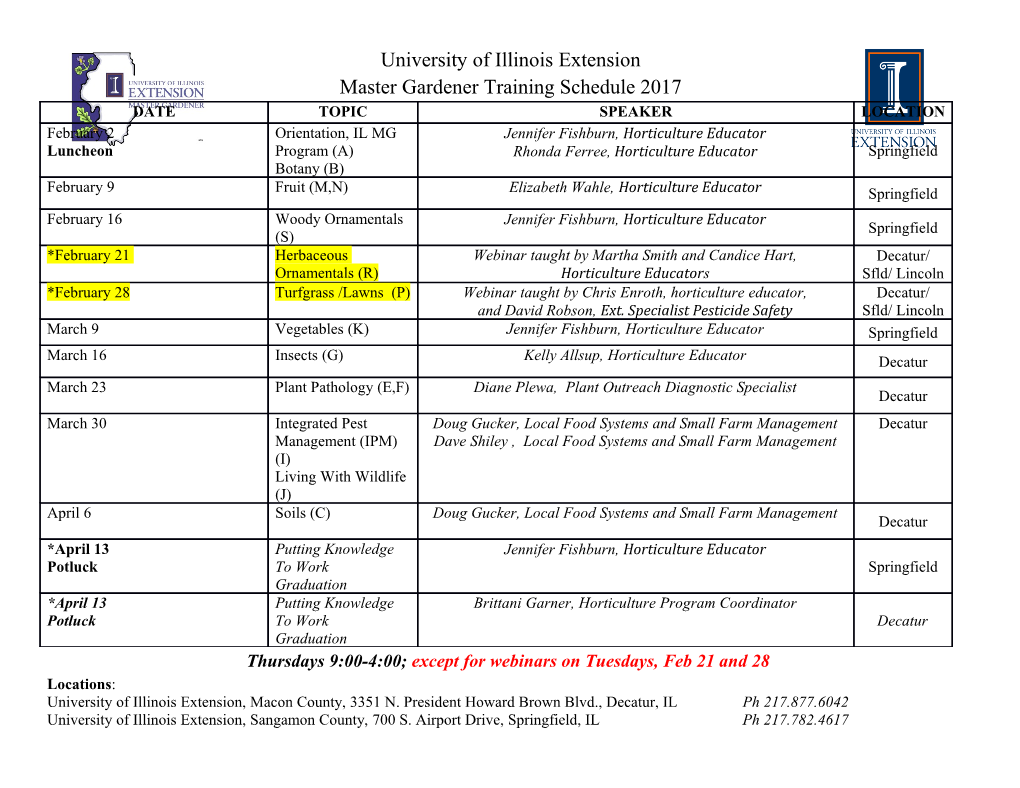
<p> PROJECT PROPOSAL</p><p>Topic: Advanced Image Coding</p><p>By Radhika Veerla</p><p>Under the guidance of Dr. K. R. Rao TABLE OF ACRONYMS</p><p>AIC advanced image coding AVC advanced video coding BMP bit map format CABAC context adaptive binary arithmetic coding DCT discrete cosine transform DWT discrete wavelet transform EBCOT embedded block coding with optimized truncation EZW embedded zero-tree wavelet coding FRExt fidelity range extensions HD-photo high-definition photo I-frame intra frame JM joint model JPEG joint photographic experts group JPEG-LS joint photographic experts group lossless coding JPEG-XR joint photographic experts group extended range LBT lapped bi-orthogonal transform MSE mean square error PGM portable graymap PNM portable any map PPM portable pixel map PSNR peak signal to noise ratio SSIM structural similarity index VLC variable length coding LIST OF FIGURES</p><p>Figure 1 The process flow of the AIC encoder and decoder 2 YCbCr sampling formats - 4:4:4, 4:2:2 and 4:2:0 3 Different prediction modes used for prediction in AIC 4 The specific coding parts of the profiles in H.264 5 Basic coding structure for a macroblock in H.264/ AVC 6 Block diagram for CABAC 7 Diagram for zig-zag scan and scan line order 8 Block diagram of JPEG encoder and decoder 9 Structure of JPEG 2000 codec 10 Tiling, DC level shifting, color transformation, DWT of each image component 11 Block diagram of JPEG-XR encoder and decoder 12 JPEG-LS block diagram 13 A causal template of LOCO-I 14 SSIM measurement system Implementation of AIC based on I-frame only coding in H.264 and comparison with other still frame image coding standards such as JPEG, JPEG 2000, JPEG-LS, JPEG-XR</p><p>Objective: It is proposed to implement advanced image coding (AIC) based on I-frame only coding using JM software and compare the results with other image compression techniques like JPEG, JPEG2000, JPEG-LS, JPEG-XR, Microsoft HD photo, H.263 I- frame coding. Coding simulations will be performed on various sets of test images. Experimental results are to be measured in terms of bit-rate, quality- PSNR, SSIM etc. This project considers only main and (FRExt) high profiles in H.264/AVC I-frame coding, JPEG using baseline method, JPEG 2000 in non-scalable, but optimal mode.</p><p>Introduction: The aim of AIC [1] is to provide better quality with reduced level of complexity while optimizing readability and clarity. Though its aim is not to optimize speed, it is faster than many of the JPEG 2000 codecs [10]. H.264 technology aims to provide good video quality at considerably low bit rates, at reasonable level of complexity while providing flexibility to wide range of applications [2]. Coding efficiency is further improved in fidelity range extensions (FRExt) using 8x8 integer transform and works well for more complex visual content. JPEG [15] is first still image compression standard which uses 8x8 block based DCT decomposition, while JPEG 2000 is a wavelet-based compression standard which has improved coding performance over JPEG with additional features like scalability and lossless coding capability has best performance with smooth spatial data. JPEG performs well in low complexity applications whereas JPEG 2000 works well in high complexity, lower bit-rate applications. JPEG2000 has rate-distortion advantage over JPEG. Microsoft HD photo [19] is a new still-image compression algorithm for continuous-tone photographic images which maintains highest image quality or delivers the most optimal performance. JPEG-XR [16] (extended range), a standard for HD-photo has high dynamic-range image coding and performance as the most desirable feature. Its performance is close to JPEG2000 with computational and memory requirements close to JPEG. With half the file size of JPEG, HD photo delivers lossy compressed image with better perceptual quality than JPEG and lossless compressed image at 2.5 times smaller than the original image. JPEG-LS [30] (lossless) is an ISO/ITU-T standard for lossless coding of still images. In addition, it also provides support for "near-lossless" compression. The main goal of JPEG-LS is to deliver a low complexity solution for lossless image coding with the best possible compression efficiency. JPEG uses Huffman coding, H.264/AVC and AIC systems adopt CABAC encoding technique, and HD photo uses reversible integer-integer-mapping lapped bi-orthogonal transform [7]. LOCO-I (low complexity lossless compression for images), an algorithm for JPEG-LS uses adaptive prediction, context modeling and Golomb coding. It supports near lossless compression by allowing a fixed maximum sample error. Transcoding converts H.263 compression format to that of