that best fits the data). </p><p>Please click on the Analyze (or “Statistics” in some versions) option from the menu at the top of the screen:</p><p>File Edit Data Transform Analyze Graphs Utilities Windows Help</p><p>Select Regression from the following menu: Reports Descriptive Statistics Tables Compare Means General Linear Models Generalized Linear Models Mixed Models Correlate Regression Loglinear Classify Dimension Reduction Scale Nonparametric Tests Forecasting Survival Multiple Response… ROC Curve</p><p>There are different types of regressions, so please select Linear ... </p><p>This opens the "Linear Regression" Dialogue Box :</p><p>Variables: Dependent Variable: [ Statistics] id [>] [ ] x1 (or the name you gave it) [ Plots] x2 (or the name you gave it) x3 (or the name you gave it) [ Previous] Block 1 of 1 [ Next ] y : Independent Variable(s): [ Save] : [ ] [>] [ ] [ Options]</p><p>Method: [ Style] [ ][] Selection Variable: [ Bootstrap] [>] [ ][Rule] Case Labels: [>] [ ] WLS Weight [ ] [OK] [Paste] [Reset][Cancel] [Help]</p><p>Note: Some versions of SPSS switch the bottom row of buttons with the row of buttons in the right column. 7 You want to predict overall job performance (y). So y will be your dependent variable. Use the mouse to move the cursor over the y variable in the variable list. Click on the [>] key to put y in the Dependent Variable Box. This will not change for your three analyses. Next, put x1 (or whatever name you gave this variable) in the Independent Variables box for your first analysis. You should see the following:</p><p>Variables: Dependent Variable: [ OK ] id [>] [ y ] x1 (or the name you gave it) [ Paste] x2 (or the name you gave it) x3 (or the name you gave it) [ Previous] Block 1 of 1 [ Next ] y : Independent Variable(s): [ Reset] : [ x1 ] [>] [ ] [Cancel]</p><p>Method: [ Enter ][] [ Help]</p><p>[ WLS>>] [ Statistics ] [ Plot ] [ Save ] [ Options ]</p><p>Next, click on the [ Statistics ] button. From this, select [ ] Regression Coefficient Estimates, [ ] Model Fit, [ ] R2 Change, [ ] Descriptives, then click on [Continue].</p><p>Include printouts of your scattergrams! If you want to create a scattergram, click on [ Plots ]. Put DEPENDNT in your Y: box and put *ZPRED in your X: box. Then click [ Continue ]. You do not need to worry about the "selection variable" or "case label" dialog boxes; leave them blank.</p><p>You are now ready to run the regression for x1. Click on [ OK ]. You will see a printout looking similar to, but not exactly like this (of course, you’ll have different numbers for your printout):</p><p>What it is: What it Means: </p><p>Multiple R .70 This is the same as a correlation coefficient when there</p><p> is only one predictor. It is properly written as "rx1y" and varies from .00 (no relationship) to 1.00 (a perfect linear relationship) R Squared .49 This means that x1 is explaining 49% of the variation in y. If the predictor was perfectly related to y, it would explain 100%. Adjusted R Square .47 This says "if you were to test this predictor on a second sample, what is the estimate of the R Square? It is usually a bit lower than the actual R square reported on the previous line because SPSS assumes that you probably capitalized on chance covariation. 8 Standard Error 1.09 This is like a standard deviation only it is for the regression line. Imagine a regression line (line that best fits the data) with two parallel lines near it (one above and one below the regression line) that are 1.09 y-units away. Such a line would tell you where most scores will fall if you plot x1 against y. The larger the Standard Error, the less confidence we have in our ability to predict. Analysis of Variance: F = 17.92 Sig. F = .001 This simply tells us that there is, indeed, a relationship between x1 and y. The probability (significance or "sig.") that such a large F statistic would emerge by chance alone is very small -- only one time in one-thousand (.001). If it is not due to chance, it must be due to a real effect. Stated differently, it tells us that the correlation (r) is different from zero. So yes Virginia, in this example there is a significant correlation: x1 predicts job performance (y). Note that the T value in the equation below also tells you the same thing for x1. When there is more than one predictor, the F-statistic tells you whether the overall equation predicts y, and the T-statistics tell you whether each individual component is significant (and belongs in the equation).</p><p>------Variables in the Equation ------</p><p>Variable Name B SE B Beta T Sig. T X1 1.14 .11 .70 8.96 .0001 (Constant) 3.14 .04 99 .0001</p><p>What does this mean? It means that the equation for the regression line is: predicted y = 1.14 (x1) + 3.14 | | 1.14 is 3.14 represents the intercept of the line. the "B" It is the "B" value for the intercept, value for (which is also called the constant). x1 and represents the weight multiplied by x1. It is the slope of the line.</p><p>If a job applicant took the x1 test, we would predict his performance appraisal score using this regression equation. If we had several applicants and one opening, we could give them all the x1 test, use the equation to calculate predicted performance, and then hire the one with the best predicted performance appraisal score. 9 The above was useful for understanding the concepts, but unless you are using a really old version of SPSS, your results won’t look like that. They will probably appear in “box tables” such as the ones shown below:</p><p>Coefficientsa</p><p>Unstandardized Standardized Coefficients Coefficients Model B Std. Error Beta t Sig. 1 (Constant) : 3.14 .04 99 .000</p><p>X1: 1.14 .11 .70 8.96 .000</p><p> a. Dependent Variable: Y: Overall Job Performance Measure (last performance appraisal)</p><p>The box table above tells us that the predicted value of Y = 3.14 + 1.14(X1 score). </p><p>ANOVA b Model Sum of Degrees of Mean Square F-statistic Significance Squares Freedom (df) (p) 1 Regression 99.51 1 49.755 23.67 .000 a Residual 416.176 199 2.102 Total 515.686 200 Footnotes: a. Predictors: (Constant), X1 b. Dependent Variable: Y</p><p>The above table tells us that, overall, our model (Model 1: using X1 to predict Y) is significantly different from zero. An F value this large (23.67) could be expected by chance less than one time in 1000. Since it is not a chance finding, it must be a ‘real’ finding. This overall effect is written as: F(1, 199) = 23.67; p<.001.