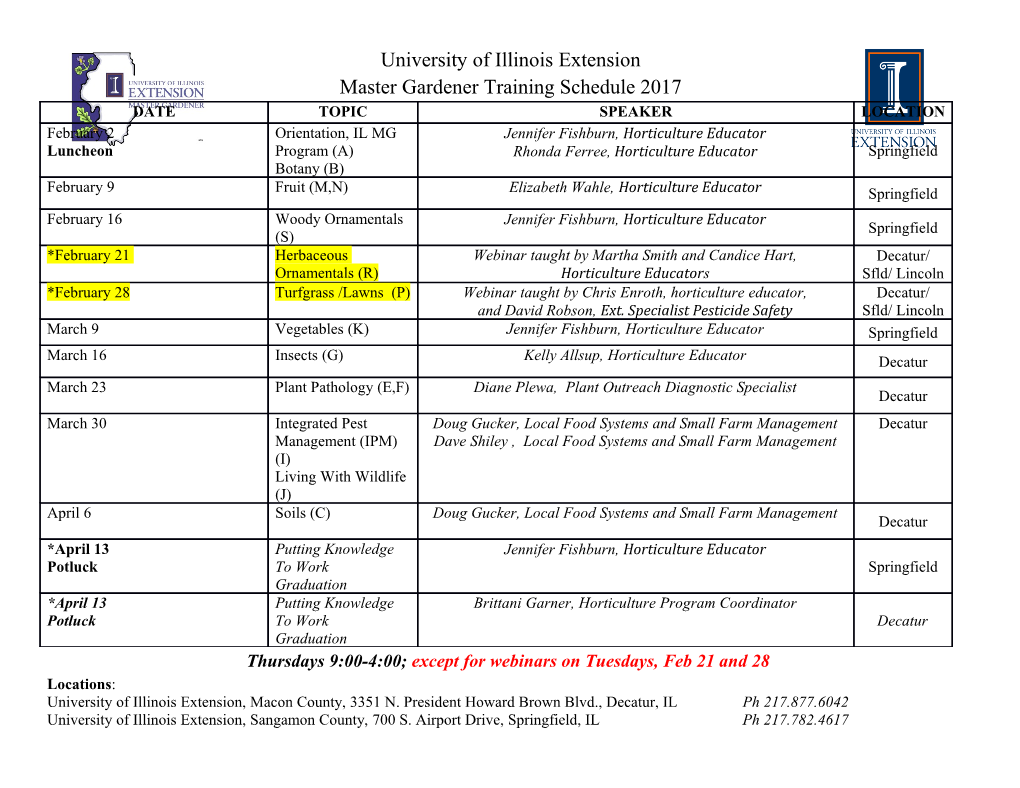
<p> SUPPLEMENTAL APPENDIX A Question Wording from CCES Surveys</p><p>Age Respondents in each survey were asked to report the year in which they were born. To obtain each respondent's age in years, we subtracted these values for each respondent from the year of the given survey. (CC06_V2020) Gender Respondents were asked to report their gender. This variable is coded "1" for males and "0" for females. (CC06_V2004) Race Respondents were asked to report the ethnic/racial category which best describes them. From this variable, we created dummies for African Americans, Hispanics, Asians, and "Other", leaving White and all other racial designations as the excluded category of comparison. (CC06_V2005) Education Respondents were asked to report the highest level of education they have completed. Response options ranged from (1)-"Did not graduate from High School" to (6)-"Post-Graduate Degree." From this question, we created six dummy variables, each coded "1" for respondents in each education category. Respondents lacking a High School diploma were used as the excluded category for comparison. ("Education") Income Respondents were asked to report their total annual family income. Response options ranged from (1)-"Less than $10,000" to (14)-"$150,000 or more." Missing data was imputed via regression imputation using respondent's education, gender, race (i.e., Black or Hispanic), and the interaction of education with gender and race. ("Income") Employment Status Respondents were asked to report their employment status. From this item, we created a dummy variable coded "1" for respondents who reported being temporarily laid off or unemployed, and "0" otherwise, as well as a dummy variable coded "1" for respondents who reported being employed part-time, and "0" otherwise. (CC06_V2030) Homeownership This is a dummy variable, coded "1" for respondents reporting ownership of their current residence, and "0" for those indicating renter status or "other." State Economic Evaluations Respondents were asked: "Now thinking about your state's economy, would you say that over the past year, your state's economy has . . ?" Response options ranged from (1)-"Gotten much better" to (3)-"Stayed the same" to (5)-"Gotten much worse." This item was coded to range from viewing the economy as gotten much worse to gotten much better. (v3009). Party Agreement This is a dummy variable coded "1" when the self-reported party ID of the respondents matched the party affiliation of the incumbent Senator in their state of residence, and "0" otherwise. Respondents partisan affiliation were based upon a standard 7-point party ID scale (CC06_V3007) Religiosity We constructed a religiosity scale based upon three questions: (1) church attendance, (2) frequency of prayer, and (3) the personal importance of religion. Each scale ranges from low to high religiosity. (CC06_V2026 & CC06_V2027 & CC06_V2029)</p><p>1 SUPPLEMENTAL APPENDIX B Creation and Distribution of Zip Code Gini Coefficients</p><p>Our zip code level estimates of the Gini Coefficient are based upon zip code household income data from the 2000 Decennial Census. We use the 2000 Decennial Census data because the Census Bureau does not provide estimates of Gini at the zip code level prior to the 2007 ACS</p><p>(i.e., after the 2006 