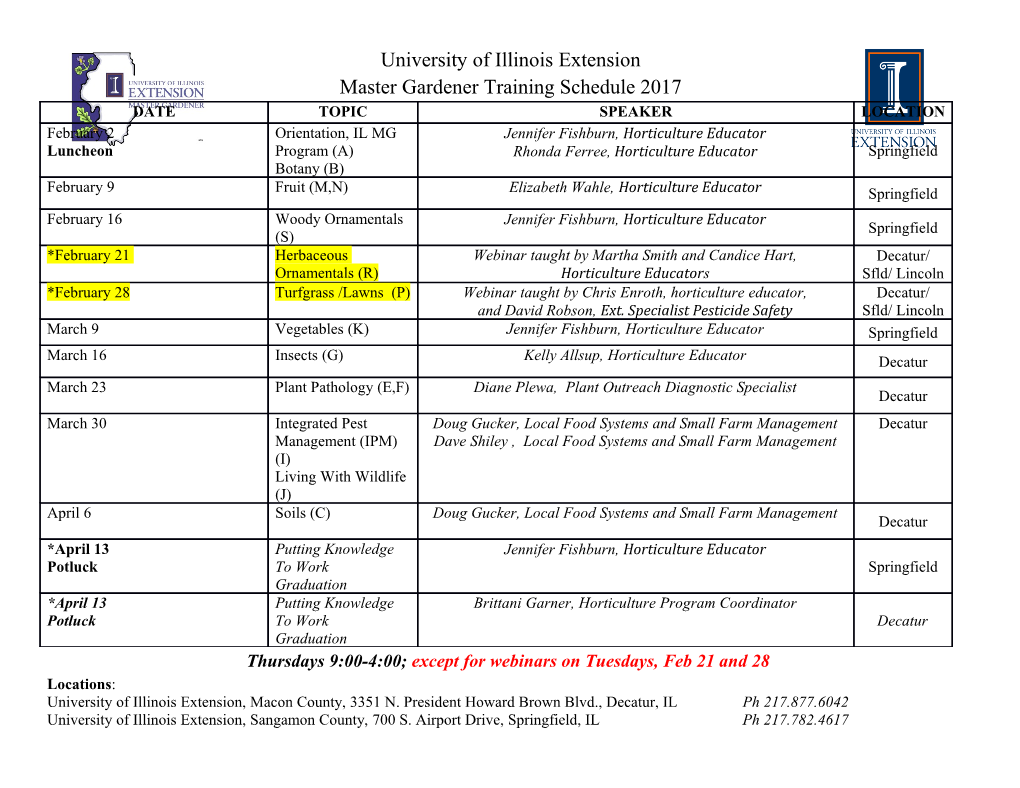
<p> Dansby and Johnston 1</p><p>Predicted Sales for Casa Bonita</p><p>David Dansby and Ryan Johnston</p><p>April 6, 2013</p><p>Empirical Linear Modeling, ECON5645 Dansby and Johnston 2</p><p>Introduction</p><p>The purpose of the following report is to analyze sales determinants for our client, Casa </p><p>Bonita, a Mexican restaurant chain across the nation. Casa Bonita executives have asked us to forecast sales for future restaurants across various parts of the United States that they are considering opening. Determinants of sales from Casa Bonita will be used to build an Ordinary </p><p>Least Squares (OLS) regression model. The model will be used to predict sales for these potential restaurants Casa Bonita executives are thinking about opening. In order for the model to be applicable to Casa Bonita’s decision making process for these potential store openings it must be statistically and logically inspected to make sure it fits sales the best in terms of explaining the changes in revenue. </p><p>Using the model, we can predict annual store revenue, and make recommendations based on these predictions to Casa Bonita executives. Our proposals will include revenue potential measures for the proposed new store locations. If forecasted sales are profitable then it is recommended, based on statistical and logical views, to open the store. On the other hand if predicted revenues have a low profit potential, then it will be recommended to Casa Bonita to avoid opening up that particular store at that location.</p><p>Casa Bonita Background Information</p><p>Casa Bonita is a family friendly Mexican food restaurant chain. They serve a wide range of authentic Mexican dishes including, but not limited to, tacos, enchiladas, and fajitas all at a moderate price. All the stores also offer patio seating for customers to relax with tacos and margaritas. They have a current total of 166 stores across the United States. About 38 percent of the Casa Bonita stores are concentrated in the Western South-Central census region, which Dansby and Johnston 3 includes the states of Texas, Oklahoma, Arkansas, and Louisiana (United States Census Bureau).</p><p>They are usually concentrated in the suburban areas of metropolitan regions in which Casa </p><p>Bonita serves, with 75 percent of all stores located in areas considered to be “suburban”.</p><p>Casa Bonita’s revenue total for their 116 stores was $304,003,913.05 for 2012. Their individual store average was $2,620,723.39. The individual store average of number of total number of seats is about 270. Additionally the sales-per-seat in the restaurant for the chain is about $9,782 in annual revenue per seat.</p><p>Casa Bonita’s first store opened its doors to customers on October 28, 1982. The original store’s size of 8,071 square feet is about 1,000 square feet larger than the Casa Bonita chain average of 7,042 square feet. They opened up their second store about a year later and have been growing steadily since. They started opening five or more stores a year, starting in 1995; sometimes reaching 16 in one year (1997), and slowing down during the Great Recession in which they only opened up one store in each year during 2008, 2009, and 2010. They have not opened a store since 2010. </p><p>As the economy has slowly start to show signs of growing again Casa Bonita executives have begun to consider opening five new stores during this new year. They have have asked us to analyze their large sales determinant data set and build a revenue forecast model to predict sales for five future stores they are questioning about opening. The model will provide insights for us to help Casa Bonita executives make the correct start decision for these potential stores in </p><p>2013.</p><p>Variable Information Dansby and Johnston 4</p><p>The data used in the following analysis and model building comes from 112 Casa Bonita locations across the United States. Casa Bonita’s data set is a cross-sectional data set. This means that the set contains variables from a number of observations over one period of time, during the year 2012. In total, the data set contains 112 observations, although some observations do not include all of the variables and will have to be analyzed for error during pre- modal analysis. Many of the following variables have a positive or negative relationship with sales at Casa Bonita restaurants.</p><p>There are a number of variable groups in the data set that include sales for each Casa </p><p>Bonita stores during 2012, population and household demographics, income and education statistics, employment information, restaurant location information, customer indexes, expenditure totals, and competitor measure variables. Please note that the all of the following variables are understood to have a subscript “i” that represents the variables measure for store </p><p>“i”. </p><p>SALES is the dollar value of total sales for store “i” during the calendar year of 2012. </p><p>SALES will be the dependent variable for our revenue forecast model. The following variables are all possible independent variables in the model. The best variables for the model will be the variables that explain sales the best.</p><p>STORE_ID represents a unique number for store “i” identification. OPEN_DATE indicates the open date of store “i”. SQFT represents the square footage for store “i”, while </p><p>DINING_SQFT is the square footage of the dining area. Also, PATIO_SQFT is the patio square footage for store “i”. BAR_SEATS, DINING_SEATS, and PATIO_SEATS are the number of bar seats, dining seats, and patio seats available at store “i”, respectively. Dansby and Johnston 5</p><p>Most of the following variables are descriptive measures at successive geographic locations. Variables with the name “VARIABLE_NAME_XTO” measure the value of the variable within an X-minute drive from store “i”. X takes on the values of {4, 8, 14}. For example:</p><p>A04V001 = the population, age 0 to 4</p><p>A04V001_8TO = the population, age 0 to 4, located within an 8-minute drive of store “i”.</p><p>A01V001_XTO measures the projected population in 5 years for the area within an X- minute drive of store “i”. A04V001_XTO through A04V0025_XTO are population measures for different age groups; specifically, ages 0 to 4, 5 to 9, 10 to 14, 15 to 17, 18 to 19, 20, 21, 22 to 24, 25 to 29, 30 to 34, 35 to 39, 40 to 44, 45 to 49, 50 to 54, 55 to 59, 60 to 61, 62 to 64, 65, </p><p>66, 67 to 69, 70 to 74, 75 to 70, 80 to 84, and 85 and older, respectively. A06V025_XTO measures the Hispanic population, ages 21 and older, within an X-minute drive of store “i”. </p><p>A12V002_XTO is a population measure for married people, ages 15 and older. </p><p>A12VBASE_XTO measures total population for people 15 and older. A15VV01_XTO is the ratio of males to females, while A16VV01_XTO is the average age of the total population within an X-minute drive of store “i”. A18VV01_XTO is the median age of the total population located within an X-minute drive of store “i”. B01V001_XTO, B01V002_XTO, and B01V003_XTO measure the total number of housing units, number of occupied housing units, and the number of vacant housing units located within an X-minute drive of store “i”, respectively. B03V001_XTO measures the number of families living within an X-minute drive of store “i”. </p><p>B12V001_XTO through B12V008_XTO measure the number of families, number of households with children, number of households without children, number of male-headed households with children, number of male-headed households without children, number of Dansby and Johnston 6 female-headed households with children, and the number of female-headed households without children, respectively. B14V001_XTO, B14V002_XTO, and B14V003_XTO measure the number of mortgaged housing units, the number of housing units owned free and clear, and the number of renter-occupied housing units, respectively. </p><p>B16VBASE_XTO and B18VBASE_XTO represent the total number of owner-occupied housing units and the total number of renter-occupied housing units for store “i”, respectively. </p><p>B26VV01_XTO measures the average, or mean, size of households within an X-minute drive of store “i”. B29VV01_XTO is the median age of household heads. </p><p>BUDS is a variable that takes on a value of 1 to 5 to indicate the degree of denseness of the area that store “i” is located in. 1 represents areas characterized as being “rural”, while 2 represents “in town”, and 3 representing “suburban”. 4 represents if the store is located in area recognized as “metropolitan” and 5 represents “urban” areas. Our analysis team made five dummy variables out of the single BUDS variable. We made the following variables: </p><p>DUMBUDS_RURAL which takes on the value of 1 if the observation is located in a “rural” area and takes on the value of 0 otherwise, DUMBUDS_INTOWN takes on the value of 1 if the observation store is located in area characterized as “in town” and takes on the value of 0 otherwise, DUMBUDS_SUB was created to take on the value of 1 if the store is located in area characterized as “suburban” and takes on the value of 0 otherwise, DUMBUDS_METRO was created to take on the value of 1 if the store is located in area characterized as “metropolitan” and takes on the value of 0 otherwise, and finally DUMBUDS_URBAN takes on values of 1 if the store is in area characterized as “urban” and takes on the value of 0 otherwise. Obviously, each store can only take on a value of 1 for one of the dummy variables used to measure Dansby and Johnston 7 neighborhood location. For example, if one of the stores has a value of 1 for DUMBUDS_SUB then it will have a 0 for the other DUMBUDS dummy variables. </p><p>The Casa Bonita data set also has income measures for households. Variables </p><p>C01V001_XTO through C01V017_XTO measure the number of households with an income in a certain range. These income groups range from less than $10,000 to the range of $250,000 to </p><p>$499,999. For example C01V005_XTO measures the number of households with income in the range of $25,000 to $29,999, while C01V015_XTO measures the number of households with income in the range of $150,000 to $199,999. C01V018_XTO measures the number households with income that is greater than $500,000. Finally, C13VV01_XTO is the per capita income of the total of population living within an X-minute drive of store “i”. </p><p>The next group 35 variables to be described measure the number of people who are 25 years or older who also have the characterized trait of the variable. D02V001_XTO measures the number of males, while D02V18_XTO measures the number of females. D02V002_XTO through D0V017_XTO are educational attainment level measures for the number males ranging from no education to males with a doctorate degree living within an X-minute drive of store “i”. </p><p>Similarly, D02V019_XTO through D02V034 give the same measures of educational attainment levels, but for females. Last, D02VBASE_XTO is the total number of females and males (aged </p><p>25 and older). </p><p>D07V001_XTO, D07V002_XTO, and D07V003_XTO measure the civilian population employed in white collar jobs, blue collar jobs, and service and farm jobs for store “i”, respectively. D07VBASE_XTO is the measure of total employed civilian population within an </p><p>X-minute drive of store “i”. EA04V002_XTO and EA04V003_XTO measure the total male and female populations, respectively, for store “i” (no age-restrictions). EA06V001_XTO, Dansby and Johnston 8</p><p>EA07V001_XTO, EA07V004_XTO, and EA07V010_XTO measure the Hispanic/Latino population, white population, black/African American population, and the Asian population living within an X-minute drive of store “i”, respectively. EB27V001_XTO is the total number of years in residence for all households, while EB28V001_XTO is the total number of vehicles. </p><p>EC14V001_XTO is the average income of households, while EC15V001_XTO is the average family income of households for store “i”. EC18V001_XTO is the median family income of households. ED12V001_XTo measure the average travel time to work for households within an </p><p>X-minute drive of store “i”. Additionally, ED13V001_XTO measures the percentage of the civilian population within an X-minute drive of store “i” that is unemployed. HH_XTO is the total number of households located within an X-minute drive of store “i”, while POP_XTO is the total population (no age restriction). Finally, WMOSTOT_XTO measures the number of people who within an X-minute drive of store “i”.</p><p>The observations in the Casa Bonita data set also includes the alpha variable REGION that takes on various alpha values indicating the United States census region location of store “i”.</p><p>The value WM is for the mountain west region of the U.S., while the value WNC is for stores located in the western north-central region. The values of ENC, WSC, and ESC are indicated for</p><p>“i” if it is located in the eastern north-central region, the western south-central region, or the eastern south-central region, respectively. The value of SA, NE, and MA are indicated for store </p><p>“i” if it is located in the south Atlantic region, the northeast region, or the mid-Atlanta region, respectively. We created eight dummy variables out of the single REGION variable. We created the following dummy variables to describe what region a store was located in: </p><p>DUMREGION_SA was created to take on the value of 1 if the store is located in the U.S. census region of the south Atlantic and takes on the value of 0 otherwise, DUMREGION_WM was Dansby and Johnston 9 created to take on the value of 1 if the store is located in the census region of the mountain west region and takes on the value of 0 otherwise, DUMREGION_WNC was created to take on the value of 1if the store is located in the western north-central census region and takes on the value of 0 otherwise, DUMREGION_ENC was created to take on the value of 1 if the store is located in the eastern north-central region and takes on the value of 0 otherwise, DUMREGION_WSC was created to take on the value of 1 if the store is located in the western south-central region and takes on the value of 0 otherwise, DUMREGION_ESC was created to take on a value of 1 if the store is located in the eastern south-central census region and takes on the value of 0 otherwise, DUMREGION_NE was created to take on the value of 1 if the store is located in the northeast census region and takes on the value of 0 otherwise, and DUMREGION_MA was created to take on the value of 1 is the store is located in the mid-Atlantic census region and takes on the value of 0 otherwise. With these thirteen variables created we now have a total of </p><p>449 variables for the 112 observations. </p><p>Continuing, the following variables are indices, which are numerical scale measurements so that one observation’s index variable measurement can be compared with another observation’s. CUST_VALUE is an index that measures the degree of “value” of the people who live in the area around store “i”. Likewise, LUNCH_CUST_VALUE is an index that measure the degree of “value” of the people who work in the area around store “i”. </p><p>Unfortunately, it is a secret as to how these interesting variables measurements are calculated. </p><p>We also have the other indexes that measure various consumer characteristics. Variables </p><p>SimI_00459_XTO, SimI_00460_XTO, and SimI_00470_XTO are indexes of the likelihood of households to consume Corona, Dos Esquis, Modelo Especial, or Tecate beer who live within an </p><p>X-minute drive of store “i”. SimI_00644_XTO and SimI_01514_XTO are index measures of the Dansby and Johnston 10 number of people who say they consume alcohol and the number of people who say they eat out, respectively. SimI_01873_XTO, SimI_01878_XTO, SimI_01908_XTO, SimI_02005_XTO, </p><p>SimI_03771 are index measures of participation in leisure activities, night club/bar frequenting, movie attendance, theme park attendance, and the number of people who are expected to graduate from high school in the next 12 months, respectively, living within an X-minute drive of store “i”. A very interesting variable is SimI_04005_XTO because it measures the degree of advertising receptiveness of residents living within an X-minute drive of store “i”. This variable should prove to be of more use than only predicting sales (i.e. how much profit portion should be allocated to advertising based on the receptiveness to advertising by the area’s population). </p><p>We also have the following expenditure variables. X01V079_XTO, X01V080_XTO, </p><p>X01V084_XTO, X01V088_XTO, X01V091_XT measure the expenditure on lunch, dinner, catered meals, beer and ale, alcohol besides beer/ale, theatre and amusement parks, and out-of- town trips by consumers, respectively, living within an X-minute drive of store “i”. </p><p>The Casa Bonita data set