</p><p>You will also obtain a second box table such as the following:</p><p>Model Summary b Model R R Square Adjusted Std. Error Change Statistics R Square of the R- F df 1 df 2 Sig. of F Estimate square Change Change Change 1 .43 .188 .184 1.45 .188 23.67 1 199 .000 a Footnotes: a. Predictors: (Constant), X1 b. Dependent Variable: Y</p><p>Model 1 only contains X1 as a predictor for Y, as indicated in footnote “a.” It produces a multiple R of .43 (with only one predictor, the multiple R is the same as a simple Pearson correlation r). This variable explains 18.8% of the variation in Y. Since we only have one variable in the model, the Change statistics are the same as those for the model, overall. If we were testing different models (for example, comparing Model 1 with only X1 with a Model 2 containing both X1 & X2), the change statistics would tell us whether the new model significantly improved our ability to predict compared to Model 1. You will make such comparisons in the next section (Section B) below.</p><p>Now, look at YOUR data. How large is your R? Is it different from zero? Does it explain a large percentage of the variation in y? What is your regression equation? 10 Depending upon which version of SPSS you are using, if you have a scattergram, it will appear either at the end of the printout (newer versions) or in a separate "Chart Carousel" file (older versions). If there is a Chart Carousel, click on this file name to see the plot. If there is no chart carousel, if you double-click on the scatterplot, it will open a chart editor. Select the “Add Fit Line At Total” to add a “line of best fit” to your graph. If you can, print your plot and include it in your report.</p><p>Next, repeat the process, only use x2 instead of x1. From the main menu, select Analyze/Statistics. Next select Regression. Then click on Linear... </p><p>In the "Linear Regression" Dialogue Box, you will see the following:</p><p>Variables: Dependent Variable: [ OK ] id [<] [ y ] x1 (or the name you gave it) [ Paste] x2 (or the name you gave it) x3 (or the name you gave it) [ Previous] Block 1 of 1 [ Next ] y : Independent Variable(s): [ Reset] : [ x1 ] [<] [ ] [Cancel]</p><p>Method: [ Enter ][] [ Help]</p><p>[ WLS>>] [ Statistics ] [ Plot ] [ Save ] [ Options ]</p><p>Click on X1, then click on the [<] button next to the Independent Variable(s) box to move x1 back to the variable list. After you do this, the arrow on the button will change direction to [>]. Then highlight x2 and click on the [>] button to move x2 into the Independent Variable(s) box. Then click on the [OK] button to run the regression.</p><p>How do these results look? Is the rx2y large? Does it explain much of the variation in y scores? Is it significantly different from zero? Is the r2 value larger than that that you found earlier for x1? What is the regression equation?</p><p>Finally, repeat this process for your third predictor (x3). </p><p>Of the three predictors, which is the single best predictor of job performance (y)? 11 SECTION B: FINDING THE BEST COMBINATION OF PREDICTORS USING MULTIPLE REGRESSION </p><p>Your previous regression told you how well each individual predictor did at predicting job success. But how well do all three predictors do? Will only one predictor work, or do you need all three? Or do you just need the best two? This next analysis (again using just the weighting sample of the first 50 cases) will help you make such decisions. If you are re-opening your data file after leaving SPSS, you may need to select the weighting sample again, as described on page 4.</p><p>For this section of the exercise, you will again use regression. However, this time, you will have more than one predictor.</p><p>First, identify your single best predictor (the variable with the largest r value). Is it x1? x2? or x3?</p><p>From the main menu, select Analyze/Statistics. Next select Regression. Then click on Linear... </p><p>In the "Linear Regression" Dialogue Box, you will see the following:</p><p>Variables: Dependent Variable: [ OK ] id [>] [ ] x1 (or the name you gave it) [ Paste] x2 (or the name you gave it) x3 (or the name you gave it) [ Previous] Block 1 of 1 [ Next ] y : Independent Variable(s): [ Reset] : [ ] [>] [ ] [Cancel]</p><p>Method: [ Enter ][] [ Help]</p><p>[ WLS>>] [ Statistics ] [ Plot ] [ Save ] [ Options ]</p><p>Highlight y and click on the [>] to move it into the Dependent Variable box.</p><p>Select your single best predictor (x1 or x2 or x3—see your printouts from Section A) from your variable list and highlight it.</p><p>Click on the [>] button to move that variable into your Independent Variable(s) box.</p><p>If this was all you did, it would give you the same results that you found in Section A. So we will go further with an additional analysis. What you have entered is the first step (block). Next, you want to enter an additional predictor for the second step. 12 Look at the portion of the dialogue box identified as: </p><p>[ Previous] Block 1 of 1 [ Next ]</p><p>Click on the [ Next ] button. The Block number should increase to Block 2 and there should not be any variables in the Independent Variable(s) box. Highlight your second-best predictor (the one with the second-highest correlation with Y --from Section A) and move it into the Independent Variable(s) box. Next, click on the [ Next ] button and enter the third variable for Block 3. After this, you can click on the [ OK ] button to run the regression.</p><p>Suppose you entered the variables in this order: Block one: x1, Block two: x2, Block three: x3.