H.264 and viceversa. If the transcoding is done in compression domain, it gives better results as the computation only needs to perform on compressed pixels. Although the above mentioned compression techniques are developed for different signals, they work well for still image compression and hence worthwhile for comparison. Different softwares like AIC reference software, JM software for H.264 [17], JPEG reference software [18] for JPEG, HD-photo reference software [19], JasPer [20] for JPEG2000, JPEG-LS reference software [30] are used for comparison between different codecs. The evaluation will be done using bit rates, different quality assessment metrics like PSNR, SSIM and complexity. The following topics are discussed in this proposal. AIC is described in detail as it is implemented and various other codecs used for comparison in brief. Different settings used in the softwares and evaluation methodology are discussed. Few results obtained by evaluating different test images and test images of different sizes using AIC reference software are included. </p><p>Advanced Image Coding Advanced image coding (AIC) is a still image compression system which combines the algorithms of H.264 and JPEG standard, shown in Fig.1, in order to achieve best compression capability in terms of quality factor with less complexity. The performance of AIC is close to JPEG 2000 and is lot better than JPEG. AIC uses the intra-frame block prediction, which is originally used in H.264 to reduce the large number of bits to code original input. Both AIC and H.264 use CABAC coding while AIC uses position of coefficient matrix as the context [1]. It is observed that each block in AIC is modified to get the best compression efficiency possible.</p><p>Fig.1: The process flow of the AIC encoder and decoder [1]. Overview: The color conversion from RGB to YCbCr allows better compression in channels as chrominance channels have less information content. Then each channel is divided into 8x8 blocks for prediction. Prediction is based on 9 modes based on previously encoded and decoded blocks. Chrominance channels use same prediction modes as corresponding blocks in luminance. Entropy is reduced further when DCT is applied to the residual blocks. CABAC is used for encoding the bit stream which uses a context where commonly encoded prediction modes and DCT coefficients use less number of bits than rarely used prediction modes and coefficients [1]. It is observed that each block in AIC is modified to get the best compression efficiency possible.</p><p>Color conversion: The color conversion from RGB to YCbCr allows better compression in channels as chrominance channels have less information content. AIC achieves higher quality/ compression ratio without the use of sub-sampling, which was employed by H.264 and JPEG. This is possible with the use of block prediction and binary arithmetic coding. AIC uses 4:4:4 format shown in Fig.2. Sub-sampling has negative impact on image quality. </p><p>4:4:4 4:2:2 4:2:0</p><p>Cb,Cr samples Y sample</p><p>Fig.2: YCbCr sampling formats - 4:4:4, 4:2:2 and 4:2:0 [33]</p><p>Block prediction: Each channel is divided into 8x8 blocks for prediction. Each 8x8 block is encoded using scan line order from left to right and top to bottom. H.264 supports 8x8 and 16x16 block prediction algorithms whereas AIC uses 4x4 block algorithms which are extended to 8x8 block case. Prediction is performed using all previously encoded and decoded blocks. Both H.264 and AIC use 9 prediction modes to predict the current block, shown in Fig.3. The mode which gives the minimum difference between the original and predicted block is chosen. Prediction needs information about all the pixels. The first block cannot be predicted by previous blocks. So DC mode is used for this purpose. Same prediction modes employed by Y are used for Cb, Cr in order to reduce complexity. Residual blocks are obtained by subtracting the predicted block from the original block. </p><p>AIC – Block Prediction Implementation Details: Different modes used for block prediction are shown in Fig.3. </p><p>Mode 0: Vertical Mode 1: Horizontal Mode 2: DC</p><p>Mode 3: Diagonal Down-Left Mode 4: Diagonal Down-Right</p><p>Mode 5: Vertical-Right Mode 6: Horizontal-Down Mode 7: Vertical-Left Mode 8: Horizontal-Up</p><p>Fig. 3: Different prediction modes used for prediction in AIC [1]</p><p>DCT and Quantization: DCT is applied on each 8x8 residual block. DCT has a property of energy compaction. Uniform quantization is applied without actually discarding the bits. Quality level setting is nothing but setting the amount of quantization. AIC uses floating point algorithms to produce the best quality images. In JPEG shown in Fig.8, the DCT coefficients are transmitted in zig-zag order shown in Fig.7(a) rather than scan-line order shown in Fig.7(b) employed by AIC. Zig-zag scanning needs reordering of coefficients to form run of zeros which can be encoded using run length coding. CABAC does not need reordering of coefficients, so run length encoding is not needed.