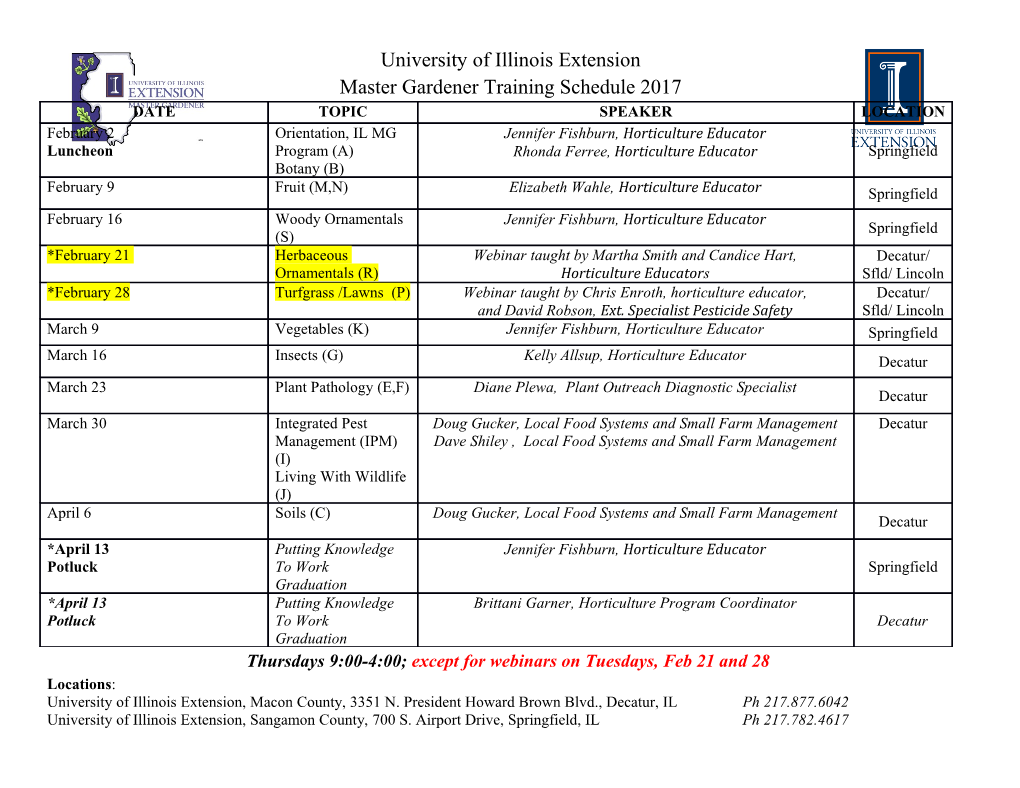
<p>Online Supplementary Material</p><p>Animal biometrics: quantifying and detecting phenotypic appearance</p><p>Hjalmar S. Kühl1 and Tilo Burghardt2</p><p>1Department of Primatology, Max Planck Institute for Evolutionary Anthropology, Deutscher Platz 6, 04103 Leipzig, Germany</p><p>2Department of Computer Science, University of Bristol, Woodland Road, Bristol, BS8 1UB, UK</p><p>Corresponding author: Kühl, H.S. ([email protected]).</p><p>Table S1: Visual Approaches towards Automated Animal Biometrics Categorization and summary of published techniques selected from the reference provided. PART I: SPECIES IDENTIFICATION</p><p>A Taxa Identificatio Pattern Recognition Techniques Annotation A S c n Requirement s y q Concept Modeling Model Matching s s F T s u (Profiled and Representati and o e a t i Pattern Learning on Locating c a r e s Information) Strategies (the Strategies i t g m i ‘Profile’) a u e t t r t M i e e L o o d e d n P T v a u y e l T b p l i y li e t p c y e a t S V M A Chimpanzee, Face - Boosting - Boxlet - Cascaded Rules training [38] P I O P Gorilla (Rigid Spatial - LookUp Features - Sliding Windows annotation of E S N P Decomposition Tables - Census facial region C U O E of Facial Features bounding box I A C A Features) - HOG Features E L U R Cat, Tiger, Animal Head - Boosting - Boxlet - Dual Approach training [48] S L A Lion, Panda, (Deformable - SVM Features - Sliding Windows annotation of [49] A N Fox, Cheetah Decomposition - Census head region and R C etc. of Head Shape Features facial features E and Texture) - HOOG Features Cat, Dog Face & Body - Latent SVM - Deformable - Sliding Windows training [75] (Deformable - Color Part-based - Grab Cut annotation of Decomposition Histograms Model - Segment facial region of Face Features - HOG Features Compactness bounding box & Homogeneity - Natural Edges Body Texture) - Pixel Color Various Distinctive - Gradient - SIFT - Scale-Space none [33] Species of Local Body Histograms - Local feature Extrema [34] Insect Features (Local concatenated - k-D Tree Gradient histograms Matching Distributions) African Body - Boosting - Boxlet - Cascaded Rules training [43] penguin (Rigid Spatial - Feature Features - Sliding Windows annotation of Decompositions Prediction key points on of Facial Trees body Features) M Quadrupeds Gait - PCA - Principal - Sparse Kanade- training based [28] O (Spatio- - Normalized Component Lucas-Tomasi on clip T temporal Convolution Vectors of tracking classification I configuration of motion fields O body part N motion) Ivory-Billed Flight - binary - principal axes - Spatio-temporal none – system [15] H Woodpecker characteristics segmentation of silhouette filters priming of Y (speed etc) + (against sky) - velocity and its profile B Silhouette derivatives parameters R information based on I anatomical D knowledge PART II: INDIVIDUAL IDENTIFICATION</p><p>A Taxa Identification Pattern Recognition Techniques Annotation A S c Concept s y q (Profiled Pattern Modeling and Model Matching Requireme s F T s u Information) Learning Representation and nts o e a t i Strategies (the ‘Profile’) Locating c a r e s Strategies i t g m i a u e t t r t M i e e L o o d e d n P T v a u y e l T b p l i y li e t p c y e a t I V M A Whale Shark Body Spots - Normalisation - Discrete - Groth’s Algorithm spot [16] N I O P (Configuration of based on Landmarks - I3S annotation or D S N P Discrete Landmarks Similarity verification I U O E on Sides of Body) Assumption V A C A Cheetah Body Spots/Stripes - Spline-based - Discrete - Convolution manual fitting [21] I L U R Tiger (Configuration of Texture Landmarks - Proprietary of spline [22] D L A Discrete Landmarks Backprojection Distance Measure model by U A N on Sides of