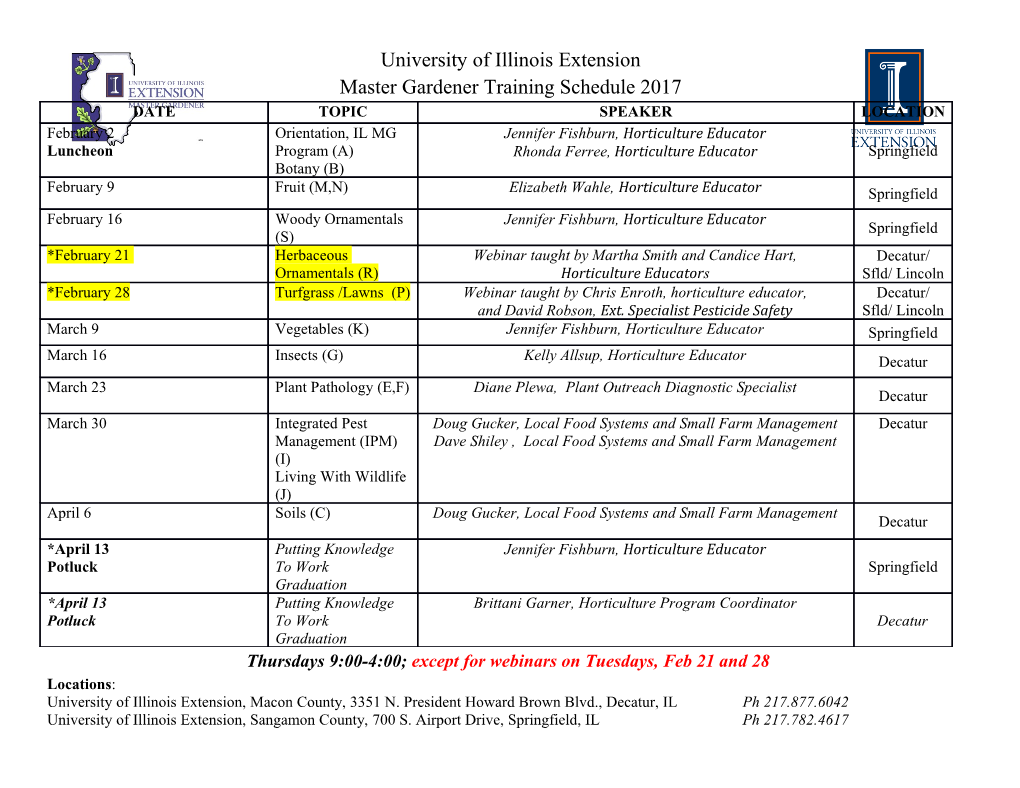
<p> A JOINT FLEXIBLE ECONOMETRIC MODEL SYSTEM OF HOUSEHOLD RESIDENTIAL LOCATION AND VEHICLE FLEET COMPOSITION/USAGE CHOICES</p><p>Naveen Eluru The University of Texas at Austin Dept of Civil, Architectural & Environmental Engineering 1 University Station C1761, Austin TX 78712-0278 Phone: 512-471-4535, Fax: 512-475-8744 E-mail: [email protected]</p><p>Chandra R. Bhat (corresponding author) The University of Texas at Austin Dept of Civil, Architectural & Environmental Engineering 1 University Station C1761, Austin TX 78712-0278 Phone: 512-471-4535, Fax: 512-475-8744 E-mail: [email protected] </p><p>Ram M. Pendyala Arizona State University School of Sustainable Engineering and the Built Environment Room ECG252, Tempe, AZ 85287-5306 Tel: (480) 727-9164; Fax: (480) 965-0557 Email: [email protected]</p><p>Karthik C. Konduri Arizona State University School of Sustainable Engineering and the Built Environment Room ECG252, Tempe, AZ 85287-5306 Tel: (480) 965-3589; Fax: (480) 965-0557 Email: [email protected]</p><p>August 2009 Revised November 2009 Eluru, Bhat, Pendyala, Konduri </p><p>ABSTRACT Modeling the interaction between the built environment and travel behavior is of much interest to transportation planning professionals due to the desire to curb vehicular travel demand through modifications to built environment attributes. However, such models need to take into account self-selection effects in residential location choice, wherein households choose to reside in neighborhoods and built environments that are conducive to their lifestyle preferences and attitudes. This phenomenon, well-recognized in the literature, calls for the specification and estimation of joint models of multi-dimensional land use and travel choice processes. However, the estimation of such model systems that explicitly account for the presence of unobserved factors that jointly impact multiple choice dimensions is extremely complex and computationally intensive. This paper presents a joint GEV-based logit regression model of residential location choice, vehicle count by type choice, and vehicle usage (vehicle miles of travel) using a copula- based framework that facilitates the estimation of joint equations systems with error dependence structures within a simple and flexible closed-form analytic framework. The model system is estimated on a sample derived from the 2000 San Francisco Bay Area Household Travel Survey. Estimation results show that there is significant dependency among the choice dimensions and that self-selection effects cannot be ignored when modeling land use-travel behavior interactions.</p><p>Keywords: land use and travel behavior, residential location choice, vehicle type choice, vehicle usage, vehicle miles of travel, joint model, copula-based approach, simultaneous equations model. Eluru, Bhat, Pendyala, Konduri 1</p><p>1. INTRODUCTION This paper focuses on understanding the effects of land use measures on vehicle ownership by type of vehicle and usage. Understanding the interaction between land use and travel behavior has been of much interest to the profession, with a long history and strand of literature devoted to this subject (e.g., 1-4). There are descriptive studies that compare travel behavior characteristics of households and individuals residing in low density land use configurations against those that reside in higher density mixed land use configurations. There are studies that consider the impacts of residential location characteristics on a host of travel behavior characteristics including, for example, mode choice (5,6), auto ownership (4,7), vehicle miles of travel, activity time use patterns (8) and amount of non-motorized travel (9). Thus, the interplay between land use and travel behavior remains a major focus area of research in the profession and continues to be of much interest particularly in the context of developing integrated land use-transport models that effectively model the impacts of alternative land use strategies on travel demand. In recent years, there has been an explicit recognition in the integrated land use-transport modeling field that the treatment of residential land use characteristics as exogenous factors (variables) in models of vehicle ownership and use (or any travel behavior model) may provide erroneous indications of the true impacts of land use on travel behavior. This is due to the phenomenon referred to as “self-selection” where households or individuals who have a proclivity towards a certain lifestyle may choose or “self-select” to reside in neighborhoods that support their lifestyle preferences. People’s attitudes, preferences, and values, not to mention their socio-economic and demographic characteristics, undoubtedly play a role in shaping behavioral choices (7,10-12). If an individual who tends to be environmentally conscious and enjoys a non-motorized travel lifestyle characterized by bicycling and walking chooses to reside in a high-density mixed land use development, it is likely that the residential location choice was influenced by the lifestyle and travel preferences of the individual (as opposed to the travel choices being driven by the land use pattern of the residential location). In other words, residential location choice is endogenous to vehicle ownership choice by vehicle type, vehicle usage decisions, and other travel behavior choices that are made by individuals and households in which they reside. In light of the key role that vehicle ownership, vehicle type choice, and vehicle usage have played in travel demand analysis over many decades, and in the global climate change debate more recently, there has been considerable research the determinants of vehicle ownership, household fleet composition (vehicle type mix), and vehicle usage (usually measured in vehicle miles of travel). Work in this area has ranged from simple regression or discrete choice models of levels of auto ownership (e.g., 13) and vehicle type choice (14-16) to more sophisticated models of vehicle acquisition, disposal, and replacement (17). More recently, there has been considerable work on modeling household fleet composition in terms of the mix of vehicle types owned by a household together with the amount that each vehicle in the household is used. But these models often treat residential location choice variables (land use measures) as exogenous variables that influence vehicle fleet ownership and usage (e.g., 18-19). Further, many of these earlier studies consider the jointness in vehicle type choice and usage for the most recent vehicle or most driven by the household [for example, see Choo and Mokhtarian (10), Mohammadian and Miller (16), Spissu et al. (20)], or confine their attention to households with two or fewer vehicles [see West (21)]. Overall, there has been relatively little research on treating residential choice as being endogenous in vehicle type and usage decisions, or on examining the entire vehicle fleet composition and usage characteristics of households. Eluru, Bhat, Pendyala, Konduri 2</p><p>This paper contributes to the literature on land use and travel demand by explicitly integrating household vehicle ownership, vehicle type, and vehicle usage decisions with residential location decisions of households. Such a joint model can be used to conduct a host of policy analyses aimed at reducing GHG emissions and fuel consumption. The joint model system is estimated on a data set derived from the 2000 San Francisco Bay Area Household Travel Survey (BATS) that has been comprehensively augmented with land use and network level of service attributes. The paper starts with a presentation of the methodology in the next section. A brief description of the data is offered in the third section. The fourth section presents model estimation results while the fifth section offers a discussion on the simultaneity in the choice processes and a sample model application. Concluding thoughts are offered in the sixth section. </p><p>2. MODELING METHODOLOGY In this section, the model framework to jointly model residential location, vehicle ownership and type choice, and vehicle usage, is discussed first followed by a detailed presentation of the model structure and model estimation procedure.