</p><p>You should see results that look something like this (only with different numbers with your data):</p><p>Equation Number 1 Dependent Variable: y</p><p>Block 1: Method: Enter. Requested variable entered:x1 Multiple R .70 R Squared .49 Adjusted R Squared .59 Standard Error 1.09</p><p>Analysis of Variance: : : F = 17.92 Sig. F = .001</p><p>------Variables in the Equation ------</p><p>Variable Name B SE B Beta T Sig. T X1 1.14 .11 .78 17.96 .0001 (Constant) 3.14 .04 99 .0001</p><p>{plus some statistics about the variables NOT yet in the equation, which you can ignore} : : End Block 1/Model 1. All requested variables entered.</p><p>Sometimes the B weights are expressed with exponential (scientific) notation. Thus, if the B weight was written as 1.14E-02, then the weight is really .0114. The general rule of thumb is move the decimal to the left the number of “places” that corresponds to the number to the right of the “E.” So if the number is 2.22E-03, it is really .00222; if the number is 2.22E-04, it is .000222, etc. You may experience this, as your predictors are on 100-point scales, but job performance is on a 7 point scale. 13 The above information is identical to what we obtained when we used ONLY x1. But since we went beyond that, and entered x2 at Block 2, we get a second part of the printout:</p><p>Block 2/Model 2: Method: Enter. Requested variable entered: x2</p><p>Multiple R .83 R Squared .69 Adjusted R Squared .64 Standard Error 1.33</p><p>Analysis of Variance: : : F = 21.98 Sig. F = .001</p><p>------Variables in the Equation ------Variable Name B SE B Beta T Sig. T X1 1.14 .11 .74 17.96 .0001 X2 1.21 .25 .25 5.07 .03 (Constant/intercept) 3.56 .07 97.09 .0001</p><p>{plus some statistics about the variables NOT yet in the equation, which you can ignore} : : End Block 2/Model 2. All requested variables entered.</p><p>First note that the Multiple R went up from .70 to .83 when x2 was added as a predictor. Also, note that while some of the regression equation statistics for X1 and the intercept (constant) changed, they did not change much. The new equation (using both predictors) is:</p><p> predicted y = 3.56 + 1.14 (X1) + 1.21 (X2)</p><p>Ask: Did adding a second variable improve our R2 significantly? Do all variables in the equation have significant T values? (yes, all the p-values are less than .05; you can ignore whether the constant is significant -- that just tells you whether the intercept differs from zero). In this example, the answer to both questions is "yes." If the answer had been "no" then that would tell us that the second variable added nothing to our first variable's ability to predict.</p><p>Suppose we then obtained the following results with all three predictors:</p><p>Block 3/Model 3: Method: Enter. Requested variable entered: x3</p><p>Multiple R .85 R Squared .72 Adjusted R Squared .70 Standard Error 1.94</p><p>Analysis of Variance: F = 29.58 Sig. F = .001 14 ------Variables in the Equation ------</p><p>Variable Name B SE B Beta T Sig. T X1 1.12 .11 .73 17.62 .0001 X2 1.20 .24 .22 5.02 .03 X3 .44 .14 .12 2.88 .09 (Constant/intercept) 4.16 .06 94.23 .0001</p><p>End Block 3/Model 3. All requested variables entered.</p><p>First note that the Multiple R went up from .83 to only .85 when x3 was added as a predictor. Also, note that while some of the regression equation statistics for X1 and the intercept (constant) changed, they did not change much. The new equation (using all three predictors) is:</p><p> predicted y = 4.16 + (1.12 *X1) + (1.20 *X2) + (.44 *x3)</p><p>Ask: Did adding a third variable improve our R square significantly? (not in this example) Do all variables in the equation have significant T values? (no, x3 is not significant; the p value is .09 and is not less than .05). In this example, we are no better off with all three predictors than if we just used x1 and x2. So we would use only the equation reported after Block 2, containing only x1 and x2. ::::::::::::::::::::</p><p>The above was useful for understanding the concepts, but unless you are using a really old version of SPSS, your results will probably appear in a series of “box tables” such as that shown below (different data, but illustrative of the formatting):</p><p>ANOVA c Model Sum of Degrees of Mean Square F-statistic Significance Squares Freedom (df) (p) 1 Regression 99.51 1 49.755 23.67 .000 a Residual 416.176 199 2.102 Total 515.686 200 2 Regression 270.137 2 135.069 108.914 .000 b Residual 245.548 198 1.240 Total 515.686 200 Footnotes: a. Predictors: (Constant), X1 b. Predictors: (Constant), X1, X2 c. Dependent Variable: Y</p><p>The above table tells us that, overall, Model 1 (using X1 to predict Y – see the “Footnotes” at the bottom of the table) is significantly different from zero. It also tells us that, overall, Model 2 (using X1 & X2 together to predict Y) is also significantly different from zero. Ah! But does adding X2 add anything to our ability to predict Y? Or is it that X1 explains almost all of the variance in both equations? To answer that, we must look at the “Model Summary” table below: 15</p><p>Model Summary c Model R R Square Adjusted Std. Error Change Statistics R Square of the R- F df 1 df 2 Sig. of F Estimate square Change Change Change 1 .433 .188 .184 1.45 .188 23.67 1 199 .000 a 2 .724 .524 .519 1.11 .336 139.74 1 198 .000 b</p><p>Footnotes: a. Predictors: (Constant), X1 b. Predictors: (Constant), X1, X2 c. Dependent Variable: Y</p><p>Model 1 only contains X1 as a predictor for Y, as indicated in footnote “a.” For this example, it produces a multiple R of .43 (with only one predictor, the multiple R is the same as a simple Pearson correlation r). This variable explains almost 19% (18.8%) of the variation in Y. Since we only have one variable in Model 1, the Change statistics are the same as those for the Model. Comparing Model 1 (with only X1) with Model 2 (containing both X1 & X2), the change statistics tells us that the new model significantly improved our ability to predict compared to Model 1. Thus, adding X2 improves our ability to predict by 33.6% (notice that 18.8 + 33.6 = 52.4%). This 33.6% is a significant increase (F change (1,198) = 139.74; p<.001). Another table (not shown here) gives the B-weights and the “constant” (the constant is the intercept – where the regression line crosses the Y-axis, if you are plotting predicted-Y score vs. Actual Y score). This is done for each model so you can report your regression equation for whichever model you select. </p><p>In addition to reporting the final regression equation that you settle on, you should summarize your findings in your paper with a table like this:</p><p>STEP # VARIABLE ENTERED R R 2 Change in R 2 1 x1 .70* 49% --- 2 x2 .83* 69% 20% 3 x3 .85 72% 3% *=significant (p<.05).