CCES), and the 2005 ACS—the only ACS conducted before the 2006 CCES</p><p>—does not provide exhaustive coverage of all U.S. zip codes. Thus, to obtain comprehensive zip code level data collected prior to the 2006 CCES, and thus a "pretreatment" measures of our key independent variable, we rely upon the 2000 Decennial Census and manually estimate Gini for all zip codes. </p><p>To generate ZIP-code-level estimates of Gini coefficients for the 2000 Census, we worked from table P055 (“Age of Householder by Household Income in 1999”) reported in </p><p>Summary File 3 (available at: http://www2.census.gov/census_2000/datasets/Summary_File_3/).</p><p>An R script first collapsed the table to eliminate age. Then, the script reshaped the data from wide to long format, such that each row uniquely reported one of sixteen income categories per </p><p>ZCTA (1 = $9,999 or less, 2 = $10,000 - $14,999, 3 = $15,000 - $19,999, 4 = $20,000 - $24,999,</p><p>5 = $25,000 - $29,999, 6 = $30,000 - $34,999, 7 = $35,000 - $39,999, 8 = $40,000 - $44,999, 9 =</p><p>$45,000 - $49,999, 10 = $50,000 - $59,999, 11 = $60,000 - $74,999, 12 = $75,000 - $99,999, 13 </p><p>= $100,000 - $124,999, 14 = $125,000 - $149,999, 15 = $150,000 - $199,999, 16 = $200,000 and over). The program then recoded income to be the rounded midpoint for each category (1 = </p><p>$5,000, 2 = $12,500, and so on), with 16 coded as $250,000. The script then expanded each </p><p>ZCTA/income dyad by the number of households therein. The unit of analysis became each household in the Census (n = 105,539,122). To avoid importing parametric assumptions and to </p><p>2 keep things simple, we deliberately eschewed solutions like simulating ZIP-level income using </p><p>R’s various random distribution functions.</p><p>We computed Gini coefficients of recoded household income for each district using the </p><p>“ineq” package, written by Achim Zeileis and Christian Kleiber, and available for download on the Comprehensive R Archive Network (CRAN). A description of the library is available at </p><p><https://cran.r-project.org/web/packages/ineq/>. A caveat is that the reported Gini coefficients are slight “lowball” estimates (mean ZIP index = 40.10; the 2000 national index as reported by the World Bank = 40.46), because incomes of high-earning households are capped at $250,000, whereas recent income growth has been especially concentrated in those earning, for example, </p><p>$1 million or more. Replication files are available upon request. As a validity check, we find that our 2000 zip code estimates of Gini are highly correlated with the estimates provided by the </p><p>Census Bureau in the 2007-2011 ACS 5-year file (r = .70), and the results presented in Table 1 hold when using the 2007-11 ACS Gini estimates instead of our year 2000 estimates</p><p>3 Figure B1. Distribution of Zip Code Gini Coefficient 8 6 y t i s n 4 e D 2 0 0 .2 .4 .6 .8 Gini Coefficient (Zip Code)</p><p>Source: 2000 Decennial Census Zip Code Data File</p><p>Notes: The x-axis is the raw Gini Coefficient (i.e., not the 0 to 1 recoded scale used for the analysis in the article). The distribution of Gini at the zip code level approximates a normal distribution. The mean of zip code Gini throughout the entire U.S. is .402 and standard deviation of .06. When we estimate the mean in states