</p><p>2.1 Model Framework The number of dimensions that need to be modeled in the joint residential choice and vehicle fleet composition/usage system is high, especially because of the consideration of multiple vehicles in the household. One appealing approach to accommodating the high number of dimensions due to multiple vehicles is to consider a multiple discrete-continuous extreme value (MDCEV) based model, as undertaken by Bhat et al. (19). The approach is quite elegant and relatively simple, but, when applied to vehicle fleet composition analysis, is predicated on the assumption that the process of acquiring vehicles is instantaneous and based on “horizontal” choice behavior. The basic supposition is that, at a given instant, individuals choose to purchase the number of vehicles they want to own as well as the vehicle type and use decisions. However, it is more reasonable to assume that the fleet ownership of households is based on repeated choice decisions over time, with the choices made at an earlier occasion influencing future choices. The MDCEV approach is fundamentally at odds with this more realistic process of household vehicle ownership and use. Further, the MDCEV approach ties the discrete and continuous choices in a restrictive framework by having a single stochastic utility function (and therefore, a single error term) that underlies both the discrete and continuous choices. Finally, the MDCEV approach needs to have an exogenous total mileage budget of households for implementation. Bhat et al. (19) develop this budget by aggregating the mileage across all vehicles held by a household and adding non-motorized mode mileage. However, the non- motorized mileage is a relatively negligible fraction of total mileage, effectively imposing the constraint that total motorized vehicle utilization is exogenous, and does not change in response to policies or fuel cost increases (though the MDCEV model allows substitution in vehicle mileage across different vehicle types). In the current paper, a different approach is adopted to accommodate the many dimensions characterizing vehicle fleet/usage decisions. Multiple vehicle ownership and usage dimensions are accommodated by assuming that vehicle fleet and usage decisions are determined through a series of unobserved (to the analyst) repeated discrete-continuous choice occasions [see Hendel (22) and Dube (23), who have earlier used a repeated choice framework to handle the purchase and consumption levels of multiple items in a marketing context]. The number of choice occasions in such a “vertical” choice behavior is linked to the number of adults in the Eluru, Bhat, Pendyala, Konduri 3 household. In particular, since the number of vehicles is never greater than the number of adults in the household plus 1 in the data used in this empirical context, the number of choice occasions is set to be equal to the number of adults plus 1. At each choice occasion, the household may choose not to purchase a vehicle or to acquire a vehicle of a certain type. However, the choice of residential location, vehicle ownership, vehicle type and vehicle utilization are likely to be multiple dimensions of a single choice bundle at each choice occasion. For example, a household that is environmentally conscious may deliberately decide to locate in a neo-urbanist neighborhood, have few cars (as reflected in the choice of zero cars on one or more choice occasions of the household), favor compact vehicles in each choice occasion, and use the chosen vehicles relatively sparingly. This joint nature of the decisions is recognized at each choice occasion by proposing a joint discrete-continuous copula-based framework [the use of a copula framework is a deviation from earlier modeling approaches of repeated discrete-continuous choices, including those of Dube (23) and Bento et al. (24)]. In the framework, the decision of residential choice, and choice of no vehicle purchase or one of several vehicle types, is captured using a GEV-based logit model, while vehicle utilization (as measured by annual vehicle miles of travel or VMT) of the chosen vehicle type is modeled using a continuous regression model. Note that one can use this framework to model any representation of residential choice (such as neo- urbanist versus traditional neighborhoods as in Bhat and Eluru (4) or multiple residential choice alternatives based on density as in Brownstone and Golob (25) and any taxonomy of vehicle types. Also important is that the number of vehicles owned by the household is endogenously, even if implicitly, determined as the sum of those choice occasions when the household selects a certain vehicle type. Overall, the proposed approach jointly models residential choice and all vehicle fleet characteristics in a unifying framework. To implement this framework in estimation, “synthetic” repeated choice occasions for each household are generated based on the number of adults in the household. Appropriate vehicle type choices are assigned to each choice occasion in the estimation sample. For example, consider a household with two adults, and two vehicles – a coupe and a compact sedan. For this household, three choice occasions (2 adults +1) are created with the chosen alternatives for the choice occasions being coupe, compact sedan and “no vehicle”. In the data set used in the empirical analysis part of this paper, the temporal sequence of the purchase of the vehicles currently owned is known. Thus, it is possible to capture the impacts of the types of vehicles already owned on the type of vehicle that may be purchased in a subsequent purchase decision. In the example above, if the coupe is the first vehicle purchased and the sedan is the second one purchased, coupe is assigned as the chosen alternative at the first choice occasion, and sedan as the chosen alternative in the second. In the second choice occasion, information that the household has a coupe is used as an explanatory variable. This “mimics” the dynamics of fleet ownership decisions.1 </p><p>1 Note that in the example just provided, one could also assign the chosen alternatives to the choice occasions as follows: coupe in first choice occasion, no vehicle in the second, and sedan in the third. This is in place of coupe in the first, sedan in the second and no vehicle in the third. But both these assignments will give the same results, because the “dynamics” are based on what the household already owns in totality, not what was chosen in the immediately previous choice occasion. Of course, for the first choice occasion, there are no explanatory variables related to the vehicle types already chosen, because there is no information on what the household owned prior to the set of vehicles currently held. Eluru, Bhat, Pendyala, Konduri 4</p><p>2.2 Model Structure 2.2.1 Joint Residential Location-Vehicle Type Choice Model Component (Discrete Choice Component) Let q be the index for households, (q = 1, 2, ..., Q ) and let i be the index for the possible combinations of residential location alternatives and vehicle type alternatives. For example, if residential location is characterized by two alternatives (residing in a neo-urbanist neighborhood and residing in a traditional neighborhood) and vehicle type is represented by three alternatives (no vehicle purchased, sedan, and coupe; for ease in presentation, the “no vehicle” purchased case will be treated as a vehicle type alternative), there are 6 possible combinations of residential location and vehicle type alternatives, and i = 1, 2, 3, 4, 5, 6. More generally, let i = 1, 2, …, I. Also, let j be the index to represent the vehicle choice occasion (j= 1, 2,... J , where J is the number of adults in the household q plus 1). With this notation, the residential location-vehicle type discrete choice model component takes the familiar random utility formulation: * ' uqij=b x qij + e qij (1)</p><p>* th In the equation above, uqij is the latent utility that the q household derives from choosing alternative i at the jth choice occasion. xqij is a column vector of known household attributes at choice occasion j (including household demographics, types of vehicles “chosen” before the jth choice occasion, and activity-travel environment characteristics), b is a corresponding coefficient column vector of parameters to be estimated, and eqij is an idiosyncratic error term assumed to be standard type-1 extreme value distributed. Then, in the usual framework of random utility maximization, household q will choose alternative i at the jth choice occasion if the following condition holds:</p><p>* * uqij> max u qsj s=1,2,..., I , s i (2)</p><p>The condition above can be equivalently written in the form of a series of binary choice formulations for each alternative i [see Lee (26)]. To see this, let Rqij be a dichotomous variable</p><p> th th that takes the values 0 and 1, with Rqij = 1 if the i alternative is