</p><p>Extra Credit opportunity (5% maximum): Do additional multiple regressions, entering the three variables in different orders. Complete tables like that above for each analysis. Be sure to discuss your findings in your paper and remind me that you did it for up to 5% extra credit. Of course, when all three variables are in the equation, the results are the same, but what about when there are only two variables in the equation? What combination of predictors seems optimal? For example, in the above example, suppose x1 and x3 together gave an R2 of 71% -- the two variables together were almost as predictive of job performance as all three -- and cheaper to administer than all three! 16 Finally, you will get a table that shows you what the “B” weights look like for your data. The tables you find with most versions of SPSS look like the chart below (this table is not from your actual data): Coefficientsa Standardized Unstandardized Coefficients Coefficients Model B Std. Error Beta t Sig. 1 (Constant) 2.501 .458 5.990 .001 X3 .028 .008 .467 3.997 .001 2 (Constant) .333 .708 .996 .681 X3 .027 .008 .462 3.899 .001 X2: .031 .007 .430 3.992 .001 3 (Constant) -.089 .738 -.199 .910 X3: .029 .008 .414 3.499 .001 X2: .028 .007 .377 3.999 .005 X1: .009 .016 .194 1.991 .210 a. Dependent Variable: Y: Overall Job Performance Measure (last performance appraisal)</p><p>From this chart you can see that there are three models for predicting job performance (Y’): *Model #1, with only X3 entered. *Model #2, with X3 entered, and then X2 *Model #3, with X3 entered, and then X2 and then X1. The “Constant” is the intercept. The equations for each model (using the “B” weights) are as follows: *Model #1: Y’= a+B3X3. Plug in the numbers and you get: Y’ = 2.501 + (0.028 * X3) *Model #2: Y’= a+B3X3 + B2X2. In this example: Y’ = .333 + (0.027 * X3) + (0.031 * X2) *Model #3: Y’=a+B3X3 + B2X2 + B1X1. Here: Y’= -.089 + (.029 * X3) + (.028*X2) + (.009*X1)</p><p>Notice that, for Model #3, X1 got a small weight. It was also not significant in the T-test (p = .21). So maybe it is not worth including that variable in the equation. Of course it also depends on the other charts; if it adds a significant amount of variance in Y that you can explain (based on R-Square Change results from the “Model Summary” table, similar to that on pg. 14), then maybe it is worth including!</p><p>IMPORTANT: Using your R (or R2) values from your multiple regression(s) and your three single- predictor regressions, decide NOW what predictor(s) you tentatively recommend. Also, write out your regression equation below for future use. You will find the components for this equation on the printout. Which printout? The one of the regression using the predictor(s) you want (see sample equations on pp. 12-13 and this page). So (1) know your predictor(s) and (2) write down the regression equation from the printout. You will need this information when you complete SECTION C.</p><p>My Equation: predicted y = ______17 SECTION C: TESTING THE REGRESSION EQUATION ON THE CROSS-VALIDATION SAMPLE </p><p>In the previous analysis, you decided whether to use one predictor, two predictors, or three predictors and you determined what the best regression equation would be. Well, now you will test the equation that you derived from your weighting sample on the remaining cases in your larger sample.</p><p>How do you do this? One overall estimate of whether your prediction equation is accurate with the second sample is to first compute a predicted y (called y^ or y’) and then correlate y and y^. If the correlation is quite high then the equation is a good one; it is stable across samples. If the correlation is low, then that suggests that the equation is not useful for other samples; a much larger "weighting sample" is needed to derive an appropriate regression equation.</p><p>Selecting your "Cross-Validation" Sample: For this part of the exercise, you will select the last 20 cases of the sample of 70. These will serve as the "cross-validation" or "holdout" group. You will use these to test the regression equation that you previously derived on the "Weighting" sample. </p><p>Please click on the Data menu:</p><p>File Edit Data Transform Analyze/Statistics Graphs Utilities Windows Help</p><p>From this menu, click on Select Cases to see the "Select Cases" Dialogue Box. This box consists of a list of variables in the left column and a list of selection options in the right column. Click on the circle to the left of the If condition is satisfied option. Next, click on the [if...] box.</p><p>This brings you to the "Select Cases: If" Dialogue Box, containing your list of variables in the left column and room for your "select if" equation in the right column. </p><p>Use the down-arrow key or mouse to highlight the ID variable, then click on the [>] box to put ID in the equation box. Next click on the [>] key. You should type this equation: ID > 50</p><p>This will select cases 51 through 70.</p><p>[continue] [OK] 18 Calculating y^ for the Cross-Validation Sample</p><p>From the main menu choose Transform Next, choose Compute... This opens the following "Compute Variable" Dialogue Box: </p><p>Target Variable: Numeric Expression: [ ] = [ ] [ Type & Label ] [ ] [>] Variable List: [ id ] [+] [<] [>] [7] [8] [9] Functions: [] [ x1 ] [-] [<=][=>] [4] [5] [6] [ x2 ] [*] [=] [~=] [1] [2] [3] [ ABS ] [ x3 ] [/] [&] [|] [0] [.] [ : ] [ y ] [**][~] [()] [ Delete ] [ : ] [ : ] [If...] [ : ] [ OK ] [ Paste ] [ Reset ] [ Cancel ] [ Help ]</p><p>In the Target Variable box, type ypredict (for y--predicted). Click on the [ Type & Label ] button to add the label y--predicted. Then click on the [ Continue] button.