thankfully also includes variables of differing statistics for competitors of Casa Bonita. The variables labeled as </p><p>CMHO_MEX_MEXCOMPETITORID#_14TO measures the percentage of households within a </p><p>14-minute drive of store “i” that are shared with the Mexican restaurant competitor. </p><p>COMPETITORID# is the competitor’s identification 5-digit number used by Casa Bonita to indicate which competitor the variable is measuring. An example would be for competitor </p><p>Mexican restaurant B which has the identification number 58508. This variable is measured for the Mexican-food restaurants A through L. Similarly, </p><p>CMHO_NONMEX_NONMEXCOMPETITORID#_14TO measures the percentage of households within a 14-minute drive of store “i” that are shared with a non-Mexican competitor Dansby and Johnston 11 and where the COMPETITORID# for non-Mexican competitors is analogous to the identification number for Mexican competitors. This variable is measured for the non-Mexican food restaurants A through U. Also, CM_MEX_MEXCOMPETITORID#_1RO measures the number of Mexican competitor restaurants located within 1 radial mile of store “i” for each </p><p>Mexican competitor A through L. Likewise, </p><p>CM_NONMEX_NONMEXCOMPETITORID#_1RO measures the number of non-Mexican competitor restaurants located within 1 radial mile of store “i” for each non-Mexican competitor </p><p>A through U. </p><p>The data set also includes variables that measure other types of retail stores that could possibly draw customers in (or out). CT_DEP_DEPTSTOREID#_0_5RO measures the number of stores within one-half radial mile of store “i” for each department store 1 through 7. The </p><p>DEPTSTOREID# part of the variable is a 5-digit identification number used by Casa Bonita in identifying the store the variable is measuring. Analogously, we have a variable that measures the number of stores for each grocery store chain 1 through 6 located within a one-half mile radius of store “i”. The variable is labeled as CT_GRO_GROCERYSTOREID#_0_5RO. </p><p>CT_MALL_54435_0_5R and CT_MALL_54443_0_5R measure the number of mall 1 or mall 2 located within a one-half mile radius of store “i”. Concluding, we have variables that measure the number of a certain power center located within a one-half mile radius of store “i”. This variable is measured individually for the power centers 1 through 35. The generic variable appears as CT_POW_POWCENTERID#_0_5RO, where the POWCENTERID# portion is also a</p><p>5-digit identification number used for individual power centers. </p><p>In total the Casa Bonita data set includes 553 variables for 112 observations (Casa</p><p>Bonita restaurants). The reader should review Table 1 below. It shows “candidate” variables Dansby and Johnston 12</p><p>(to be discussed later) definitions. On the left side is the table is the variable name as shown in the data set and on the right side in the same row is the variable’s definition. The table will be of great use since the variable names in the data set are not exactly revealing about what the variable is Dansby and Johnston 13</p><p>Table 1: 49 Candidate Variable Definitions Variable name Variable Definition A01V001_14TO the projected population in 5 years located within a 14-minute drive of store “i” A12VBASE_14TO total population, ages 15 and older, located within a 14-minute drive of store “i” B03V001_14TO number of familes living within a 14-minute drive of store “i” B12V004_14TO number of households without children located within a 14-minute drive of store “i” B14V002_14TO # of housing units owned free and clear located within a 14-minute drive of store “i” B16VBASE_14TO total number of owner-occupied housing units located within a 14-minute drive of store “i” B29VV01_14TO median age of household heads living within a 14-minute drive of store “i” BAR_SEATS the # of seats at the bar in store “i” C01V012_14TO # of households with income $75K - $124,999 CM_MEX_60647_1RO # of competitor Mexican restaurant H located within 1 radial mile of store “i” CM_NONMEX_54598_1RO # of competitor non-Mexican restaurant I located within 1 radial mile of store “i” CMHO_MEX_58508_14TO % of households within a 14-minute drive of store “i” that are shared with competitor Mexican restaurant B CMHO_MEX_58514_14TO % of households within a 14-minute drive of store “i” that are shared with competitor Mexican restaurant C CMHO_MEX_62081_14TO % of households within a 14-minute drive of store “i” that are shared with competitor Mexican restaurant K CMHO_MEX_62094_14TO % of households within a 14-minute drive of store “i” that are shared with competitor Mexican restaurant L CMHO_NONMEX_55727_14TO % of households within a 14-minute drive of store “i” that are shared with competitor non-Mexican restaurant N CT_POW_53889_0_5RO # of power center 4 located within a one-half radial mile of store “i” CT_POW_54432_0_5RO # of power center 13 located within a one-half radial mile of store “i” CT_POW_55095_0_5RO # of power center 20 located within a one-half radial mile of store “i” CT_POW_55491_0_5RO # of power center 26 located within a one-half radial mile of store “i” CT_POW_56128_0_5RO # of power center 31 located within a one-half radial mile of store “i” CUST_VALUE an index that measures the degree of value of the people who live in the area around store “i” D02V001_14TO # of males who are 25 years or older, living within a 14-minute drive of store “i” D02V007_14TO # of males who are 25 years or older, with education up 10th grade, living within a 14-minute drive of store “i” D02V018_14TO # of females who are 25 years or older, living within a 14-minute drive of store “i” D02V024_14TO # of females who are 25 years or older, with education up to 10th grade, living within a 14-minute drive of store “i” D02VBASE_14TO # of males and females who are 25 years or older, living within a 14-minute drive of store “i” D07V002_14TO civilian population (non-military service) employed in blue collar jobs within a 14-minute drive of store “i” D07V003_14TO civilian population (non-military service) employed in service and farm jobs within a 14-minute drive of store “i” D07VBASE_14TO total employed civilian population (non-military service) within a 14-minute drive of store “i” DINING_SEATS the # of dining seats abailable for dining in store “i” DUMREGION_MA dummy (binary) variable that takes on the value of 1 if store “i” is located in the U.S. census region of the mid-Atlantic region DUMREGION_WM dummy (binary) variable that takes on the value of 1 if store “i” is located in the U.S. census region of the mountain west region DUMREGION_WSC dummy (binary) variable that takes on the value of 1 if store “i” is located in the U.S. census region of the western south-central region EA04V002_14TO male population (no age restriction) living within a 14-minute drive of store “i” EA04V003_14TO female population (no age restriction) living within a 14-minute drive of store “i” EA07V010_14TO Asian population living within a 14-minute drive of store “i” EB27V001_14TO total # of years in residence for all households within a 14-minute drive of store “i” LUNCH_CUST_VALUE an index that measures the degree of value of the pople who work in the area around store “i” PATIO_SEATS the # of patio seats at store “i” POP_14TO total population within a 14-minute drive of store “i” SIMI_04005_14TO an index of the degree of receptiveness to advertising of residents who live within a 14-minute drive of store “i” SQFT total square footage of store “i” WMOSTOT_14TO the # of people who work within a 14-minute drive of store “i” X01V079_14TO expenditure on lunch by consumers who live within a 14-minute drive of store “i” X01V080_14TO expenditure on dinner by consumers who live within a 14-minute drive of store “i” X01V084_14TO expenditure on catered meals by consumers who live within a 14-minute of store “i” X01V088_14TO expenditure on beer and ale by consumers who live within a 14-minute drive of store “i” X01V091_14TO expenditure on other alcohol by consumers who live within a 14-minute drive of store “i” measuring. </p><p>Since we have such a large number of variables that seem to be related to sales it would seem that the job of building a parsimonious and cost effective model for predicting annual revenue would be very hard and tedious. In order for the model to be parsimonious we need the simplest model with the fewest possible number of independent variables, all while still explaining sales the greatest. Luckily, we can use the data analysis software program Statistical </p><p>Analysis System (SAS) to perform various statistical techniques to cut the number of variables to Dansby and Johnston 14 a select few of “candidate” variables that would be appropriate and most effective during our building of the final sales forecast model. It should be noted that SAS only provides the user with the results from tedious mathematical calculations in a timely fashion. SAS does not provide the user with interpretations of the data. The data and the statistical measurement results will be intensively inspected by the author and his analysis team in finding the best variables in terms of explaining sales. </p><p>Pre-Model Analysis, Steps</p><p>Before any forecasting models for sales can be created, the data set for Casa Bonita must be analyzed. This calls for a few steps in analyzing the data by looking at a number of statistics for variables. The first step in any pre-model analysis is to clean the data of errors that could give us invalid results in the final model. Next, we must generate various summary statistics for the variables in order to understand them. Finally, we must look at the relationships between our dependent variable, SALES, and all the possible independent, or regressor, variables to highlight any more clues as to which variables will be important for model building. In the end, we will be able to select a small group of “candidate regressors” from such a large number of variables </p><p>(436) for model building and selection.</p><p>The beginning of analysis on any data set meant for building a OLS model must begin with a review of the observations and the variables. Values for the variables must subscribe to the nature of the variable measurement. For example, if we have a variable that measures total population at successive geographic distance, such as a 4-minute, 8-minute, and 14-minute drive from store “i”, then the value for that total population variable at 14 minutes should be greater than or equal to the value at an 8-minute drive. If observations have values for this variable that Dansby and Johnston 15 do not subscribe to the logical increase in value with an increase in geographic distance then the observation must be thrown out because it likely has an incorrect value because of errors during the data collection process. Also, even if that variable is not used for the model, the observation with a value not subscribing to the nature of that variable must still be thrown out because we cannot be sure that the rest of data for that single observation does not have any more errors that we can be easily detected. All observations with erroneous values for some variables will be thrown out during the actual pre-model analysis. After the data has been cleaned, then we can look at the simple statistics that reveal more about the variables and observations.</p><p>The next process in the pre-model analysis is to calculate the summary statistics for all the variables in the data set. The summary statistics to be generated are the number of observations in which the variable of discussion takes a value on. The second summary statistic to be provided during pre-model analysis will be the mean, or average, value of the variable. </p><p>Third, the median value of the variable will be provided. The median value of a variable is found by arranging the variable’s values in order from lowest value to highest value and choosing the middle value. This middle value out of all the values the variable takes on is the median. Also, provided during pre-model analysis for the summary statistics is the minimum and maximum values for the variables. Additionally, the standard deviation of each variable will also be calculated. The last variable to be provided with the summary statistics is the coefficient of variation. All of these variables give us more insight into the nature of the variables.</p><p>By looking at the mean, median, and standard deviation of the variables we can get a range the variable’s values will take. Then, we can look at the minimum and maximum values for values that appear as outliers or extreme values that could make our model results invalid. </p><p>These extreme outliers could be due to data entry error or because of other peculiar cases. Dansby and Johnston 16</p><p>Sometimes it is better to look a variables median than its mean. While the average can be skewed by an extreme outlier pulling the average up or down, the median statistic measures the middle value of the variable. </p><p>Interestingly enough, the mean can be used in lieu of the coefficient of variation (to be discussed next) for measuring the binary variable’s variation. Dummy variables must have sufficient weight in a sample in order for the model to give accurate results if the dummies are used. The mean of a dummy variable gives us the proportion of the sample that has the trait the dummy variable is measuring for. A rule of thumb for the “sufficient” weight of a binary variable is for its mean in the sample to be greater than or equal to 10 percent, but less than or equal to 90 percent. Thus, we must make sure all dummy variables created for the data set must subscribe to that range in order for their results in the model (if they are used as explanatory variables) to be valid. </p><p>In order for the model to be constructed both the dependent, or explained, variable (sales) and explanatory variables must have “sufficient variation”. A statistical measure of this </p><p>“sufficient variation” is usually determined by the calculation of a variable’s coefficient of variation (CV). The CV is calculated by dividing the variable’s sample standard deviation by its mean, or average, and multiplying that result by 100. The CV’s formula is as follows,</p><p>1.) Coefficient of variation = CV = </p><p>A CV greater than 2 for the explained variable sales or explanatory variables usually qualifies as statistical rule of thumb for having a large enough variation for explanation power in the model. </p><p>In terms of the explained variable, sales, if sales never has any variation, does not change, or only changes very little, then the explanatory variables cannot reveal anything to us. This is because a change in one variable cannot explain the movement in another variable that does not Dansby and Johnston 17 move in either direction at all. On the other hand, if there is no variation in the explanatory variables then the estimates for the model we intend to formulate using OLS will not exist. OLS estimates will not exist if we have perfect correlation between two or more regressors in our model. This correlation between two or more independent variables in our model is known as multicollinearity and will be discussed later during mode building. In this case the estimates from the OLS model do not exist. If the explanatory variables have no movement, then the model cannot say whether or not that they actually cause movements in the dependent, or explained variable, sales. When looking at the summary statistics we must make sure all the variables we wish to select for model building will have “sufficient” variation.</p><p>The next step in the pre-modal analysis is to look at the relationships between the independent variables and the dependent variable, sales. In order for us to build a model predicting sales we must use variables that explain the change in sales the most. The statistical measure of one variable explaining the change in the other is called the correlation coefficient, which is calculated for two variables Xj and Xs by taking the covariance of Xji and Xsi and dividing by the product of the variance of Xji and the variance of Xji.