</p><p>CABAC: The resulting prediction modes and DCT coefficients obtained from the above processes must be stored in a stream. AIC uses CABAC algorithms to minimize the bit stream. CABAC uses different contexts to encode symbols. Arithmetic coding can encode fractional number of bits and outperforms Huffman coding but is more complex and slower. Position of coefficient in a matrix may be context. This can be derived as DCT has high probability of zero coefficients in high-frequency domain. Different contexts AIC use are prediction-prediction mode, prediction mode, coefficient map, last coefficient, coefficient greater than 1, absolute coefficient value, coded block [1].</p><p>H.264 standard H.264 or MPEG-4 part 10 aims at coding video sequences at approximately half the bit rate compared to MPEG-2 at the same quality. It also aims at having significant improvements in coding efficiency using CABAC entropy coder, error robustness and network friendliness. Parameter set concept, arbitrary slice ordering, flexible macroblock structure, redundant pictures, switched predictive and switched intra pictures have contributed to error resilience / robustness of this standard. Adaptive (directional) intra prediction (Fig. 3) is one of the factors which contributed to the high coding efficiency of this standard [2]. High Profiles Adaptive transform block size Extended Profile Quantization scaling matrices Main Profile CABAC Data partition B slice SI slice Weighted prediction</p><p>SP slice I slice P slice CAVLC</p><p>Arbitrary slice order Flexible macroblock order Redundant slice</p><p>Baseline Profile</p><p>Fig. 4: The specific coding parts of the profiles in H.264 [2]</p><p>Each profile specifies a subset of entire bitstream of syntax and limits that shall be supported by all decoders conforming to that profile. There are three profiles in the first version: baseline, main, and extended. Main profile is designed for digital storage media and television broadcasting. H.264 main profile which is the subset of high profile was designed with compression coding efficiency as its main target. Fidelity range extensions [3] provide a major breakthrough with regard to compression efficiency. The profiles are shown in Fig. 4.</p><p>There are four High profiles defined in the fidelity range extensions: High, High 10, High 4:2:2, and High 4:4:4. High profile is to support the 8-bit video with 4:2:0 sampling for applications using high resolution. High 10 profile is to support the 4:2:0 sampling with up to 10 bits of representation accuracy per sample. High 4:2:2 profile supports up to 10 bits per sample. High 4:4:4 profile supports up to 4:4:4 chroma sampling up to 12 bits per sample thereby supporting efficient lossless region coding [2]. </p><p>H.264/AVC Main Profile Intra-Frame Coding: Main difference between H.264/AVC main profile intra-frame coding and JPEG 2000 is in the transformation stage. The characteristics of this stage also decide the quantization and entropy coding stages. H.264 uses block based coding, shown in Fig. 5 which is like block translational model employed in inter-frame coding framework [7]. 4x4 transform block size is used instead of 8x8. H.264 exploits spatial redundancies using intra-frame prediction of the macro-block using the neighboring pixels of the same frame, thus taking the advantage of inter-block spatial prediction. The result of applying spatial prediction and wavelet like 2-level transform iteration is effective in smooth image regions. This feature enables H.264 to be competitive with JPEG2000 in high resolution, high quality applications. JPEG cannot sustain in the competition even though it uses DCT based block coding. DCT coding framework is competitive with wavelet transform coding if the correlation between neighboring pixels is properly considered using context adaptive entropy coding. In H.264, after transformation, the coefficients are scalar quantized, zig-zag scanned and entropy coded by CABAC. Another entropy coding CAVLC operates by switching between different VLC tables which are designed using exponential Golomb codes [32] based on locally available contexts collected from neighboring blocks- used sacrificing some coding efficiency [2]. </p><p>H.264/AVC FRExt High Profile Intra-Frame Coding: Main feature in FRExt that improves coding efficiency is the 8x8 integer transform- and all the coding methods as well as prediction modes associated with adaptive selection between 4x4 and 8x8 integer transforms. Other features include [3, 7] higher resolution for color representation such as YUV 4:2:2 and YUV 4:4:4, shown in Fig.2. addition of 8x8 block size is a key factor in very high resolution, high bit rates achieve very high fidelity – even for selective lossless representation of video</p><p>Fig.5: Basic coding structure for a macroblock in H.264/AVC [2].