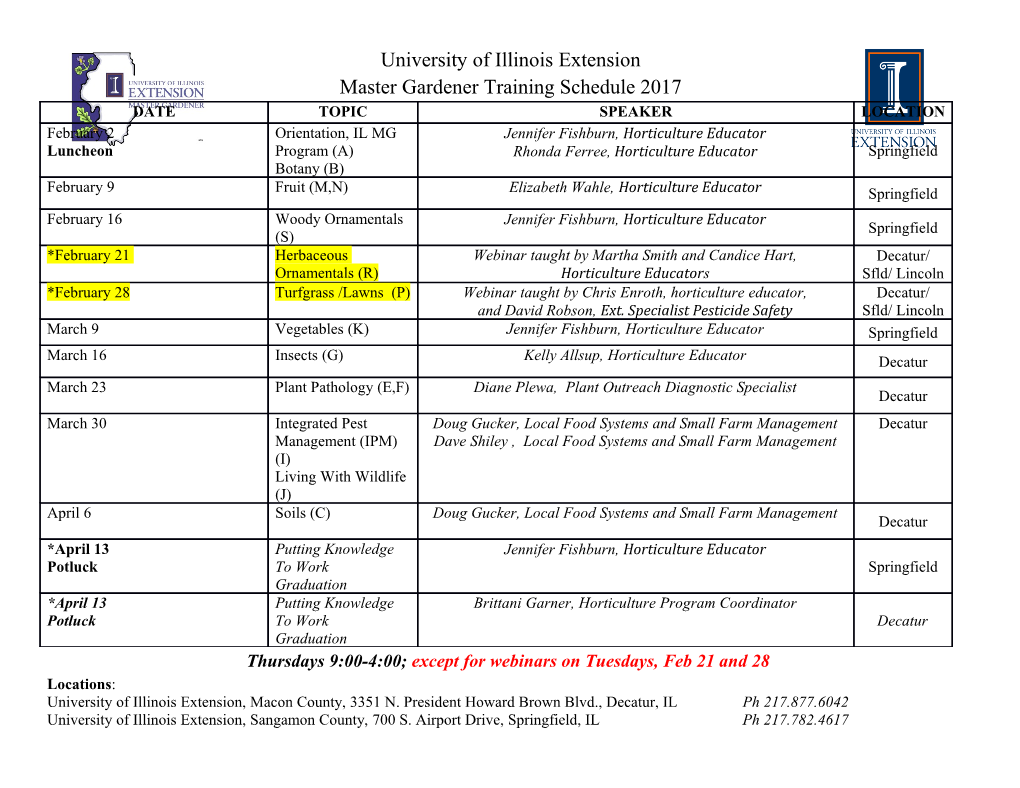
<p> 1 A numerical model of birch pollen emission and dispersion 2 in the atmosphere. Description of the emission module 3M.Sofiev1, P.Siljamo1, H.Ranta2, T.Linkosalo4, S.Jaeger5, A.Rasmussen6, A.Rantio-Lehtimaki3, 4E.Severova7, J.Kukkonen1 51 Finnish Meteorological Institute, Finland, [email protected] 62 EVIRA, Finland 73 University of Turku, Finland 84 Finnish Forest Research Institute, Finland 95 Medical University of Vienna, Austria 106 Danish Meteorological Institute, Denmark 117 Moscow State University, Russia</p><p>12</p><p>131. Abstract 14A birch pollen emission model is described and its main features are discussed. The 15development is based on the double-threshold temperature sum model that describes the 16propagation of the flowering season and naturally links to the Thermal Time models for 17predicting the flowering onset and duration. During the flowering season, the emission model 18takes into account ambient humidity and precipitation rate, which both suppress the pollen 19release, as well as wind speed and turbulence intensity, which promote it. These dependencies 20are qualitatively evaluated with pollen concentration observations. Reflecting probabilistic 21character of the flowering of an individual tree in a population, the model introduces 22relaxation functions at the start and end of the season. The physical basis of the suggested 23birch pollen emission model is compared with another comprehensive emission module 24reported in literature. The emission model has been implemented in the dispersion modelling 25system SILAM, which results are evaluated in the companion paper.</p><p>26</p><p>27Key words: birch pollen, pollen emission, pollen forecasting, dispersion modelling </p><p>28</p><p>29</p><p>30</p><p>1 1 312. Introduction 32A large-scale dispersion of pollen grains and spores has been known since the middle of the 33previous century [Erdtman, 1931; 1935; Gregory, 1961] but attracted large attention 34comparatively recently. The long-range transport of pollen and spores has two evident 35consequences: (i) short-term (hours or days) changes in the pollen concentrations over 36receptor regions and (ii) comparatively rapid (a matter of days) large-scale redistribution of 37genetic material along the atmospheric pathways [Lindgren et al., 1995]. Recently, a 38substantial progress has been achieved in understanding the first phenomenon, which leads to 39seemingly irregular modifications in the allergenic seasons over large areas [Corden et al., 402002; Damialis and Gioulekas, 2005; Hjelmroos, 1992; Latalova et al., 2002; Mahura et al., 412007; Ranta and Satri, 2007; Rantio-Lehtimaki, 1994; Siljamo et al., 2008c; Skjøth et al., 422008; Sofiev et al., 2006a]. It is also getting clear that, despite the features of every specific 43episode vary randomly and widely in comparison with other episodes and other regions, there 44is a systematic pattern in the spring-time pollen redistribution in Europe. There were several 45attempts to reveal such a pattern via multi-annual analysis [Damialis and Gioulekas, 2005; 46Siljamo et al., 2008a; 2008c; 2006; Skjøth et al., 2009; 2008; 2007; Smith et al., 2008; Sofiev 47et al., 2006a; Stach et al., 2007] and the amount of material and the number of analysed cases 48are growing. However, the integrated picture for Europe is still to be drawn. </p><p>49Different taxa exhibit varying potential to the long-range transport of their pollen. Arguably 50one of the most-important allergenic species in Europe is birch. It is a strong allergy- 51provoking plant with substantial population sensitivity in nearly all parts of Europe. In 52Northern Europe, it is the most abundant allergenic pollen type, with approximately 15% of 53the population being sensitized to its allergens [WHO, 2003]. The wide habitation areas of 54silver birch (Betula pendula Roth.) and downy birch (B. pubescens L.) extend from the 55mountainous regions of southern Europe to the northernmost Fennoscandia, and through 56Siberia to the east coast of Asia [Atkinson, 1992; OECD, 2003]. </p><p>57The phenomenon of the pollen long-range transport has one peculiarity. It is known for 58decades that the bulk of pollen is deposited near the source plant [Raynor et al., 1970; 59Tampieri et al., 1977; Wright, 1952; 1953]. However, birches, as well as the other species 60(Alnus, Carpinus, Corylus, Ostrya, Fagus, Quercus, Castanea) belonging to the order 61Fagales, are the wind-pollinated trees generating vast amounts of pollen to ensure sufficient 62level of fertilization of female flowers in receptor areas. The grains are quite small and light, 63so that substantial fraction of the released material can be transported over hundreds and 64thousands of kilometres if weather conditions are suitable (Sofiev et al. 2006b). Since the total</p><p>2 2 65number of the released grains is high and the threshold levels for provoking symptoms in 66sensitized people are quite low this long-range transported pollen can have substantial health 67impact [Viander and Koivikko, 1978].</p><p>68A key tool for analysing the pollen redistribution in air is the atmospheric dispersion 69modelling. </p><p>70Many studies address the local redistribution of pollen and seeds [Arritt et al., 2007; Aylor et 71al., 2006; Kuparinen et al., 2007] mainly addressing the distribution of the genetically 72modified species and possibilities of unwanted distribution of these plants. At large scale, the 73integrated approaches based on dynamic models covering the main parts of the pollen life 74cycle and its atmospheric transport are under development in several countries. </p><p>75The European-scale operational System for Integrated modeLling of Atmospheric 76coMposition (SILAM) presented in the current paper has been created by an international 77consortium within the scope of POLLEN project (http://pollen.fmi.fi) and recently its pollen 78source term has been accepted for the allergenic computations by the MACC European 79modelling consortium (http://www.gmes-atmosphere.eu). Various versions of the model have 80been used for forecasts of the pollen distribution in Europe starting from 2005 [Sofiev et al., 812006a]. Also, the system has been applied to reanalysis of the flowering seasons starting from 821997 [Siljamo et al., 2008c; Veriankaitė et al., 2010]. </p><p>83Regional-to-local scale system was started within the ASTHMA project 84(http://www.enviport.com/) in Southern Europe. Together with SILAM, these systems formed 85a demonstration service in the ESA PROMOTE project (GMES service element, 86http://www.gse-promote.org/promote.html) from where the SILAM-based service continued 87in the EU-PASODOBLE downstream service (http://www.myair-eu.org). A system COSMO- 88ART (http://www.cosmo-model.org, http://www.imk-tro.kit.edu/download/LM-ART.pdf) has 89been developed in University of Karlsruhe [Helbig et al., 2004; Vogel et al., 2008] and is 90being refined in MeteoSwiss, aiming at operational forecasting for Central and South-Western 91Europe. An integrated-model development is also going on in Denmark with regional 92ENVIRO-HIRLAM (http://www.pollenprognose.dk) [Mahura et al., 2009] and local-scale 93OML systems (http://www.oml.dmu.dk). Regional activities are going on in several European 94countries, US (e.g., http://pollen.utulsa.edu, [Efstathiou et al., 2011]), and Japan. </p><p>95The goals of the current paper are: (i) to present the birch pollen emission model that is 96implemented in SILAM; (ii) to highlight the main driving processes and the uncertainties 97inherent in the model; (iii) to compare the structure of the new model with another pollen </p><p>3 3 98emission algorithm reported in literature. A companion paper quantifies the performance of 99the SILAM system with the current pollen emission module.