with Democratic incumbents, the mean is .407 and the standard deviation is .047. For states with Republican incumbents, the mean is .403 and the standard deviation is .050. Thus, the distribution of zip code inequality does not differ much across states with Democratic and Republican incumbents in the 2006 Senate Election. Additionally, when looking at the distribution of Gini across values of incumbent Senator's E- score, we again find negligible differences. For example, when breaking E-NOMINATE scores into tertiles, incumbents in the low tertile (i.e., economic liberals), the mean zip code Gini is .646 and the standard deviation is .077. For incumbents in the middle tertile of E-NOMINATE Score (i.e., economic moderates), the mean zip code Gini is .646 and the standard deviation is . 070. For incumbents in the high tertile of E-NOMINATE scores (i.e., economic conservatives), the mean is .641 and the standard deviation is .083. </p><p>4 SUPPLEMENTAL APPENDIX C Auxiliary Analyses</p><p>Table C1. Re-Estimation of Interactive Model in Table 1 Using Alternative Measure of Zip Code Income Inequality Vote for Incumbent Local Inequality 80/20 Ratio 1.46** (.536) Moderator E-NOMINATE -.628 (.956) Interaction 80/20 E-NOMINATE -2.79** (.903) Contextual Controls Median Income -.170 (.636) Unemployment Rate -.567 (1.04) Percent Black .006 (.241) Republican Vote -1.21*** (.325) Population Density -.19 (.578) Individual Controls Education .303** (.098) Income -.304** (.121) Age -.0002 (.002) Male -.066 (.047) Black .428*** (.106) Hispanic .156^ (.086) Unemployed .082 (.142) Homeowner -.066 (.066) State Economic Evaluation -.261** (.096) Party Agreement 4.43*** (.061) Religiosity -.286*** (.069) Controlled Interactions Median Income E-NOMINATE -1.10 (1.01) Republican Vote E-NOMINATE 2.83*** (.556) Constant -.55 (.588) LR Test 336.21*** # Level 1 units (Individuals) 17,013 # Level 2 units (Zips) 7,075 # Level 3 units (States) 28 Notes: Entries are unstandardized regression coefficients from a random intercept logistic regression model estimated using xtmelogit in Stata®, standard errors are in parentheses. ^p<.10, *p<.05, **p<.01, ***p<.001, based upon two-tailed hypothesis tests.</p><p>5 Table C2. Re-Estimation of Interactive Model in Table 1 Controlling for Interaction of E-NOMINATE with Population Density Vote for Incumbent Local Inequality Gini Coefficient 2.77*** (.761) Moderator</p><p>6 E-NOMINATE 2.48* (1.35) Interaction Gini E-NOMINATE -5.91*** (1.27) Contextual Controls Median Income .655 (.678) Unemployment Rate -.365 (1.07) Percent Black .002 (.243) Republican Vote -.988** (.331) Population Density .343 (.686) Individual Controls Education .308** (.099) Income -.303** (.121) Age -.0003 (.001) Male -.07 (.049) Black .438*** (.106) Hispanic .157^ (.087) Unemployed .087 (.143) Homeowner -.072 (.066) State Economic Evaluation -.259** (.095) Party Agreement 4.43*** (.061) Religiosity -.288*** (.069) Controlled Interactions Median Income E- NOMINATE -3.25** (1.14) Republican Vote E-NOMINATE 2.01*** (.615) Pop. Density E-NOMINATE -6.13** (2.91) Constant -1.74 (.719) LR Test 338.35*** # Level 1 units (Individuals) 17,039 # Level 2 units (Zips) 7,094 # Level 3 units (States) 28 Notes: Entries are unstandardized regression coefficients from a random intercepts logistic regression model estimated using xtmelogit in Stata®, standard errors are in parentheses. p<.10, *p<.05, **p<.01, ***p<.001, based upon two-tailed hypothesis tests.