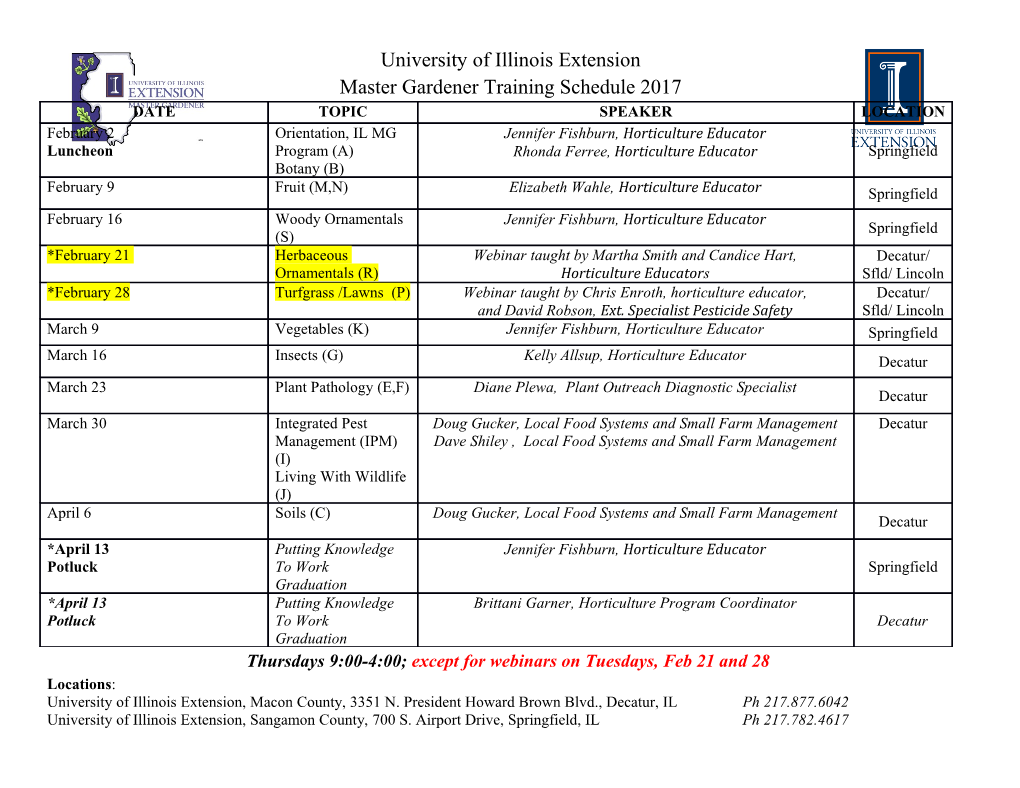
<p>Bayes Factors in complex genetics.</p><p>Author: Stephen Sawcer</p><p>Affiliation: University of Cambridge, Department of Clinical Neuroscience, Addenbrooke’s, Hospital, Hills Road, Cambridge, CB2 2QQ, UK</p><p>Corresponding Author: Stephen Sawcer University of Cambridge, Department of Clinical Neuroscience, Addenbrooke’s, Hospital, Hills Road, Cambridge, CB2 2QQ, UK Phone: +44 1223 216071 FAX: +44 1223 336941 email: [email protected]</p><p>Supplementary Data What sample size should be used? If one makes the simplifying assumption that all relevant common risk alleles have the same frequency and the same effect size, so that the power to identify each one is the same, then it is possible to generate plots like those shown in figure S1.1 The figure illustrates the impact of sample size on the relationship between the significance threshold employed and the odds that any positive result will be true. At any given significance threshold as the sample size gets smaller the improvement in the odds indicated by obtaining a positive result are reduced; that is the curves become more horizontal. The figure also shows that as study size falls below the critical value of 2,000 cases and 2,000 controls the significance threshold required to ensure adequate compensation for the low prior odds becomes more extreme. If we restrict the plotted curves to just those significance values where the sample size considered provides meaningful power (i.e. at least 1% power) we can see that studies involving fewer than 2,000, cases and 2,000 controls have little if any power to indentify significance at 5x10-7, let lone the more extreme significance threshold that would be required to compensate for the low prior odds (see figure S2). In the setting of a systematic genome-wide association screen (GWAS), where we might consider to have screened all 10 million common variants (either directly or indirectly through linkage disequilibrium, LD), we can think of the posterior odds as a measure of the extent to which results exceeding any given significance level have been enriched for true positives. In this context we can see that even at high levels of significance like 5x10-7 small studies will result in little enrichment as these extreme results will still contain more false positives than true positives (figure S1). As a result of this logic 2,000 cases and 2,000 controls has been recommended as a minimum in the genetic analysis of complex disease.2 If the effect sizes are smaller then the critical sample size required increases, see figure S3. Figure S1 The figure shows the relationship between the Posterior Odds that a result is true (plotted on a log scale on the y-axis) in relation to the significance threshold employed (plotted on a -log scale on the x-axis). Five sample sizes are listed in the legend, in each the number of cases and controls are equal, the 200 line thus indicates the posterior odds for a study involving 200 cases and 200 controls and so on. Power was calculated using the on-line genetic power calculator,3 assuming risk alleles have a frequency of 10%, a Genotype Relative Risk (GRR) of 1.3 and a multiplicative model. This figure probably should be viewed as a best case scenario for association studies in complex disease since in relativity effect sizes are likely to be smaller than the maximum suggested by linkage analysis. Reprinted from Brain, Vol. 131, 3118- 31, Copyright (2008), with permission from Oxford University Press.1</p><p>Figure S2 An adaptation of figure S1 but with each curve limited to just those significance threshold values for which the power is greater than 1% (the curves for 2,000 and 10,000 extend beyond the range plotted). Even if all 100 relevant common variants were tested we would only expect 1 to exceed the limiting significance threshold indicated by the right hand end of the plotted curve. Adapted from Brain, Vol. 131, 3118-31, Copyright (2008), with permission from Oxford University Press.1 Figure S3 This figure shows the minimum number of cases required in order to have sufficient power to identify association with p ≤ 5x10-7 in relation to the size of effects being sought. Sample sizes were calculated using the online genetic power calculator assuming a multiplicative model, a risk allele frequency of 50% and a power of 80% (the calculations assume an equal number of controls).3 Genotype Relative Risk indicates the increased relative risk that