chosen by the q household</p><p> th at the j choice occasion, and Rqij = 0 otherwise. Then, one can recast the discrete choice model ' * formulation in Equation (2) by substituting bxqij+ e qij for uqij [from Equation (1)]:</p><p>' Rqij =1 ifb xqij > vqij , ( i = 1,2,... I ) (3) * wherevqij= { max u qsj } - eqij s=1,2,..., I , s i (4)</p><p>With the structure in Equation (4) and an appropriate Generalized Extreme Value (GEV) distribution assumption on the eqij terms, the residential location-vehicle type choice probability expressions at each choice occasion j take the usual GEV form [see McFadden (27)]. In the Eluru, Bhat, Pendyala, Konduri 5 model, it is assumed that the error terms eqij are independent and identically distributed (IID) across households q and choice occasions j, and that they are identically distributed (but not 2 necessarily independent) across alternatives i. Let Fvi (.) be the marginal distribution of vqij implied by the assumed GEV distributional form for the eqij terms and the relationship in Equation (4). This implied distribution is very straightforward to obtain, since it is based on the probability expression for the corresponding discrete choice model. For example, if the eqij terms are independent across alternative i, then, from Equation (3), it must be the case that:</p><p> exp( x ) F (v x ) qij vi qij qij , and therefore exp( xqij ) exp( xqsj ) si exp(w ) Fvi( v qij<b x qij ) = exp(w )+ exp(b xqsj ) s i</p><p>If some other GEV form is used for the eqij terms, then the implied distribution of vqij will take the corresponding GEV probability form. </p><p>2.2.2 The Vehicle Mileage Model Component In the current modeling framework, the vehicle mileage model component takes the form of the classic log-linear regression, as shown below:</p><p>* ' * mqij=a z qij + h qij, m qij = 1[ Rqij = 1] mqij (5)</p><p>* In the equation above, mqij is a latent variable representing the logarithm of annual mileage on the vehicle of type i if it had been chosen at the jth choice occasion. This latent vehicle usage variable is mapped to the observed household attributes and the corresponding attribute effects in ' the form of column vectors zqij and a , respectively, as well as to unobserved factors through a hqij term. On the right hand side of this equation, the notation 1[Rqij = 1] represents an indicator function taking the value 1 if household q chooses vehicle type i in the jth choice * q occasion, and 0 otherwise. That is, mqij is observed (in the form of mqij ) only if household is observed to actually acquire a vehicle of type i at the jth choice occasion. It is assumed that the</p><p>qij error terms are independent and identically distributed (IID) across households q and choice occasions j, and that they are identically distributed (but not necessarily independent) across alternatives i. Further, since the annual mileage for the chosen vehicle is only observed at each choice occasion, any dependence between the qij terms across alternatives i is not identified. </p><p>2 The IID assumptions across households and choice occasions can be relaxed in a conceptually straightforward manner by accommodating mixing distributions. This is left for future research, and focus in the current paper on implementing the fundamental “vertical” choice approach. Eluru, Bhat, Pendyala, Konduri 6</p><p>2.2.3 The Joint Model: A Copula-based Approach In this sub-section, the specifications of the individual model components discussed in the previous two subsections are brought together in the following equation system:</p><p>R=1 ifb' x >, ( i = 1, 2,... I ) ( j = 1, 2,... J ) qij i qij vqij (6) *' * mqij=a i z qij+ h qij, m qij = 1[ R qij = 1] m qij</p><p>The type and the extent of the dependency between the stochastic terms vqij and hqij for household q determines the level of dependency between the underlying propensity of vehicle type choice and vehicle usage decisions for the household. In the research effort, as indicated earlier, copula-based methods are used to capture and explore these dependencies (or correlations). In particular, copulas are used to describe the joint distribution of the vqij and hqij terms. In this approach, first, the vqij and hqij terms are transformed into uniform distributions using their inverse cumulative distribution functions. Subsequently, copulas are applied to “couple” the uniformly distributed inverse cumulative distributions into multivariate joint distributions. To explicate, lets assume that the marginal distributions of vqij and hqij be Fvi (.) F (.,.) and Fhi (.) , respectively, and let the joint distribution of vqij and hqij be vi,h i . </p><p>Subsequently, consider Fvi,h i ( y 1 , y 2 ), which can be expressed as a joint cumulative probability distribution of uniform [0,1] marginal variables U1 and U 2 as below:</p><p>F( y , y )= P(v < y ,h < y ) vi,h i 1 2 qij1 qij 2</p><p>= P(F-1 ( U )< y , F - 1 ( U ) < y ) vi11h i 2 2 (7)</p><p>= P(U< F ( y ), U < F ( y )) 1vi1 2 h i 2</p><p>Then, the above joint distribution (of uniform marginal variables) can be generated by a function</p><p>Cq (.,.) such that (28):</p><p>F( y , y )= C ( u= F ( y ), u = F ( y )) vi,h i 1 2 q1 vi1 2 h i 2 (8) where Cq (.,.) is a copula function and q is a dependency parameter (assumed to be scalar), together characterizing the dependency between vqij and hqij . The joint distribution formed in the above-discussed manner is used to derive the joint residential location, and vehicle ownership and type combination choice, and vehicle mileage probabilities and log-likelihood expressions. Eluru, Bhat, Pendyala, Konduri 7</p><p>2.3 Model Estimation The joint model based on the formulation above has the following log-likelihood expression for a random sample of Q households (q = 1, 2, ..., Q ) :</p><p>Q 轾J I 禳 '(R * H ) ' Rqij L= 犏照 睚 P mqij|bx qij> n qijqij qj � P b x qij n qij . (9) 犏 ( ) ( ) q=1 臌j=1 i = 1铪</p><p> where Hqj = 1 if it is not the case that the household q chooses no vehicles at choice occasion j, and 0 otherwise. The conditional distributions in the above expression can be expressed as:</p><p>骣 ' -1 mqij-a z qij P m|b' x> v =轾 P b ' x > v F琪 b ' x , ( qij qijqij) 臌犏( qij qij ) vi,h i 琪 qij mqi 桫 shi -1 轾 '1 ' =Pb xqij > v qij创 Fvi,h i b x qij , t m-a' z 臌犏 ( ) t ( ) qij qij (10) shi t = shi i i ' -1 C u, u 骣m- a z 轾 ' 1 qi( q1 q 2 ) qij qij =P(b xqij > v qij ) 创 fhi 琪 臌犏 sui 琪 s hi q2 桫 hi</p><p> i i i ' where Cqi (.,.) is the copula corresponding to Fvi,h i ( uq1 , u q 2 ) with uq1 = F vi(b i x qij ) and i i 骣m -a' z Cqi( u q1, u q 2 ) ui = F 琪 qij i qij , is the partial derivative of the copula with respect to q2 h i 琪 s i 桫 hi uq2 i h uq2 [see Bhat and Eluru (4)], fhi is the probability density function of qij , and shi is the scale parameter of hqij . Substitution of the above conditional distribution expression back into Equation (10) provides the following log-likelihood expression for the joint residential location, and vehicle ownership and type combination choice, and vehicle usage model:</p><p>轾 (R * H ) 禳 i i qij qj Q J I 犏 Cqi u ,u 骣m -a' z 犏镲1 ( q1 q 2 ) 琪 qij qij ' (1- Hqj ) L= 照 睚 � fhi琪 *P(b x qij n qij ) (11) 犏s ui 琪 q=1 j=1 i = 1 镲hiq2 s h i 犏铪 桫 臌犏</p><p>A particular advantage of the copula-based approach is that, in the above log-likelihood expression, several different copula [i.e.,Cqi (.,.) ] functions can be explored to characterize the dependency between the residential location-vehicle type choice discrete component and the continuous vehicle miles of travel (VMT) component [see Bhat and Eluru (4) for a review of alternative copula functions available in the literature]. Specifically, the copula approach allows Eluru, Bhat, Pendyala, Konduri 8 us to test a variety of radially symmetric and asymmetric joint distributions to appropriately accommodate the dependency between choice dimensions. Another appealing feature is that the copula approach separates the marginal distributions from the dependence structure so that the dependence structure is entirely unaffected by the marginal distributions assumed. Finally, Equation (11) has a closed form expression for most of the copulas available in the literature and hence obviates the need to adopt the more computationally intensive simulation-based procedures for parameter estimation. In this paper, six different copulas are chosen from the rich set of copulas available. These include the following: (1) Gaussian copula, (2) Farlie-Gumbel- Morgenstern (FGM) copula, (3) Clayton, (4) Gumbel, (5) Frank, and (6) Joe copulas (the reader is referred to Bhat and Eluru (4) for more details on these six copula structures). To complete the model specification, in this paper, it is assumed that the marginal h 2 distribution of the qij terms follows a normal distribution centered at zero with variance shi .