</p><p>100</p><p>1013. Input information and models</p><p>102 3.1. Components of the SILAM dispersion model 103As shown by [Sofiev et al., 2006a], the main components of virtually any comprehensive 104chemistry transport model can be used to describe pollen dispersion: advection with mean 105wind, mixing due to turbulence, gravitational settling (the main mechanism of pollen dry 106deposition), and scavenging with precipitation. The pollen model presented in this paper is 107constructed as a part of the SILAM modelling system [Sofiev et al., 2008]. The model 108dynamic core includes both Lagrangian [Sofiev et al., 2006b] and Eulerian [Galperin, 2000; 109Sofiev, 2002] advection-diffusion formulations. The removal processes are described via dry 110and wet deposition. Dry deposition of pollen is described via the gravitational settling. The 111SILAM wet deposition parameterization [Horn et al., 1987; Jylha, 1991; Smith and Clark, 1121989; Sofiev et al., 2006b] is based on direct observations performed for moderately 113hydrophobic aerosols. It distinguishes between sub- and in-cloud scavenging by both rain and 114snow. The particle size dependence of the impaction scavenging is taken into account by 115increasing the scavenging rate for super-micron particles in relation to their settling velocity. </p><p>116 3.2. Thermal Time flowering models 117There are several approaches for prediction of dates of the phenological phases. Linkosalo et 118al., [2008] compared the Thermal Time, Sequential [Hänninen, 1990], Parallel [Cannell and 119Smith, 1983], and Flexible models [Chuine, 2000; Schaber and Badeck, 2003] of leaf bud 120burst against independent control dataset and found that the simplest Thermal Time models 121performed better than more complicated approaches. They suggested that the complex 122phenological models were over-parameterized and able to adapt to noise in the learning 123dataset in addition to the phenological phenomenon itself. Therefore, the current development 124was based on the Thermal Time approach.</p><p>125The parameterization of flowering in the current emission model follows a principle of two 126thresholds for the temperature sum [Linkosalo et al., 2010], which assumes that the timing of 127birch flowering is mostly driven by accumulated ambient temperature during certain time 128period. As a result, the temperature sum threshold for both start of the season and end of the 129season are stable from year to year. As shown by [Linkosalo et al., 2010], as well as by a</p><p>4 4 130number of modelling works on birch phenology [Häkkinen et al., 1998; Hänninen, 1990; 1311995; Linkosalo et al., 2008], this assumption is usually well fulfilled.</p><p>132According to [Linkosalo et al., 2010], the cumulative fraction R of pollen released from the 133beginning of a year until the time t is piecewise linear and proportional to the temperature 134sum H during the main flowering season. </p><p> 0, H(t) H fs (before the season) H(t) H fs 135( 0) R(t) , H fs H(t) H fe (season goes on) H fe H fs 1, H(t) H fe (season is over)</p><p>136The temperature sum thresholds for start and end of the season Hfs and Hfe as well as the form 137of the function H(t) have to be identified from observational data. </p><p>138There exist numerous parameterizations for Hfs [Cannell and Smith, 1983; Chuine, 2000; 139Hänninen, 1990; Linkosalo et al., 2008; Menzel, 1997; Rotzer and Chmielewski, 2001; 140Schaber and Badeck, 2003]. However, they appeared practically incomparable with each 141other and applicable only to the regions and the species, for which they were developed: both 142the temperature sum threshold and the formulations for H(t) vary from model to model even if 143their regions overlap. Attempts to generalise the formulations by introducing e.g. the</p><p>144latitudinal dependence of Hfs also did not resolve the problem. For example, the temperature 145sum threshold formula with the explicit latitudinal dependence for Germany [Menzel, 1997] 146cannot be extrapolated to the Finnish latitudes: it would lead to the negative threshold value 147all over the country. </p><p>148As a result, none of the existing Thermal Time model parameterizations was found directly 149applicable to the European-wide applications, so that the parameters of the eq. ( 0) have to be 150identified afresh. </p><p>151 3.3. Input data 152For identification of parameters in eq. ( 0), the database of more than 15000 records of dates 153of the phenological phases across Europe has been collected [Siljamo et al., 2008b]. From that 154database, the date of the leaf unfolding subset (the largest in the database) was taken for the 155current study. </p><p>156Observational data on pollen concentrations in air were obtained from European Aeroallergen 157Network (EAN, https://ean.polleninfo.eu/Ean/), which receives the data from about 35 158countries and 300 site all over Europe. For 2006 (the year highlighted in this paper) the set 159includes 5787 daily data points from 213 stations. </p><p>5 5 160The temperature time series for phenological and aerobiological station were extracted from 161the meteorological archive of European Re-Analysis (ERA-40, http:///www.ecmwf.int). The 162ERA data cover the period from 1957 till 2001 with the six hours time resolution of the 163analysis. ERA-40 applied the three dimensional variational data assimilation to accommodate 164several types of meteorological observations, such as in-situ data, satellite products, 165soundings, etc. Such approach combines the modelling capabilities and data from 166observational networks in optimal way, thus representing the best available knowledge on the 167state of the atmosphere, land and surface. Owing to the spatial averaging over the model grid 168(1.1250 in case of ERA-40), the extreme observations are filtered out. </p><p>169During the main season, the actual meteorological parameters are required for predicting the 170pollen release rate. These meteorological data were extracted from the ECMWF archive of the 171operational forecast. The time resolution of this dataset was 3 hours, whereas the spatial grid 172had 0.25º spacing. </p><p>173</p><p>1744. Pollen emission model 175Following (Sofiev et al. 2006a; Sofiev et al. 2006b), the output of the emission module is 176described as a release flux of pollen grains E(t,i,j): the number of grains emitted from 1 m2 of 177birch forest within 1 sec in a given model grid cell (i,j). For dispersion computations, the</p><p>178model emission flux Emdl(t,i,j) is obtained from E(t,i,j) by multiplication with the fraction of 179the birch forest (i,j), and the grid cell area S(i,j):</p><p>180( 0) Emdl ( t , i , j )= E ( t , i , j )j ( i , j ) S ( i , j ) 181The term E(t,i,j) has to be further decomposed using the double-threshold model eq. ( 0): dR 182( 0) E( t , i , j )= N p ( t , i , j ) F ( meteo ) dt total</p><p>2 183Here Ntotal is the total number of pollen grains release from 1m of birch forest during the 184whole season, p(t,i,j) is the probability of the trees to flower in the given grid cell, F(meteo) is 185the meteo-dependent flux correction.