</p><p>2.) rXj Xs = , where r </p><p>The correlation coefficient shows how closely related the two movements or changes in the two variables are with one another. Values of the correlation coefficient that are equal in absolute value to 1 indicate a perfection correlation between the two variables while at the other spectrum, a value of zero indicates that there is no correlation between the two variables. Negative correlation coefficients indicate a negative relationship between the variable and sales, while positive correlation coefficients indicate a positive relationship between the variable and sales. Dansby and Johnston 18</p><p>When we generate a correlation coefficient between an independent variable and the dependent variable sales, we often test this value to see if it really does have an effect on the change in sales. Specifically, we test the null hypothesis that independent variable has no effect on the sales. When we test this we get a p-value. The p-value measures whether or not the value real existence is different from zero, or not equal to zero, for the case of the null hypothesis that the correlation coefficient equals zero. The smaller the p-value, the stronger the existence measure is different from zero and indicates a strong, linear relationship with sales. For example, a p- value of 0.09 for an explanatory variable and sales means that the we can say with 91% probability that the explanatory variable has some effect on sales, although we cannot say specifically how much because we are only testing whether the variable has any effect versus no effect on sales. We could have a correlation of coefficient of 0.8 for an independent variable and sales that shows the two variables explain a great deal of their movements together but still have a large p-value of 0.2 (although this is highly unlikely for this case and only used for illustration). So, we could only say with 80% probability that that independent variable has some effect on sales. </p><p>So, when conducting pre-model analysis we must first generate the correlation coefficients to learn which variables explain the change in sales the greatest. In other words, we must choose variables that have highest correlation coefficients between sales with small p- values for our model (because as stated above, the smaller the p-value the more we can be sure, in probabilistic terms, that the explanatory variable does have an effect on sales). Thus, the variables we end up choosing for our model will explain sales the greatest and will give us the most consistent and efficient results in predicting sales. Dansby and Johnston 19</p><p>Unfortunately, the correlation coefficient between two variables only expresses a linear relationship. In some cases, we need to determine if there is a non-linear relationship between a variable and sales. If there is a non-linear relationship then we must make a new variable to accommodate this relationship in the forecast model. In order to find possible non-linear relationships between independent variables and the dependent variable, sales, we will generate scatter plots between them. By looking at the scatter plots we will be able to see with a naked eye whether the function exhibits a linear relationship, a quadratic relationship, cubic relationship, or any other non-linear relationship. If we do find any non-linear relationships between any of the variables we choose for model selection and sales we must consider it during the actual model building.</p><p>Now that we have discussed the steps and processes used in pre-model analysis we can show the reader the results. From these results of pre-model analysis we can pick 30-50 candidate regressors for our model building process. The following section reviews and summarizes the results of these steps and which candidate regressors were chosen and why. </p><p>Pre-Model Analysis, Results</p><p>Below you will see the summary statistics table (Table 2) for the dummy variables. N is the number of observations the variables take a value in. Mean is the average, median is the median of the variable, and Std Dev is the standard deviation of the variable. Also, the minimum and maximum are the minimum and maximum values of the variable. Normally, the coefficient of variation is included in the summary statistics, but, as the reader will recall from the pre- modal analysis steps, for dummy variables we cannot use the CV for determining if the dummy variable has enough variation, or more specifically for dummy variables, weight. Thus, we have Dansby and Johnston 20 left out the CV in the summary statistics for the dummy variables we created. From the steps of pre-model analysis we know that we must look at the mean of the dummy variable and make sure it is in the range of 10% to 90%. Looking at Table 2 we can see that no store for Casa </p><p>Table 2: Dummy Variable Summary StatisticsBonita is located in an urban Variable N Mean Median Std Dev Minimum Maximum CV DUMBUDS_RURAL 112 0.008929 0 0.0944911 0 area. Also,1 we can see that1058.3 a DUMBUDS_INTOWN 112 0.142857 0 0.3514998 0 1 246.0498741 DUMBUDS_SUB 112 0.758929 1 0.429656 0 majority of1 the stores 56.6134917are DUMBUDS_METRO 112 0.089286 0 0.2864373 0 1 320.8097862 DUMBUDS_URBAN 112 0 0 0 0 located in 0suburban. areas and DUMREGION_SA 112 0.178571 0 0.3847144 0 1 215.4400483 DUMREGION_WM 112 0.089286 0 0.2864373 0 the western1 south-central320.8097862 DUMREGION_WNC 112 0.089286 0 0.2864373 0 1 320.8097862 censusDUMREGION_ENC region. We will112 not0.107143 be able to use0 the0.3106849 variables DUMBUDS_RURAL,0 1 289.9725575 DUMREGION_WSC 112 0.383929 0 0.4885267 0 1 127.2441543 DUMREGION_ESC 112 0.026786 0 0.1621823 0 1 605.4804758 DUMBUDS_METRO, DUMREGION_WM, DUMREGION_WNC, DUMREGION_ESC, and DUMREGION_NE 112 0.035714 0 0.1864109 0 1 521.9506034 DUMREGION_MA 112 0.089286 0 0.2864373 0 1 320.8097862 DUMREGION_MA because all have means less than 0.10 (10%). However, </p><p>DUMBUDS_METRO, DUMREGION_WM, DUMREGION_WNC, DUMREGION_MA are very close to 0.10 (the difference from 0.10 is negligible), so we could use them if we wanted to drop a few observations with values of 0 for those variables to give those variables more weight in our smaller sample. We, however, could make the assumption that it is so close to 0.10 that it will not reduce the model’s accuracy and efficiency, but we will still consider dropping some observations during model building to make some of the dummy variables that we want to have a weight of at least equal to 0.10.</p><p>The next step in pre-modal analysis is to examine the observations for any errors that may have occurred during the data collection process. All of the variables with _XTO at the end have measurements of the variable at the successive geographic distances of 4, 8, and 14-minute drive time distances from store “i”. These variables’ values should increase or stay the same as the geographic area increases. If any observation has conflicting numbers for any of these Dansby and Johnston 21 variables’ drive time distances then that observation should be removed due to data entry. Our analysis did not find any observations with conflicting values for these types variables, thus we still have 112 observations with 449 variables for analysis and eventually model building. </p><p>Table 3: Summary StatisticsContinuing, our analysis team then Variable N Mean Median Std Dev Minimum Maximum Coeff of Variation A01V001_14TO 112 214233.1 199499 84854.12 51605 458310 39.6083218 A12VBASE_14TO 112 161355.2 153880.5 63057.33generated37916 the summary371820 statistics39.0798373 for all the B03V001_14TO 112 49857.28 47200 19793.73 12751 113703 39.7007907 B12V004_14TO 112 19370.15 18512 7196.95 5734 43718 37.1548684 B14V002_14TO 112 11543.22 10160.5 5203.29variables to3051 see if these29706 statistics45.0765864 revealed B16VBASE_14TO 112 47168.47 46168.5 17683.44 13051 99169 37.4899667 B29VV01_14TO 112 48.19643 49 3.4844294 33 57 7.2296424 BAR_SEATS 112 57.26786 58 8.7291395any information30 about72 the variables15.2426508 and C01V012_14TO 112 9905.27 9816 3902.6 1943 20389 39.399225 CM_MEX_60647_1RO 112 0.071429 0 0.2586969 0 1 362.1756082 CM_NONMEX_54598_1RO 112 0.178571 0 0.3847144observations0 that we did1 not detect215.4400483 by CMHO_MEX_58508_14TO 112 4.864576 0 15.2700816 0 83.7938888 313.9036301 CMHO_MEX_58514_14TO 112 4.578668 0 16.90052manual analysis0 97.8421217 of the Casa Bonita369.1143664 data CMHO_MEX_62081_14TO 112 15.82225 0 30.9241738 0 100 195.4473436 CMHO_MEX_62094_14TO 112 15.19597 0 32.6767907 0 100 215.0358814 CMHO_NONMEX_55727_14TO 112 19.36885 0.01872 31.9193779set. Above the0 reader100 will see Table164.7974617 3 </p><p>CT_POW_53889_0_5RO 112 0.017857 0 0.1330273 0 1 744.9529884 CT_POW_54432_0_5RO 112 0.142857 0 0.3514998which gives 0us the summary1 statistics246.0498741 for CT_POW_55095_0_5RO 112 0.017857 0 0.1330273 0 1 744.9529884 CT_POW_55491_0_5RO 112 0.071429 0 0.2586969 0 1 362.1756082 CT_POW_56128_0_5RO 112 0.089286 0 0.2864373our “candidate”0 variables1 (these320.8097862 CUST_VALUE 112 3165608 2901585.3 1706428.68 407261.16 9262996.64 53.9052433 D02V001_14TO 112 63043.59 59038.5 25336.38 15109 147974 40.1886681 “candidate”D02V007_14TO variables will be discussed112 1052.53 later).870.5 As in680.896838 Table 2, the 107headings3054 represent the64.6916399 same D02V018_14TO 112 69384.06 65704 28215.89 16288 169100 40.6662366 D02V024_14TO 112 1143.35 971.5 747.092873 56 3458 65.3425495 thing. WeD02VBASE_14TO have the number of observations112 132427.7 124357.5 that each53456.2 variable takes31397 a value313843 for in, the40.3663281 mean and D07V002_14TO 112 16811.25 14108 9479.86 3685 48730 56.3899705 D07V003_14TO 112 15521.68 14308.5 6421.96 3282 40184 41.3741377 median, asD07VBASE_14TO well as the standard 112deviation.96191.65 Table92231 3 also38036.73 has the 21229minimum,223860 maximum, and39.5426494 the DINING_SEATS 112 166.5536 178 30.3234335 110 275 18.2064144 DUMREGION_MA 112 0.089286 0 0.2864373 0 1 320.8097862 coefficientDUMREGION_WM of variation. We first112 analyze0.089286 our dependent0 0.2864373 variable sales,0 and see1 that it320.8097862 has DUMREGION_WSC 112 0.383929 0 0.4885267 0 1 127.2441543 EA04V002_14TO 112 99243.38 93277 39161.15 23572 220436 39.4597097 sufficient EA04V003_14TOvariation because its CV112 104465.9is well above99352.5 2. 41303.92Also, its minimum24437 and235769 maximum39.5381755 adhere to EA07V010_14TO 112 11871.96 7465 11658.38 460 54299 98.2009107 the range EB27V001_14TOof its mean and standard112 deviation.835592 761542 The 374210.21maximum value203948 of sales2462004 is still below44.7838435 three LUNCH_CUST_VALUE 112 830316.8 784573.19 529243.64 20852.76 3063420.44 63.7399641 PATIO_SEATS 112 46.85714 48 11.626203 16 109 24.8120187 standard deviationsPOP_14TO from its mean.112 203709.2 Now we 192201can look80376.03 at all the possible48009 explanatory456204 variables’39.4562566 SALES 112 2620466 2519274.7 697152.36 1317062.07 4489364.22 26.6041404 SimI_04005_14TO 112 98.49681 98.37855 4.8216613 77.1069 115.6381 4.8952463 summary statistics.SQFT 112 7028.02 7234 795.171272 4312 8868 11.3143035 WMOSTOT_14TO 112 113751.7 104557 63480.27 16091 352797 55.805994 X01V079_14TO 112 56790257 53862203 23531902.1 12411594 138524409 41.4365128 First,X01V080_14TO a majority of all the112 variables84815547 81663918reviewed34805053.1 during summary18354049 208347912statistics analysis41.0361711 all had X01V084_14TO 112 6909395 6314298.5 3365606.29 1244387 20520222 48.7105758 X01V088_14TO 112 12991407 12265214 5179138.17 2753959 34449064 39.8658753 statistics thatX01V091_14TO did not show any 112signs5054894 of irregularity4814267.5 2047066.01 or hints of 961680data entry.13136940 However, a40.4967113 few variables warrant discussion. Beginning, Store_ID’s summary statistics are worthless because Dansby and Johnston 22 they are just unique numbers for identifying a store and do not even affects sales. All the variables that measure percentage households shared between Casa Bonita and one of the competitors (Mexican and non-Mexican) all have sufficient variation because their CV’s are all above 2. Also, for types of variables, such as CMHO_MEX_62081_14TO, their maximum values should not exceed 100. This is because it is a percentage measure and a value greater than 100% does not make mathematical since, and thus would be signs of data entry for the variable. Luckily, all the maximums for these percentage typed variables do not exceed 100. </p><p>Continuing, we noticed that almost all of the variables – save the odd one or two – measuring the number of competitor stores (Mexican and non-Mexican), department stores, grocery stores, malls, and power centers of each individual type only take on the values of 0 or 1. This indicates that each Casa Bonita restaurant either is located to that Mexican competitor restaurant A or department 6, for example, or not. This means that these variables truly act like dummy variables although they are technically not dummy/binary variables. This makes sense because you would not think that a competitor would place two of their stores in a 1 mile or half-mile radius of one of Casa Bonita’s stores. Thus, although these variables all have CV’s above 2 </p><p>(except for the variables which take no values for any of the observations in our sample, for example CT_POW_56133_0_5RO takes no value in our sample set) we cannot judge these variables’ variation or weight based on their CV’s because they act like dummies. So, we must look at the mean of each of these variables to see if they adhere to the dummy variables range we described earlier (0.l0 ≤ dummy mean ≤ 0.90). </p><p>The reader can see from Table 3, of all dummy type variables included in our candidate regressor list, only CM_NONMEX_54598_1RO and CT_POW_54432_0_5RO subscribe to sufficient weight range for dummy variables. If we want to include the other variables of this Dansby and Johnston 23 nature in our final model we must account for them being so low in terms of proportion of our sample. We could either combine some of these variables or decrease the size of our sample size in order to make those smaller means have a greater proportion than 10% in the new sample. </p><p>This will be discussed and analyzed in more detail during model building and analysis. Now we have noted a majority of the variables have sufficient variation and their other summary statistics do not indicate irregularities that we would not expect with the variables nature, we can begin to look at the relationships between the explanatory variables and the explained variable, sales. </p><p>As the pre-model analysis steps stated, we must next evaluate which variables explain sales the most. We measure this by using the correlation coefficient defined by equation 2.). </p><p>Our analysis team generated the correlations between all the variables and sales and ordered them from greatest in absolute value to lowest. Next, we selected the top 140 variables in that list in terms of having the highest correlation value in absolute terms. We chose this top 140 list on the basis of having a correlation coefficient with an absolute value of 0.15 or higher. This is the list that we chose our top 49 variables from except for one variable, </p><p>LUNCH_CUST_VALUE. We chose that variable specifically because it was not very far from having a correlation coefficient with an absolute value of 0.15. It had a correlation coefficient of</p><p>0.14227 with a p-value of 0.1345. So, we can say with about 86% certainty that </p><p>LUNC_CUST_VALUE does have some effect on the sales, and we believe that variable should be accounted for in the final. We expected this variable would also explain sales in much of the same way CUST_VALUE does. In order to choose the other 48 of the 49 out of the top 140 variable correlation coefficient list we needed to take more statistically subjective course of action. Dansby and Johnston 24</p><p>Although it seemed a tedious task of withering down the list of 140 top correlation coefficients with sales to 48, it was easier than it first seemed because many of the variables in that top 140 list included the same variables just at different drive times, most notably at either an 8-minute or a 14-minute drive time. To our analysis team, it seems more likely that a 14- minute drive time variable’s value measures the change in sales for a particular store “truer” than the same variable measured at an 8-minute drive time. This is because when measured out an 8- minute drive time, a variable does not take into account the people inside a 14-minute drive time but outside an 8-minute drive time. This 6-minute gap could affect how the variable really explains changes in sales because we assume that the 14-minute drive time is considered to be the area of business for each particular Casa Bonita store. Also, many variables in the top 140 list contained the same variables at 8-minute and 14-minute drive time measures and most of their correlations were not very different. Thus, we chose to not include the 8-minute variables and chose 14-minute variables when possible, excluding the competitor and other different types of store measures because they were only measured at a half-mile of a 1 mile radius. </p><p>Below the reader will see the correlation coefficients for each of the 49 “candidate” variables between sales in Table 4. The p-values are shown below the correlation coefficients. </p><p>They are listed from greatest correlation coefficient value in absolute terms to least in value. </p><p>These candidate variables, or regressors, are called such because they will be candidates for variables to be used in