</p><p>For the eqij terms, two GEV-based distributional assumptions were explored. The first was independence across alternatives i, leading to a multinomial logit (MNL) model for the residential choice-vehicle type choice component of the model system. The second was a nesting structure with residential location choice at the top level and vehicle type choice at the bottom level, to recognize that common unobserved residence location-based effects may increase the sensitivity between certain vehicle types, For example, a household whose individuals are environmentally conscious may decide to reside in neo-urbanist neighborhoods and also purchase coupe or compact sedans as a way of contributing less to environmental pollution). </p><p>3. DATA The data for this study is drawn from the 2000 San Francisco Bay Area Household Travel Survey (BATS) designed and administered by MORPACE International Inc. for the Bay Area Metropolitan Transportation Commission (MTC). There are three dependent variables in this study. The first dependent variable is that of residential location neighborhood type. A binary dependent variable, neo-urbanist or conventional, was constructed to characterize the traffic analysis zone (TAZ) of the household residence using a factor analysis and clustering technique. Pinjari et al. (9) provide complete details on the development of the neighborhood characterization. In brief, two principal components were identified through the factor analysis – one describing residential density and the transportation/land use environment and the second describing accessibility to activity center. The factors loading on the first component included bicycle lane density, number of zones accessible from the home zone by bicycle, street block density, household population density, and fraction of residential land use in the zone. The factors loading on the second component included bicycle lane density and number of physically active and natural recreation centers in the zone. A cluster analysis of the traffic analysis zones based on these two principal components or dimensions helped characterize all zones as either neo-urbanist or conventional. The second dimension of the discrete dependent variable is the vehicle type. The vehicle types of the vehicles in the dataset were classified into six categories: (1) Coupe, (2) Sports utility vehicle (SUV), (3) Pickup truck, (4) Vans (including minivans), (5) compact sedans (including subcompact sedans) and (6) large sedans (including mid-size sedans and station wagons). In addition to these six alternatives, there exists the “no vehicle” alternative. Eluru, Bhat, Pendyala, Konduri 9</p><p>The third dependent variable is the logarithm of annual vehicle miles traveled (for each vehicle). This is the continuous choice dimension of interest in this study. Annual vehicle mileage was computed for each vehicle using the odometer readings recorded at the end of the diary period, reported mileage at the time of vehicle possession, the survey year, and the year of possession. The annual vehicle mileage is then: Mileage recorded at end of survey - Miles on possession Annual Mileage (12) Survey year Year of possession Only those households with four or fewer vehicles and that provided complete information on all vehicles in the household were included in the final data set used for model estimation. This yielded a final sample of 5,082 households. Of these households, 68.5% reside in conventional neighborhoods; these households report average annual mileage on vehicles equal to 12,023 miles, which is about 600 miles more than that reported by the households in neo-urbanist zones. Table 1 offers a summary of the characteristics of the sample used in this study. For both types of neighborhoods, it is found that SUV’s are used more than other vehicle types as indicated by the higher vehicle mileage. In both neighborhood types, it is found that sedans account for a larger share of vehicle types than other vehicle types. Other salient characteristics of the sample are that about one-half of the households own only one vehicle, about 40 percent of the households are single-person households, nearly two-thirds own their residence, about one-third have two or more workers, and about three-quarters have no children.</p><p>4. EMPIRICAL ANALYSIS This section presents a detailed discussion on the model estimation results. Models were estimated using a host of explanatory variables including household demographics, land use or built environment variables, and a variety of transportation network and accessibility measures. Bhat and Guo (7) provide a detailed description of the nature and definition of the various land use and transportation network/accessibility measures used in the model specification. Joint nested logit-regression models of residential location choice, vehicle type choice, and vehicle mileage were estimated using the copula-based framework. The approach accommodates correlations across alternatives and potential self-selection effects on vehicle usage (via the correlation between vqij and hqij ). In the model estimation effort, it was found that the correlation across alternatives was statistically insignificant. As a result, GEV-based logit model collapses into a simpler MNL-regression copula structure. The empirical analysis involved estimating models with six different copula structures (Gaussian, FGM, Frank, Clayton, Gumbel, and Joe) for specifying the dependency between the vqij and hqij terms (4). Finally, an independent model that ignores the possible dependency between the discrete and continuous choice dimensions was also estimated. The maximum-likelihood estimation of the models with different copulas leads to a case of non-nested models. The most widely used approach to select among competing non-nested copula models is the Bayesian Information Criterion (BIC) [see Genius and Strazzera (29), Trivedi and Zimmer (30)]. The BIC for a given copula model is equal to 2ln(L) K ln(Q) , where ln(L) is the log-likelihood value at convergence, K is the number of parameters, and Q is the number of observations. The copula that results in the lowest BIC value is the preferred copula. However, if all of the competing models have the same exogenous variables and the same number of thresholds, as is the case here, the BIC information selection procedure measure Eluru, Bhat, Pendyala, Konduri 10 is equivalent to selection based on the largest value of the log-likelihood function at convergence. Among the copula models, it was found that the Frank copula model provides the best data fit with a likelihood value of –37291.3. The corresponding likelihood value for the independent copula model is –38607.1, clearly rejecting the hypothesis of independence between the combined residential location - vehicle type combination choice and vehicle usage equations in favor of the model structure that accommodates correlations between the vqij and hqij terms. The joint model in which the dependency parameters were specified to be Gaussian (i.e., equivalent to Lee’s model) yielded a log-likelihood value very close to the independent model log-likelihood. This result clearly underlines the importance of accommodating dependencies using flexible copula structures. In the interest of brevity, only estimation results for the Frank copula model are presented in Table 2. The parameters (and the t-statistics in parenthesis beneath the parameters) are presented for the discrete component for the fourteen residential location- vehicle type choice combinations and twelve continuous components separately.3</p><p>4.1 Model Estimation Results This section is devoted to describing the findings reported in Table 2. The constant terms clearly indicate an overall preference to reside in conventional neighborhoods (as indicated by the higher coefficient for all vehicle types in the conventional neighborhood compared to their counterparts in the neo-urbanist neighborhood). Among the vehicle acquisition choices, the highest propensity is associated with acquiring “no vehicle” suggesting that, for most households, the number of vehicles is less than the number of adults plus 1 implying that every household is likely to have at least one “no vehicle” purchase decision. Among the vehicle types themselves, there is a greater propensity to acquire sedans in comparison to other vehicle