</p><p>186</p><p>187 4.1. Start and propagation of the flowering season 188To obtain dR dt , we used the most-common formulations for the temperature sum in the</p><p>189Thermal Time models, which is an integral of temperature T above a cut-off level Tc-o starting</p><p>190from some time moment t0:</p><p>6 6 t H(t) (T(t) T ) 1(T(t) T )dt 191( 0) co co t0 192Here 1(x) is the Heaviside function that is equal to 0 for x<0 and 1 for x>0. </p><p>193Then the relative release rate becomes a piecewise linear function of temperature:</p><p>0,H ( t )< H fs ;</p><p> dR( t ) dH ( t ) dt (T ( t )- Tc- o ) * 1( T ( t ) - T c - o ) 194( 0) = =,Hfs < H ( t ) < H fs + D H dt Hfe- H fs D H</p><p>0,H ( t ) > Hfs + D H</p><p>195Formulations ( 0) require three parameters: starting temperature sum threshold Hfs, a</p><p>196difference between the thresholds for the start and the end of the pollen release H=Hfe-Hfs,</p><p>197and the cut-off temperature Tc-o. </p><p>198 4.2. Probabilistic description of the emission flux 199As shown in [Siljamo et al., 2008b], the irreducible uncertainties in the season timing are 200large and exceed the meteorological turnover time (~3 days persistence of the weather pattern 201at synoptic scale in Europe). As a result, any deterministic model of the flowering season 202would be inaccurate. Indeed, the transport conditions are dictated by meteorological situation, 203so the shift of pollen release by more than the above 3 days would mean wrong parameters of 204the release and different dispersion pattern of emitted pollen. A solution implemented in 205SILAM considers the flowering description in probabilistic terms. The approach is based on 206assuming certain probability for an individual tree to flower during a specific day</p><p>207 pf( H / H fs , N / N total )= p fs ( H / H fs ) p fe ( N / N total ) , where the functions pfs and pfe describe 208the probability for a single tree to start and end the flowering, respectively, N is the</p><p>209cumulative pollen amount released since the season start, and Ntotal is the total number of 210grains available for the whole season.</p><p>211 4.2.1. Start of the season</p><p>212Before the season, with H approaching Hfs, pfs determines the gradual start of the pollen</p><p>213release. Specific shape of the pfs function is uncertain and hardly important. The only crucial</p><p>214parameter is duration of the flowering spin-up, i.e. the transition range H (in terms of relative 215temperature sum) between, say, 5% and 95% of emission intensity in the grid cell. This 216parameter is not measured but can be transformed into the observable quantity:</p><p>1 dH (Tfs- T c- o ) 217( 0) dH� d t d t H dt H fsH= H fs fs</p><p>7 7 218Here t is the time lag between the 5% and 95% of the flowering intensity and Tfs is the</p><p>219temperature at the first flowering day. From the point of view of phenological observations, t 220represents the uncertainty of determination of the phenological phases. Such uncertainty has 221been quantified by [Siljamo et al., 2008b]. </p><p>222For simplicity, pfs is assumed to be piece-wise linear with regard to temperature sum:</p><p>0,x < 1 -d H 骣H x -1 +d ( 0) p琪 p( x )-H , 1 <d < x + 1 d 223 fs琪 � fs H H 桫H fs 2d 1,x > 1 +d H</p><p>224Here x=H / Hfs. After [Siljamo et al., 2008b], H ~20% can be taken as a rough estimate.</p><p>225The application of the blurring function ( 0) results in: (i) gradual start of pollen release 226already when the temperature sum is approaching the threshold but is still below it; and (ii) all 227trees in a grid cell are involved into the process somewhat later than the threshold is passed. </p><p>228</p><p>229 4.2.2. End of the season 230The end of the season is determined from the principle of the “open pocket”, i.e. the emission</p><p>231continues until N=Ntotal. </p><p>232The total amount of pollen developed in catkins Ntotal is a very uncertain parameter, which is 233presently estimated semi-manually using the data from the previous year and introduced into 234the model as a prescribed fixed map. Some regional studies show the possibility to predict this 235parameter based on the previous-year meteorological data [Rasmussen, 2002] but the 236approach is yet to be extended to the European scale.</p><p>237Following the same approach as that of the pfs function, pfe reads as:</p><p>1,R < 1 -d N</p><p>骣 N 1+d N - R 238( 0) pfe琪 = p fe( R ) = , 1 -d N < R < 1 + d N 桫Ntotal 2d</p><p>0,R > 1 +d N</p><p>239An estimate of N ~20% is used in the current SILAM setup.</p><p>240</p><p>241 4.3. Meteorology-dependent corrections 242During the main season, there are three meteorology-dependent correction functions applied 243to the dynamic release rate E(t,i,j): for wind speed, relative humidity and precipitation rate.</p><p>8 8 244Precipitation- and humidity- related corrections follow from known “prohibiting” thresholds 245totally suppressing the pollen release. Until these thresholds are reached, these variables do 246not affect the release (they do not promote it). Near the threshold, the piece-wise linearly 247decreasing transition function is taken:</p><p>轾 1, x x 犏 low 犏xhigh - x 248( 0) f( x , x , x )= , x < x < x thr low high犏x- x low high 犏high low 犏 0, x x 臌 high</p><p>249The lower and upper thresholds for relative humidity are taken as qlow=50% and qhigh=80%. </p><p>250For precipitation, Plow=0. For selection of Phigh several considerations have to be taken into 251account. Any noticeable rain suppresses the release and scavenges out the emitted grains. The 252pollen release can also be stopped by high relative humidity associated with rain, which 253covers wider areas than the rain event itself. However, short-term convective rains cover the 254territory much smaller than the grid cell [Morel and Senesi, 2002]. Such scattered 255precipitation still allows the trees to emit pollen from the dry parts of the grid cell area.