our final model building process. Many of the variables in the top 140 list for correlation coefficients between sales and explanatory variables were variables measuring the level of education attainment for males and females, separately. We tried to choose a variable that accounted for most of these strongly correlated variables. So, since the variables measuring educational attainment were mostly for no education, up to 4th grade, up to 6th, up to 7th, and up Dansby and Johnston 25 through 12th, were strongly correlated with sales for both males and females we chose to pick a middle ground. We chose to include the two variables D02V006_14TO and D02V024_14TO since they measured the number of males or females, respectively, who had obtained an education of at least up to 10th grade in a 14-minute drive time of store “i”. Luckily, to shorten Dansby and Johnston 26</p><p>Table 4: Top 49 Candidate Variables' Correlation Coefficient with Sales Correlation Coefficient Explanatory Variables (p-value) SQFT 0.30341 (0.0011) DUMREGION_MA 0.29517 (0.0016) D02V007_14TO 0.27363 (0.0035) D02V024_14TO 0.28245 (0.0026) B14V002_14TO 0.27148 (0.0038) DINING_SEATS 0.26363 (0.0050) EB27V001_14TO 0.26217 (0.0052) BAR_SEATS 0.25224 (0.0073) SimI_04005_14TO 0.24078 (0.0105) PATIO_SEATS 0.22785 (0.0157) B29VV01_14TO 0.22704 (0.0161) CMHO_MEX_62094_14TO 0.22373 (0.0177) CMHO_MEX_58514_14TO 0.21417 (0.0234) EA07V010_14TO 0.21139 (0.0253) D07V002_14TO 0.20843 (0.0274) CM_MEX_60647_1RO 0.19098 (0.0437) DUMREGION_WM -0.18906 (0.0459) CT_POW_55491_0_5RO -0.18769 (0.0475) X01V091_14TO 0.18465 (0.0513) X01V080_14TO 0.18416 (0.0519) X01V084_14TO 0.18077 (0.0565) EA04V002_14TO 0.17524 (0.0646) CMHO_NONMEX_55727_14TO -0.17521 (0.0646) X01V088_14TO 0.17508 (0.0648) D02V001_14TO 0.1746 (0.0656) X01V079_14TO 0.17411 (0.0664) CT_POW_53889_0_5RO 0.17249 (0.0690) B03V001_14TO 0.17167 (0.0703) CMHO_MEX_62081_14TO 0.17146 (0.0707) POP_14TO 0.17056 (0.0722) D02VBASE_14TO 0.16889 (0.0751) CMHO_MEX_58508_14TO 0.16767 (0.0772) WMOSTOT_14TO 0.16683 (0.0787) CT_POW_55095_0_5RO 0.16683 (0.0787) B12V004_14TO 0.16578 (0.0807) the large list of educationalEA04V003_14TO attainment variables that were strongly correlated with sales0.16575 we had (0.0807) D07V003_14TO 0.16518 other variables that essentially measured the same thing to choose from, such as the (0.0818) income Dansby and Johnston 27 variables, in which a few were strongly correlated sales. Thus, we chose the variable </p><p>C01V012_14TO which measured the number of households with income in the range of $75 thousand to $99,999 dollars in a 14-minute drive time area of store “i”. Luckily, we had other variables to choose from that also measured income or wealth in other ways. We chose the variables that measured the number of a certain type of worker that worked in a 14-minute drive time of store “i”. For example, we chose the strongly correlated variable of D07V003_14TO which measured the civilian population employed in service and farm jobs. Our analysis team also chose many population variables that measured number of males and females at certain age Table 4, continued: Top 49 Candidate Variables' Correlation Coefficient with Sales Explanatory Variables Correlationrestrictions, Coefficient number of households, (p-value) A12VBASE_14TO and the number of Asian people0.16427 in a 14- 0.0835 D02V018_14TO minute drive time of store0.16319 “i”, for 0.0856 C01V012_14TO example. We also chose0.16289 to include 0.0862 DUMREGION_WSC the variables that measured the0.16007 percent of 0.0918 B16VBASE_14TO 0.1598 households that a Casa Bonita store 0.0924 CM_NONMEX_54598_1RO -0.15715 share with one of its full-service restaurant 0.098 D07VBASE_14TO 0.1548 competitors. Additionally, we chose 0.1032 CT_POW_54432_0_5RO -0.15445 PATIO_SEATS, BAR_SEATS, and DINING_SEATS because they were all strongly correlated 0.104 CUST_VALUE 0.15437 with sales, so they would be very good candidate variables for our forecast model. 0.1041 CT_POW_56128_0_5RO 0.15265 When consumers make a purchase of food at one of Casa Bonita’s stores they are 0.1081 A01V001_14TO 0.15028 increasing their expenditure for certain goods, whether it be the beer they got or the taco they 0.1138 LUNCH_CUST_VALUE 0.14227 purchased. Obviously, as we assumed, all the expenditure variables were strongly correlated0.1345 with sales; even the variables that were not bar/restaurant related, such as the variable measuring expenditure for out-of-town trips, X02V072_XTO. Thus, we chose to include all the Dansby and Johnston 28 expenditures variables that were related only to a restaurant’s products or services. So, we left out the expenditure on theater and amusement parks and expenditure on out-of-town trips variables in our 49 candidate variable list. We also included the very interesting variables </p><p>SimI_04005_14TO and A01V001_14TO. We chose SimI_04005_14TO because it measuring how receptive the potential customers in the area are to advertising. Not only is this variable strongly correlated with sales, it also provides for us, if we include it in the final model, an idea of how much money we should be putting forward towards advertising for that particular Casa </p><p>Bonita restaurant. Since A01V001_14TO measures the project population in 5 years for the 14- minute drive around store “i”, we can think of this measure as not only a good variable for causing changes in sales, but also as a measure of stability and income level in the neighborhood surrounding store “i”. So, by using this variable Casa Bonita could determine how susceptible the area around a store “i” is to economic downturns which could affect sales negatively, or vice verse if it was a positive economic affect. Thus, these two variables should prove to be of great use for Casa Bonita, regardless if they are used in the final model or not. </p><p>The reader should familiarize themselves with the correlation coefficient values of each of the candidate variables for model selection in Table 4. Their absolute values of the correlation coefficients will determine their worth in the final model, and the effect each variable chosen for the model has on sales should adhere to the sign of its correlation coefficient. So, if we use a variable in the model for predicting sales that has a positive correlation coefficient, but its slope parameter in the model is negative then we know we have something wrong in our model, possibly by such things as multicollinearity (to be discussed in model building and selection). </p><p>Unfortunately, as we discussed in during the discussion on pre-model analysis steps, correlation coefficients between the each explanatory variable and sales only show us a linear Dansby and Johnston 29 relationship. Sometimes the independent variables may exhibit a non-linear relationship with sales, such a quadratic relationship. To look into the candidate regressors possibly having a non- linear relationship with sales, we generated the scatter plots between the candidate regressors and sales. Below the reader will find 10 scatter plot matrices label 1-10. The top row and first column in each matrix shows the relationship of the chosen variables and sales. The top row plots sales on the vertical axis and the explanatory variable on the horizontal access, while the first column plots the explanatory variable on the vertical access and sales on the horizontal access. It is clear from the plots on the first row of both the Scatter Plot Matrices1 and 2 that the relationship of every chosen variable with sales is linear. Consequently, the model does not call for non-linear relationships to be introduced in our forecast models. As, the reader can obviously see from the plots on the top row of each Scatter Plot matrix, 1-10, all of the plots show that the candidate regressors only have a linear relationship (although not a perfect one) with sales. However, one should take particular note to the variables that are dummies or act like dummies (i.e. the variables measuring the number of competitors or other types of stores around store “i” because they are variables that only take on values of zero or one). Those variables do not show a particularly great linear relationship so that will have to be accounted for during model building if those variables are to stay in the final model for predicting sales. Also, the reader should take note of the poor, however still linear relationship, between sales and the variables measuring the percentage households shared between a Casa Bonita store and one of its competitors (Mexican and non-Mexican). This too, will need to be considered during model building, because it is not a particularly great showing of a linear relationship – which our model is trying to measure and predict. Finally, we are left with 49 candidate regressors from 112 Dansby and Johnston 30 observations for which we can use for constructing a model for predicting annual sales at individual Casa Bonita stores. Dansby and Johnston 31 Dansby and Johnston 32</p><p>Model Building, Steps:</p><p>After pre-model analysis has been completed, our analysis took the following steps to build revenue forecast models to be used for model selection for Casa Bonita. The first step in Dansby and Johnston 33 model building is to create or add variables to the top correlated variables (with sales) list that are called for by logic and/or economic demand theory. Next, we must make sure our model will not have the problem of micronumerosity. Finally, we must make check the correlation between the possible regressors for our final models during the selection process. This can sometimes lead to the problem of multicollinearity, which could invalidate our models estimates if we are not careful in our model building. Finally, we can build our models out of the chosen candidate regressors while taking into account the problems discussed below. A full discussion of the results of these processes for model building will be discussed after following discussion on the steps taken for model building. </p><p>Using the summary statistics and correlation of the variables’ relationships with sales we have reduced the number of variables ready for model building drastically. These, variables have already shown to explain a large amount of the change in sales. However, when building a model, the mathematics of statistics can make us lose sight of variables that should logically be included in the final model for predicting sales. One of the first things that logic brings to mind in explaining sales for individual stores is using the explained variable, sales-per-square feet instead of just sales. Our analysis team created this variable under this notion, but upon further review, we came to realize that sales was still a better dependent variable for Casa Bonita’s sales prediction model because of the nature of their business. One their restaurants could have a large amount of space, yet have fewer tables than another Casa Bonita’s across the nation in a denser city that has a smaller amount of square feet. Thus, sales-per-square feet is not a good measure of the predicted revenue for the potential new stores. Also, since our case, square feet was moderately correlated with sales, it is, in our opinion, better to include it in the model as a regressor so that the Casa Bonita market analysis team can use the model to even estimate the Dansby and Johnston 34 returns from the size of particular stores on sales revenue for that individual store. Thus, sales is a better measure, since we are only concerned with the revenue potential of individual stores and whether or not that forecasted revenue will be profitable. A similar discussion holds for another possible dependent variable. </p><p>A second possible dependent variable our analysis team discussed was, the variable we created, SALES_BY_TOTAL_SEATS. This variable measured sales per seat in the restaurant. </p><p>TOTAL_SEATS was calculated by the adding patio seats, dining seats, and bar seats variable measures, then we calculated the SALES_BY_TOTAL_SEATS variable by taking the SALES variable divided by the TOTAL_SEATS variable. The reason as to why our team continued to choose sales as the dependent variable over this sales-per-seat variable was similar to the reasoning for not using sales-per-square-feet. Thus we chose to include it in our top list of regressors to be used in model building, so that the Casa Bonita market analysis team could see the amount of money that could be generated for a store if a certain number of seats were added to the service area. Thus, we have stated our reasons as to why sales is a better dependent variable over sales-per-square-feet and sales-by-total-seats.</p><p>Now that we have discussed our independent variable to be used for our model building in depth we much discuss possible regressors that we did not include because the statistics did not call for it, but our logic and demand theory, however do call for them. It should be noted that the summary statistics, correlations with sales, and the scatter plots with sales will be included and discussed in full during the results of model building for these variables added to the list of candidate regressors. We shall first start with the variables we created and added to our list of top correlated variables (with sales) based on logic. First, and most obvious, we chose to include our TOTAL_SEATS because since the patio seats, dining seats, and bar seats variables were all Dansby and Johnston 35 somewhat strongly correlated with sales. Next, to reduce the number of possible regressors in model (the problem of micronumerosity to be discussed next) we combined the lunch customer value and the customer value variables to obtain the variable TOTAL_CUST_VALUE. </p><p>From the Table 4, the reader can clearly see that two of the power center variables are negatively correlated with sales. Since, the variables CT_POW_55491_0_5RO and </p><p>CT_POW_54432_0_5RO, for the power centers 26 and 13, respectively, were both moderately negatively correlated with sales our analysis team summed the two together to create the variable</p><p>BAD_POW_0_5RO. We also combined these together to get rid of the insufficient variation or weight measure of the variable CT_POW_55491_0_5RO that acts like a dummy variable. </p><p>Similarly, we summed together the power centers variables CT_POW_56128_0_5RO, </p><p>CT_POW_55095_0_5RO, and CT_POW_53889_0_5RO for the power centers 31, 20, and 4, respectively, because they were all positively correlated with sales and to relieve the problem of each of those variables having insufficient weight individually to be used in the revenue forecast model. The variable created from the summation of these three positively correlated variables with sales is named GOOD_POW_0_5RO. </p><p>Since a few dummy variables for the regions were moderately correlated with sales to a good extent we created a variable that combined the dummy region variables that were positively correlated with sales and a variable that combined dummy region variables that were negatively correlated with sales. We made the variable DUMREGION_GOOD out of the two dummy region variables for the mid-Atlantic region and the western south-central region, or </p><p>DUMREGION_MA and DUMREGION_WSC, respectively, that were both postiviley correlated with sales. We combined the two variables to reduce the number of possible regressors in our final model if we decided to use both the variables and also to relieve the problem of insufficient Dansby and Johnston 36 weight of the dummy variable DUMREGION_MA that we discussed earlier during pre-model analysis (it had a mean of only about 0.0892). Correspondingly, we created the variable </p><p>DUMREGION_BAD out of two dummy region variables that were negatively correlated with sales. The two dummy region variables negatively correlated with sales were </p><p>DUMREGION_SA and DUMREGION_WM. It should be noted that during pre-model analysis of the variables DUMREGION_SA failed to make it into the top 149 variables correlated with sales that we used to construct our candidate regressors list. Luckily, our team noticed this during a later review of our pre-model analysis before we began model building. It was quite correlated with sales and should have been included in the top 149 list and subsequently in the candidate regressor list. The DUMREGION_BAD variable was also made to get rid of the problem of insufficient weight for the variable DUMREGION_WM measured by its low mean of about 0.0892 that was discussed earlier. All these variables that were created from our analysis team’s logic beyond statistical review will be included with the top 49 candidate regressors list in our model subsequent model building. Continuing, our model analysis and building team used economic theory concepts to add variables to the candidate regressor list. </p><p>On the basis of economic theory, if