Body) using body A R C Grey Seal Body Texture - Binarised Surface - covariance-based landmarks as [40] L E (Dense texture of Texture Map texture matching control points main body segment) Leatherback Head/Body Surface - Gradient - SIFT - Scale-Space None [35] Turtle, Masai (Distinctive gradient Histograms - Local feature Extrema [47] giraffe orientation of head or concatenated - k-D Tree Matching body texture) histograms Chimpanzee, Face - Random Faces - Hybrid Global - Random Faces near-frontal [39] Gorilla (Conglomerate of and Local Features face, Pre- local and global face detection characteristics) using work in [48] Salamander Body Texture - Coarse-to-fine - None, the method - Scale-cascaded ground truth [41] (Blob-like shape of decomposition produces match diffeomorphic for learning of markings over main using Gabor decisions for input alignment spectral body segment) filters pairs weights during training n Manta Ray, Body Spots/Blobs - Procrustes’ - Discrete - L -Type distance spot [13] (Configuration of Alignment of I3S Landmarks measures or annotation [76] Raggedtooth discrete landmarks system entropy [77] Shark, Weedy on main body) Seadragons African penguin Chest Spots - Polar Spatial - Shape Contexts - Earth Movers none [43] (Configuration of Histograms Distance [46] discrete landmarks - Phase Curls on chest feathers) Elephants Ears - Edge Tracing - Shape features of - Local edge rough ear- [45] (Shape of ear ear silhouette refinement line silhouette sections) annotation Zebra Body Stripes - Median Filter - Stripe Strings - Dynamic bounding box [25] (Binary pattern - Binarisation Programming around body formed by stripe - Run-Length - Edit Distance side paths) Coding</p><p>PART III: BEHAVIOR IDENTIFICATION</p><p>Taxa Identification Pattern Recognition Techniques A A Concept n s (Profiled Pattern Modeling and Model Matchi n s A Information) Learning Representation ng o o S c Strategies (the ‘Profile’) and t c y q Locati a i F T s u ng t a e a t i Strate i t a r e s gies o e t g m i n d u e t P r t M i R u e L o o e b e d n q li T v a u c y e l T i a p l i y r t e t p e i y e m o e n n s t s B V M A Mice Home-cage Activity - Spatiotemporal - Motion, position - SVM- none – after [50] E I O P (Spatiotemporal volume analysis and velocity based training H S N P characteristics of features classifier model A U O E particular activities) Birds Nesting Behaviour - Classification - SIFT Scales - Fisher [78] (Bird based on local Information Linear A presence/absence at spatial frequency Discrimi C R nest, egg count) and scale nant U A - Hidden L N Markov A C Models V R E Flocking Behaviour - Tracking by - Particle Cloud and - [53] I (Bird pose and propagation of Subordination Subordin O A & trajectory in flock) positional beliefs ated U L Condens R M ation</p><p>O Giant honey Shimmering - Template - 3D position - [29] S T bees matching estimates of Triangul T Behaviour I individual bees ation of E (3D movements of O correspo R hive) N nding E templat O es</p><p>ADDITIONAL REFERENCES:</p><p>75. Parkhi, O. M. et al. (2011) The truth about cats and dogs. Proceedings of ICCV, 1427-1434. 76. Marshall, A.D. et al. (2008) Morphological measurements of manta rays (Manta birostris) with a description of a foetus from the east coast of Southern Africa. Zootaxa 1717, 24–30.</p><p>77. Martin-Smith, K. M. (2011) Photo-identification of individual weedy seadragons Phyllopteryx taeniolatus and its application in estimating population dynamics. Journal of Fish Biology 78, 1757-1768. </p><p>78. Ko, T. et al. (2010) Heartbeat of a nest: Using imagers as biological sensors. TOSN 6, 1-30.</p>
Details
-
File Typepdf
-
Upload Time-
-
Content LanguagesEnglish
-
Upload UserAnonymous/Not logged-in
-
File Pages7 Page
-
File Size-