</p><p>-1 256Taking into account the above uncertainties, an estimate of Phigh =0.5 mm hr (a grid-cell 257average rate) is taken as the threshold suppressing the pollen emission. </p><p>258Wind-dependent correction has to take into account three phenomena: (i) in case of low wind 259but developed thermal convection, turbulence alone is sufficient to kick-start the release by 260generating sub-grid convective winds, (ii) stronger wind promotes the release by picking the 261grains from open catkins; (iii) after reaching some level, further increase of wind does not 262affect the release rate, which is then limited by availability of ready-to-fly pollen grains in the 263catkins. These phenomena can be included into a single function as follows: </p><p>轾 骣 U+ w* 264 ( 0) fwind= f stagnant + f promote 犏1 - exp琪 - 臌犏 桫 Usatur</p><p>265Here U is the wind speed, w* is convective velocity scale, Usatur is the saturation wind speed,</p><p>266(fstagnant+fpromote) is the maximum “promotion” that wind can give to the release rate. In stagnant</p><p>267conditions, the function ( 0) suppresses the release by the fstagnant factor.</p><p>-1 268In the current SILAM version, Usatur=5 m sec fstagnant=0.5, and fpromote=1.</p><p>269Resulting emission rate is a product of the above-described specific terms:</p><p>9 9 轾0 : H< H fs 犏 犏 T- T 骣H S( i , j )j ( i , j ) Nc- o f ( U , w *) p琪 p( R) * E = 犏 totalDH wind fs琪 H fe 270( 0) model 犏 桫 fs 犏 f( q , q , q ) f ( P , P , P ) : H< H < H + D H 犏 thr low high thr low high fs fs 0 : H> H + D H 臌犏 fs 271</p><p>2725. Determination of parameters of the temperature sum 273 model </p><p>274The parameters needed for the eq ( 0) – Hfs, H, Tc-o, t0 – were identified by optimal fitting of 275the flowering start and end dates into the phenological and aerobiological observations. To 276overcome the problems connected with regional variations of these parameters, the European 277continent was split to 33 sub-regions, which together cover its entire territory and have 278limited but noticeable overlap with each other (~10% of their areas). Inside each region the 279independent fittings were performed. The overlap between the regions resulted in partly 280overlapping sets of observations used by the fitting procedures in the neighbouring regions. 281This smoothed out the contrasts between the parameterizations in the neighbouring regions.</p><p>282Since none of the above parameters is observed directly, the fitting variables were the dates of 283the phenological phases, such as the leaf unfolding. These dates are the primary outputs of the 284phenological model ( 0) - ( 0) and also observed directly. The fitting then minimised the 285difference between the observed dates and the corresponding model predictions by varying 286the above parameters.</p><p>287To compute the temperature sum, we used the discrete version of the definition ( 0): </p><p>D 288( 0) H(D) (T(d) Tco ) 1(T(d) Tco ) d Ds 289Here D is day and bar denotes the daily averaging constructed from the 6-hours ERA values,</p><p>290Ds is the starting day of the H integration. </p><p> mdl 291The modelled date of the flowering start D fs (s) for the specific station s was then defined as</p><p>292the first day when H(D,s) Hfs. The criterion for the fitting was the RMS of the model</p><p> mdl obs 293predictions Dfs ( s ) versus observed D fs (s) :</p><p>Nr 1 mdl obs 2 294( 0) Jfs( H fs , D s , T c- o , r )= ( D fs ( s ) - D fs ( s )) min H, D , T Nr s=1 fs s c- o</p><p>295Here Nr is the number of stations in the sub-region r and Jfs is the sub-regional cost function.</p><p>10 10 296Fitting of Hfe followed the same way, except for the observational dataset. Since the 297phenological observations of the end of flowering are scarce, we used the aerobiological data 298of EAN. The ending date of the flowering was computed using the 97.5% criterion for the 299season end [Goldberg et al., 1988]. </p><p>300Upon completion of fitting procedures for Hfs and for Hfe, their difference H was taken for 301each sub-region.</p><p>302Expectedly, the optimal fitting problem was ill-posed and the uniqueness of the solution was 303not guaranteed, which was a roadblock because the level of noise in the observational data 304was sometimes overwhelming [Siljamo et al., 2008b]. Certain improvement was achieved</p><p>305owing to the interdependence of the above parameters. It turned out that Ds and Tc-o could be 306prescribed, so that the fitting has to be done only for the temperature sum thresholds. The</p><p>307resulting value of the cost function Jfs was practically the same as for the fit with all three 308parameters varying.</p><p>309The prescribed values for birch were: Tc-o=3.5 C and Ds=60 (March 1). </p><p>310The quality of the fitting outcome can be verified using several criteria. Firstly, the residual of</p><p>2 311the fit should be larger than the objective uncertainty of the observations: J > s obs . This 312requirement ensures that the model is not over-fitted to noise in the data. Secondly, the 313residual should be smaller than the sum of the observational uncertainty and the inter-annual</p><p>2 2 314variability of the flowering dates: J <sobs + s time . It means that the model resolves this 315variability. Thirdly, the large-scale features of the observed and fitted spatial patterns of the 316flowering dates should be similar but the high-frequency fluctuations in the data map should 317be smoothed out by the model. Finally, according to the classical work of [Linsser, 1867], for 318any given phenomenon at any geographical place the threshold (in the scale of temperature 319sum) should be a constant fraction of the overall accumulated Effective Temperature Sum 320(ETS) over the whole growing period.</p><p>321The results of the fitting (Figure 1) show that the variation of the temperature-sum threshold</p><p>322for the start of flowering Hfs is more than a factor of three between the Southern and Northern 323Europe and is indeed smooth. The Figure 2 shows that the error in the start of flowering is 324indeed comparable but larger than the irreducible uncertainty in the phenological data 325themselves, as estimated by [Siljamo et al., 2008b]. Hence, the procedure is conservative 326enough avoiding the over-fit of the model to the noise in the data. At the same time, the error 327is lower than the inter-annual variability of the flowering dates, which can reach several 328weeks (not shown). Hence, the suggested fit utilises (most of) useful information stored in the</p><p>11 11 329phenological records. The spatial trends of observational uncertainty and residuals (Figure 2) 330are similar, which confirms that the main contributor to the residuals is the observational</p><p>331uncertainty itself. Finally, the ratio of Hfs to ETS computed for 2006 (Figure 3) is indeed 332nearly constant over the European continent. Exceptions are the mountain areas and northern 333Lapland, where the phenological information was almost non-existent and the accuracy of 334both Lensser’s law and our fit is questionable. Therefore, all above quality criteria are 335satisfied.