types. Vans are least likely to be the vehicle type of choice as evidenced by the high negative constant relative to other vehicle types. A host of household demographics impact joint residential location-vehicle count and type choice. Larger households are likely to acquire larger vehicles (large sedan, van, pickup truck) or choose not to acquire a vehicle. Households with children also exhibit preference for larger vehicles. Further, a comparison of coefficients across neighborhood types indicates that households with children are likely to prefer living in conventional neighborhoods, a finding earlier reported also by Bhat and Eluru (4). A higher number of workers is associated with an inclination to acquire pickup truck while higher number of females is associated with a disinclination to acquire a pickup, suggesting gender related differences in vehicle type choice. Those who own a household tend to reside in conventional neighborhoods as opposed to neo-urbanist neighborhoods. Further, they are less likely to opt for a “no vehicle” option indicating a tendency to acquire as many vehicles as the number of adults + 1. This holds true for higher income households who show an inclination to acquire SUV and coupe type vehicles as opposed to other vehicle types, a finding consistent with earlier research [see Choo and Mokhtarian (10)]. Built environment variables significantly impact vehicle type choice. In general, as land use density and land use mix increase, the likelihood of acquiring pickup trucks decreases. This finding is consistent with expectations as one would expect households in such environments to shun the larger pickup truck type vehicles [also see Choo and Mokhtarian (10) for a similar result]. Further, with the increase in density of non-motorized transport facilities, the likelihood of choosing neo-urbanist neighborhoods and not acquiring a vehicle increases. Transportation 3 The “no vehicle” alternative would not have any associated mileage component. Eluru, Bhat, Pendyala, Konduri 11 accessibility measures also impact joint neighborhood-vehicle type choices. As walk access time to a transit stop increases, the likelihood of owning vehicles of various types increases while enhanced bicycle accessibility reduces the likelihood of owning a pickup truck. Enhanced transit accessibility is associated with acquiring compact and large sedans as opposed to larger pickup trucks, SUVs, or vans. One of the virtues of the model specification and form adopted in this study is that it allows one to capture household fleet dynamics [see Mannering and Winston (13) for a discussion on the importance of household fleet dynamics on vehicle type choice]. By considering each vehicle type choice as a choice occasion, and ordering the choices in a chronological manner, one can model the choice of acquiring a vehicle type as a function of the previously held vehicle types in the household. An examination of the last set of variables in Table 2 shows that there is considerable household fleet dynamics and history dependency in vehicle type choice. If a household already owns a coupe, then the likelihood that the household will choose a different vehicle (than a coupe) increases across the vehicle types with the SUV vehicle type indicating the highest positive coefficient. Parameters along the diagonal are negative, suggesting that households are less likely to repeat the same vehicle type choice; instead, households are likely to acquire a mix of vehicle types suitable to different types of trips. The presence of a car (of any type) or SUV in the vehicle fleet increases the likelihood of not acquiring a vehicle. The presence of a van in the household reduces the likelihood that the household will acquire another large vehicle (SUV or pickup truck). To accommodate the influence of vehicle make/model for each vehicle type, a logsum variable was computed from the multinomial logit (MNL) model results presented in Bhat et al. (19). This logsum variable contains information on the vehicle attributes, fuel price, and household characteristics (i.e., household size and income) that affected the choice of vehicle make/model within each vehicle type category, i.e., the logsum variable is employed to capture the utility derived from the different make/model combinations within each vehicle type. In the context of residential location-vehicle type choice, the logsum parameter was not found to be statistically different from one, and is therefore set to one, indicating independence among the utilities of make/model alternatives within each vehicle body type category in vehicle make/model decisions. The regression component of the model presented in Table 2 shows how various factors influence vehicle usage for the various residential zone-vehicle type choice combinations. The constants indicate that mileage in conventional neighborhoods is higher than that in neo-urbanist neighborhoods for all vehicle types clearly suggesting that there is a neighborhood effect even when controlling for all other factors. The presence of children contributes to higher levels of mileage across virtually all vehicle types, except vans, a somewhat surprising finding given that vans are often the “family” vehicle. However, the coefficients associated with van mileage are not statistically significant. The presence of employed individuals contributes to higher vehicle mileage for all vehicle types (except vans). The finding is consistent with intuitive expectations because employed individuals travel regularly to their work place accruing significant mileage on the vehicle. The presence of senior adults, on the other hand has an opposite effect on vehicle mileage. Senior adults are less likely to be mobile, thus accruing less miles on their vehicles. Higher income levels are generally associated with higher levels of mileage, except for pickup trucks, which may be driven more by lower income blue-collar workers (see 20, 25). Virtually all built environment measures indicative of density and land use mix contribute negatively to vehicle usage [similar to the findings of Brownstone and Golob (25)]. Similarly, Eluru, Bhat, Pendyala, Konduri 12 non-motorized transportation accessibility also contributes negatively to vehicle usage. As such, there is a clear finding that land use and built environment does impact vehicular travel demand, even after accounting for residential self-selection effects. Cao et al. (31), in their review of research on the influence of residential self-selection on travel behavior, find that most research efforts lend credence to our finding. The strong presence of unobserved factors is amply demonstrated by the highly statistically significant scale parameters that represent the variance of the error term in the continuous model component. </p><p>4.2 Model Assessment This section presents a discussion of the model findings focusing on the simultaneity among choice processes to better understand the nature of the dependency among residential location- vehicle type choices and vehicle usage. This section also presents an application of the model to demonstrate its ability to replicate multi-dimensional choice processes. In the last row of Table 2, it can be found that all dependency parameters are significantly different from zero, lending strong credence to the belief that there is substantial self-selection in the residential location-vehicle count by type-vehicle usage choice processes. The significant dependency parameters suggest that there are non-ignorable unobserved factors that affect both residential location-vehicle ownership and type combination choice and vehicle miles of travel for each type of vehicle. In the interest of parsimony in specification, the parameters are constrained to be equal across the residential location neighborhood types. The dependency parameters can be converted into a measure similar to a correlation coefficient that takes on a value between –1 and 1. This measure is called the Kendall’s and it is essentially a transformation of the copula dependency parameters such that the value is constrained to a range of –1 to 1. It is computed as the probability of concordance minus the probability of 4轾 1 q t t =1 - 1 - dt discordance. For the Frank copula, 犏 t and –1 < < 1 [for details, see q臌 q t=0 e -1 (4)]. The Kendall’s measures of dependency by vehicle type are: Coupe: –0.52 SUV: –0.56 Pickup truck: –0.55 Van: –0.61 Compact sedan: –0.59 Large sedan: –0.58 All of these values indicate that there is substantial dependency among the choice dimensions due to common unobserved factors. To interpret these dependency parameters R= 1 if b' x > 0, further, note that Equation (3) can be rewritten as: qij i qij - vqij and R= 0 if b' x < 0. v qij i qij - vqij The error term qij enters with a negative sign in the equation.