we can assume that the transactions and prices between the Casa Bonita restaurants and consumers are in equilibrium, we can assume sales is the amount of quantity demanded. Thus, theory calls for a variable that measures income or a variable measure that is a proxy for measuring income, such as expenditure. Since expenditures by a consumer are directly related with their income, a variable measuring expenditures on bar- related services and products will suffice for type of income measurement variable. We already have expenditure variables in our 49 candidate regressor list (which can be seen in Table 4), but to avoid having to many regressors in our models that could lead to problems (micronumerosity, Dansby and Johnston 37 to be discussed next) we created an expenditure variable measuring the total expenditures on bar- related items and services. Specifically, we summed the five expenditure variables to arrive at the variable, TOTAL_BAR_EXPEND_14TO. This variable measures the total annual expenditures on lunch, dinner, catered meals, beer & ale, and other alcohol for the full calendar year of 2012 by consumers living within a 14-minute drive of store “i”. Analysis team, found this variable to be quite correlated with sales (as the reader will see in the model building results section), but considered adding the two other expenditure variables that were not included in our </p><p>49 candidate regressor list. The two variables, X02V071_14TO and X02V072_14TO that measured expenditure on theater & amusement parks and out-of-town trips, respectively, we added to the TOTAL_BAR_EXPEND_14TO to get TOTAL_EXPEND_14TO. As first thought, our analysis showed that adding these two variables to the </p><p>TOTAL_BAR_EXPEND_14TO variable did not increase its correlation with sales, statistically speaking. So, we chose to add TOTAL_BAR_EXPEND_14TO to our candidate regressor list for model building instead of TOTAL_EXPEND_14TO. This also makes sense, as we are trying to predict sales for a bar, so we need measures that effect sales; specifically, bar type expenditures which X02V071_14TO and X02V072_14TO do not measure. Additionally, our model construction team also created a per-capita expenditure variable for a 14-minute drive time out of the total bar expenditure variable by dividing TOTAL_BAR_EXPEND_14TO by the total population variable POP_14TO. This variable was found to not be correlated with sales, statistically speaking, and thus will not be included in our candidate regressor list for model building and will also be excluded from further discussion. So, after creating and adding the variables discussed above we now have a list of candidate regressors totaling 57 variables that all effects sales. Since we have 57 candidate regressors that could all possibly used in model Dansby and Johnston 38 building, we need to discuss the problem of having including too many regressors in a single </p><p>OLS model. The reader should review Table 5 below to familiarize themselves with the definitions and/or formulas for the new variables added to the list of candidate regressors for </p><p>Table 5: Variables Added to the Candidatemodel Regressor building. List Variable name Column1 Variable Formula # of power centers 13 and 26 located within a one-half CT_POW_55491_0_5RO + CT_POW_54432_0_5RO BAD_POW_0_5RO radial mile of store “i” CT_POW_56128_0_5RO + CT_POW_55095_0_5RO # of power centers 4, 20, and 31 located within a one- GOOD_POW_0_5RO + CT_POW_53889_0_5RO half radial mile of store “i” An issue of concern when building</p><p> an index that measures the degree of value of the CUST_VALUE + LUNCH_CUST_VALUE TOTAL_CUST_VALUE people who live in the area and the people OLSwho work models for forecasting sales is in the area around store “i” TOTAL_SEATS # of bar seats, patio seats, and dining seats inwhether store “i” BAR_SEATSor not our + PATIO_SEATS sample size + DINING_SEATS is large expenditure on lunch, dinner, catered meals, beer, X01V079_14TO + X01V080_14TO + X01V084_14TO TOTAL_BAR_EXPEND_14TO ale, and other alcohol by consumer who live within a + X01V088_14TO + X01V091_14TO 14-minute drive of store “i” enough to provide us with accurate dummy (binary) variable that takes on the value of 1 if DUMREGION_MA + DUMREGION_WSC estimatesDUMREGION_BAD for the model. store The “i” term is located used in the for U.S. the census issue region of of having the a small data set is south Atlantic region or the mountain west region DUMREGION_MA + DUMREGION_WSC micronumerosity. If wedummy have (binary) a large variable amount that takes of onvariables, the value of yet1 if only a small sample size we DUMREGION_GOOD store “i” is located in the U.S. census region of the mid- Atlantic region or the western south-central region cannot apply the statistical techniques used to calculate the forecast model because a small dummy (binary) variable that takes on the value of 1 if DUMREGION_SA store “i” is located in the U.S. census region of the sample set will obviouslysouth have Atlantic a large region sample standard deviation, or sample error. These large standard deviations give us mixed results when we are looking at variable existence, or significance. We cannot be certain about our results then. There only two ways to combat the problem of micronumerosity. One is to increase the sample size, while the other way calls for the model to have less parameters estimated that the size of the sample provided. Luckily, the sample size of 112 is fine for OLS regression analysis and we can apply the statistical techniques needed. Also, we will not need a large amount of explanatory, or regressor, variables in the final models because our forecast model does not call for it because many of the variables in our candidate regressor list of 55 variables in essence, measure the same thing. So, as long as we create a parsimonious, or simple, model with fewer regressors than the number of observations in the sample, we should be clear of the problem of micronumerosity. Our team has made sure this Dansby and Johnston 39 did not happen during model building, so we have gotten rid of the possibility of micronumerosity in our final models and thus will not be discussed here forth. </p><p>Now that we have our 55 candidate variables chosen, we must look at the correlations between the explanatory variables of sales so that we can avoid the problem of multicollinearity. </p><p>Multicollinearity is defined as measure of the degree of correlation between the independent, or explanatory, variables in the OLS regression model. It becomes a problem when we have severe to perfect multicollinearity, while models with weak to no multicollinearity do not affect the </p><p>OLS model. Perfect correlation in a model results when one independent variable is perfectly correlated with another variable. In this case the estimates from the OLS model do not exist. </p><p>Severe multicollinearity can cause many problems with the OLS model. Some of the common signs associated with severe multicollinearity are having counterintuitive signs on the estimates, an artificially high R2 value, and/or general F-test that is significant yet the individual t-tests for the estimated parameters are insignificant. The t-tests are smaller than they should be under multicollinearity and parameter magnitudes and signs become counterintuitive because the p- values of the variables are over inflated due to the strong multicollinearity between independent variables. The measure for determining whether multicollinearity exists in a model and whether or not it is severe is determined by the correlation coefficient, r (from equation 2.) between two explanatory variables. A value of 0.9≤IrI<1 is considered severe multilcollinearity, while a value of 0.5≤IrI<.9 indicates strong multicollinearity. A value of 0< IrI<.5 is indicative of weak multicollinearity between independent variables in regression analysis. There are a few of ways that one can alleviate multicollinearity problems. First, the sample size could be increased, but as the data set has already been chosen, this option is impossible. Secondly, we could combine independent correlated variables, which we have done in the case of adding the sports Dansby and Johnston 40 interest index scores, excluding NASCAR, together in SPORTS_SCORE. Third, we could avoid adding strongly correlated explanatory variables to the same models. Last, instead, we could do nothing about the strong multicollinearity, because according to the mathematical properties of </p><p>OLS regression the estimated parameters will still be unbiased. Thus, we must calculate and analyze the correlation coefficients between the candidate regressors to avoid the problem of multicollinearity in building our models. </p><p>Once we have analyzed the summary statistics of the new variables added to our candidate variables list, evaluated the correlation coefficients between the new variables and sales, and ensure that multicollinearity will not be a large problem in our models, we finally begin the construction of sales forecast models to be selected in our final model selection portion of this report below. The results of the steps of model building discussed below are reported and discussed in full below. </p><p>Model Building, Results</p><p>Before we can begin actually building and selecting the models, we must analyze the summary statistics of the 57 candidate regressors. Our analysis team calculated and compiled the summary statistics of this variables in the Table 6 below. The Table is analogous to Tables 2 and 3. As we can see, the three dummy variables all have sufficient weight for indicating that there is enough sufficient variation in those variables so that if used in the model, they estimates would be legitimate. Looking at the CV of the other five variables, we can see that they all have sufficient variation because each of their CV’s is well above 2. While, BAD_POW_0_5RO and </p><p>GOOD_POW_0_5RO both take on the values of 0, 1, and 2, both only take on the value of 2 for one of the observations while 0’s and 1’s are the values for the other 111 observations. Thus, Dansby and Johnston 41 these two variables act more like dummy variables although they are not exactly binary </p><p>Table 6: Summary Statistics for the 8 Variables Added to the Listvariables. of Candidate So, Regressors it is the opinion of Variable N Mean Median Std Dev Minimum Maximum Coeff of Variation BAD_POW_0_5RO 112 0.2142857 0 0.4334769 our model0 analysis2 and building202.2892012 GOOD_POW_0_5RO 112 0.125 0 0.3582993 0 2 286.6394288 TOTAL_CUST_VALUE 112 3995924.8 3669176.34 2191143.8 428113.91 11676110.7 54.8344609 TOTAL_SEATS 112 270.67857 290 42.7475755 team that166 it is wiser385 to look 15.7927446at the TOTAL_BAR_EXPEND_14 112 166561501 161483914 68438179.94 35725669 414978547 41.0888347 means ofTO these two variables to see if they have sufficient weight. As the reader can see from DUMREGION_BAD 112 0.2678571 0 0.4448331 0 1 166.0710076 TableDUMREGION_GOOD 5, both means of the112 BAD_POW_0_5RO0.4732143 0 and0.501526 GOOD_POW_0_5RO0 are above1 0.10105.9828497 (or DUMREGION_SA 112 0.1785714 0 0.3847144 0 1 215.4400483 10%), although GOOD_POW_0_5RO is just barely above that level. So, now we can be sure that all eight of these new variables added to our list of candidate regressors has sufficient weight for OLS estimation. Also, because BAD_POW_0_5RO, GOOD_POW_0_5RO, </p><p>TOTAL_CUST_VALUE, TOTAL_SEATS, TOTAL_BAR_EXPEND_14TO, </p><p>DUMREGION_BAD, and DUMREGION_GOOD are summations of variables whose summary statistics were already discussed, their mean, median, standard deviation, minimum, and maximum values all adhere to the nature of these variables and are not indicative of any entry error. DUMREGION_SA’s mean, median, standard deviation, minimum, and maximum also do not show any signs of error and fine for further analysis. </p><p>After the summary statistics were analyzed for these new variables added to our candidate regressor list, our team then evaluated their correlation coefficients between sales to see which of the eight new variables explained sales the greatest. Below the reader will find Table 7 which contains the correlation coefficient between each of the new candidate regressors and sales. Dansby and Johnston 42</p><p>Table 7: Variables Added to Candidate Regressors List Explanatory Variables Correlation Coefficient (p-value) TOTAL_SEATS 0.30048 0.0013 TOTAL_BAR_EXPEND_14TO 0.18119 0.0559 TOTAL_CUST_VALUE 0.15458 0.1037 BAD_POW_0_5RO -0.23725 0.0118 GOOD_POW_0_5RO 0.24802 0.0084 DUMREGION_GOOD 0.3245 0.0005 DUMREGION_BAD -0.30666 0.001 DUMREGION_SA -0.21381 0.0236 Obvious from Table 7, all the new variables are quite correlated with sales and would have been easily added to the top 149 correlated variables with sales list that was used to pick the original </p><p>49 candidate regressors. As expected for the two variables BAD_POW_0_5RO and </p><p>DUMREGION_BAD, their correlation coefficients between sales are both negative. We expected this because our team made these variables out of variables that were all negatively correlated with sales. Similarly, DUMREGION_GOOD is positively correlated with sales because it was created using the dummy variables for the mid-Atlantic and western south-central regions that were both positively correlated with sales. Since DUMREGION_BAD is the a summation of the two variables DUMREGION_SA and DUMREGION_WM, our team decided to not include DUMREGION_SA in further statistical analysis and subsequent model building and selection since it is included in the DUMREGION_BAD variable which is more correlated with sales than DUMREGION_SA alone. Thus, for further model building we are left with 56 Dansby and Johnston 43 candidate variables to construct an OLS model for predicting annual sales revenue for Casa </p><p>Bonita. </p><p>Before the discussion of potential multicollinearity problems between the variables candidate regressors we selected for model building, we must discuss below a summary of why each variable was chosen for the building of the sales forecast models. Below the reader will see</p><p>Table 8 that gives the definitions of the twelve variables from the list of 56 candidate regressors selected for model building. The reader should refer to the table for the discussion of the variables below. </p><p>TOTAL_CUST_VALUE was chosen because it was a summation of the two variables </p><p>Table 8: Variable Defitions for Twelve Candidate RegressorsCUST_VALUE Selected for Model and Building Variable Name Variable Defitions TOTAL_CUST_VALUE an index that measures theLUNCH_CUST_VALUE degree of value of the people who already listed in live in the area and the people who work in the area around store “i” our BAD_POW_0_5RO # of power centers 13 andtop 26 located 56 candidate within a one-half regressor radial milelist. Although of store “i” the GOOD_POW_0_5RO # of power centers 4, 20, andexact 31 located definition within aof one-half the variable’ radial measures mile of store “i” and DUMREGION_BAD dummy (binary) variable thatways takes of on calculating the value of 1 the if store two “i” variables is are located in the U.S. census region of the south Atlantic region or the mountain west region not SQFT total square footage of storeknown “i” the to the analysis team, we can SimI_04005_14TO an index of the degree of receptiveness to advertising of residents who live withinmake a 14-minute some drive logical of store assumptions. “i” Since B29VV01_14TO median age of household heads living within a 14-minute drive TOTAL_CUST_VALUE is a combinationof store “i” of the two variables, its defined at the measure of the CMHO_MEX_58514_14TO % of households within a 14-minute drive of store “i” that are shared with competitor Mexican restaurant C degree CMHO_NONMEX_55727_14TOof value of the people who %live of households in the area within and a 14-minutewho work drive in of the store area “i” that of are store “i”. shared with competitor non-Mexican restaurant N Although vague,TOTAL_SEATS our team realized# that of bar the seats, reason patio seats, for thisand dining overgeneralized seats in store “i” and vague definition TOTAL_BAR_EXPEND_14TO expenditure on lunch, dinner, catered meals, beer, ale, and other alcohol by consumer who live within a 14-minute drive of and exclusion of the way in which storethose “i” two variables were measured means that it holds DUMREGION_GOOD dummy (binary) variable that takes on the value of 1 if store “i” is extreme value to the market analysislocated team in thefor U.S. Casa census Bonita’s region of datathe mid-Atlantic sets. So, region based or theon the western south-central region definition of the two variables that make up TOTAL_CUST_VALUE, our analysis team came to the conclusion that the greater the value of TOTAL_CUST_VALUE the greater the value of the Dansby and Johnston 44 people who live and/or work in the area of