</p><p>336Fitting of Hfe was less straightforward because the end of the season had to be defined from 337aerobiological observations, which are sensitive to long-range transport of pollen. Since 338observations cannot distinguish between the LRT grains and those produced locally, the 339flowering duration tends to be over-estimated. In addition, the temperature sum is 340accumulated throughout the whole season including rainy and high-humidity days when the 341release does not take place, which is another uncertainty pushing towards over-estimation.</p><p>342Therefore, the Hfs map in Figure 1 will require adjustments (see a companion paper 343discussion). An empirical value H=50 degree-days has shown to provide acceptable results.</p><p>344There are some peculiarities in the threshold maps. Thus, both start- and end-season 345thresholds are the highest in marine climate, which can be quite warm in early spring but 346clearly colder during the main flowering season in April-May. An explanation of this 347behaviour can be the suppressed diurnal variation of temperature in the coastal regions and 348much slower warming-up of the environment, which makes the cut-off temperature uncertain 349and probably different than in more continental conditions. As a result, the single-parameter 350Europe-wide fitting may not be appropriate in those regions: the cut-off temperature should 351possibly be taken somewhat higher than in continental climate. </p><p>352The other peculiarity is the very low thresholds in the southern (for Hfs) and central (for Hfe) 353Norway. Despite these are the coastal regions, the values there are lower than even in Finnish 354Lapland. This suspicious behaviour can possibly originate from the mountainous relief and a 355sharp rise of the topography from the sea level up to the ridges. The ERA-40 temperature 356field and the fitting procedure simply do not resolve the narrow valleys and the quick rise of 357the relief. The start of flowering of trees in the valleys is then driven by local temperatures, 358which may have little common with the mean temperature over 1.125 1.125 ERA grid 359cell. Also, there are too few observations reported in Norway. Similar effect shows up in 360Alpine region, where the valley temperatures and the corresponding thresholds are evidently 361higher than up in the mountains. These regions are also outliers in the Lenssers’s ratio map in</p><p>12 12 362Figure 3. To resolve these features, the grid cell size should be a couple of km, which lies 363outside the scope of the European model development study.</p><p>364</p><p>3656. Evaluation of the process representations in the 366 emission module 367In this section we analyse the short-term reaction of the emission module to meteorological 368forcing via comparison with observed features. The emission fluxes are not measured 369explicitly, therefore the analysis has to be based on the pollen counts during the main pollen 370season and SILAM-predicted concentrations. For this evaluation, we took the EAN data for 3712006 and ran SILAM with 30km resolution over Europe using the ECMWF meteorological 372data. </p><p>373In Figure 4, the four main meteorological parameters extracted from the meteorological 374model fields are plotted against the observed and predicted pollen counts at aerobiological 375sites. The left-hand column shows the scatter plot of the observed daily pollen counts and the 376meteorological parameters picked at 12:00 UTC. In the middle column, the SILAM-predicted 377daily pollen counts are presented against the same meteorological parameters picked at the 378same time. Finally, in the right-hand column the model-predicted hourly pollen counts are 379shown against the meteorological parameters collected with hourly resolution. </p><p>380As seen from Figure 4 (upper row), rain suppresses the pollen release in the model, which 381results in sharp decline of the concentrations – in agreement with the observations. However, 382the still-substantial concentrations predicted and observed in a small fraction of cases exist 383and largely originate from the regional transport (in the model, the local emission is fully 384stopped for P>0.5 mm hr-1). </p><p>385Dependence on relative humidity is more complicated (Figure 4, second row). Since the daily 386concentrations are plotted against humidity picked at 12:00 UTC, there are few cases with RH 387> 80% and the counts are indeed low during these days. The hourly scatter plot reveals that 388the high-humidity hours ( RH > 60% ) are characterised by very low pollen counts; 389intermediate-humidity levels 90%>RH > 60% correspond to intermediate concentrations, 390whereas dry periods in most cases correspond to high counts. </p><p>391The scatter plots against temperature (Figure 4, third row) do not show strong relations: 392variability is large and the signal appears weak even for hourly averaging. The barely visible 393tendency is that for the hours with high temperatures the model tends to predicts higher pollen</p><p>13 13 394concentrations (cannot be verified with daily averaging of the observations), whereas very 395low temperatures correspond to low pollen load (both predicted and observed). </p><p>396Similarly weak correlation is seen for wind speed: strong winds are mainly associated with 397somewhat elevated concentrations (both predicted and observed) but the overall scatter is very 398large in both observed and predicted fields. Reduction of wind speed results in two 399competitive effects: the emission rate becomes lower but also the ventilation of the 400atmosphere gets worse. The net effect is then practically negligible.</p><p>401The scatter-plots of Figure 4 also illustrate the mechanisms responsible for the diurnal 402variation of emission. Indeed, formulations ( 0) do not include forcing dependent on the time 403of the day. Therefore, the higher humidity, lower temperature, and lower wind speed during 404night time are the only factors reducing the emission during night and causing the diurnal 405variation of the rates. A commonly accepted zero level of emission during night time can thus 406be reached only if humidity is above the upper threshold or temperature is below the cut-off 407limit. In Figure 4, these cases were characterised by quite low pollen concentrations, which 408could still reach tens of grains per m3 – in agreement with the observations. </p><p>409</p><p>4107. Comparison with another emission parameterization 411In this section, we compare the formulations of the above pollen emission module with the 412birch pollen emission parameterization developed by [Helbig et al., 2004] – further referred to 413as H04. Apart from the current model, the H04 approach seems to be the only comprehensive 414parameterization of pollen emission applicable at regional-to-continental scale. It was 415implemented in the COSMO-ART modelling system and used for e.g. modelling of the birch 416pollen episode in Switzerland in 2006 [Vogel et al., 2008]. Recently, a combination of H04 417with our formulations has been applied in the US to birch and ragweed simulations 418[Efstathiou et al., 2011].