</p><p>Therefore a negative correlation (or dependency) between this error term and the error term hqij in the vehicle usage equation implies that unobserved factors that increase (decrease) the propensity to choose a residential location-vehicle type i also increase (decrease) the usage of that vehicle type. Similarly, a positive correlation between the vqij and the hqij terms implies that unobserved factors that increase (decrease) the propensity to choose a residential location- Eluru, Bhat, Pendyala, Konduri 13 vehicle type i also decrease (increase) the usage of that vehicle type. Based on intuitive consideration, one can expect the estimated dependency parameters between the vqij and the hqij terms to be negative, implying that the dependency between vehicle type choice and usage is positive. In this study, it is indeed found that the dependency parameters are negative suggesting that unobserved factors that make a household more (less) inclined to acquire a certain vehicle type also make the household more (less) inclined to use that vehicle more (i.e., accumulate more miles). As mentioned earlier, it is important to note that the model using Gaussian copula fails to capture these correlations and suggests that there is no self-selection bias. To further emphasize the importance of this finding, in a recent effort to examine the influence of self-selection on vehicle usage, Brownstone and Golob (25) estimate a joint model of residential density and vehicle usage assuming a Gaussian error dependency structure. They conclude from their model results that there are no statistically significant self-selection impacts on vehicle usage. However, the results from the Frank copula clearly suggest the presence of dependency underscoring the importance of using flexible copula structures in modeling self-selection impacts.</p><p>4.3 Model Application To demonstrate the applicability of the model system developed in the paper, the model results presented in Table 2 are employed to predict changes in residential location, vehicle type shares, and vehicle usage. In this exercise, changes in the choice dimensions are examined as a function of changes in exogenous factors. In particular, the impacts of changes in household demographics, built environment variables, and local transportation measures are examined in this simple simulation exercise. The specific changes in exogenous factors considered are increasing household size, number of employed individuals, and number of females by unity, increasing land use mix value of the neighborhood by 25 percent, and increasing the number of zones accessible by bicycle within a six mile radius by 50 percent. The results of the application exercise are presented in Table 3. The table shows changes in shares and usage by residential location and vehicle type for the Frank copula model and the model of independence that sets all dependency parameters to zero. In general, it is found that the sensitivity (changes in shares and usage) provided by the Frank copula model differs from that of the model of independence. While some differences are small, there are some that are quite substantial (e.g., effect of household size increase on usage of van), suggesting that ignoring dependency among choice dimensions could result in serious over- or under-estimation of impacts of changes in exogenous variables. Larger household sizes and an increase in number of employed individuals result in a shift towards conventional neighborhoods, although an increase in the number of females results in a shift towards neo- urbanist neighborhoods. Similarly, improvements in land use density and bicycle accessibility result in shifts towards neo-urbanist neighborhoods. An increase in the number of employed individuals increases the share of pickup trucks significantly, while resulting in a decrease across all other vehicle types, including the choice of no vehicle purchase. On the other hand, an increase in the number of females reduces the share of all vehicle types, except that for compact and large sedans whose shares increase. Increases in household sizes are met with a higher likelihood of not making a vehicle purchase suggesting that households reach a vehicle ownership saturation point beyond which they do not acquire additional vehicles even when an additional person enters the household. Built environment and transportation accessibility are associated with a decreased share of pickup trucks and slight increases in shares of all other Eluru, Bhat, Pendyala, Konduri 14 vehicle types, including the choice of not acquiring a vehicle. In fact, an increase in the number of zones accessible by bicycle results in reduction of all vehicle types leading to an increase in the share of the “no vehicle” alternative. It is interesting to note that the Frank copula model shows consistently different levels of sensitivity in vehicle usage compared to the model of independence. It appears that ignoring unobserved dependency among choice dimensions (self- selection) may result in biased estimation of the potential impacts (benefits) of enhanced non- motorized transport accessibility. </p><p>5. CONCLUSIONS There has been substantial interest in the transportation literature on examining the influence of residential neighborhood choice on vehicle count by type and vehicle usage. These choice phenomena are of much interest to the profession given the recent attention being paid to global warming, public health, sustainable development and mobility patterns, and energy independence. The current research proposes a simple, yet effective methodological approach that focuses on incorporating the impact of “self-selection” of individuals in residential location- vehicle ownership and type choice and its influence thereof on vehicle usage. In this paper, a simultaneous model of residential location choice, vehicle count and type choice, and vehicle usage is presented with a view to capture the potential effects of the presence of common unobserved factors that may jointly impact these choice dimensions. The research effort employs the structure of the copula-based joint GEV-based logit – regression modeling framework to jointly model the choice dimensions. Multiple vehicle ownership and usage dimensions are accommodated by assuming that the current vehicle fleet and its usage are determined through a series of unobserved (to the analyst) repeated discrete-continuous choice occasions. The number of choice occasions is linked to the number of adults in the household. At each choice occasion the household is faced with a choice of acquiring different vehicle types or “acquiring no vehicle”. The estimation of such complex multi-dimensional discrete-continuous model systems that accommodate error correlations or dependencies has proven to be a challenge, both from an analytical and a computational burden perspective. In this paper, a GEV based logit – regression copula-based modeling approach that offers a closed form solution to the evaluation of the likelihood function is employed to overcome the computational and analytical challenges associated with estimating such model systems. In the current study, six different copulas are tested from the rich set of copulas generated in literature including (1) Gaussian copula, (2) Farlie-Gumbel-Morgenstern (FGM) copula, (3) Clayton, (4) Gumbel, (5) Frank, and (6) Joe copulas for the simultaneous model of residential location choice, vehicle ownership and type choice, and vehicle usage. The paper offers an advanced methodology that can be used to specify, estimate, and apply travel models that simultaneously represent multiple choice dimensions. The findings in the paper confirm that there are significant common unobserved factors that simultaneously impact residential location choice, vehicle type choice, and vehicle usage. The Frank copula models offers a substantially superior data fit compared to the model that ignores the presence of self-selection impacts. Notably, the Gaussian copula estimation results are not statistically superior to the independent model results. A conventional joint modeling of these choices (assuming normal correlated errors across choice dimensions) would have one conclude that self-selection impacts are negligible in affecting vehicle usage. However, the Frank copula model results support