store “i”. Thus, this translates into these people having a higher probability of dining at Casa Bonita, and ultimately spending money there to increase the sales of each restaurant. Although the correlation coefficient between </p><p>TOTAL_CUST_VALUE sales is only about 0.15 (although quiet high for our sample), our team chose this variable because it puts a number on the value of those customers that are most likely be the largest proportion of sales for a Casa Bonita store. </p><p>BAD_POW_0_5RO and GOOD_POW_0_5RO were both added because there summations of either negatively correlated power center variables or positively correlated power center variables, respectively. Both were strongly correlated with sales compared to the top 56 candidate regressors (negatively and positively correlated), as we would expect since many of the power center variables were already correlated with sales and in our candidate regressor list. </p><p>By comparing Table 7 with Table 4 we can see that by adding negatively or positively correlated</p><p>(with sales) power center variables make the BAD_POW_0_5RO and GOOD_POW_0_5RO variables even more negatively and positively correlated with sales, respectively. From Table 4 it is apparent that all the power center variables correlation coefficient (positive or negative) with sales were centered around 0.15, while the BAD_POW_0_5RO and GOOD_POW_0_5RO variables are correlated with sales in an absolute value of about 0.25. This is because they are summations of top correlated variables with sales. Thus, by choosing these two top correlated regressors with sales our team has also reduced the potential for problem of micronumerosity because now we can have two variables that measure the same thing as five individual variables. </p><p>Both the SQFT and TOTAL_SEATS variables were chosen because they were both in the top three list of variables correlated with sales. By looking at Table 4 and Table 7, the reader can see that this is obvious with both variables having correlation coefficients with sales of about Dansby and Johnston 45</p><p>0.30. These are the two highest correlation coefficients in our 56 candidate regressor list. It makes sense that these variables were also chosen for model building on the basis of logic. The more square footage or the more seats a store has, the more customers it can serve and host, which his directly related to increases in sales. </p><p>DUMREGION_BAD and DUMREGION_GOOD were both included so that when our analysis team includes them in the models we can have estimates for predicting how much or less sales will be at particular store if it was in the regions included in the DUMREGION_BAD and DUMREGION _GOOD variables, respectively, versus being in the areas not included in the two variables. They were also included in our top twelve variables to be used in model building because they were both strongly correlated with sales compared to the other 56 candidate regressor list; having a correlation coefficient of about -0.31 and 0.32 for DUMREGION_BAD and DUMREGION_GOOD. </p><p>TOTAL_BAR_EXPEND_14TO was included because of the demand theory discussion above. If we can assume that we are in equilibrium, then the OLS regression for sales will be a quantity demanded function. According, to economic theory demand is a function of income. </p><p>So, our team chose to use TOTAL_BAR_EXPEND_14TO because it is directly related to income of consumers, and thus correlated with sales as well with a correlation coefficient of about 0.18. </p><p>CMHO_MEX_58514_14TO was chosen to reflect the overall positive relationship with sales when a particular Casa Bonita store shares are large percentage of customers with Mexican competitors. Only one other Mexican-competitor for this variable had a negative correlation coefficient with sales, although its p-value was about 0.42, so we cannot say that it does in fact have a correlation with sales, statistically speaking. CMHO_MEX_58514_14TO reflects the Dansby and Johnston 46 largest positive relationship with sales for these variables for Mexican competitors, with the highest correlation coefficient of the CMHO_MEX_COMPETITORID#_14TO variables being about 0.22. So, if included in the final model, we can estimate for the Casa Bonita executives how much more sales will increase (decreases) if the percentage of customers (with in a 14- miute drive time) with Mexican competitor C increases (decreases). Thus, being closer to this competitor might indeed help increase sales. Not many competitor variables that measured the percentage of consumers shared with a Casa Bonita store showed a negative relationship with sales for store “i”. Since demand theory also calls for the quantity demand being a function of substitutability of products. Mexican food is very common in the U.S. and thus is very easy to substitute between the large amount of differing Mexican restaurants. Our analysis team decided to include the variable for the non-Mexican competitor N, CMHO_NONMEX_55727_14TO, to account for the possible negative effect on sales if sharing customers with this competitor that decide to substitute away from Casa Bonita in lieu of non-Mexican competitor N. It was also included because it had quite a negative correlation coefficient with sales of almost -0.18. </p><p>The variable SimI_04005_14TO was included because it measures the degree of receptiveness to advertising of the residents who live within a 14-minute drive of store “i”. </p><p>Thus, since it is already strongly correlated with sales compared to the other regressors in our list of 55 candidate regressors with a correlation coefficient of 0.24, it was included in our list of 12 regressor variables for model building. It was also included in that list because if used in the final model we will be able to estimate the effect of advertising campaigns on sales for a particular Casa Bonita restaurant. Also included in the list of the twelve candidate regressors used for model building is the variable B29VV01_14TO which measures the median age of the household heads living within a 14-minute drive of store “i”. This variable was included because Dansby and Johnston 47</p><p> it was one of the variables correlated with sales more than a lot of variables in our 56 variables of</p><p> the candidate regressor list with a correlation coefficient of 0.23. It was also included because </p><p> the older the head of the household the more financially stable they are and the more likely they </p><p> are to have a family, all else constant. Thus, if they are more financially stable and have a family</p><p> not only are the more likely to be able to spend the money to eat at a Casa Bonita, they are also </p><p> likely to spend more because they will pay for the whole family eating. This a great reason as to </p><p> why this variable is positively correlated with sales and has a correlation coefficient of 0.23. </p><p>Next, our model building team then calculated the correlation coefficients between the 56</p><p> candidate regressors to analyze the potential problem of multicollinearity in our models. Table 9</p><p> below shows the correlation coefficients between the twelve candidate regressors chosen for </p><p> model building. Their correlations coefficients were very important for analysis because if any </p><p> of the variables were strongly correlated with each other, and chosen to be in the same model, </p><p> then there would possibly be problems associated with multicollinearity (discussed above). </p><p>Below the reader will see Table 8 which shows the correlation coefficients between the twelve </p><p>Table 9: Correlation Coefficients Between Top Candidate Regressors Used in Final Models Variable Names TOTAL_CUST_ BAD_POW_0_ GOOD_POW_ DUMREGIONvariablesSQFT to beSimI_04005 used forB29VV01_ modelCMHO_MEX buildingCMHO_NON andTOTAL_SEATS the p-valuesTOTAL_BAR_ DUMREGION VALUE 5RO 0_5RO _BAD _14TO 14TO _58514_14T MEX_55727_ EXPEND_14T _GOOD O 14TO O TOTAL_CUST_VALUE 1.0000 0.0457 0.0273 -0.0452 0.4098 0.1736 -0.1240 0.2005 0.3769 0.4212 0.5787 0.1054 (0.6322) (0.7753) for(0.6363) those coefficients,(<.0001) (0.0672) which(0.1928) are(0.0341) in parentheses(<.0001) below.(<.0001) (<.0001) (0.2686) BAD_POW_0_5RO 0.0457 1.0000 -0.1160 0.1669 0.1334 -0.0659 0.0673 0.1114 0.1897 0.0903 0.0188 -0.2634 (0.6322) (0.2232) (0.0787) (0.1607) (0.4903) (0.4807) (0.2423) (0.0452) (0.3438) (0.8442) (0.0050) GOOD_POW_0_5RO 0.0273 -0.1160 1.0000 The-0.2120 table is-0.1336 a correlation-0.0453 0.0812 matrix-0.0805 that shows-0.1313 the correlation-0.0932 0.0363 0.3697 (0.7753) (0.2232) (.0249) (0.1602) (0.6351) (0.3948) (0.3990) (0.1675) (0.3282) (0.7037) (<.0001) DUMREGION_BAD -0.0452 0.1669 -0.2120 1.0000 0.0185 -0.0579 -0.0982 0.1878 0.1839 -0.0613 -0.0008 -0.5733 (0.6363) (0.0787) (0.0249) (0.8469) (0.5442) (0.3030) (0.0473) (0.0523) (0.5209) (0.9934) (<.0001) SQFT 0.4098 0.1334 -0.1336between 0.0185the 1.0000 -0.0166 -0.0096 0.0894 0.0642 0.8946 0.1977 -0.0363 (<.0001) (0.1607) (0.1602) (0.8469) (0.8618) (0.9198) (0.3487) (0.5011) (<.0001) (0.0367) (0.7041) SimI_04005_14TO 0.1736 -0.0659 -0.0453 -0.0579 -0.0166 1.0000 0.1949 -0.0009 0.0169 0.0370 0.3834 0.1663 variables on the(0.0672) horizontal(0.4903) access(0.6351) the variables(0.5442) on(0.8618) the vertical(0.0395) axis. Under(0.9924) each(0.8595) correlation(0.6987) (<.0001) (0.0797) B29VV01_14TO -0.1240 0.0673 0.0812 -0.0982 -0.0096 0.1949 1.0000 0.0628 0.0673 -0.0798 0.0569 -0.0846 (0.1928) (0.4807) (0.3948) (0.3030) (0.9198) (0.0395) (0.5109) (0.4809) (0.4031) (0.5513) (0.3751) CMHO_MEX_58514_14TO 0.2005 0.1114 -0.0805 0.1878 0.0894 -0.0009 0.0628 1.0000 0.2054 0.0832 0.1486 -0.1146 coefficient between(0.0341) two(0.2423) variables(0.3990) is the p-value.(0.0473) (0.3487) Along(0.9924) the diagonal(0.5109) of the matrix(0.0298) are (0.3832)the values(0.1179) (0.2289) CMHO_NONMEX_55727_14TO 0.3769 0.1897 -0.1313 0.1839 0.0642 0.0169 0.0673 0.2054 1.0000 0.0576 0.5084 -0.1733 (<.0001) (0.0452) (0.1675) (0.0523) (0.5011) (0.8595) (0.4809) (0.0298) (0.5464) (<.0001) (0.0677) TOTAL_SEATSof 1 which indicates0.4212 perfect0.0903 correlation-0.0932 between-0.0613 the0.8946 variable0.0370 and-0.0798 itself.0.0832 Apparent0.0576 from1.0000 Table 90.2842 0.0189 (<.0001) (0.3438) (0.3282) (0.5209) (<.0001) (0.6987) (0.4031) (0.3832) (0.5464) (0.0024) (0.8430) TOTAL_BAR_EXPEND_14TO 0.5787 0.0188 0.0363 -0.0008 0.1977 0.3834 0.0569 0.1486 0.5084 0.2842 1.0000 0.1669 (<.0001) (0.8442) (0.7037) (0.9934) (0.0367) (<.0001) (0.5513) (0.1179) (<.0001) (0.0024) (0.0787) DUMREGION_GOODis that there are 0.1054a few variables-0.2634 that0.3697 could -0.5733pose a -0.0363problem0.1663 for multicollinearity-0.0846 -0.1146 -0.1733 in our model.0.0189 0.1669 1.0000 (0.2686) (0.0050) (<.0001) (<.0001) (0.7041) (0.0797) (0.3751) (0.2289) (0.0677) (0.8430) (0.0787) Correlation Coefficient Although a valuable variable, TOTAL_CUST_VALUE(p-value) poses a particularly great issue of Dansby and Johnston 48 multicollinearity. It has a strong weak multicollinearity correlation coefficient with the variables</p><p>SQFT, CMHO_NONMEX55727_14TO, and TOTAL_SEATS, but luckily not above the level of moderate multicollinearity correlation coefficient value of 0.5. Only one value is above the 0.5 level for moderate to strong multicollinearity. That variable is TOTAL_BAR_EXPEND_14TO with a correlation coefficient of about 0.58. This is expected because the more seats at store is related to a larger number of sales transactions, which in turn is related to expenditures of consumers in the area. Our analysis team paid particular attention to the relationship between to these variables during model building and selection. Another particular regressor relationship we need to notice is the one between SQFT and TOTAL_SEATS. The coefficient of 0.89 indicates very strong multicollinearity. This is expected because the square feet of the store is directly related to how many seats will be available in that restaurant. Thus, the two variables will often be used in model building, but always separately because they are virtually a measure of the same thing, store size. Another regressor relationship that poses a problem of moderate multicollinearity is the one between CMHO_NONMEX_55727_14TO and </p><p>TOTAL_BAR_EXPEND_14TO. With a correlation coefficient of about 0.51 we could possibly have problems such as opposite signs on the parameter estimates or inflated p-values that are indicative of multicollinearity. Unfortunately, both variables are very good in terms of large correlation coefficients with sales and called for by logic and demand theory so our team had to work hard to include these variables in our model all while avoiding the problems associated with multicollinearity. The correlation coefficient between TOTAL_CUST_VALUE and </p><p>TOTAL_SEATS, although below 0.5 is still about 0.42, so both variables should be used wisely when building our models. Although a very good variable for model building, </p><p>DUMREGION_GOOD is moderately correlated with DUMREGION_BAD with a correlation Dansby and Johnston 49 coefficient of about 0.37. DUMREGION_GOOD also has correlation coefficients between </p><p>BAD_POW_0_5RO and GOOD_POW_0_5RO that shows signs of high week multicollinearity with correlation coefficients between 0.26 and 0.36 in absolute terms. Thus, we must also be careful when using this variable in our models with the power center variables and the </p><p>DUMREGION_BAD variable. From the analysis of possible multicollinearity problems between our twelve candidate regressors for model building we have seen that multicollinearity should not be a large problem since none of the variables had correlation coefficients between each other above the threshold for severe multicollinearity (discussed above). Therefore, taking the potential moderate multicollinearity problems between our regressors into account, our analysis team constructed five models that strong predictors of sales for Casa Bonita. </p><p>Our analysis constructed numerous table and withered them down to the top five models. </p><p>These models labeled A-E are shown below in Table 10. The regressor variable names are on the vertical most-left column while their parameter estimates for each model are under the columns for those models. The p-values are in parentheses below the parameter estimates. It should be first noted that DUMREGION_GOOD was not used in of these five models to be used for model selection of the final model. This was because it was artificially deflating t-test values and over-inflating p-values, as well as signs on the estimates that are opposite of logic/theory which are signs of multicollinearity (to be discussed below in the model selection discussion). </p><p>As discussed above, we stated that our analysis team had to be careful with </p><p>DUMREGION_GOOD because it was moderately correlated BAD_POW_0_5RO and </p><p>GOOD_POW_0_5RO, and showed signs of moderately strong multicolloinearity with </p><p>DUMREGION_BAD. Our team could not get around the problems associated with variable and thus excluded it in our final five models. That left the analysis and model building team with Dansby and Johnston 50 eleven regressors for building these final five models. Model A was made using all those eleven regressors except SQFT and TOTAL_BAR_EXPEND_14TO. SQFT was not used because </p><p>TOTAL_SEATS was used and was strongly correlated with SQFT, as well as measuring virtually the same thing. Model A also takes into account how much less in terms of sales a store will be predicted to have if they were in a region considered bad (the south-Atlantic region or the mountain west region) compared to not being in those areas by estimating the slope parameter on the DUMREGION_BAD variable. TOTAL_BAR_EXPEND_14TO was not used because it had a correlation coefficient above 0.5 with TOTAL_CUST_VALUE and early models that used both these variables had many of the signs of multicollinearity (specifically both </p><p>TOTAL_CUST_VALUE had a negative sign on its slope parameter). Model B is Model A without TOTAL_SEATS. Our team made this model to see if TOTAL_SEATS really had a large effect on Model A’s goodness-of-fit statistics (which is did; to be discussed during model selection). Model C is like Model A, but does has SQFT instead of TOTAL_SEATS to see if </p><p>SQFT was a better independent variable for our models that TOTAL_SEATS. Additionally, </p><p>Model C does not contain TOTAL_CUST_VALUE because our analysis team wanted to know whether the variable truly did have an effect on predicting sales or not compared with its effect on the goodness-of-fit statistics for Model A. Model D is exactly like Model C, but uses </p><p>TOTAL_SEATS instead of SQFT. This model was made to compare the two variables parameter estimates for the models predicting sales. Model E is Model A, but uses SQFT instead of TOTAL_SEATS, as well as using TOTAL_BAR_EXPEND_14TO in lieu of </p><p>TOTAL_CUST_VALUE. TOTAL_BAR_EXPEND_14TO and TOTAL_CUST_VALUE were not used together in this model because signs of multicollinearity were popping up in early models in which both variables were used together (correlation coefficient between the two Dansby and Johnston 51 variables was above the 0.5 level with a value of almost 0.58). Model E was also made because our team wanted to have a final model that included TOTAL_BAR_EXPEND_14TO so that </p><p>Casa Bonita executives would have the option of seeing how much sales would increase </p><p>(decrease) if the total bar/restaurant type purchases for consumers in the area increased </p><p>(decreased). In order to pick between these five models that were all strong predictors of sales, our analysis team computed the goodness-of-fit statistics for each model and compared them to pick the final model for predicting the annual sales revenue for the four potential new Casa </p><p>Bonita restaurants. </p><p>Table 10: OLS Model Results Model Selection, Steps Parameter Estimate (p-value) In order to choose the best Model A Model B Model C Model D Model E Constant -3005807 -1569274 -3830636model-3032443 in terms of-3330466 accurately (0.0183) (0.2178) (0.0029) (0.0162) (0.0143) TOTAL_CUST_VALUE 0.00577 0.05353 prediction sales our model - - - (0.8491) (0.0683) BAD_POW_0_5RO -337519 -293571 -355006selection-338083 team calculated-345197 and (0.0080) (0.0281) (0.0044) (0.0076) (0.0056) GOOD_POW_0_5RO 393994 315292 428795compared398343 the goodness-of-fit399213 (0.0111) (0.0515) (0.0044) (0.0091) (0.0088) DUMREGION_BAD -316396 -345472 -356991statistics-319061 for the models-347443 as well (0.0133) (0.0108) (0.00390) (0.0117) (0.0050) SQFT 311.79881 293.50771 - - evaluating- the number of (<.0001) (<.0001) SimI_04005_14TO 25382 23268 29070significant25874 slope parameters22936 in (0.0276) (0.0558) (0.0081) (0.0206) (0.0610) a B29VV01_14TO 38320 38123 31684model, and37659 whether or33187 not these (0.0178) (0.0260) (0.0376) (0.0166) (0.0301) parametersCMHO_MEX_58514_14TO have the logically correct11770 signs. Additionally,11878 we11979 took into account11870 models11723 that are (0.0004) (0.0008) (0.0002) (0.0003) (0.0003) parsimonious,CMHO_NONMEX_55727_14TO or relatively simple.