</p><p>419The principal difference between the approaches is that the H04 algorithm computes the 420emission flux as a product of a characteristic velocity scale with a characteristic pollen 421concentration, adjusted with correction functions dependent on other parameters. The flux is 422then driven by the turbulent stress, whereas our approach follows the Linkosalo et al. (2010) 423temperature-driven model. As a result, the H04 approach has to utilise the prescribed duration 424of the flowering (included into the formulation of the probability of the trees to bloom), while 425the current model follows the actual meteorology-driven developments.</p><p>14 14 426The characteristic velocity scale in H04 is taken to be the friction velocity u*, which is useful 427as a measure of the mechanically induced turbulence near the surface. However, it may be not 428the ideal parameter describing the mechanical stress to the birch flowers, which are several 429meters far from the surface and located in the tree crown where the similarity theory is not 430applicable. Such stress should be represented by the regular wind blowing through the canopy 431rather than by the turbulent stress. The closest standard meteorological variable would be the 432wind speed at 10 metres above the displacement height (i.e. near the tree top) – the one used 433in SILAM. It is a diagnostic variable in all meteorological models and indirectly incorporates 434the horizontal wind shear stress, thus making the involvement of u* unnecessary. </p><p>435The turbulence-driven stress would become dominant in free-convection conditions, i.e. in 436unstable stratification and low mean wind. The corresponding term is absent from H04 437whereas the current approach includes it via the convective velocity scale w*.</p><p>438The impacts of precipitation and relative humidity to the emission rate are similar in both 439parameterizations. Also temperature is an emission promoter in H04 formulations but it does 440not play that central role as in our model.</p><p>441One of the key factors in H04 is the leaf area index (LAI), whose increase reduces the 442flowering intensity. We did not include this parameter because its relation to the birch 443flowering stage is neither straightforward nor easy to determine. A usual consideration that 444the flowering finishes before the leaves reach full size (in order not to inhibit the pollen 445dispersal) corroborates with H04 approach. Nevertheless, in the dataset [Siljamo et al., 2008b] 446the bud burst day was often before the first flowering day. As a result, LAI might start rising 447already before the flowering begins. However, LAI can still be useful in case of prolonged 448flowering in wet cold weather when leaves grow up before all pollen grains are released. </p><p>449The other process, which is not included into the current parameterization but considered in 450H04, is the pollen resuspension. As noted by H04, this process is very poorly known and can 451take place only at the very strong winds and wind gusts (above 15 m sec-1). Its influence can 452possibly be noticed at the end of the season, over flat terrains, and in dry conditions only. 453Therefore, we considered it as a rare phenomenon, which uncertainty is larger than the 454potential gain of its inclusion into the model.</p><p>455</p><p>15 15 4568. Summary 457The suggested pollen emission model for birch follows the concept of Thermal Time 458phenological models and, in particular, the double-threshold temperature sum approach 459determining the development of the flowering during the whole spring season.</p><p>460Apart from temperature, the pollen release rate is modulated by ambient humidity, 461precipitation, and wind speed. Higher humidity and rain suppress the release, whereas 462stronger wind promotes it. Atmospheric turbulence is taken into account via the turbulent 463velocity scale and thus becomes important only in cases close to free convection.</p><p>464A probability of an individual tree to enter the flowering stage is considered via uncertainty of 465the temperature sum threshold determining the start of flowering. </p><p>466The end of the season is described via the open-pocket principle, according to which the 467flowering continues until the initially available amount of pollen is released.</p><p>468Numerical values of the model parameters – temperature sum threshold for start and end of 469flowering, critical levels of relative humidity and precipitation intensity, and characteristic 470wind speed were identified via optimal fitting to the European phenological and 471aerobiological data.</p><p>472The model does not include explicit diurnal variation forcing, which is obtained as a by- 473product of the meteorological forcing. The pollen resuspension and relation of flowering to 474leaf area index are also left out of the parameterization due to high uncertainties in both 475parameters and their unclear relation to the pollen release processes.</p><p>476The model processes have been qualitatively evaluated using the observed pollen counts in 4772006, which were related to the meteorological input data and compared with the model 478predictions. Quantitative analysis of the model performance is the subject of the companion 479paper.</p><p>480The described model is freely available from the SILAM model Web site http://silam.fmi.fi.</p><p>481</p><p>4829. Acknowledgements 483Current work was performed within the scope of the Academy of Finland POLLEN project 484and supported by FP7 projects HIALINE, MACC and PASODOBLE. Networking activities 485were supported by the COST-ES0603 EUPOL Action. The pollen observational data by the 486European Aeroallergen Network (EAN) are kindly acknowledged.</p><p>16 16 487</p><p>48810. References</p><p>489Arritt, R. W., C. A. Clark, A. S. Goggi, H. L. Sanchez, M. E. Westgate, and J. M. Riese (2007), Lagrangian numerical 490 simulations of canopy air flow effects on maize pollen dispersal, Field Crops Research, 102, 151-162, 491 doi:10.1016/j.fcr.2007.03.008.</p><p>492Atkinson, M. D. (1992), Betula pendula Roth (B. verrucosa Ehrh.) and B. pubescens Ehrh, Journal of Ecology, 80, 837-870.</p><p>493Aylor, D. E., M. T. Boehm, and E. J. Shields (2006), Quantifying aerial concentrations of maise pollen in the atmopsheric 494 surface layer using remote-piloted airplanes and lagrangian stochastic modelling, JOURNAL OF APPLIED 495 METEOROLOGY AND CLIMATOLOGY, 45, 1003-1015.</p><p>496Cannell, M. G. R., and R. I. Smith (1983), Thermal time, chill days and prediction of budburst in Picea sitchensis, Journal of 497 applied ecology, 20, 951-963.