</p><p>Context-based Adaptive Binary Arithmetic Coding (CABAC): CABAC utilizes the arithmetic coding, also in order to achieve good compression. The CABAC encoding process, shown in Fig. 6, consists of three elementary steps [11]. Fig.6: Block diagram for CABAC [8] step 1 : binarization – Mapping non binary symbols into binary sequence before given to arithmetic coder. step 2 : context modeling – It is a probability model for defining one or more elements based on previously encoded syntax elements. step 3 : binary arithmetic coding – Encodes elements based on selected probability model.</p><p>JPEG JPEG is the first ISO/ITU-T standard for continuous tone still images [15]. It allows lossy and lossless coding of still images. JPEG gives good compression results for lossy compression with the least complexity. There are several modes defined for JPEG including baseline, progressive and hierarchical. The baseline mode, which supports lossy compression alone, is most popular. Average compression ratio of 15:1 is achieved using lossy coding with the help of DCT-block based compression. Lossless coding is made possible with predictive coding compression techniques which include differential coding, run length coding and Huffman coding. JPEG employs uniform quantization with HVS weighting. Zig-zag scanning is performed on quantized coefficients since it allows entropy coding to be performed in the order from low frequency to high frequency components [15]. Fig.7(a): Zig-zag scan [15] Fig.7(b): Scan line order [1]</p><p>The process flow of JPEG baseline (lossy) algorithm is shown in the Fig.8.</p><p>(a) (b) Fig.8(a): Block diagram of JPEG encoder (b): Block diagram of JPEG decoder [15] </p><p>The process flow starts with the color conversion for color images followed by 8x8 block based DCT (process flow starts here for gray scale images), quantization, zig-zag ordering, and entropy coding using Huffman tables in the encoding process and vice versa for decoding process. Different quantization matrices are used for luminance and chrominance components. Quality factor ‘Q’ is set using quantization tables and different kinds of artifacts in varied ranges are observed [15].</p><p>JPEG2000 JPEG 2000 [10] is image compression standard which supports lossy and lossless compression of gray scale or color images. In addition to the compression capability, JPEG 2000 supports excellent low bit rate performance without sacrificing the performance at high bit rate, region of interest coding, EBCOT (Embedded Block Coding with Optimal Truncation) which overcomes the limitations of EZW (embedded zero-tree wavelet coding) which are random access to specific regions of the image, error resilience. It also supports flexible file format and progressive decoding of the image to allow from lossless to lossy by fidelity and resolution. It is a transform based framework, uses wavelet based decomposition. Wavelet transform has 3dB improvement over DCT based compression [14]. Lossless compression is the result of transform, entropy coding. We consider non-scalable, single layer mode since scalability feature leads to adverse effect on rate-distortion performance. Also we disable tiling mode because it also lowers rate-distortion performance. Tiling allows the image be partitioned into non-overlapped rectangular tiles to be encoded independently [7].</p><p>Fig.9: Structure of JPEG 2000 codec. The structure of the (a) encoder and (b) decoder [22] Fig.10: Tiling, DC level shifting, color transformation, DWT of each image component [9]</p><p>JPEG XR JPEG XR [16], a coded file format is designed mainly for storage of continuous- tone photographic content. It supports wide range of color formats including n-channel encodings using fixed and floating point numerical representations, bit depth varieties giving a way for wide range of data compression scenarios. The ultimate goal is to support wide range of color encodings, maintain forward compatibility with existing formats and keep device implementation simple. It also aims at providing same algorithm for lossless as well as lossy compression. HD photo format [19] is a new file format standardized using JPEG-XR. Just like JPEG-2000, Microsoft HD photo works on advanced features like lossy-lossless compression, bit-rate scalability, editing, region-of-interest decoding, integer implementation without division etc. on top of compression capability. HD photo minimizes objectionable spatial artifacts preserving high frequency detail and outperforms other lossy compression technologies in this regard.