-3735.07619 The goodness-of-fit-4736.26519 statistics-3473.75680 are measures-3586.97437 used-4711.00615 in (0.0494) (0.0181) (0.0394) (0.0375) (0.0209) TOTAL_SEATS 5107.90217 5218.65418 comparing the value of one model to others are the- general F-test,- R2, adjusted R2 (), and- in- (0.0003) (<.0001) TOTAL_BAR_EXPEND_14TO 0.00111 - - - - (0.2736) Dansby and Johnston 52 sample and out-of-sample Mean Absolute Percentage Error (MAPE). Also, our team looked at the significance and magnitudes of the parameter estimates in each model. </p><p>From the discussion on micronumerosity it is apparent that our models should be parsimonious. Additionally, the more parameters estimated in OLS model the more data collection must take place and this can often be very expensive. By looking at the five models in table it is readily apparent that micronumerosity should not be a problem, and with only a maximum of 10 independent variables (including the constant) in our five models we also have a relatively simple model compared to the large 112 sample data set for Casa Bonita stores. </p><p>Another requirement for the final model is that it must have signs on the parameters estimates that match the correlation coefficient between that regressor estimated and sales. The true sign is determined by the correlation coefficient and any counterintuitive sign the model is likely due to multicollinearity or human error. Obvious from Tables 4,6, and 9 is that all the signs for the parameter estimates for the independent variables all adhere to the signs of their correlation coefficient with sales. Thus, this was not concern during model selection and will not be discussed below in the results of our model selection. </p><p>In order to explain how well the regressors in the model explain sales, we must have a measure of whether or not overall the variables do explain sales. The general F-test measures the overall strength of the independent variables as a whole in predicting sales. Statistically, the general F-test tests to see if at least one of the estimated coefficients is not equal to zero. A high </p><p>F-test value and a low p-value for the F-test indicate the F-test is significant, and overall, the variables are strong at explaining sales. </p><p>R2 and each measure the percentage of variation in sales that can be explained by the independent, explanatory variables. accounts for the number of parameters used in estimating Dansby and Johnston 53 the model and can be compared across models with differing numbers of estimated coefficients with the formula . Also, unlike R2, does not always increase in value when a variable is added to the OLS estimation, and can sometimes even decrease. The higher is the better fit the variables are in explaining sales, with taking on the value in the range 0≤≤1. Since R2 does not take into the number of variables, we cannot use this statistics to compare the fit of models with differing numbers of regressors, so we must use to compare the fit of models in explaining sales across models. Thus, will be used when comparing the power of explaining sales by the independent variables across the models rather than R2. </p><p>Two other powerful fit statistics for the models are the out-of-sample Mean Absolute </p><p>Percentage Error (MAPE) and the in-sample MAPE. MAPE is used to see how well the model predicts sales. Four potential Casa Bonita stores were already held out of the data set in order to formulate each model’s out-of-sample MAPE to see whether or not the model is good predictor of sales. All of the 112 observations in our sample used for model building were used to calculate in-sample MAPE. The MAPE of a model is calculated by using the predicted sales of the observation from a model, taking the absolute value of the difference between this predicted value and the real value of sales, and dividing by the real value of sales. The formula for in- sample or out-of-sample MAPE is as follows</p><p>3.) </p><p>We then add these MAPE values for the four holdout stores or the 112 stores in our sample and average their values to arrive at the final out-of-sample MAPE and in-sample MAPE, respectively, to compare across models. Ideally, we want the MAPE to be as low possible, and a Dansby and Johnston 54 good rule of thumb is to get a MAPE with a value below 0.30, or 30%. A low MAPE means we have less error in predicting sales. </p><p>The next step in being about to compare models is to observe the number of individual slope estimates by looking at their t-test and p-values. A large t-test value and p-value under </p><p>0.10 indicates significance of the individual slope coefficient and provides a measure of true existence in explaining sales. However, if an individual estimate is not significant, but economic theory states it should be in the model, the variable should still be included in the model; especially if its estimate has a meaningful magnitude and has the true sign. For the variable to be relevant it should have a meaningful magnitude. If, for example, GOOD_POW_0_5RO had a parameter estimate of 0.000000001, it would mean that there would have to be 100,000,000 power centers 4, 20, and/or 31 within a half-mile radius of a Casa Bonita store to increase sales by a dollar. Obviously, this would prove that this variable would be useless in estimating sales and would not call for that variable to be included in the final model. Now that we have reviewed the goodness-of-fit measures to compare across models with differing numbers of explanatory variables, we can review the results of model selection and show which of the five final models were selected to predict sales for the four potential new Casa Bonita stores. </p><p>Model Selection, Results</p><p>In order to select the final model to be used for predicting sales for Casa Bonita stores our team calculated and analyzed the number of significant slope parameter estimates (by having a p- value of 0.10 or below), as well as the goodness-of-fit statistics and other measures. Below the reader of this report will see Table 11 which shows the values of the fit measures for each of the five models. In order to select the final model we compared the fit statistics across models to Dansby and Johnston 55 arrive at the best model for predicting sales. Unfortunately, as we discussed above, R2 does not take into account the numb and cannot be used to compare the measure of explanation of sales by regressors across models with differing numbers of regressors. Thus, our model selection team chose to use only to compare fit across our five models. Dansby and Johnston 56</p><p>The reader will notice fromTable Table 11: Goodness-of-Fit 11 that Model StatisticsB has the Model A Model B Model C Model D Model E #lowest of Parameter . This Estimatesproved to with our model selection and analysis team that Correct Signs Out of Total # of 9/9 8/8 8/8 8/8 8/9 Parametersin fact the size of the store does in fact explain a large portion of General F-Test 8.75 7.20 10.93 9.93 9.87 sales and should be required in the final model. Thus, our team (<.0001) (<.0001) (<.0001) (<.0001) (<.0001) R-Squared 0.4357 0.3588 0.4592 0.4355 0.4656 decided to not use Model B as the final model for predicting Casa Adjusted R-Squared 0.3859 0.3090 0.4172 0.3916 0.4184 out-of-sampleBonita sales. MAPEAlthough Model C had0.1701 the second0.1595 highest , our0.1934 team did not0.1681 select this model0.2038 in-sample MAPE 0.1708 0.1845 0.1690 0.1737 0.1664 because it did not have a variable that measured the income of consumers or the likelihood of </p><p> expenditure at a Casa Bonita store (TOTAL_BAR_EXPEND_14TO and </p><p>TOTAL_CUST_VALUE). This is a strong reason as to why the out-of-sample MAPE is the </p><p> second largest out of the five models; Model C has a large amount of error possibly caused by </p><p> not using TOTAL_BAR_EXPEND_14TO and/or TOTAL_CUST_VALUE. As the reader can </p><p> recall, Model D is like Model C, but uses TOTAL_SEATS instead of SQFT. Model D was also </p><p> not chosen on the basis of concluding that TOTAL_BAR_EXPEND_14TO and/or </p><p>TOTAL_CUST_VALUE should be in the model because economic theory concludes that </p><p> demand (sales, if we can safely assume we are equilibrium) is partly a function of income. </p><p>Expenditure (TOTAL_BAR_EXPEND_14TO) and the likelihood that customers will go to a </p><p>Casa Bonita and spend money there (TOTAL_CUST_VALUE) are thus function of income and </p><p> should be included in the final model. This is possibly a reason as to why Model D’s in-sample </p><p>MAPE was the third highest out of the group of five, and thus was not be selected as the final </p><p> model. </p><p>In the end our model selection team had to choose to pick either Model A or Model E. </p><p>Obvious from Table 11, Model A’s out-of-sample MAPE is almost three and half percentage </p><p> points better than Model E’s. Both models also have very significant general F-test with p- Dansby and Johnston 57 values for both being well below the 0.10 level. On one hand the for Model E is the highest out of the five models, while on the other hand Model E has eight significant regressors out of the nine independent variables used while all nine of Model A’s independent variables were significant. In Model A we used TOTAL_SEATS to measure store capacity, while Model E used SQFT. Our team believed that TOTAL_SEATS was more related to sales for each store because of the possibility of having a large store in terms of square feet that has a smaller amount of seats for customers than a small store in terms of square feet with a larger amount of seats. In the end, our team realized that Casa Bonita ultimately makes it money from where the consumers sit, their lunch/dinner seats. Thus, in the end our selection team chose Model A to predict sales for the potential Casa Bonita stores. With TOTAL_CUST_VALUE has a one of the regressors, </p><p>Model A has a variable to measure the loyalty of customers in the area and the likelihood that they will spend their money at Casa Bonita. Our team would have liked to include </p><p>TOTAL_BAR_EXPEND_14TO, but its moderately strong correlations with SimI_04005_14TO and CMHO_NONMEX_55727_14TO were likely reasons it was the only regressor out of our five models to consistently show an insignificant p-value (0.2736 in Model E). Also, we can safely assume that TOTAL_CUST_VALUE is a close measure of </p><p>TOTAL_BAR_EXPEND_14TO because it is strong correlation coefficient between the two with a value of 0.5787. Model A is a strong model with all of is regressors significant, a high of </p><p>0.3859, the third lowest out-of-sample MAPE, as well as the third lowest in-sample MAPE, very significant general F-test value, and including the two strong variables for predicting sales, </p><p>TOTAL_CUST_VALUE and TOTAL_SEATS. Thus, our final model is Model A where sales is predicted by Dansby and Johnston 58</p><p>4.) SALES = –3005807 + 0.00577×(TOTAL_CUST_VALUE) – 337519× (BAD_POW_0_5RO) + </p><p>393994× (GOOD_POW_0_5RO) – 316396(DUMREGION_GOOD) + 25382×(SimI_04005_14TO) + </p><p>38320(B29VV01_14TO) + 11770×(CMHO_MEX_58514_14TO) – </p><p>3735.07619×(CMHO_NONMEX_55727_14TO) + 5107.90217×( (TOTAL_SEATS) Dansby and Johnston 59</p><p>The estimated slope parameter for each regressor has a significant meaning when predicting sales. All else constant, If TOTAL_CUST_VALUE increases by one, the model predicts that one average sales for store “i” will increase by about half a cent. However, the variable has a range of about 400,000 to over 11 million, so it is more likely that if this variable increased it would do so by a large amount. So for example, if TOTAL_CUST_VALUE increased by 100,000, then sales, on average, would be predicted to increase by $500, all else constant. Additionally, all else constant, if store “i” is located within a half-mile of one more power center 13 or 26, then sales, on average, is predicted to decrease by $337,519, all else constant. </p><p>This is a substantial decrease of money, so Casa Bonita should be weary of locating any potential stores very close to power centers 13 and 26. On the other hand, if store “i” is located within a half-mile radius of one more of power center 4, 20, or 31 then sales is predicted to increase, on average, by about $393,994, all else constant. This is substantial increase in sales, so Casa </p><p>Bonita executives should try and locate near these power centers. The sales forecast model also predicts that one average sales will be about $316,396 less per year if store “i” is located in the south-Atlantic region of the mountain west region of the United States than if it were not, all else constant. Thus, Casa Bonita executives should be wary of locating in those two regions or try and increase brand exposure and acceptance in those areas if it plans to locate there. Also, if </p><p>SimI_04005_14TO increase by one unit, the model predicts that on average sales will increase by $25,382, all else constant. This estimate is very valuable because it can be used in determining the value of marketing the Casa Bonita brand in the area. Furthermore, if the median age of household heads in the 14-minute drive time area of store “i”, Model A predicts Dansby and Johnston 60 that sales will increase on average by $38,320, all else constant. Additionally, one on hand if store “i” increases the percentage of customers shared with Mexican competitor C within a 14- minute drive by only one percentage point the model predicts that on average sales will increase by almost $12,000 (exact, $11,770), all else constant. While on the other hand if store “i” increases the percentage of customers shared with non-Mexican competitor N by only one percentage point, the model predicts that on average sales per year will decrease by $3,735.08, all else constant. So, Casa Bonita should try and avoid being close to non-Mexican competitor </p><p>N, but try and locate near Mexican competitor C. Finally, if the number of total seats in restaurant “i” increases by one sales is expected to , on average, increase by $5,107.90 per year, all else constant. This shows that the number of seats in a particular Casa Bonita store can have a large positive impact on sales revenue each year. Now that we have selected out final sales forecast model and discussed the interpretation of each estimate coefficient we can finally predict sales for the four potential Casa Bonita restaurant. Concluding, we can make recommendations based on those predictions as to whether the stores should be opened or not. </p><p>Predicted Sales and Recommendations</p><p>Based on the chosen regression model, sales