</p><p>498Chuine, I. (2000), A unifief model for budburst of trees, Journal of theoretical biology, 207, 337-347.</p><p>499Corden, J. M., A. Stach, and W. Milligton (2002), A comparison of Betula pollen season at two European sites; Derby, 500 United Kingdom and Poznan, Poland (1995–1999), Aerobiologia, 18, 53-54.</p><p>501Damialis, A., and D. Gioulekas (2005), Transport of airborne pollen into the city of Thessaloniki : the effects of wind 502 direction , speed and persistence, International Journal of Biometeorology, 49, 139-145, doi:10.1007/s00484-004- 503 0229-z.</p><p>504Efstathiou, C., S. Isukapalli, and P. Georgopoulos (2011), A mechanistic modeling system for estimating large-scale 505 emissions and transport of pollen and co-allergens, Atmospheric Environment, 45(13), 2260-2276, 506 doi:10.1016/j.atmosenv.2010.12.008. [online] Available from: http://dx.doi.org/10.1016/j.atmosenv.2010.12.008</p><p>507Erdtman, G. (1931), Pollen-statistics: A new research method in paleoecology, Science, 73, 399-401.</p><p>508Erdtman, G. (1935), Pollen statistics: A botanical and geological research method, in Pollen Grains, edited by R. P. 509 Wodehouse, pp. 110-125.</p><p>510Galperin, M. V. (2000), The Approaches to Correct Computation of Airborne Pollution Advection, in Problems of 511 Ecological Monitoring and Ecosystem Modelling. XVII (in Russian), pp. 54-68, Gidrometeoizdat, St.Petersburg.</p><p>512Goldberg, C., H. Buch, L. Moseholm, and E. V. Weeke (1988), Airborne pollen records in Denmark, Grana1, 27, 209-217.</p><p>513Gregory, P. H. (1961), The microbiology of the atmosphere, Interscience, New York.</p><p>514Helbig, N., B. Vogel, H. Vogel, and F. Fiedler (2004), Numerical modelling of pollen dispersion on the regional scale, 515 Aerobiologia, 3, 3-19.</p><p>516Hjelmroos, M. (1992), Long-distance transport of Betula pollen grains and allergic symptoms, Aerobiologia, 8(2), 231-236.</p><p>517Horn, H.-G., H. Bonka, and M. Maqua (1987), Measured particle bound activity size-distribution, deposition velocity, and 518 activity concentration in rainwater after the Chernobyl accident, journal of aerosol science, 18, 681-684.</p><p>519Häkkinen, R., T. Linkosalo, and P. Hari (1998), Effects of dormancy and environmental factors on timing of bud burst in 520 Betula pendula, Tree Physiology, 18, 707-712.</p><p>521Hänninen, H. (1990), Modeling bud dormancy release in trees from cool and temperate regions, Acta Forestalia Fennica, 522 213, 1-47.</p><p>523Hänninen, H. (1995), Effects of climatic change on trees from cool and temperate regions: An ecophysiological approach to 524 modelling of bud burst phenology, Canadian Journal Of Botany-Revue Canadienne De Botanique, 73, 2030-2043.</p><p>525Jylha, K. (1991), Empirical scavenging coefficients of radioactive substances released from Chernobyl, ATMOSPHERIC 526 ENVIRONMENT, 25A(2), 263-270.</p><p>17 17 527Kuparinen, A., T. Markkanen, and H. Riikonen (2007), Modeling air-mediated dispersal of spores , pollen and seeds, , 8, 528 177-188, doi:10.1016/j.ecolmodel.2007.05.023.</p><p>529Latalova, M., M. Miętus, and A. Uruska (2002), Seasonal variations in the atmospheric Betula pollen count in Gdañsk 530 (southern Baltic coast) in relation to meteorological parameters, Aerobiologia, 18, 33–43.</p><p>531Lindgren, D., L. Paule, X.-H. Shen, R. Yazdani, U. Segerstrom, J.-E. Wallin, and M. L. Lejdebro (1995), Can viable pollen 532 carry Scotch pine genes over long distances?, Grana, 34, 64-69.</p><p>533Linkosalo, T., H. Lappalainen, and P. Hari (2008), A comparison of phenological models of leaf bud burst and flowering of 534 boreal trees using independent bservations, Tree Physiology, 28, 1873-1882.</p><p>535Linkosalo, T., H. Ranta, A. Oksanen, P. Siljamo, A. Luomajoki, J. Kukkonen, and M. Sofiev (2010), A double-threshold 536 temperature sum model for predicting the flowering duration and relative intensity of Betula pendula and B. 537 pubescens, Agricultural and Forest Meteorology, 6-11, doi:10.1016/j.agrformet.2010.08.007. [online] Available from: 538 http://dx.doi.org/10.1016/j.agrformet.2010.08.007</p><p>539Linsser, C. (1867), Die periodischen Ercheinungen des Pflanzenlebens in ihrem Verhältniss zu den Wärmeerscheinungen, 540 Memoires de LʼAcadémie Impériale des Sciences de St.-Pétersbourg, VIIE Serie(7), 44 pp.</p><p>541Mahura, A., A. Baklanov, and U. Korsholm (2009), Parameterization of the birch pollen diurnal cycle, Aerobiologia, 25(4), 542 203-208, doi:10.1007/s10453-009-9125-7. [online] Available from: 543 http://www.springerlink.com/index/10.1007/s10453-009-9125-7 (Accessed 5 July 2011)</p><p>544Mahura, A., U. Korsholm, A. Baklanov, and A. Rasmussen (2007), Elevated birch pollen episodes in Denmark : 545 contributions from remote sources, Aerobiologia, 23, 171-179, doi:10.1007/s10453-007-9061-3.</p><p>546Menzel, A. (1997), Phänologie von Waldbäumen unter sich ändernden Klimabedingungen - Auswertung der Beobachtungen 547 in den Internationalen Phänologischen Gärten und Möglichkeiten der Modellierung von Phänodaten,</p><p>548Morel, C., and S. Senesi (2002), A climatology of mesoscale convective systems over Europe using satellite infrared 549 imagery. II: Characteristics of European mesoscale convective systems, Quarterly Journal of the Royal 550 Meteorological Society, 128(584), 1973-1995. [online] Available from: http://cat.inist.fr/? 551 aModele=afficheN&cpsidt=13876728 (Accessed 7 June 2011)</p><p>552OECD (2003), Consensus document on the biology of European white birch (Betula pendula Roth). Series on harmonisation 553 of regulatory oversight in biotechnology, 28. [online] Available from: 554 http://www.olis.oecd.org/olis/2003doc.nsf/LinkTo/env-jm-mono(2003)12</p><p>555Ranta, H., and P. Satri (2007), Synchronized inter-annual fluctuation of flowering intensity affects the exposure to allergenic 556 tree pollen in North Europe, Grana, 46, 274-284.</p><p>557Rantio-Lehtimaki, A. (1994), Short, medium and long range transported airborne particles in viability and antigenicity 558 analyses, Aerobiologia, 10, 175-181.</p><p>559Rasmussen, A. (2002), The effects of climate change on the birch pollen season in Denmark, Group, 1997, 253-265.</p><p>560Raynor, G. S., E. C. Ogden, and J. V. Hayes (1970), Dispersion and deposition of ragweed pollen from experimental sources, 561 JOURNAL OF APPLIED METEOROLOGY AND CLIMATOLOGY, 9, 885-895.</p><p>562Rotzer, T., and F.-M. Chmielewski (2001), Phenological maps of Europe, Climate Research, 18, 249-257.</p><p>563Schaber, J., and F.-W. Badeck (2003), Physiology-based phenology models for forest tree species in Germany, International 564 Journal of Biometeorology, 47, 193-201.</p><p>565Siljamo, P., M. Sofiev, T. Linkosalo, H. Ranta, and J. Kukkonen (2008a), Development and application of biogenic emission 566 term as a basis of long-range transport of allergenic pollen, in NATO Science for piece and security Serties C: 567 Environmental Security. Air pollution modelling and its application, XIX, , Springer, edited by C. Borrego and A. I. 568 Miranda, pp. 154-162, SPRINGER-VERLAG BERLIN.