</p><p>Adaptive VLC 8x8 blocks Quantization tables table switching</p><p>Reversible int-int Scalar VLC mapping LBT quantization Encoding Original Coded image image Block based encoder (a) HD photo encoder Adaptive VLC table switching Quantization tables</p><p>VLC Scalar Reversible int-int Decoding Dequantization mapping inverse Original Coded LBT image image (b) HD photo decoder Fig.11(a): Block diagram of JPEG-XR encoder (b): Block diagram of JPEG decoder </p><p>HD photo is a block-based image coder similar to traditional image-coding paradigm: color conversion, transform, coefficient scanning, scalar quantization and entropy coding. Main blocks of HD photo include transformation stage and the coefficient-encoding stage. HD photo employs a reversible integer-to-integer-mapping lapped bi-orthogonal transform (LBT) as its decorrelation engine. The reversible property of the algorithm supports both lossy and lossless compression. Thus, it simplifies the overall implementation of the system. HD photo’s encoder contains many adaptive elements: adaptive coefficient scanning, flexible quantization, inter-block coefficient prediction, adaptive VLC table switching, etc as shown in Fig. 11. JPEG XR supports a number of advanced pixel formats in order to avoid limitations and complexities of conversions between different unsigned integer representations. This feature allows flexible approach to numerical encoding of image data. This results in low- complexity implementations in the encoder and decoder [16].</p><p>JPEG-LS Hewlett Packard proposed a simpler predictive coder for low complexity [30]. LOCO-I (LOw COmplexity LOssless COmpression for Images) is a lossless compression algorithm for continuous-tone images which combines the simplicity of Huffman coding with the compression potential of context models. Lossless image compression schemes often consist of two distinct and independent components: modeling and coding. The modeling part can be formulated as an inductive inference problem, in which an image is observed pixel by pixel in some pre-defined order (e.g. raster-scan). Fig.12: JPEG-LS block diagram [30] Description of LOCO-I The prediction and modeling units in LOCO-I are based on the causal template depicted in Fig 12.</p><p>Fig.13: A causal template of LOCO-I [29] a) Prediction The prediction approach is a variation of median adaptive prediction in which the predicted value is the median of a, b, c pixels. The initial prediction is obtained using the following algorithm. if c>=max(a,b) x=max(a,b) else { if c<=min(a,b) x=min(a,b) else x=a+b-c } The initial prediction is then refined using the average value of the prediction error in that particular context [30]. b) Context modeling The key objective in a context modeling scheme is reducing the number of parameters. i) Coding distributions The distribution of prediction residuals in continuous tone images can often be approximated by a Laplacian distribution, i.e. a two-sided exponential decay centered at zero. For each context, the encoder adaptively chooses the best among a limited set of Huffman codes, matched to exponentially decaying distributions, based on past performance. As these distributions are assumed to be centered at 0, a single parameter (e.g., the average of error magnitudes at the corresponding context) is sufficient to characterize each one of them [29]. ii) Context determination The contexts in JPEG-LS also reflect the local variations in pixel values. The context that conditions the encoding of the current prediction residual in LOCO-I is built out of the differences g1=d−a; g2=a−c; g3=c−b, and g4=b−e. The context is built out of the prediction errors incurred in previous encodings. Since further parameter reduction is obviously needed, each difference gj, j = 1, 2, 3, 4 is quantized into a small number or approximately equiprobable regions (the same regions for j = 1, 2, 3, 4) [29]. c) Coding LOCO-I combines the simplicity of Huffman (as opposed to arithmetic) coding with the compression potential of context models [30]. The prediction errors are encoded using adaptively selected codes based on Golomb codes, which is also optimal for sequences with a geometric distribution [30]. i) Sequential parameter estimation A sequential scheme is mandatory in a context-based method, as pixels encoded in a given context are not necessarily contiguous in the image and, thus, cannot be easily blocked. ii) Bias cancellation Golomb-Rice codes [32] rely heavily on the distribution of prediction residuals being a two-sided, symmetric, exponential decay centered at zero. While these assumptions are usually satisfied in the case of memoryless models, the situation is quite different in the case of context-based models, where systematic, context-dependent biases in the prediction residuals are not uncommon. These systematic biases can produce a very significant deterioration in the compression performance of a Golomb-Rice coder. To alleviate the effect of systematic biases, LOCO-I uses an error feedback aimed at centering the distributions of prediction residuals [29].