the notion that there are significant self-selection effects in residential location choice and vehicle type choice and usage. People choose neighborhoods and Eluru, Bhat, Pendyala, Konduri 15 vehicles that support their lifestyle preferences and attitudes and values. Estimation results from the Frank copula model were employed to undertake a simulation exercise by varying different exogenous variables. The simulation exercise clearly highlights the importance of household demographics and built environment variables on residential location, vehicle ownership by type, and vehicle usage choices. Further, the model system presented in this paper offers the ability to not only model vehicle fleet composition or holdings, but also the vehicle acquisition process itself as a function of previously held vehicles in the household. This model provides an effective solution to obtain a complete and accurate picture of the land use-vehicle fleet-vehicle use choices of a household while controlling for self-selection effects in these choice processes. Future efforts should consider analyzing number of travel tours and trips by purpose, and mileage of individual trips, rather than considering vehicle miles of travel in the aggregate. Further, measures of transit accessibility should also be considered in the analysis.</p><p>ACKNOWLEDGEMENTS The authors acknowledge the helpful comments of three anonymous reviewers on an earlier version of the paper. The authors would like to thank Sudeshna Sen, Erika Spissu, and Abdul Pinjari for their help in assembling the data set used in this study. The authors are also grateful to Lisa Macias for her help in formatting this document. Eluru, Bhat, Pendyala, Konduri 16</p><p>REFERENCES</p><p>1. Ewing, R., and R. Cervero. Travel and the Built Environment – Synthesis. In Transportation Research Record: Journal of the Transportation Research Board, No. 1780, Transportation Research Board of the National Academies, Washington, D.C., 2001, pp. 87-114. 2. Lund, H. Testing the Claims of New Urbanism. Journal of the American Planning Association, Vol. 69,No. 4, 2003, pp. 414-429. 3. Song, Y., and G. J. Knaap. New Urbanism and Housing Values: A Disaggregate Assessment. Journal of Urban Economics, Vol. 54, 2003, pp. 218-238. 4. Bhat, C. R., and N. Eluru. A Copula-Based Approach to Accommodate Residential Self- Selection Effects in Travel Behavior Modeling. Transportation Research Part B, Vol. 43, No. 7, 2009, pp. 749-765. 5. Pinjari, A. R., R. M. Pendyala, C. R. Bhat, and P. A. Waddell. Modeling the Choice Continuum: An integrated model of residential location, auto ownership, bicycle ownership, and commute tour mode choice decisions. Technical paper, Department of Civil, Architectural & Environmental Engineering, The University of Texas at Austin, 2007. 6. Chen, C., H. Gong, and R. Paaswell. Role of the Built Environment on Mode Choice Decisions: Additional Evidence on the Impact of Density. Transportation, Vol. 35, 2008, pp. 285-299. 7. Bhat, C. R., and J. Y. Guo. A Comprehensive Analysis of Built Environment Characteristics on Household Residential Choice and Auto Ownership Levels. Transportation Research Part B, Vol. 41, No. 5, 2007, pp. 506-526. 8. Pinjari, A. R., C. R. Bhat, and D. A. Hensher. Residential Self-Selection Effects in an Activity Time-Use Behavior Model. Transportation Research Part B, Vol. 43, No. 7, 2009, pp. 729-748. 9. Pinjari, A. R., N. Eluru, C. R. Bhat, R. M. Pendyala, and E. Spissu. Joint Model of Choice of Residential Neighborhood and Bicycle Ownership: Accounting for Self-Selection and Unobserved Heterogeneity. In Transportation Research Record: Journal of the Transportation Research Board, No. 2082, Transportation Research Board of the National Academies, Washington, D.C., 2008, pp. 17-26. 10. Choo, S., and P. L. Mokhtarian. What Type of Vehicle do People Drive? The Role of Attitude and Lifestyle in Influencing Vehicle Type Choice. Transportation Research Part A, Vo. 38, No. 3, 2004, pp. 201-222. 11. Cao, X., P. L. Mokhtarian, and S. L. Handy. Neighborhood Design and Vehicle Type Choice: Evidence from Northern California. Transportation Research Part D, Vol. 13, No. 2, 2006, pp. 133-145. 12. Handy, S. Critical Assessment of the Literature on the Relationships among Transportation, Land Use, and Physical Activity. Prepared for the Transportation Research Board and Institute of Medicine Committee on Physical Activity, Health, Transportation, and Land Use. Washington, D.C., January 2005. Available at http://trb.org/downloads/sr282papers/sr282Handy.pdf. 13. Mannering, F., and C. Winston. A Dynamic Empirical Analysis of Household Vehicle Ownership and Utilization. Rand Journal of Economics, Vol. 16, No. 2, 1985, pp. 215-236. 14. Feng Y., D. Fullerton, and L. Gan. Vehicle Choices, Miles Driven and Pollution Policies. NBER Working Paper Series, Working Paper 11553, National Bureau of Economic Research, Washington, D.C., 2005. Eluru, Bhat, Pendyala, Konduri 17</p><p>15. Goldberg, P. The Effects of the Corporate Average Fuel Efficiency Standards in the US. Journal of Industrial Economics, Vol. 46, No. 1, 1998, pp. 1-33. 16. Mohammadian, A., and E. J. Miller. Empirical Investigation of Household Vehicle Type Choice Decisions. In Transportation Research Record: Journal of the Transportation Research Board, No. 1854, Transportation Research Board of the National Academies, Washington, D.C., 2003, pp. 99-106. 17. Yamamoto, T., R. Kitamura, and S. Kimura. Competing-Risks-Duration Model of Household Vehicle Transactions with Indicators of Changes in Explanatory Variables. In Transportation Research Record: Journal of the Transportation Research Board, No. 1676, Transportation Research Board of the National Academies, Washington, D.C., 1999, pp. 116-123. 18. Shay, E., and A. Khattak. Automobile Ownership and use in Neotraditional and Conventional Neighborhoods. In Transportation Research Record: Journal of the Transportation Research Board, No. 1902, Transportation Research Board of the National Academies, Washington, D.C., 2005, pp. 18-25. 19. Bhat, C. R., S. Sen, and N. Eluru. The Impact of Demographics, Built Environment Attributes, Vehicle Characteristics, and Gasoline Prices on Household Vehicle Holdings and Use. Transportation Research Part B, Vol. 43, No. 1, 2009, pp. 1-18. 20. Spissu, E., A. R. Pinjari, R. M. Pendyala, and C. R. Bhat. A Copula-Based Joint Multinomial Discrete-Continuous Model of Vehicle Type Choice and Miles of Travel. Transportation, Vol. 36, No. 4, 2009, pp. 403-422. 21. West, S. Distributional Effects of Alternative Vehicle Pollution Control Policies. Journal of Public Economics, Vol. 88, 2004, pp. 735-757. 22. Hendel, I. Estimating Multiple-Discrete Choice Models: An Application to Computerization Returns. Review of Economic Studies, Vol. 66, 1999, pp. 423-446. 23. Dube, J. P. Multiple Discreteness and Product Differentiation: Demand for Carbonated Soft Drinks. Marketing Science, Vol. 23, No. 1, 2004, 66-81. 24. Bento, A. M., M.L. Cropper, A.M. Mobarak, K. Vinha. The Impact of Urban Spatial Structure on Travel Demand in the United States. Review of Economics and Statistics, Vol. 87, 2005, pp. 466-478. 25. Brownstone, D., and T. F. Golob. The Impact of Residential Density on Vehicle Usage and Energy Consumption. Journal of Urban Economics, Vol. 65, 2009, pp. 91-98. 26. Lee, L. F. Generalized Econometric-Models with Selectivity. Econometrica, Vol. 51, 1983, pp. 507- 512. 27. McFadden, D. Modelling the Choice of Residential Location. In Spatial Interaction Theory and Planning Models, A. Karquist et al. (eds.), North-Holland Press, Amsterdam, 1978. 28. Sklar, A. Random Variables, Joint Distribution Functions, and Copulas. Kybernetika, Vol. 9, 1973, pp. 449-460. 29. Genius, M., and E. Strazzera. Applying the Copula Approach to Sample Selection Modeling. Applied Economics, Vol. 40, No. 11, 2008, pp. 1443-1455. 30. Trivedi, P. K., and D. M. Zimmer. Copula Modeling: An Introduction for Practitioners. Foundations and Trends in Econometrics, Vol. 1, No. 1, 2007, pp. 1-110. 