</p><p>569Siljamo, P. et al. (2008b), Representativeness of point-wise phenological Betula data collected in different parts of Europe, 570 Global Ecology and Biogeography, doi:10.1111/j.1466-8238.2008.00383.x.</p><p>18 18 571Siljamo, P., M. Sofiev, E. Severova, H. Ranta, J. Kukkonen, S. Polevova, E. Kubin, and A. Minin (2008c), Sources, impact 572 and exchange of early-spring birch pollen in the Moscow region and Finland, AEROBIOLOGIA, 24(4), 211-230, 573 doi:10.1007/s10453-008-9100-8.</p><p>574Siljamo, P., M. Sofiev, E. Severova, H. Ranta, and S. Polevova (2006), On influence of long-range transport of pollen grains 575 onto pollinating seasons, in Developments in Environmental Science, 6. Air Polution Modelling and its Applications 576 XVIII, edited by C.Borrego & E.Renner, pp. 708-716.</p><p>577Skjøth, C. A., M. Smith, J. Brandt, and J. Emberlin (2009), Are the birch trees in Southern England a source of Betula pollen 578 for North London ?, International Journal of Biometeorology, 75-86, doi:10.1007/s00484-008-0192-1.</p><p>579Skjøth, C. A., J. Sommer, J. Brandt, M. Hvidberg, C. Geels, K. M. Hansen, O. Hertel, L. M. Frohn, and J. H. Christensen 580 (2008), Copenhagen – a significant source of birch (Betula) pollen?, International Journal of Biometeorology, 52, 453- 581 462, doi:10.1007/s00484-007-0139-y.</p><p>582Skjøth, C. A., J. Sommer, A. Stach, M. Smith, and J. Brandt (2007), The long-range transport of birch ( Betula ) pollen from 583 Poland and Germany causes significant pre-season concentrations in Denmark, Clinical and Experimental Allergy, 37, 584 1204-1212, doi:10.1111/j.1365-2222.2007.02771.x.</p><p>585Smith, F. B., and M. J. Clark (1989), The transport and deposition of radioactive debris from the Chernobyl nuclear power 586 plant accident with special emphasis on consequences to the United Kingdom., Meterorological Office Scientific 587 Paper, (42).</p><p>588Smith, M. et al. (2008), Long-range transport of Ambrosia pollen to Poland, Environmental Research, 148, 1402-1411, 589 doi:10.1016/j.agrformet.2008.04.005.</p><p>590Sofiev, M. (2002), Extended resistance analogy for construction of the vertical diffusion scheme for dispersion models, 591 JOURNAL OF GEOPHYSICAL RESEARCH-ATMOSPHERES, 107(D12), doi:10.1029/2001JD001233.</p><p>592Sofiev, M., M. V. Galperin, and E. Genikhovich (2008), Construction and evaluation of Eulerian dynamic core for the air 593 quality and emergency modeling system SILAM, in NATO Science for piece and security Serties C: Environmental 594 Security. Air pollution modelling and its application, XIX, edited by C. Borrego and A. I. Miranda, pp. 699-701, 595 SPRINGER-VERLAG BERLIN.</p><p>596Sofiev, M., P. Siljamo, H. Ranta, and A. Rantio-Lehtimaki (2006a), Towards numerical forecasting of long-range air 597 transport of birch pollen: theoretical considerations and a feasibility study, INTERNATIONAL JOURNAL OF 598 BIOMETEOROLOGY, 50(6), 392-402, doi:10.1007/s00484-006-0027-x.</p><p>599Sofiev, M., P. Siljamo, I. Valkama, M. Ilvonen, and J. Kukkonen (2006b), A dispersion modelling system SILAM and its 600 evaluation against ETEX data, ATMOSPHERIC ENVIRONMENT, 40(4), 674-685, 601 doi:10.1016/j.atmosenv.2005.09.069.</p><p>602Stach, A., M. Smith, C. A. Skjøth, and J. Brandt (2007), Examining Ambrosia pollen episodes at Poznań ( Poland ) using 603 back-trajectory analysis, International Journal of Biometeorology, 275-286, doi:10.1007/s00484-006-0068-1.</p><p>604Tampieri, F., P. Mandrioli, and G. L. Puppi (1977), Medium range transport of airborne pollen, Agricultural Meteorology, 605 18, 9-20.</p><p>606Veriankaitė, L., P. Siljamo, M. Sofiev, I. Sauliene, and J. Kukkonen (2010), Modelling analysis of source regions of long- 607 range transported birch pollen that influences allergenic seasons in Lithuania, AEROBIOLOGIA, 26(1), 47-62, 608 doi:10.1007/s10453-009-9142-6.</p><p>609Viander, M., and A. Koivikko (1978), The seasonal symptoms of hyposensitized and untreated hay fever patients in relation 610 to birch pollen counts: correlation with nasal sensitivity, prick tests and RAST, Clinical Allergy, 8, 387-396.</p><p>611Vogel, H., A. Pauling, and B. Vogel (2008), Numerical simulation of birch pollen dispersion with an operational weather 612 forecast system, International Journal of Biometeorology, (2006), doi:10.1007/s00484-008-0174-3.</p><p>613WHO (2003), Phenology and human health: allergic disorders, Copenhagen.</p><p>614Wright, J. W. (1952), Pollen dispersion of some forest trees.</p><p>615Wright, J. W. (1953), Pollen dispersion studies: Some practical applications, Journal of Forestry, 114-118.</p><p>19 19 616</p><p>617</p><p>618</p><p>619</p><p>620Figure captions</p><p>621</p><p>622</p><p>623</p><p>624Figure 1. Map of the temperature sum threshold for: a) the start of the season Hfs, b) end of the season Hfe. Unit 625= [degree day] 626</p><p>627 628Figure 2. Residuals of the fitting (panel a) and objective irreducible uncertainties of the phenological data (panel 629b, source: Siljamo et al, 2008). Unit = [day] 630 631 0 632Figure 3. A fraction of the Hfs in relation to the ETS for 2006, Tc-o=3.5 C. Relative units. 633 634 635 636Figure 4. Dependence of the daily observed (left), daily predicted (middle), and hourly predicted (right) 637concentrations [# m-3] of birch pollen on the meteorological forcing: rain intensity (first row, mm hr-1), relative 638humidity (second row, %), temperature at 2m above the surface (third row, 0C), and wind speed at 10m above 639the surface (fourth row, m s-1). 640</p><p>641</p><p>642</p><p>643</p><p>20 20 644</p><p>645</p><p>646</p><p>647</p><p>648</p><p>649</p><p>21 21 650</p><p>651</p><p>652Figure 1. Map of the temperature sum threshold for: a) the start of the season Hfs, b) end of 653the season Hfe. Unit = [degree day]</p><p>22 22 654</p><p> a) b) 655</p><p>656</p><p>657</p><p>658</p><p>659</p><p>660</p><p>661</p><p>662</p><p>663Figure 2. Residuals of the fitting (panel a) and objective irreducible uncertainties of the 664phenological data (panel b, source: Siljamo et al, 2008). Unit = [day]</p><p>23 23 665</p><p>666</p><p>667</p><p>668</p><p>669</p><p>670</p><p>671</p><p>672</p><p>673Figure 3. A fraction of the Hfs in relation to the ETS for 2006, Tc-o=3.50C. Relative units.</p><p>674 675</p><p>24 24 676 Daily observed Daily model-predicted Hourly model-predicted</p><p>25 25 677Daily observed Daily model-predicted Hourly model-predicted</p><p>678 679 680Figure 4. Dependence of the daily observed (left), daily predicted (middle), and hourly predicted (right) concentrations [# m-3] of birch pollen on the meteorological forcing: rain intensity (first row, mm hr-1), relative humidity (second row, %), temperature at 2m above the surface (third row, 0C), and wind speed at 10m above the surface (fourth row, m s-1). 681</p><p>26 26</p>
Details
-
File Typepdf
-
Upload Time-
-
Content LanguagesEnglish
-
Upload UserAnonymous/Not logged-in
-
File Pages26 Page
-
File Size-