</p><p>(d) Embedded alphabet extension LOCO-I addresses the redundancy of Huffman or Golomb/Rice codes due to very skewed distributions, by embedding an alphabet extension into the context conditioning. Because of the ability to function in multiple modes, it performs very well on compound documents, which may contain images along with text [30]. LOCO-I is within a few percentage points of the best available compression ratios (given by CALIC), at a complexity level close to an order of magnitude lower [29]. JPEG-LS performs very well on compound documents because of the ability to function in multiple modes. It works well for cost sensitive and embedded applications that do not require any JPEG-2000 functionalities such as progressive bit-streams, error resilience, region of interest coding (ROI) etc. </p><p>Main Differences [1, 3, 7, 29, 30]: The main difference between the AIC, JPEG, JPEG2000, JPEG-LS and JPEG-XR codecs is at the transformation stage. JPEG2000 decorrelates image data via the global discrete wavelet transform (DWT) or the more general decomposition of wavelet packet while H.264 and HD Photo choose the block-based coding framework with the same 16x16 macro-block size and a core 4x4 block transform that is very similar to the discrete cosine transform (DCT). JPEG and AIC use discrete cosine transform (DCT) to de- correlate the image. The major difference between H.264’s and HD Photo’s transformation stage is the way the two coders handle inter-block decorrelation. While H.264 relies heavily on adaptive spatial prediction of the current block from its neighbors, HD Photo employs an overlap operator which performs preprocessing of pixels along the block boundaries before feeding them into the core DCT-like 4x4 block transform. The main difference between JPEG and AIC transformation stage is the block based coefficients to which decorrelation is applied. The decorrelation is applied to all the coefficients in the original image in JPEG whereas in the AIC, it is applied to the residual block coefficients. Equivalently, the combination of the overlap operator and the core block transform generates a lapped transform. Similar to JPEG2000, the entire transform step of HD Photo is constructed with dyadic-rational lifting steps such that it maps integers to integers with perfect reversibility, allowing a unifying lossless to lossy coding framework. On the contrary, H.264 and AIC achieve lossless compression from residue coding. Another obvious difference is at the entropy coding stage where each coder tunes its context-based adaptive model to take advantage of the specific behavior of its transform coefficients and/or parameters. H.264/AVC employs intra prediction in spatial domain. AIC follows the same technique. This avoids propagating the error due to the motion compensation in inter-coded macro-blocks. On the other hand, all the previous video coding standards like H.263 and MPEG-4 visual use intra prediction in transform domain [10]. LOCO-I significantly outperforms other one-pass schemes (of comparable complexity (e.g. JPEG-Huffman), and it attains compression ratios similar or superior to those of higher complexity schemes based on arithmetic coding (e.g. JPEG-Arithmetic) [30]. The complexity of JPEG2000 is relatively high, compared with JPEG and JPEG- LS. Evaluation Methodology:</p><p>Image Test Sequences: In the evaluation using AIC, different images of same size and different sizes are considered to completely evaluate its performance. Test images that evaluate different textures and patterns of the image will be considered so that image can be analyzed in full detail and also have compatibility with different softwares in terms of file formats etc.