</p><p>7 Table C3. Re-Estimation of Interactive Model in Table 1 Controlling for Additional Incumbent and Election Characteristics Vote for Incumbent Local Inequality Gini Coefficient 2.81*** (.785) Moderator E-NOMINATE 1.45 (1.20) Interaction Gini E-NOMINATE -6.18*** (1.26) Incumbent / Election Controls Nonwhite Incumbent -1.64** (.609) Female Incumbent -.189 (.384) Tenure in Office .025^ (..014)</p><p>8 Incumbent Spending -5.62** (2.04) Challenger Spending 3.98 (3.39) Contextual Controls Median Income .741 (.701) Unemployment Rate -.212 (1.08) Percent Black -.114 (.244) Republican Vote -1.05** (.337) Population Density -.571 (.598) Individual Controls Education .200** (.101) Income -.269* (.121) Age .0002 (.001) Male -.054 (.049) Black .312*** (.108) Hispanic .141 (.087) Unemployed .075 (.144) Homeowner -.036 (.065) State Economic Evaluation .244* (.107) Presidential Approval -.788*** (.075) Party Agreement 4.52*** (.065) Religiosity -.083 (.072) Controlled Interactions Median Income E-NOMINATE -3.41** (1.13) Republican Vote E-NOMINATE 2.41*** (.559) Constant -51.27^ (29.17) # Level 1 units (Individuals) 17,000 # Level 2 units (Zips) 7,087 # Level 3 units (States) 28 Notes: Entries are unstandardized regression coefficients from a random intercept logistic regression model estimated using xtmelogit in Stata®, standard errors are in parentheses. Spending variables are in units of $1,000. ^p<.10, *p<.05, **p<.01, ***p<.001, based upon two-tailed hypothesis tests.</p><p>9 SUPPLEMENTAL APPENDIX D Re-Analysis Excluding Politically Atypical Senators</p><p>One concern with our analysis is that our results a being influenced by the couple of </p><p>Senators who had atypical V-Scores. When reviewing our data, we found two such Senators: (1) </p><p>Lincoln Chaffee (R) from Rhode Island, whose E-NOMINATE scores are below the 5th percentile relative to his peers in the Republican Party, and (2) Ben Nelson (D) from Nebraska, whose E-NOMINATE score is above the 95th percentile relative to his peers in the Democratic </p><p>Party. Both of these Senators were well known for being substantially out-of-step with their respective parties, with Nelson being known as one of the most conservative Democrats in the </p><p>10 Senate, and Chaffee ultimately switched his affiliation to the Democratic Party in 2008. To ensure that respondents in our data voting for these two incumbents were not exerting a strong influence over our results, we re-estimated the interactive model presented in Table 1 excluding respondents in the RI and NE Senatorial Elections. The results are presented in Table D1 below.</p><p>As can be seen, the results completely hold when removing respondents voting for these atypical </p><p>Senators from the analysis.