</p><p>At the end of SECTION B, you were asked to identify your recommended predictors and your regression equation, "for future use." Well, the future has arrived. In the Numeric Expression box, type your regression equation, highlighting the variable names in your Variable List and clicking on the [>] to move them into the box. You can also click on the number pad or function keys as needed to construct the formula. For example, if we decided to only use two variables, our equation (determined from our Multiple Regression in Section B) might be: 3.56 + (1.14 * x1) + (1.21 * x2) {this is only an example!!! use YOUR equation!!! By the way, each weight will probably have a zero after the decimal (4.53E02 is “exponential notation” and means .0453) because you are trying to predict something on a 7-point scale from predictors that use 100-point scales.}</p><p>When finished, your "Compute Variable" Dialogue Box should look something like this (only with YOUR regression equation in the Numeric Expression box): </p><p>Target Variable: Numeric Expression: [ ypredict ] = [ 3.56 + (1.14 * x1) + (1.21 * x2) ] [ Type & Label ] [>] Variable List: [ id ] [+] [<] [>] [7] [8] [9] Functions: [] [ x1 ] [-] [<=][=>] [4] [5] [6] [ x2 ] [*] [=] [~=] [1] [2] [3] [ ABS ] [ x3 ] [/] [&] [|] [0] [.] [ : ] [ y ] [**][~] [()] [ Delete ] [ : ] [ : ] [If...] [ : ] [ OK ] [ Paste ] [ Reset ] [ Cancel ] [ Help ] Click on the [ OK ] button. 19 Correlating y^ with y for the Cross-Validation Sample</p><p>Now that you have computed ypredict (predicted y or y^), you'll want to correlate it with actual job performance to see if the regression line gives you accurate predictions. If the correlation is very high, then that indicates that the predicted scores are very close to the actual scores.</p><p>From the main menu select analyze (or “statistics” depending on the version of SPSS you have). Next, select Correlate. Select Bivariate.</p><p>You will see the following "Bivariate Correlation Dialogue Box:"</p><p>Variables Variables to [ Options ] in Data Set: Correlate: [ Style ] [ID [ ] [ Bootstrap ] x1 x2 x3 y : [ > ] : : ypredict ]</p><p>Type of Correlation Coefficients to use: [x] Pearson [ ] Kendall's tau-b [ ] Spearman</p><p>Test of Significance [x] Two-tailed [ ] One-tailed</p><p>[x] Flag Significant Correlations or Display actual significance level [ OK ] [ Paste] [ Reset] [ Cancel] [ Help]</p><p>You will need to "move" the variable names y and ypredict from the "Variables in data set" list to the "Variables to correlate" list. Do this by putting the cursor on the name of the first variable. Then move the cursor over the [ > ] box and click the mouse. This will move the variable name into the "variables to correlate" list. Repeat for the other variable. This way, you can correlate the two variables. </p><p>Do NOT change any of the default boxes (e.g., Type of Correlation Coefficient).</p><p>Click on [ OK ] to obtain your correlation. 20 You should see results that look something like this (only perhaps not as large):</p><p>VARIABLE Y VARIABLE YPREDICT VARIABLE Y 1.00 .7733 N (20) N ( 20) SIG=.000 SIG=.000</p><p>In this example, variable Y is perfectly correlated with itself (r = 1.00), which is exactly what you would expect, so ignore it. More generally, the r is the indicator used for the value of the correlation coefficient statistic. If you are hoping for a relationship, then you want a large r value. </p><p>Variable Y is also highly correlated with Variable YPREDICT (ry,ypredict = .7733, p < .001). This means that as the predicted job performance score (YPREDICT) increases, actual job performance (Y) also increases. If the significance or probability level (written as p in your report) is less than .05, the relationship is significant, that is, not likely to be a chance relationship. You want to see if variables have a SIG=.05 or smaller. In this case . 000 is very small indeed. It is less than 1 chance in 1000.</p><p>So...if you want a significant relationship, then you want a LARGE r AND a small p value! Got it?</p><p>Suppose a correlation (for demonstration purposes only) is NOT "statistically significant" (for example, ryz = . 1309; p = .22, ns). Such a value shows a positive relationship, meaning as Variable X increases, Z also tends to increase; however, the relationship between the two variables is so slight that it is simply what one would expect by chance -- there is not a real relationship. If you are discussing such findings in the text of your report, you should include the probability level, followed by "ns" for "not significant." In your report, you will probably want to include a clean copy of your correlation table. </p><p>Housekeeping Matter: To print output, click on the printer icon in the upper left part of the screen. Click on the minimize button in the upper right-hand corner of the window (or the [x]) to minimize the “output” window and to continue analyzing your data. Or, click on window and you will see a drop-down menu of files—one has the name of the data set and one has the output file. Double-click on the data set to see that file on the screen. Note: Versions of SPSS may vary in how to print files and in how to “toggle” back and forth between your data set and your output files.</p><p>Is YOUR correlation between y and ypredict a high correlation (e.g., between .40 and 1.00 with a p < .01)? Or a low correlation (close to 0.00 -- or not significantly different from zero)? Or somewhere in between?</p><p>What do you conclude about the generalizability of your regression equation?</p><p>{If it helps you explain and/or understand your results, you could examine your datafile with the ypredict and y variables included for the people in the cross-validation sample. Then you could mention an example or two in your paper. "For Employee #81, the predicted performance level was 4.233; this was very close to his actual average performance level of 4.20, as shown in a printout of the data in the Appendix...” [I just made up this example—you should offer examples based on your data]. If you want to check your work, do this: go back to just the “weighting sample” – the first 50 cases – and do a bivariate correlation (the commands would be “analyze…correlate...bivariate” between y and ypredict), you should get the exact same regression results that you got in Part B when you did the work that produced your regression equation (p. 12). If you don’t get the same R (or r) value, then you probably mis-typed your equation (Obviously, the correlation between y and y- predict is usually higher for the weighting sample than it is for the holdout sample).