</p><p>Codec Settings: In the coding experiments, publicly available software implementations are used for AIC, H.264/AVC, JPEG2000, HD photo and JPEG-LS. Reference software (JM 12.2) (latest is JM 14.0) [15] is used for H.264/AVC encoder, and each frame of the test sequences is coded in the I–frame mode. For JPEG, JPEG baseline reference software [17] is used. In JPEG, it is used to code each frame to reach the target quality factor to indirectly control bit rate for lossy coding. For JPEG 2000 coding, M.D. Adams “JasPer” (version 1.900.1) software [19] is used. This software is written in C programming language. This software can handle image data in many formats like PGM/PPM, windows BMP, but it does not accept all the BMP files. In JPEG 2000, it is used to code each frame to reach target rate specification in terms of compression factors, which is well defined for multi-component images.</p><p>The configuration of the H.264/AVC JM12.2 encoder [15] is chosen as follows: 8x8 transform mode: enabled, allowing adaptive choice between 4x4/8x8 transform and all associated prediction modes ProfileIDC = 77 # Profile IDC (77=main, FREXT Profiles: 100=High) IntraProfile = 1 # Activate Intra Profile for FRExt (0: false, 1: true) CABAC: enabled CABAC context initialization ContextInitMethod = 1 # Context init (0: fixed, 1: adaptive) R-D optimization: 1 # 1: RD-on (High complexity mode) Deblocking filter: off Q_Matrix: disabled , ScalingMatrixPresentFlag: 0 </p><p>For Microsoft HD Photo [19], all options are set to their default values with the only control coming from the quality factor setting: No tiling One-level of overlap in the transformation stage No color space sub-sampling Spatial bit-stream order All sub-bands are included without any skipping.</p><p>Subjective vs. Objective Image Quality Measures Lossless and lossy compression use different methods to evaluate compression quality. Standard criteria like compression ratio, execution time, etc are used to evaluate the compression in lossless case, which is a simple task whereas in lossy compression, it is complex in the sense that it should evaluate both the type and amount of degradation induced in the reconstructed image [24] . The goal of image quality assessment is to accurately measure the difference between the original and reconstructed images. The result thus obtained is used to design optimal image codecs. The objective quality measure like PSNR, measures the difference between the individual image pixels of original and reconstructed images. The SSIM [27] is designed to improve on traditional metrics like PSNR and MSE (which have proved to be inconsistent with human visual perception) and is highly adapted for extracting structural information. The SSIM index is a full reference metric, in other words, the measure event of image quality is based on an initial uncompressed or distortion free image as reference. The SSIM measurement system is shown in Fig. 14. Fig.14: Structural similarity (SSIM) measurement system [25]</p><p>Typical artifacts are: Blocking effect – is due to block-based DCT coding schemes. So it can be observed in AIC, JPEG. HD-photo has reduced block boundary artifacts. Blurring effect – results from wavelet based encoders. JPEG 2000 suffers from this kind of artifact. Ringing- result of quantization. Occurs in both luminance and chrominance components Almost all codecs employ quantization. So it can be an important factor. Color bleeding- due to chroma sub-sampling. In AIC, color bleeding can be neglected as it does not employ sub-sampling. All other codecs have this artifact.</p><p>Standard distortion metrics can be used for transcoding, rate-distortion control and quality requirement for new standards [23].</p><p>Conclusions: The project aims to implement AIC encoder and decoder shown in Fig.1. and check the results with the AIC reference software. These results will be compared with those of other compression techniques in terms of bit rates, objective and structural quality measures (quality- PSNR, SSIM respectively) using different softwares like JM software for H.264, JasPer for JPEG2000, HD-photo reference software and JPEG-LS reference software. Different test images are used to evaluate varied textures and patterns of the image so that image compression technique can be studied in full detail. The project can also be extended to compare the lossless compression. References: [1] AIC website: http://www.bilsen.com/aic/ [2] T. Wiegand, G. Sullivan, G. Bjontegaard and A. Luthra, “Overview of the H.264/AVC Video Coding Standard”, IEEE Transactions on Circuits and Systems for Video Technology, vol. 13, pp.560-576, July 2003. [3] G. Sullivan, P. Topiwala and A. Luthra, “The H.264/AVC Advanced Video Coding Standard: Overview and Introduction to the Fidelity Range Extensions,” SPIE Conference on Applications of Digital Image Processing XXVII, vol. 5558, pp. 53-74, Aug. 2004. [4] I. Richardson, H.264 and MPEG-4 Video Compression: Video Coding for Next- Generation Multimedia, John Wiley & Sons, 2003. [5] P. Topiwala, “Comparative