for Casa Bonita’s five potential locations can be accurately predicted. Data on the independent variables from the five stores and estimated total annual revenue, from Model B, for each store are as follows:</p><p>STORE 20415038; TOTAL_SEATS = 290, TOTAL_CUST_VALUE = 4969545.517, </p><p>BAD_POW_0_5RO = 0, GOOD_POW_0_5RO = 0, DUMREGION_BAD = 1, Dansby and Johnston 61</p><p>SIMI_04005_14TO = 92.1887, B29VV01_14TO = 45, CMHO_MEX_58514_14TO = 0, </p><p>CMHO_NONMEX_55727_14TO = 53.16973415</p><p>SALES = -3005807 + 5107.90217(290) + 0.00577(4969545.517) – 337519(0) + 393994(0) – </p><p>316396(1) + 25382(92.1887) + 38320(45) + 11770(0) – 3735.07619(53.16973415) = $2,369,899</p><p>STORE 20415053; TOTAL_SEATS = 290, TOTAL_CUST_VALUE = 2675368.587, </p><p>BAD_POW_0_5RO = 0, GOOD_POW_0_5RO = 0, DUMREGION_BAD = 1, </p><p>SIMI_04005_14TO = 99.3221, B29VV01_14TO = 47, CMHO_MEX_58514_14TO = </p><p>5.274476014, CMHO_NONMEX_55727_14TO = 0</p><p>SALES = -3005807 + 5107.90217(290) + 0.00577(2675368.587) – 337519(0) + 393994(0) – </p><p>316396(1) + 25382(99.3221) + 38320(47) + 11770(5.274476014) – 3735.07619(0) = $2,875,036</p><p>STORE 20415089; TOTAL_SEATS = 290, TOTAL_CUST_VALUE = 6396467.034, </p><p>BAD_POW_0_5RO = 0, GOOD_POW_0_5RO = 0, DUMREGION_BAD = 0, </p><p>SIMI_04005_14TO = 98.24, B29VV01_14TO = 53, CMHO_MEX_58514_14TO = 0, </p><p>CMHO_NONMEX_55727_14TO = 23.00703775</p><p>SALES = -3005807 + 5107.90217(290) + 0.00577(6396467.034) – 337519(0) + 393994(0) – </p><p>316396(0) + 25382(98.24) + 38320(53) + 11770(0) – 3735.07619(23.00703775) = $2,950,947</p><p>STORE 20415111; TOTAL_SEATS = 267, TOTAL_CUST_VALUE = 5498374.356, </p><p>BAD_POW_0_5RO = 1, GOOD_POW_0_5RO = 0, DUMREGION_BAD = 0, Dansby and Johnston 62</p><p>SIMI_04005_14TO = 102.3981, B29VV01_14TO = 51, CMHO_MEX_58514_14TO = 0, </p><p>CMHO_NONMEX_55727_14TO = 0</p><p>SALES = -3005807 + 5107.90217(267) + 0.00577(5498374.356) – 337519(1) + 393994(0) – </p><p>316396(0) + 25382(102.3981) + 38320(51) + 11770(0) – 3735.07619(0) = $2,605,598</p><p>STORE 20415141; TOTAL_SEATS = 267, TOTAL_CUST_VALUE = 1684061.915, </p><p>BAD_POW_0_5RO = 0, GOOD_POW_0_5RO = 0, DUMREGION_BAD = 0, </p><p>SIMI_04005_14TO = 97.4326, B29VV01_14TO = 49, CMHO_MEX_58514_14TO = 0, </p><p>CMHO_NONMEX_55727_14TO = 0</p><p>SALES = -3005807 + 5107.90217(267) + 0.00577(1684061.915) – 337519(0) + 393994(0) – </p><p>316396(0) + 25382(97.4326) + 38320(49) + 11770(0) – 3735.07619(0) = $2,718,434</p><p>The average sales revenue for Casa Bonita across the nation is $2,620,465, while the standard deviation of revenue for Casa Bonita is $697,152. The minimum sales revenue during </p><p>2012 for any Casa Bonita store was $1,317,062, with maximum revenue of $4,489,364. Stores </p><p>20415089 and 20415053 sales are somewhat higher than the national average for Casa Bonita with predicted revenues of $2,950,947 and $2,875,036 respectively. Store 20415141 has a revenue potential of $2,718,434, right at the peak of the upper bound. The sales revenue for a potential store location will be seen as high if it is above the upper bound of the 95% confidence interval of sales revenue. A confidence interval gives an estimated range of values of sales revenue, the estimated range being calculated from the sample data of the estimate. In other words, it can be said that 95% of all of the sales in the sample fall within the range from lower Dansby and Johnston 63 bound, $2,491,350, and the upper bound, $2,749,579. The middle of this range is the mean, or average, $2,620,465. The formula for calculating a 95% confidence interval is shown below:</p><p>5.)</p><p>Where, = Average sales revenue for Casa Bonita in 2012 ($2,620,465)</p><p>S = Standard deviation of sales revenue ($697,152)</p><p> n = Sample size (112)</p><p>The lower bound is the formula on the left side of the average itself. The upper bound is the formula on the right side of the average itself. </p><p>After calculating the upper and lower bounds for the predicted sales for each of the five stores, it can be said with a 95% confidence that those predicted sales will fall within a given range. For store 20415308, that range is from $2,268,716 to $2,471,081. For store 20415053, that range is $2,773,853 to $2,976,218. For store 20415089, that range is $2,849,764 to $3,051,129. </p><p>For store 20415111, the range of sales revenue with a 95% confidence is $2,504,415 to </p><p>$2,706,780. For store 20415141, that range is $2,617,251 to $2,819,616. Again, these CI’s were calculated from the equation above; with “X bar” being the predicted sales the predicted restaurant, the standard deviation of 546336, and the 112 sample size. Dansby and Johnston 64</p><p>After looking at the confidence interval for sales revenue, it can be seen that store </p><p>20415089 and 20415053 are above the upper bound amount, with store 20415141 being very close to the upper bound. A major difference between the restaurants above the upper bound </p><p>(201415089 and 20415053) and store 20415141 is the number of seats in the restaurants. Store </p><p>20415141 only has 267 seats in its restaurant. Stores 20415089 and 2415053 have 290 seats in their restaurants. On average, stores with 290 seats make $215,252.48 more than stores with 267 seats. That is $9,358.80 per seat. Subtracting 23 seats from stores 20415089 and 20415053 to make them have 267 seats in the restaurants makes the sales revenue predictions more comparable with store 20415141’s predicted sales revenue, because now they both have the same number of seats. Still, with store 20415089 having 267 seats, its predicted revenue is $2,735,694.</p><p>This is still very close to the upper bound limit of $2,749,579. With only 263 seats, store </p><p>20415053 has predicted revenue of $2659783. This is only slightly above the national sales revenue average. It is safe to say that stores 20415089, 20415053 and 20415141 have a relatively high revenue potential compared to the sample data. Store 2015038 has predicted sales below the lower bound of $2,491,350, so this restaurant is seen to have relatively low sales revenue compared to other sample data restaurants. Store 20415111 has potential sales a little below the average sales revenue. It would be recommended not to open this store if Casa Bonita is looking for “high” revenue potential as compared to other sample data stores.</p><p>Conclusion</p><p>It is essential to have the correct variables in a regression model for good estimates of the dependent variable. The 141 variables were sifted through by looking at various pre-model analysis methods like summary statistics correlation coefficients and p-values. Through the Dansby and Johnston 65 research it can be seen that there are a number of factors that affect the sales revenue of a store. </p><p>The negative signs of DUMREGION_BAD, BAD_POW, and CMHO_NONMEX_55727_14TO indicate that these factors will decrease potential sales revenue. If non-Mexican food restaurant </p><p>55727 is located near a new Casa Bonita location, is may add to a decrease in sales revenue for that Casa Bonita. It could broadly be stated that for restaurants located in the Southern Atlantic and Mountain West region, sales revenue will not be as high. There is a positive influence on a store’s sales when observing an increase in TOTAL_SEATS, TOTAL_CUST_VALUE, </p><p>GOOD_POW_0_5RO, SIMI_04005_14TO, B29VV01_14TO, and CMHO_MEX_58514_14TO.</p><p>It may be a wise decision to consider if Mexican restaurant 58514 is located near a new Casa </p><p>Bonita. That could potentially lead to a higher revenue location.</p><p>Based on the total sales revenue calculated for the five potential restaurant locations, a recommendation that Casa Bonita open up stores 20415089, 20415053 and 20415141 is made; if they want to make high sales revenue. A last second side note is to possibly consider the amount of seats in store 20415141. If that could be changed to 290 seats instead of 267 seats, that revenue potential for store 20415141 could increase on average by $215,252.48. This would make store 20415141 the second highest predicted sales revenue of the five restaurant locations. </p><p>Through thorough linear modeling techniques great things can happen. In this case, Casa Bonita is going to open up three restaurants. The great thing is all the money they are going to receive from customers. Dansby and Johnston 66</p><p>Appendix</p><p>ODS HTML CLOSE ; ODS HTML ; DM 'LOG; CLEAR; OUTPUT; CLEAR;' ; TITLE ' DAVID DANSBY AND RYAN JOHNSTON '; TITLE2 'CASA BONITA HW2'; TITLE3 'ECON5645'; RUN;</p><p>PROC IMPORT DATAFILE = 'E:\BUXTON\CASA1.CSV' OUT=CASA1 DBMS=CSV REPLACE; GETNAMES = YES; RUN;</p><p>PROC IMPORT DATAFILE = 'E:\BUXTON\CASA2.CSV' OUT= CASA2 DBMS=CSV REPLACE; Dansby and Johnston 67</p><p>GETNAMES = YES; RUN;</p><p>PROC SORT DATA = CASA1; BY STORE_ID; RUN;</p><p>PROC SORT DATA = CASA2; BY STORE_ID; RUN;</p><p>DATA CASABONITA; SET CASA1; SET CASA2; RUN;</p><p>DATA CASABONITANEW; SET CASABONITA; DUMBUDS_RURAL=0; IF BUDS="1" THEN DUMBUDS_RURAL=1; DUMBUDS_INTOWN=0; IF BUDS = "2" THEN DUMBUDS_INTOWN=1; DUMBUDS_SUB=0; IF BUDS = "3" THEN DUMBUDS_SUB=1; DUMBUDS_METRO=0; IF BUDS = "4" THEN DUMBUDS_METRO=1; DUMBUDS_URBAN=0; IF BUDS = "5" THEN DUMBUDS_URBAN=1;</p><p>DUMREGION_SA=0; IF REGION = "SA" THEN DUMREGION_SA=1; DUMREGION_WM=0; IF REGION = "WM" THEN DUMREGION_WM=1; DUMREGION_WNC=0; IF REGION = "WNC" THEN DUMREGION_WNC=1; DUMREGION_ENC=0; IF REGION = "ENC" THEN DUMREGION_ENC=1; DUMREGION_WSC=0; IF REGION = "WSC" THEN DUMREGION_WSC=1; DUMREGION_ESC=0; IF REGION = "ESC" THEN DUMREGION_ESC=1; DUMREGION_NE=0; IF REGION = "NE" THEN DUMREGION_NE=1; DUMREGION_MA=0; IF REGION = "MA" THEN DUMREGION_MA=1;</p><p>SALESBYSQFT = SALES/SQFT; TOTAL_SEATS = BAR_SEATS + PATIO_SEATS + DINING_SEATS; SALES_BY_TOTAL_SEATS = SALES/TOTAL_SEATS;</p><p>TOTAL_BAR_EXPEND_14TO = X01V079_14TO + X01V080_14TO + X01V084_14TO + X01V088_14TO + X01V091_14TO; TOTAL_EXPEND_14TO = X01V079_14TO + X01V080_14TO + X01V084_14TO + X01V088_14TO + X01V091_14TO + X02V072_14TO + X02V071_14TO;</p><p>PER_CAP_BAR_EXPEND_14TO = TOTAL_BAR_EXPEND_14TO/POP_14TO;</p><p>TOTAL_CUST_VALUE = CUST_VALUE + LUNCH_CUST_VALUE;</p><p>BAD_POW_0_5RO = CT_POW_55491_0_5RO + CT_POW_54432_0_5RO; GOOD_POW_0_5RO = CT_POW_56128_0_5RO + CT_POW_55095_0_5RO + CT_POW_53889_0_5RO;</p><p>DUMREGION_GOOD = DUMREGION_MA + DUMREGION_WSC; DUMREGION_BAD = DUMREGION_SA + DUMREGION_WM; Dansby and Johnston 68</p><p>/* convert character to numeric */ NUMBER_REGION = input(REGION,3.0); drop REGION; rename NUMBER_REGION=REGION; </p><p>RUN;</p><p>PROC EXPORT DATA= CASABONITANEW OUTFILE = 'E:\BUXTON\CASABONITANEW.CSV' DBMS = CSV REPLACE; RUN;</p><p>PROC PRINT; RUN;</p><p>PROC MEANS N MEAN MEDIAN STD MIN MAX CV; RUN;</p><p>PROC MEANS N MEAN MEDIAN STD MIN MAX CV; VAR DUMBUDS_RURAL DUMBUDS_INTOWN DUMBUDS_SUB DUMBUDS_METRO DUMBUDS_URBAN DUMREGION_SA DUMREGION_WM DUMREGION_WNC DUMREGION_ENC DUMREGION_WSC DUMREGION_ESC DUMREGION_NE DUMREGION_MA ; RUN;</p><p>PROC MEANS N MEAN MEDIAN STD MIN MAX CV; VAR SALES TOTAL_SEATS SQFT DUMREGION_MA D02V007_14TO D02V024_14TO B14V002_14TO DINING_SEATS EB27V001_14TO BAR_SEATS SIMI_04005_14TO PATIO_SEATS B29VV01_14TO CMHO_MEX_62094_14TO CMHO_MEX_58514_14TO EA07V010_14TO D07V002_14TO CM_MEX_60647_1RO DUMREGION_WM CT_POW_55491_0_5RO X01V091_14TO X01V080_14TO X01V084_14TO EA04V002_14TO CMHO_NONMEX_55727_14TO X01V088_14TO D02V001_14TO X01V079_14TO CT_POW_53889_0_5RO B03V001_14TO CMHO_MEX_62081_14TO POP_14TO D02VBASE_14TO CMHO_MEX_58508_14TO WMOSTOT_14TO CT_POW_55095_0_5RO B12V004_14TO EA04V003_14TO D07V003_14TO A12VBASE_14TO D02V018_14TO C01V012_14TO DUMREGION_WSC B16VBASE_14TO CM_NONMEX_54598_1RO D07VBASE_14TO CT_POW_54432_0_5RO CUST_VALUE CT_POW_56128_0_5RO A01V001_14TO LUNCH_CUST_VALUE ; RUN; Dansby and Johnston 69</p><p>PROC MEANS N MEAN MEDIAN STD MIN MAX CV; VAR BAD_POW_0_5RO GOOD_POW_0_5RO TOTAL_CUST_VALUE TOTAL_SEATS TOTAL_BAR_EXPEND_14TO DUMREGION_BAD DUMREGION_GOOD DUMREGION_SA; RUN;</p><p>PROC CORR; VAR SALES; WITH _ALL_; RUN;</p><p>PROC CORR BEST=140; VAR _ALL_; WITH SALES; RUN;</p><p>PROC CORR BEST=95; VAR _ALL_; WITH SALESBYSQFT; RUN;</p><p>PROC CORR BEST=95; VAR _ALL_; WITH SALES_BY_TOTAL_SEATS; RUN; proc corr ; var TOTAL_SEATS EB27V001_14TO SIMI_04005_14TO EC15V001_14TO TOTAL_CUST_VALUE WMOSTOT_14TO; run; PROC CORR; VAR TOTAL_SEATS EB27V001_14TO SIMI_04005_14TO D02V006_14TO D02V023_14TO EA07V010_14TO TOTAL_EXPEND_14TO TOTAL_CUST_VALUE; RUN;</p><p>PROC CORR; VAR SALES; WITH TOTAL_SEATS SQFT DUMREGION_MA D02V007_14TO D02V024_14TO B14V002_14TO DINING_SEATS EB27V001_14TO BAR_SEATS SIMI_04005_14TO PATIO_SEATS B29VV01_14TO CMHO_MEX_62094_14TO CMHO_MEX_58514_14TO EA07V010_14TO D07V002_14TO CM_MEX_60647_1RO DUMREGION_WM CT_POW_55491_0_5RO X01V091_14TO X01V080_14TO X01V084_14TO EA04V002_14TO CMHO_NONMEX_55727_14TO X01V088_14TO D02V001_14TO X01V079_14TO CT_POW_53889_0_5RO B03V001_14TO CMHO_MEX_62081_14TO POP_14TO D02VBASE_14TO CMHO_MEX_58508_14TO WMOSTOT_14TO CT_POW_55095_0_5RO B12V004_14TO EA04V003_14TO D07V003_14TO A12VBASE_14TO D02V018_14TO C01V012_14TO DUMREGION_WSC B16VBASE_14TO CM_NONMEX_54598_1RO D07VBASE_14TO CT_POW_54432_0_5RO CUST_VALUE CT_POW_56128_0_5RO A01V001_14TO LUNCH_CUST_VALUE ; RUN;</p><p>PROC CORR; VAR SALES; Dansby and Johnston 70</p><p>WITH TOTAL_SEATS TOTAL_BAR_EXPEND_14TO TOTAL_CUST_VALUE BAD_POW_0_5RO GOOD_POW_0_5RO DUMREGION_GOOD DUMREGION_BAD DUMREGION_SA; RUN;</p><p>PROC SGSCATTER DATA = CASABONITANEW; title 'Scatter Plot 1'; MATRIX SALES TOTAL_SEATS SQFT DUMREGION_MA D02V007_14TO D02V024_14TO ; RUN;</p><p>PROC SGSCATTER DATA = CASABONITANEW; title 'Scatter Plot 2'; MATRIX SALES B29VV01_14TO CMHO_MEX_62094_14TO CMHO_MEX_58514_14TO EA07V010_14TO D07V002_14TO ; RUN;</p><p>PROC SGSCATTER DATA = CASABONITANEW; title 'Scatter Plot 3'; MATRIX SALES X01V084_14TO EA04V002_14TO CMHO_NONMEX_55727_14TO X01V088_14TO D02V001_14TO ; RUN;</p><p>PROC SGSCATTER DATA = CASABONITANEW; title 'Scatter Plot 4'; MATRIX SALES D02VBASE_14TO CMHO_MEX_58508_14TO WMOSTOT_14TO CT_POW_55095_0_5RO B12V004_14TO ; RUN;</p><p>PROC SGSCATTER DATA = CASABONITANEW; title 'Scatter Plot 5'; MATRIX SALES DUMREGION_WSC B16VBASE_14TO CM_NONMEX_54598_1RO D07VBASE_14TO CT_POW_54432_0_5RO; RUN;</p><p>PROC SGSCATTER DATA = CASABONITANEW; title 'Scatter Plot 6'; MATRIX SALES CUST_VALUE CT_POW_56128_0_5RO A01V001_14TO LUNCH_CUST_VALUE SIMI_04005_14TO; RUN;</p><p>PROC SGSCATTER DATA = CASABONITANEW; title 'Scatter Plot 7'; MATRIX SALES EA04V003_14TO D07V003_14TO A12VBASE_14TO D02V018_14TO C01V012_14TO; RUN;</p><p>PROC SGSCATTER DATA = CASABONITANEW; title 'Scatter Plot 8'; MATRIX SALES X01V079_14TO CT_POW_53889_0_5RO B03V001_14TO CMHO_MEX_62081_14TO POP_14TO; RUN;</p><p>PROC SGSCATTER DATA = CASABONITANEW; title 'Scatter Plot 9'; Dansby and Johnston 71</p><p>MATRIX SALES CM_MEX_60647_1RO DUMREGION_WM CT_POW_55491_0_5RO X01V091_14TO X01V080_14TO; RUN;</p><p>PROC SGSCATTER DATA = CASABONITANEW; title 'Scatter Plot 10'; MATRIX SALES B14V002_14TO DINING_SEATS EB27V001_14TO BAR_SEATS PATIO_SEATS; RUN;</p><p>PROC CORR; VAR SALES A01V001_14TO A12VBASE_14TO B03V001_14TO B12V004_14TO B14V002_14TO B16VBASE_14TO B29VV01_14TO BAR_SEATS C01V012_14TO CM_MEX_60647_1RO CM_NONMEX_54598_1RO CMHO_MEX_58508_14TO CMHO_MEX_58514_14TO CMHO_MEX_62081_14TO CMHO_MEX_62094_14TO CMHO_NONMEX_55727_14TO CT_POW_53889_0_5RO CT_POW_54432_0_5RO CT_POW_55095_0_5RO CT_POW_55491_0_5RO CT_POW_56128_0_5RO CUST_VALUE D02V001_14TO D02V007_14TO D02V018_14TO D02V024_14TO D02VBASE_14TO D07V002_14TO D07V003_14TO D07VBASE_14TO DINING_SEATS DUMREGION_MA DUMREGION_WM DUMREGION_WSC EA04V002_14TO EA04V003_14TO EA07V010_14TO EB27V001_14TO LUNCH_CUST_VALUE PATIO_SEATS POP_14TO SIMI_04005_14TO SQFT WMOSTOT_14TO X01V079_14TO Dansby and Johnston 72</p><p>X01V080_14TO X01V084_14TO X01V088_14TO X01V091_14TO TOTAL_BAR_EXPEND_14TO TOTAL_EXPEND_14TO TOTAL_CUST_VALUE PER_CAP_BAR_EXPEND_14TO ; run;</p><p>PROC CORR; VAR TOTAL_CUST_VALUE BAD_POW_0_5RO GOOD_POW_0_5RO DUMREGION_BAD SQFT SimI_04005_14TO B29VV01_14TO CMHO_MEX_58514_14TO CMHO_NONMEX_55727_14TO TOTAL_SEATS TOTAL_BAR_EXPEND_14TO DUMREGION_GOOD ; RUN;</p><p>PROC REG DATA = CASABONITANEW; A: MODEL SALES = TOTAL_CUST_VALUE BAD_POW_0_5RO GOOD_POW_0_5RO DUMREGION_BAD SIMI_04005_14TO B29VV01_14TO CMHO_MEX_58514_14TO CMHO_NONMEX_55727_14TO TOTAL_SEATS; B: MODEL SALES = TOTAL_CUST_VALUE BAD_POW_0_5RO GOOD_POW_0_5RO DUMREGION_BAD SIMI_04005_14TO B29VV01_14TO CMHO_MEX_58514_14TO CMHO_NONMEX_55727_14TO; C: MODEL SALES = BAD_POW_0_5RO GOOD_POW_0_5RO DUMREGION_BAD SQFT SIMI_04005_14TO B29VV01_14TO CMHO_MEX_58514_14TO CMHO_NONMEX_55727_14TO; D: MODEL SALES = BAD_POW_0_5RO GOOD_POW_0_5RO DUMREGION_BAD SIMI_04005_14TO B29VV01_14TO CMHO_MEX_58514_14TO CMHO_NONMEX_55727_14TO TOTAL_SEATS; E: MODEL SALES = BAD_POW_0_5RO GOOD_POW_0_5RO DUMREGION_BAD SQFT SIMI_04005_14TO B29VV01_14TO CMHO_MEX_58514_14TO CMHO_NONMEX_55727_14TO TOTAL_BAR_EXPEND_14TO; RUN; QUIT;</p>
Details
-
File Typepdf
-
Upload Time-
-
Content LanguagesEnglish
-
Upload UserAnonymous/Not logged-in
-
File Pages72 Page
-
File Size-