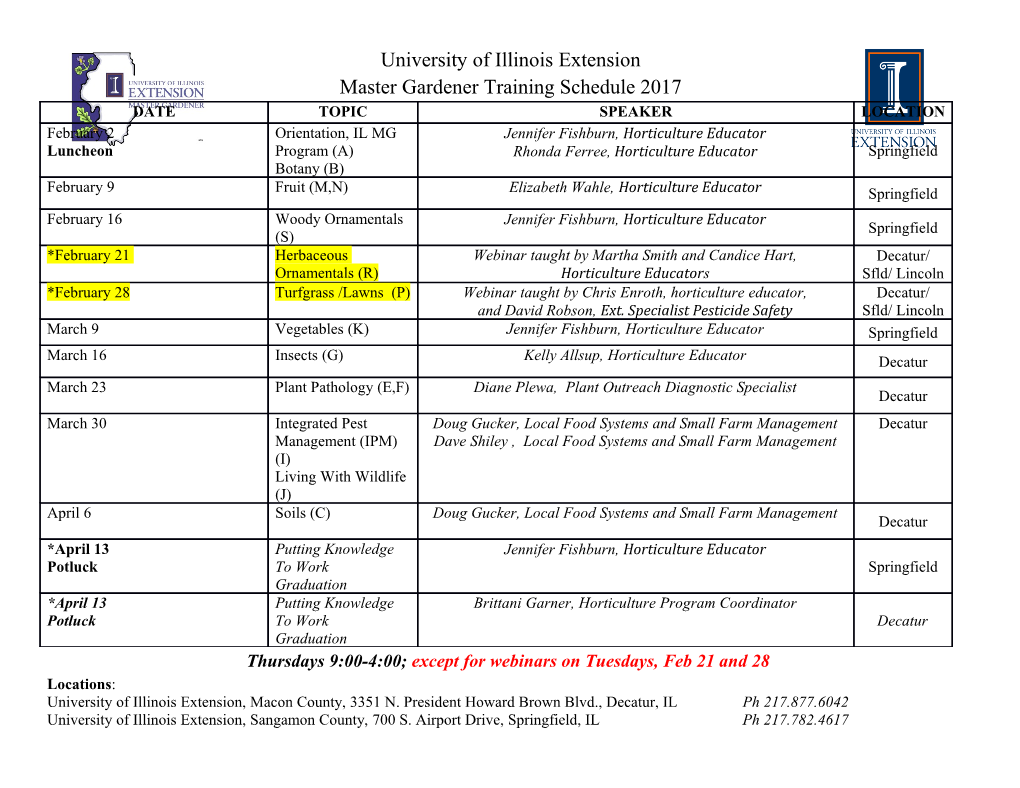
<p>Biomath/HG 207B and Biostat 237 1/20/04 Selecting Measured Covariates</p><p>Note: Here I only looked at main effects. For your homework you also needed to consider interaction terms between main effects. (1) Compare all models to the most general model using a LRT.</p><p>Model logL LRT DF p-value Accept Null hypothesis? (1) no -122.81 35.94 3 7.8x10-8 no covariates (2) age -108.80 7.92 2 0.019 no (3) male -122.52 35.36 2 2.10x10-8 no (4) bmi -109.82 9.76 2 0.0069 no (5) age, male -108.51 7.34 1 0.0067 no (6) age, bmi -105.76 1.84 1 0.175 Yes, (6) is not significantly worse than (8). (7) male, bmi -108.29 6.90 1 0.0086 no (8)age, male, -104.84 ------bmi (2) We could compare all models by LRT incrementing one variable at a time. </p><p>Model logL test (null LRT DF p-value model Comment vs. altern.) (1) no covariates -122.81 (2) age -108.80 1 vs 2 28.02 1 1.2 x 10-7 2 best 1 parameter model (3) male -122.52 1 vs 3 0.58 1 0.45 1 (4) bmi -109.82 1 vs 4 25.98 1 3.4 x 10-7 4 (5) age, male -108.51 2 vs 5 0.58 1 0.45 2 3 vs 5 28.02 1 1.2 x 10-7 5 (6) age, bmi -105.76 4 vs 6 8.12 1 0.0044 6 best 2 parameter 2 vs 6 6.08 1 0.014 4 model (6) is significantly better than (2) (7) male, bmi -108.29 4 vs 7 3.06 1 0.080 6 3 vs 7 28.46 1 9.6 x 10-8 7 (8)age, male, bmi -104.84 7 vs 8 6.9 1 0.0086 8 (8) is not 6 vs 8 1.84 1 0.18 6 significantly 5 vs 8 7.34 1 0.0067 8 better than (6) (3) Approach (2) is inefficient especially when there are a lot of covariates to try. Here is a more parsimonious approach using the LRT, stepwise regression. </p><p>Model loglikelihood test (null LRT DF p-value preferred versus model alternative) (1) no covariates -122.81 (2) age -108.80 1 vs 2 28.02 1 1.2 x 10-7 2 (3) male -122.52 1 vs 3 0.58 1 0.45 1 (4) bmi -109.82 1 vs 4 25.98 1 3.4 x 10-7 4 (5) male, age -108.29 2 vs 5 0.58 1 0.45 2 (6) age, bmi -105.76 2 vs 6 6.08 1 0.014 6 (8)age, male, bmi -104.84 6 vs 8 1.84 1 0.18 6 (4) When comparing models that are not nested, when the parameters are constrained to boundary values or when they are undefined then the LRT no longer has a simple chisquare distribution. One alternative for model selection is to use the Akaike Information Criterion (AIC). AIC = -2*logL+kN where N is the number of parameters and k is usually chosen to be 2. The best model is the one with the lowest AIC. The problems with using the AIC are (a) that the AIC constant k is arbitrary and (b) we no longer have a statistical test of significance (we can't tell if a model is significantly better). </p><p>Model logL N AIC Comments (1) no covariates -122.81 2 249.62 (2) age -108.80 3 223.60 (3) male -122.52 3 251.04 (4) bmi -109.82 3 225.64 (5) age, male -108.51 4 225.02 (6) age, bmi -105.76 4 219.52 Smallest AIC (7) male, bmi -108.29 4 224.58 (8)age, male, bmi -104.84 5 219.68</p>
Details
-
File Typepdf
-
Upload Time-
-
Content LanguagesEnglish
-
Upload UserAnonymous/Not logged-in
-
File Pages4 Page
-
File Size-