31. Cao, X., P. L. Mokhtarian, and S. L. Handy. Examining the Impacts of Residential Self- Selection on Travel Behavior: Methodologies and Empirical Findings. Research Report No. CTS 08-24, 2008. http://www.tc.umn.edu/~cao/08-24WEB.pdf >. Eluru, Bhat, Pendyala, Konduri 18</p><p>LIST OF TABLES</p><p>TABLE 1. Sample Characteristics</p><p>TABLE 2. Frank Copula Model Results: MNL component Frank Copula Model Results: Regression component</p><p>TABLE 3. Model Application Eluru, Bhat, Pendyala, Konduri 19</p><p>TABLE 1 Sample Characteristics</p><p>Dependent variable Conventional neighborhood Neo-urbanist neighborhood Vehicle type Sample share (%) Annual mileage Sample share (%) Annual mileage Coupe 7.8 10319 3.1 9926 SUV 7.6 13555 3.0 12901 Pickup truck 9.6 12005 3.2 11512 Vans 6.2 13252 2.0 12200 Compact sedan 15.4 12257 8.0 11350 Large sedan 24.4 11637 9.6 11369 Overall by neighborhood 71.1 12023 28.9 11439 Number of vehicles 1 51.8 2 40.9 3 or more 7.3 Household size 1 39.2 2 36.1 3 9.6 4 11.1 5 or more 4.0 Household tenure Own 66.6 Rent 33.4 Number of Employed individuals 0 18.9 1 48.1 2 or more 33.0 Number of children 0 75.7 1 9.5 2 or more 14.8 Sample size 5082 Eluru, Bhat, Pendyala, Konduri 20 TABLE 2 Frank Copula Model Results: MNL component MNL (Dependent variable = Combined Residential location, vehicle count and type) Variable Conventional neighborhood Neo-urbanist neighborhood Pickup Comp Large No Pickup Comp Large No Coupe SUV Van Coupe SUV Van Truck Sedan Sedan vehicle Truck Sedan Sedan vehicle -0.444 -1.302 -2.878 0.358 0.389 3.145 -0.639 -0.968 -2.041 -3.455 0.036 -0.143 2.476 Constant - (-3.92) (-6.43) (-12.73) (3.61) (2.82) (17.01) (-6.97) (-7.47) (-9.66) (-15.43) (0.32) (-0.98) (11.69) Household demographics 0.377 0.765 -0.192 0.205 0.259 0.377 0.765 -0.192 0.205 0.327 Household size - - - - (5.91) (14.92) (-3.26) (4.24) (4.93) (5.91) (14.92) (-3.26) (4.24) (5.14) No. of children 0.504 0.372 -0.157 0.348 -0.157 -0.157 0.215 -0.157 -0.157 #children ≤ 4 yrs ------(5.95) (4.66) (-4.82) (3.83) (-4.82) (-4.82) (2.50) (-4.82) (-4.82) 0.417 0.238 -0.154 0.263 -0.154 0.084 -0.154 -0.154 -0.154 #children 5 - 10 yrs - - - - (5.00) (3.07) (-5.15) (2.97) (-5.15) (1.01) (-5.15) (-5.15) (-5.15) 0.493 0.353 0.201 -0.162 0.331 -0.162 0.191 0.039 -0.162 -0.162 #children 11 - 15 yrs - - - - (4.93) (4.22) (2.04) (-4.38) (3.11) (-4.38) (2.10) (0.37) (-4.38) (-4.38) 0.355 0.237 -0.113 0.355 0.237 -0.113 #employed individuals ------(7.18) (4.98) (-2.93) (7.18) (4.98) (-2.93) -0.280 0.367 0.155 0.153 -0.280 0.367 0.155 0.166 #females ------(-3.55) (5.82) (2.71) (2.33) (-3.55) (5.82) (2.71) (2.09)</p><p>Annual household income</p><p>0.180 0.180 35K-90K ------(1.89) (1.89) -0.859 -0.339 -0.405 -0.344 -0.570 -0.859 -0.339 -0.405 -0.344 -0.536 >90K - - - - (-7.69) (-2.93) (-4.41) (-3.99) (-5.98) (-7.69) (-2.93) (-4.41) (-3.99) (-5.02)</p><p>Household tenure</p><p>0.346 0.534 0.792 0.326 -0.189 -0.446 -0.100 0.087 0.346 -0.446 -0.121 -0.446 Own household - (3.74) (6.05) (6.77) (5.17) (-2.27) (-10.00) (-0.98) (0.88) (2.76) (-10.00) (-1.57) (-10.00)</p><p>Built environment variables</p><p>-0.001 -0.001 Employment density ------(-1.16) (-1.16) -0.345 -0.345 Land use mix (0-1) ------(-2.00) (-2.00) -0.012 -0.104 -0.012 0.149 Density of bicycle lanes ------(-1.13) (-7.34) (-1.13) (10.90) Local transportation measures Eluru, Bhat, Pendyala, Konduri 21 MNL (Dependent variable = Combined Residential location, vehicle count and type) Variable Conventional neighborhood Neo-urbanist neighborhood Pickup Comp Large No Pickup Comp Large No Coupe SUV Van Coupe SUV Van Truck Sedan Sedan vehicle Truck Sedan Sedan vehicle Walk access time to in-zone 0.030 0.038 0.020 0.032 0.020 -0.036 ------transit stop (4.32) (4.68) (4.03) (5.02) (4.03) (-4.51)</p><p>No. of zones accessible by -0.008(- -0.004 -0.008 0.010 ------bike within 6 miles 4.78) (-2.86) (-4.78) (7.27) No. of zones accessible by ------transit within 30 minutes</p><p>Household fleet dynamics</p><p>1.142 0.466 0.512 0.243 0.501 0.667 1.142 0.466 0.512 0.243 0.501 Presence of coupe - - (5.88) (2.38) (2.09) (1.25) (2.82) (4.21) (5.88) (2.38) (2.09) (1.25) (2.82)</p><p>0.325 0.325 Presence of SUV ------(2.46) (2.46)</p><p>-1.733 -1.733 -0.291 Presence of Pickup truck ------(-7.30) (-7.30) (-1.97)</p><p>-0.474 -0.372 -2.033 -0.474 -0.372 -2.033 Presence of Van ------(-2.27) (-2.13) (-6.20) (-2.27) (-2.13) (-6.20)</p><p>0.329 Presence of compact sedan ------(3.32)</p><p>0.310 Presence of large sedan ------(3.73)</p><p>Log-sum parameter 1.000 1.000 1.000 1.000 1.000 1.000 - 1.000 1.000 1.000 1.000 1.000 1.000 - Eluru, Bhat, Pendyala, Konduri 22</p><p>TABLE 2 Frank Copula Model Results: Regression component Regression (Dependent variable = LnVMT) Conventional neighborhood Neo Neighborhood Pickup Com- Large Pickup Com- Large Coupe SUV Van Coupe SUV Van Truck Sedan Sedan Truck Sedan Sedan 7.562 8.246 7.897 7.692 8.077 8.041 7.459 8.142 7.798 7.578 7.985 7.893 Constant (53.24) (80.57) (54.67) (58.89) (128.87) (160.66) (46.54) (79.16) (46.13) (47.09) (104.56) (131.50) Household demographics -0.047 -0.073 -0.205 0.152 -0.059 -0.047 -0.073 -0.205 0.152 -0.059 Household size - - (-1.50) (-1.70) (-3.16) (4.64) (-1.67) (-1.50) (-1.70) (-3.16) (4.64) (-1.67) No. of children in the household 0.188 0.301 -0.103 0.091 0.037 0.188 0.301 -0.103 0.091 0.037 #children ≤ 4 yrs - - (2.69) (2.63) (-1.66) (1.55) (1.93) (2.69) (2.63) (-1.66) (1.55) (1.93) 0.144 0.246 -0.077 0.070 0.037 0.144 0.246 -0.077 0.070 0.037 #children 5-10 yrs - - (2.39) (2.90) (-1.36) (1.42) (1.93) (2.39) (2.90) (-1.36) (1.42) (1.93) 0.144 0.246 0.070 0.037 0.144 0.246 0.070 0.037 #children 11-15 yrs - - - - (2.39) (2.90) (1.42) (1.93) (2.39) (2.90) (1.42) (1.93) 0.090 0.068 0.234 0.158 0.093 0.090 0.068 0.234 0.158 0.093 #employed individuals - - (1.80) (1.70) (3.75) (4.42) (4.67) (1.80) (1.70) (3.75) (4.42) (4.67) -0.154 -0.145 -0.190 -0.212 -0.084 -0.154 -0.145 -0.190 -0.212 -0.084 #senior adults - (-2.01) (-2.03) (-3.16) (-5.53) (-3.55) (-2.01) (-2.03) (-3.16) (-5.53) (-3.55) Annual hhld income 0.330 0.228 0.179 0.330 0.228 0.179 35K-90K ------(3.27) (2.31) (4.49) (3.27) (2.31) (4.49) 0.348 0.126 -0.095 0.306 0.179 0.348 0.126 -0.095 0.306 0.179 >90K - - (3.08) (2.23) (-1.17) (2.69) (4.49) (3.08) (2.23) (-1.17) (2.69) (4.49) Household tenure -0.105 -0.023 -0.105 -0.023 Own household ------(-1.22) (-0.71) (-1.22) (-0.71) Built environment variables -0.002 -0.002 Population density ------(-1.39) (-1.39) 0.001 0.001 Employment density ------(0.90) (0.90) -0.012 -0.021 -0.012 -0.011 -0.012 -0.021 -0.012 -0.011 Density of bicycle lanes - - (-1.15) (-1.58) (-1.64) (-1.79) (-1.15) (-1.58) (-1.64) (-1.79) Presence of 4+ physical -0.135 -0.135 ------activity centers (-1.26) (-1.26) Local transportation measures No. of zones accessible -0.002 -0.003 -0.004 -0.001 -0.002 -0.002 -0.003 -0.004 -0.001 -0.002 - - by bike within 6 miles (-1.47) (-2.09) (-3.27) (-1.87) (-4.27) (-1.47) (-2.09) (-3.27) (-1.87) (-4.27) 1.122 0.926 1.303 0.989 1.007 0.953 1.122 0.926 1.303 0.989 1.007 0.953 Scale parameter (76.99) (89.57) (60.93) (87.89) (142.97) (157.86) (76.99) (89.57) (60.93) (87.89) (142.97) (157.86) Copula dependency -6.034 -6.999 -6.723 -8.085 -7.780 -7.365 -6.034 -6.999 -6.723 -8.085 -7.780 -7.365 parameter (θ) (-14.27) (-15.20) (-13.40) (-12.07) (-21.69) (-27.24) (-14.27) (-15.20) (-13.40) (-12.07) (-21.69) (-27.24) Eluru, Bhat, Pendyala, Konduri 23</p><p>TABLE 3 Model Application</p><p>Variables No. of employed individuals Zonal land use mix increased by No. of zones accessible by bicycle Household size increased by 1 No. of females increased by 1 increased by 1 25% within 6 miles Frank Independent Frank Independent Frank Independent Frank Independent Frank Independent Sample Shares Usage Shares Usage Shares Usage Shares Usage Shares Usage Shares Usage Shares Usage Shares Usage Shares Usage Shares Usage Residential neighborhood</p><p>Conventional -0.69 -3.35 -0.35 -6.05 0.02 11.53 0.03 12.86 -0.50 0.00 -0.77 0.00 -0.01 0.00 -0.01 0.00 -3.71 -3.00 -3.50 -2.99</p><p>Neo-urbanist 1.55 -3.35 0.79 -6.05 -0.03 11.53 -0.07 12.86 1.12 0.00 1.73 0.00 0.03 0.00 0.03 0.00 8.30 -3.00 7.84 -2.99</p><p>Vehicle Type</p><p>Coupe -18.39 -4.62 -22.02 -7.10 -4.54 9.44 -4.78 12.56 -13.00 0.00 -11.94 0.00 0.22 0.00 0.24 0.00 -2.14 -2.57 -2.37 -2.43</p><p>Suv -19.48 -7.04 -23.19 -9.24 -4.47 7.07 -4.71 5.32 -12.42 0.00 -11.31 0.00 0.23 0.00 0.25 0.00 -1.51 0.00 -1.71 0.00</p><p>Pickup 16.85 -18.56 16.27 -20.94 35.20 26.36 37.19 29.60 -33.42 0.00 -33.38 0.00 -2.83 0.00 -3.03 0.00 -9.56 -4.58 -9.27 -3.97</p><p>Van 64.98 16.36 67.89 11.24 -4.21 0.00 -4.45 0.00 -11.15 0.00 -10.04 0.00 0.26 0.00 0.28 0.00 -0.42 -5.82 -0.52 -6.45</p><p>Compact sedan -32.86 -5.74 -31.34 -7.31 20.79 17.06 20.07 15.68 25.39 0.00 25.40 0.00 0.23 0.00 0.24 0.00 -2.01 -2.22 -2.21 -1.29</p><p>Large sedan -0.96 0.00 -0.25 0.00 -14.59 9.74 -14.03 11.46 1.96 0.00 0.84 0.00 0.24 0.00 0.25 0.00 -1.24 -3.38 -1.35 -3.74</p><p>No vehicle 7.07 - 7.24 - -4.37 - -4.63 - 1.83 - 2.01 - 0.22 - 0.24 - 3.67 - 3.85 -</p>
Details
-
File Typepdf
-
Upload Time-
-
Content LanguagesEnglish
-
Upload UserAnonymous/Not logged-in
-
File Pages25 Page
-
File Size-