</p><p>Table D1. Re-Estimation of Interactive Model in Table 1 Excluding Respondents in RI and NE with Politically Atypical Incumbents Vote for Incumbent Local Inequality Gini Coefficient 2.93*** (.761) Moderator E-NOMINATE 2.31^ (1.22) Interaction Gini E-NOMINATE -6.28*** (1.26) Contextual Controls Median Income .68 (.680) Unemployment Rate -.516 (1.09) Percent Black -.039 (.244) Republican Vote -1.10*** (.327) Population Density -.391 (.585) Individual Controls</p><p>11 Education .208** (.101) Income -.301* (.122) Age -.0001 (.002) Male -.066 (.050) Black .437*** (.107) Hispanic .158^ (.086) Unemployed .094 (.143) Homeowner -.068 (.066) State Economic Evaluation -.247** (.096) Party Agreement 4.46*** (.062) Religiosity -.279*** (.069) Controlled Interactions Median Income E-NOMINATE -3.24** (1.14) Republican Vote E- 2.52*** (.564) ConstantNOMINATE -1.85 (.724) # Level 1 units (Individuals) 16,835 # Level 2 units (Zips) 6,975 # Level 3 units (States) 26 Notes: Entries are unstandardized regression coefficients from a random intercept logistic regression model estimated using xtmelogit in Stata®, standard errors are in parentheses. ^p<.10, *p<.05, **p<.01, ***p<.001, based upon two-tailed hypothesis tests.</p><p>12 SUPPLEMENTAL APPENDIX E Alternative Low Knowledge Model</p><p>In the analysis presented in Table 3, "low knowledge" is defined by voters either (a) reporting an incorrect position for their Senator's vote on minimum wage increases or capital gains tax cuts, or (b) reporting that they "Don't Know" their Senator's position on these roll call votes. This measure, however, lumps together voters who attempt to report a position taken by their Senators with those who flat-out report not knowing. In the following table (Table E1), we re-analyze the Low Knowledge model in Table 3 focusing only on voters who reported "Don't </p><p>Know" to the two CCES questions asking about their Senator's roll call votes. Here, we see that the results for our core variables of interest become even worse, as the estimated coefficients for </p><p>Gini and the interaction term are nowhere near statistically significant. These results demonstrate that when we further isolate the unknowledgeable via voters that report not knowing their </p><p>13 Senator's votes, we uncover even weaker results, which comport with our proposed mechanism that political knowledge facilitates punishment of inequality-enhancing elites for inequality. </p><p>Table E1. Re-Estimation of Low Knowledge Model in Table 3 Using Alternative Definition of Low Knowledge Vote for Incumbent Local Inequality Gini Coefficient -1.26 (1.80) Moderator E-NOMINATE -4.73^ (2.56) Interaction Gini E-NOMINATE 2.20 (2.88) Contextual Controls Median Income -3.41* (1.65) Unemployment Rate -.521 (2.56) Percent Black -.172 (.490) Republican Vote -2.47*** (.752) Population Density -1.94 (1.56) Individual Controls Education .675** (.233) Income -.414^ (.262) Age -.001 (.003) Male -.012 (.112) Black .088 (.246) Hispanic .259 (.195) Unemployed -.071 (.269) Homeowner .134 (.133)</p><p>14 State Economic Evaluation -.091 (.223) Party Agreement 3.51*** (.185) Religiosity -.310* (.153) Controlled Interactions Median Income E-NOMINATE 1.17 (2.66) Republican Vote E-NOMINATE 4.46*** (1.25) Constant 2.67 (1.56) # Level 1 units (Individuals) 2,889 # Level 2 units (Zips) 2,329 # Level 3 units (States) 28 Notes: Entries are unstandardized regression coefficients from a random intercept logistic regression model estimated using xtmelogit in Stata®, standard errors are in parentheses. p<.10, *p<.05, **p<.01, ***p<.001, based upon two-tailed hypothesis tests.</p><p>15 16</p>
Details
-
File Typepdf
-
Upload Time-
-
Content LanguagesEnglish
-
Upload UserAnonymous/Not logged-in
-
File Pages16 Page
-
File Size-