results from carrying a single copy of the risk allele. Bayes Factors</p><p>The probabilities P1 and P0 presented in the main text are conditional probabilities and can thus be more fully written as P(D|M1) - the probability of observing this particular set of data (D) conditional on the tested variant being genuinely associated (i.e. assuming underlying model M1), and P(D|M0) - the probability of observing this particular set of data (D) conditional on the tested variant being unassociated (i.e. assuming underlying model M0, the null hypothesis of no association). With this notation equation 3 becomes</p><p> PD | M1 PosteriorOdds * PriorOdds PD | M 0 By Bayes theorem P D | M P D | ,M * P | M d x x x x x where θX denoted the parameters of Model MX. Assuming a multiplicative model (an additive model on the log scale) we have p i log e 1 pi under the null hypothesis and p i log e Zi 1 pi </p><p> th under the model of association M1. Where pi is the probability of disease in the i th person, Zi is the number of risk alleles carried by the i person (0, 1 or 2), μ is the baseline log-odds of disease and β is the increase in log-odds for each copy of the risk allele carried. Each likelihood is then</p><p>N Yi (1Yi ) PD | pi (1 pi ) i1</p><p> th th where Yi = 1 if the i person is a case and 0 if the i person is a control. In order to calculate the conditional probabilities the likelihood under each model must be integrated over a prior distribution for the model parameters. The SNPTEST program developed by the Wellcome Trust Case Control Consortium (WTCCC) allows users to define normal distributions for the model parameters (μ and β) and thereby solve the integrals and generate Bayes factors.2 In this paper SNPTEST was used with the default setting for the prior distribution of μ = N(0,1). The prior distribution of β was then set according to the model being tested (see below). The notation and explanation provided above is based on that provided by the WTCCC,2 the SNPTEST program is freely available at http://www.stats.ox.ac.uk/~marchini/software/gwas/snptest.html There are alternate ways to solve these integrals and thereby calculate Bayes factors such as those described by Wakefield.4 The BF calculated for a given data set depends on what underlying model is assumed. Figures S4 and S5 show the BF’s calculated assuming that risk alleles have a frequency of 10% and exert OR’s of 1.3 and 1.1 respectively.</p><p>Figure S4 This reproduces figure 2 except the OR is assumed to be 1.3</p><p>Figure S5 This reproduces figure 2 except the OR is assumed to be 1.1 Methodological information In order to produce the figures based on Bayes Factors I generated hypothetical data sets for each sample size with Hardy Weinberg Equilibrium genotype counts based on a minor allele frequency of 10% in the controls and (10 + x)% in the cases. Values of x were chosen in order to generate a range of -log(p) values from 0 to 9. Each of these hypothetical data sets was analysed with the SNPTEST program2 in order to determine the log10(BF) corresponding to the calculated p-value. The results were generated assuming a multiplicative model (using the log additive option in SNPTEST2). In calculating the Bayes Factors I set the prior distribution for the β parameter to be N(0.1823 0.001) for figure 2, N(0.2624 0.001) for figure S4 and N(0.09531 0.001) for figure S5. This essentially sets the OR (odds ratio) to be 1.2 (=e0.1823), 1.3 (=e0.2624) or 1.1 (=e0.09531) respectively. Note for modest effects like these the OR is ≈ to the GRR. The on-line power calculator used to generate figures S1, S2 and S3 is parameterised in terms of the GRR while the SNPTEST program used to generate figures S4 and S5 is parameterised in terms of the OR. References</p><p>1 Sawcer S: The complex genetics of multiple sclerosis: pitfalls and prospects. Brain 2008; 131: 3118-3131. 2 Wellcome Trust Case Control Consortium (WTCCC) Genome-wide association study of 14,000 cases of seven common diseases and 3,000 shared controls. Nature 2007; 447: 661-678. 3 Purcell S, Cherny SS, Sham PC: Genetic Power Calculator: design of linkage and association genetic mapping studies of complex traits. Bioinformatics 2003; 19: 149-150. 4 Wakefield J: Bayes factors for genome-wide association studies: comparison with P-values. Genet Epidemiol 2009; 33: 79-86.</p>
Details
-
File Typepdf
-
Upload Time-
-
Content LanguagesEnglish
-
Upload UserAnonymous/Not logged-in
-
File Pages7 Page
-
File Size-