}</p><p>21 SECTION D: CALCULATING DECISION ACCURACY STATISTICS</p><p>Assume that the minimal level of acceptable performance for this job is an average of 3.41 on a 7-point rating scale. If you assume that the 70 employees are representative of the population of minimally-qualified job applicants, then you can use this rating threshold to compute the Base Rate, correct?</p><p>Further assume that Metro Hospital will be hiring 14 new people and that it is not uncommon to have five applicants for each job opening (what type of Selection Ratio is that?). Further assume that the applicant's distribution of scores on each variable will be similar to the distribution you have in your database for current employees.</p><p>Your purpose for this section: You want to compute decision accuracy statistics to see if you are hiring the "right" people (good workers) with your new selection system. For this section we will use ALL 70 people in our database (we will NOT divide them into two groups as we did previously). </p><p>To make sure you are using the entire database, select Data from the main menu. Then choose Select Cases... If it is not already selected, click on the circle to the left of the All Cases option in the "Select Cases Dialogue Box." Then click on the [ OK ] button.</p><p>Computing the Base Rate It is important to know what percentage of applicants could do the job even if no selection system existed at all (the Base Rate). To compute this, we will first put all who successfully perform into one group and all who do not into another group.</p><p>Therefore, we will do the following:</p><p>From the main menu, select Transform, followed by Recode. Then select Into Different Variables... </p><p>This opens the "Recode into Different Variables Dialogue Box" where you should enter the following information:</p><p>Variable Names: Input Variable -> Output Variable Output Variable: [ id ] [ y ] Name: [ x1 ] [>] [ ] [ OKworker] [ Change ] [ x2 ] Label: [ : ] [ Who is an OK worker?] [ : ] [ If... ] [ Old and new values... ] [ OK ] [ Paste ] [ Reset ] [ Cancel ] [ Help ]</p><p>Next, click on the [ Old and New Values... ] box. 22 This opens the "Old and New Values Dialogue Box" where you need to enter the following information:</p><p>Old Value: New Value: ( ) Value: [ ] (•) Value: [ 1 ] ( ) System Missing ( ) System-missing ( ) Copy old value(s) ( ) System- or user-missing (•) Range: [ Add ] Old -> New [ 3.411 ] through [ 7.0 ] [ Change ] [ ] ( ) Range: Lowest through [ ] [ Remove ] [ ] ( ) Range: [ ] through highest. ( ) All other values ( ) Output variables are strings ( ) Convert strings to numbers [ Continue ] [ Cancel ] [ Help ]</p><p>Next click on the [ Add ] button. This will recode those whose performance scores are satisfactory (3.411 or higher) on y into a value of "1" for the new variable OKworker. However, you still have to code values for those with a y score of 3.410 or lower in a value of "2." How would you do that?</p><p>While still in the "Old and New Values Dialogue Box" click on the circle next to the Range: Lowest through [ ]. Next enter 3.410 in that box. In the New Value box enter a 2 where you had previously entered a "1." This tells SPSS that unacceptable workers are coded as 2 on the new OKworker variable. Note that this recode procedure does not allow you to give the new variable values identifying labels (after leaving these procedures, when looking at the data editor window, you can double-click on the variable name and then click on [ labels ] and add value labels if you wish). Otherwise, just remember 2= poor worker and 1= satisfactory worker.</p><p>Click on [ Add ]. Then click on [ Continue ]. This returns you to the "Recode into Different Variables Dialogue Box." Please click on [ Change ]. Click on [ OK ]</p><p>How does this tell you the base rate? Well, it doesn't (yet). But in a few minutes you will do a Crosstabs. And you should be able to tell from that output how many people (and what percentage) can do the job. In the meantime, since you are probably so curious that you can't stand to wait, you can click on the following: Analyze/Statistics Summarize Frequencies </p><p>This dialogue box is similar to ones you have been using. One highlights the variable of interest, then click on the [>] button to "move" it into the variable list upon which you will compute frequencies.</p><p>Select OKWorker as the variable for your frequencies calculation. Then click on [ OK ] to compute the frequencies. Upon receiving your output, you can see what percentage of workers (the number of satisfactory workers divided by the total sample) had a value of "1". That is the Base Rate! 23 Calculating the cutoff score for those who will be hired: It is important to know what the "cutoff" score on your predictor(s) will be when hiring the top 14 applicants. To compute this, you need to sort the cases based on the ypredict variable that you created earlier. Try the following:</p><p>From the main menu, select Data, then select Sort Cases... </p><p>This opens the "Sort Cases Dialogue Box:"</p><p>Variables: Sort by: [ OK ] [ id [ ] [ Paste ] x1 [>] [ ] [ Reset ] x2 [ ] [ Cancel] x3 y : ypredict Sort Order: [ ( ) Ascending ] [ (•) Decending ]</p><p>Move ypredict from the Variables box to the Sort by box, if you are using more than one predictor in your selection system. If you are using only one predictor, then move that X-variable name into the Sort by box. It doesn't matter if you sort by ascending or descending order. Click [ OK ]. </p><p>Look at your newly reordered data. Count down (or up) from the top score (on the variable you used to sort the data). Put the cursor (box) over that score. What is the 14th-best score? What is the 15th-best score? Write those scores on a piece of paper for future reference. If each score has a decimal point, be sure to write it out to the last digit beyond the decimal point; note that the number at the upper left of the screen may go to a further decimal than what you see in the data set. Now, pick a “cutoff” that is between these two scores. Also… MAKE SURE THAT YOU HAVE FOUND THE 14TH BEST SCORE AND NOT THE 14TH WORST SCORE! PLEASE DOUBLE CHECK YOUR WORK HERE!