study of JPEG2000 and H.264/AVC FRExt I-frame coding on high definition video sequences,” Proc. SPIE Int’l Symposium, Digital Image Processing, San Diego, Aug. 2005. [6] P. Topiwala, T. Tran and W.Dai, “Performance comparison of JPEG2000 and H.264/AVC high profile intra-frame coding on HD video sequences,” Proc. SPIE Int’l Symposium, Digital Image Processing, San Diego, Aug. 2006. [7] T. Tran, L.Liu and P. Topiwala, “Performance comparison of leading image codecs: H.264/AVC intra, JPEG 2000, and Microsoft HD photo,” Proc. SPIE Int’l Symposium, Digital Image Processing, San Diego, Sept. 2007. [8] D. Marpe, T.Weigand and G. Sullivan, “The H.264/MPEG4 advanced video coding standards and its applications”, IEEE Communications Magazine, vol. 44, pp.134-143, Aug. 2006. [9] A. Skodras, C. Christopoulus and T. Ebrahimi, “The JPEG2000 still image compression standard,” IEEE Signal Processing Magazine, vol. 18, pp. 36-58, Sept. 2001. [10] D.S. Taubman and M.W. Marcellin, JPEG 2000: Image compression fundamentals, standards and practice, Kluwer academic publishers, 2001. [11] W.B. Pennebaker and J.L. Mitchell, JPEG: Still image data compression standard, Kluwer academic publishers, 2003. [12] D. Marpe, V. George, and T.Weigand, “Performance comparison of intra-only H.264/AVC HP and JPEG 2000 for a set of monochrome ISO/IEC test images”, JVT-M014, pp.18-22, Oct. 2004. [13] D. Marpe et.al, “Performance evaluation of motion JPEG2000 in comparison with H.264 / operated in intra-coding mode”, Proc. SPIE, vol. 5266, pp. 129-137, Feb. 2004. [14] Z. Xiong et.al, “A comparative study of DCT- and wavelet-based image coding,” IEEE Trans. on Circuits and Systems for Video Tech., vol.9, pp. 692-695, Aug. 1999. [15] G. K. Wallace, “The JPEG still picture compression standard,” Communication of the ACM, vol. 34, pp. 31-44, April 1991. [16] G. J. Sullivan, “ ISO/IEC 29199-2 (JpegDI part 2 JPEG XR image coding – Specification),” ISO/IEC JTC 1/SC 29/WG1 N 4492, Dec 2007 [17] H.264/AVC reference software (JM 12.2) Website: http://iphome.hhi.de/suehring/tml/download/ [18] JPEG reference software Website: ftp://ftp.simtel.net/pub/simtelnet/msdos/graphics/jpegsr6.zip [19] Microsoft HD photo specification: http://www.microsoft.com/whdc/xps/wmphotoeula.mspx [20] JPEG2000 latest reference software (Jasper Version 1.900.0) Website: http://www.ece.ubc.ca/mdadams/jasper [21] M.D. Adams, “JasPer software reference manual (Version 1.900.0),” ISO/IEC JTC 1/SC 29/WG 1 N 2415, Dec. 2007. [22] M.D. Adams and F. Kossentini, “Jasper: A software-based JPEG-2000 codec implementation,” in Proc. of IEEE Int. Conf. Image Processing, vol.2, pp 53-56, Vancouver, BC, Canada, Oct. 2000. [23] J. J. Hwang and S. G. Cho, “Proposal for objective distortion metrics for AIC standardization”, ISO/IEC JTC 1/SC 29/WG 1 N4548, Mar 2008. [24] A. Stoica, C. Vertan, and C. Fernandez-Maloigne, “Objective and subjective color image quality evaluation for JPEG 2000- compressed images,” Int’l Symposium on Signals, Circuits and Systems, vol. 1, pp. 137 – 140, July 2003. [25] X. Shang, “Structural similarity based image quality assessment: pooling strategies and applications to image compression and digit recognition,” M.S. Thesis, EE Department, The University of Texas at Arlington, Aug. 2006. [26] A. M. Eskicioglu and P. S. Fisher, “Image quality measures and their performance,” IEEE Signal Processing Letters, vol. 43, pp. 2959-2965, Dec. 1995. [27] Z. Wang and A. C. Bovik, “Image quality assessment: from error visibility to structural similarity,” IEEE Trans. Image Processing, vol. 13, pp. 600 – 612, Apr. 2004. [28] H. R. Wu and K. R. Rao, “Digital video image quality and perceptual coding,” Boca Raton, FL: Taylor and Francis, 2006. [29] M. J. Weinberger, G. Seroussi, and G. Sapiro, “LOCO-I: A low complexity, context-based, lossless image compression algorithm”, Hewlett-Packard Laboratories, Palo Alto, CA. [30] M.J. Weinberger, G. Seroussi and G. Sapiro, “The LOCO-I lossless image compression algorithm: principles and standardization into JPEG-LS”, IEEE Trans. Image Processing, vol. 9, pp. 1309-1324, Aug.2000. http://www.hpl.hp.com/loco/ [31] Ibid, “LOCO-I A low Complexity Context-based, lossless image compression algorithm”, Proc. 1996 DCC, pp.140-149, Snowbird, Utah, Mar. 1996. [32] K. Sayood, “Introduction to Data Compression”, Third Edition, Morgan Kaufmann Publishers, 2006. [33] M.Ghanbari, “Standard Codecs: Image Compression to Advanced Video Coding”. IEE, London, UK, 2003. [34] Z. Wang and A. C. Bovik, “Modern Image quality assessment”, Morgan and Claypool Publishers, 2006.</p>
Details
-
File Typepdf
-
Upload Time-
-
Content LanguagesEnglish
-
Upload UserAnonymous/Not logged-in
-
File Pages23 Page
-
File Size-