</p><p>From the main menu, select Transform, followed by Recode. Then select Into Different Variables... </p><p>This opens the "Recode into Different Variables Dialogue Box." Click [ Reset ] to clear any previous cell entries. Please enter the following information:</p><p>Variable Names: Numeric Input Variable -> Output Variable Output Variable: [ id ] [ ypredict (if you are using more] Name: [ x1 ] [>] [ than one predictor) or] [ Hired] [ Change ] [ x2 ] [ x ? (if you are using one predictor)] Label: [ : ] [ Who is hired?] [ If... ](Optional Case Selection Condition) [ Old and new values... ] [ OK ] [ Paste ] [ Reset ] [ Cancel ] [ Help ]</p><p>Next, click on the [ Old and New Values... ] box. 24 This opens the "Old and New Values Dialogue Box" where you need to enter the following information:</p><p>Old Value: New Value: ( ) Value: [ ] (•) Value: [ 2 ] ( ) System Missing ( ) System-missing ( ) Copy old value(s) ( ) System- or user-missing ( ) Range: [ Add ] Old -> New [ ??? ] through [ ] [ Change ] [ ] ( ) Range: Lowest through [ ] [ Remove ] [ ] (•) Range: [ ??? ] through highest. ( ) All other values ( ) Output variables are strings ( ) Convert strings to numbers [ Continue ] [ Cancel ] [ Help ]</p><p>Instead of ??? put in the cutoff that is between the 14th and 15th-highest predictor score. Next click on the [ Add ] button. This will recode those whose predictor scores are high enough to be hired on ypredict (or your single predictor) into a value of "2" for the new variable Hired. However, you still have to code values for those with a ypredict score that is lower than the 15th-highest score with a value of "1." How will you do that?</p><p>While still in the "Old and New Values Dialogue Box" click on the circle next to the Range: Lowest through [ ]. Next enter the same cutoff that is between the 14th and the 15th-highest score. In the New Value box enter a 1 where you had previously entered a "2." This tells SPSS that unacceptable applicants are coded as 1 on the new Hired variable. Note that this recode procedure does not allow you to give the new variable values identifying labels (after leaving these procedures, when looking at the data editor window, you can double-click on the variable name and then click on [ labels ] and add value labels if you wish). Otherwise, just remember 1= not hired with your selection system and 2= hired with your system.</p><p>Click on [ Add ]. Then click on [ Continue ]. This returns you to the "Recode into Different Variables Dialogue Box." Please click on the [ Change ] button. Click on [ OK ]</p><p>Save your updated data set with the new variables (to use with Part III at a later date). </p><p>Checking your work: Let's check your work with that last operation: From the main menu, select Analyze/statistics. Next select Summarize... Then select Frequencies... </p><p>This opens the "Frequencies Dialogue Box." This is similar to the dialogue boxes you have used previously. Here, you will see a variable list in the left column and a box of Variables (to use in the Frequencies operation) to the right. Highlight Hired and click on the [>] to move it into the Variables column. Then click on the [OK] button. Look at the total number of cases in the frequencies printout with a value of "2" (meaning ypredictor is equal to the 14th-highest score or higher). The frequencies column should say "14"!!! 25 Obtaining your 2 x 2 Crosstabulation table You want to know how the data are distributed across the two variables of “Hired” and “OKworker.” Obviously, you hope most of the 14 who would be "hired" with your system (Hired = 2) are also satisfactory workers (OKworker = 1) and most of those rejected by your system (Hired = 1) are also not OK workers (OKworker = 2). But you don't know, until you calculate a crosstabulation table, do you?</p><p>From the main menu, select Analyze/Statistics. Then select Summarize and then Crosstabs... You will see the following "Crosstabs Dialogue Box" where you should enter the underlined information: Variable List: Row(s): [ OK ] [ id ] [>] [OKworker] [ Paste] [ x1 ] [ Reset] [ x2 ] [ Cancel] [ : ] Col(s): [ Help] [ : ] [>] [Hired]</p><p>[ Previous ] Layer 1 of 1 [ Next ]</p><p>If you click on the [ Cells ] button, you can specify row, column, or total percentages, as you prefer.</p><p>Click on [ OK ] you should see a table similar to the following (only with your own data – this is just an example; note that you might need to type some labels): Hired 1 2 [not] [hired] Row [hired] Total: OKworker: 1 [OK worker] 22 12 34 2 [not OK] 34 2 36 Column Total: 56 14 70</p><p>Note that the 2 x 2 table here may not match exactly the type of table discussed in class or in various textbooks. For example, you may have to switch either rows or columns (either physically or mentally) before computing any decision accuracy statistics in some readings. Regardless, once you have the 2 x 2 table, you can calculate the various decision accuracy statistics. Using your calculator, compute these decision accuracy statistics for your data set. Consult your instructor's handouts and/or course readings for additional information on decision accuracy statistics.</p><p>In your paper, be sure to report the following: *Hit Rate (percentage of correct predictions). Here it is (34 + 12)/70 or 65.7%. *Success Rate: The "proportion of correct hires among those hired" is 12/14 or 85.7%. *Base Rate (the percentage of people who can do the job; in this case 34/70 = 48.6%) *Selection Ratio (Assuming 14 hires and 70 applicants in this example = 20%) *Improvement of the Success Rate over the Base Rate: (85.7% - 48.6%)/48.6% = 76.3% improvement. 26 SECTION E: UTILITY ANALYSIS</p><p>Several different utility methods exist; these are described in in-class handouts. You need to use the Decision Accuracy method of utility for this assignment. </p><p>Utility Based on Decision Accuracy (REQUIRED METHOD):</p><p>You will use the following formula to assess utility:</p><p>Estimated = (true positives * $15,000) – (false positives * $32,000) – (70 * testing costs) Utility</p><p>Where did we get these numbers? After working with a cost accountant, assume that for each person predicted to be a good employee (the predictor(s) recommended "hire") who actually was a satisfactory worker in this job (for each "True positive"), hospital profits rose $15,000/year.</p><p>Assume that each person predicted to be a good employee ("hire") but who wasn't a satisfactory worker (each "False Positive") costs the hospital $32,000/year.</p><p>Assume that there are no costs associated with those who are not hired (i.e., false negatives and true negatives).</p><p>With either only one predictor or with Multiple Regression, all 70 applicants were tested. </p><p>Assume that the following selection methods have the following development and administration costs per applicant: X1 (CSAP): $40 (includes both test cost and clerks’ wage to administer and proctor test) X2 (SO-WAB): $60 (assume this includes the administration/scoring costs) X3 (TST): $80 (includes test cost, PC equipment costs (for PC administration), and administration costs; must be administered individually)</p><p>Your testing costs will also vary, based on whether you chose one, two, or three predictors.</p><p>Using information from class and/or text or readings, compute a utility analysis for the predictor(s) you have chosen. What is the value for (or cost to) the organization for testing 70 applicants and hiring 14?</p><p>Extra Credit opportunity (5%): If a person in this job earns $35,000/year, can you compute utility using the 40% method (covered in class)? Remember to “plug” the value into the larger “Utility” equation described in the Standard Deviation method of utility in-class handout. Don’t expect this method to yield identical results to the decision accuracy method.</p><p>You may do extra analyses if you wish, but remember two things: First, be sure to call my attention to the extra credit that you do in your report. Second, the maximum extra credit you can earn is 10%. 27 SECTION F: Do Typing Test Scores Differ from PC and iPads?</p><p>For this analysis you want to see if taking the typing test (x3) via a PC results in different average test scores than taking the same test via an iPad or iPad mini with a Bluetooth keyboard. You should use all 70 cases for this analysis.</p><p>Select Analyze… Compare Means… One Way ANOVA </p><p>You will see the following dialog box: Variables: Dependent list: [ Contrasts] ID [ ] [ Post-hoc] X1 [ Options] X2 [>] [ Bootstrap] X3 Y Race Factor: Sex [>] [ ] Age App [OK] [Paste] [Reset] [Cancel] [Help]</p><p>Using the [>] button, Put X3 in the Dependent list and put App in the Factor list.</p><p>Click [Options] and select Descriptives. From the main dialog box select [OK] to run the analysis.</p><p>You will get an F statistic that tells you whether the means for the PC and mobile-app groups differ. A large F and a small Significance level (p < .05) means that the means are significantly different. For example (not identical to your data set):</p><p>“The means of the group taking the typing test via PC were higher than the means for the group taking the typing test via iPad (Ms = 62.5 vs. 51.1) and this difference was statistically significant, F (1, 68) = 14.78; p < . 05.”</p><p>Think about it: If you find significant differences between the two groups, what does that mean for your use of X3? What does that mean for your validity study? What should you do in that situation? If you do NOT find significant differences between the two groups, what does that suggest for your administration of X3 as a hiring test? 28 Names______Grading Checklist</p><p>Section of Report: Selection System Evaluation for Call Center Representatives, 2015 Checklist: Purpose/Overview ____Mentioned type of job ____Mentioned Predictors briefly Literature Review & ____Did you cite ten sources? Explanation & Logic; Rationale leads to your study ____Discuss validity ____Job characteristics related to predictors ____Discuss X1 – Customer Service test ____Discuss X2 – Service Orientation WAB ____Discuss X3 – Typing test ____Discuss Computerized/Mobile Testing Method ____Mention correct type of validity ____Tell how variables are scaled ____Discuss Multiple Regression ____Discuss cross-validation ____Discuss decision accuracy ____Utility Method reported Results Decision Accuracy: ____ Pearson r results correct ___Hit Rate ___Base Rate ____ Multiple R results correct ___Success Rate ___Selection Ratio ____ Regression equation? ___% improvement over random selection ____ Cross-Validation Correct ____ Utility results provided ____ PC vs. Mobile Apps compared (X3) Discussion and Recommendations ____Discusses results ____Recommends selection system ____Other related recommendations ____Discusses limits of the study ____Directions for future research Reference Page ____At least ten references, all cited ____All references are complete Figures/Results/Printouts In an Appendix—are the answers correct? ____ Pearson r results correct ____ Multiple R results correct ____ Decision Accuracy correct HR Policies/Procedures in an Appendix: Are These Relevant and Clear? ____ Policy</p><p>EXTRA CREDIT: Additional analyses, ___ Added Multiple Rs (5% max) Details, graphs, etc. The amount of ___ Decision Acc’y for Random Selection Extra credit depends upon the amount ___ Extra Utility (up to 5%) of and quality of work (10% max) ____Utility for Random Selection (5%) ____Other (10% max)</p>
Details
-
File Typepdf
-
Upload Time-
-
Content LanguagesEnglish
-
Upload UserAnonymous/Not logged-in
-
File Pages28 Page
-
File Size-