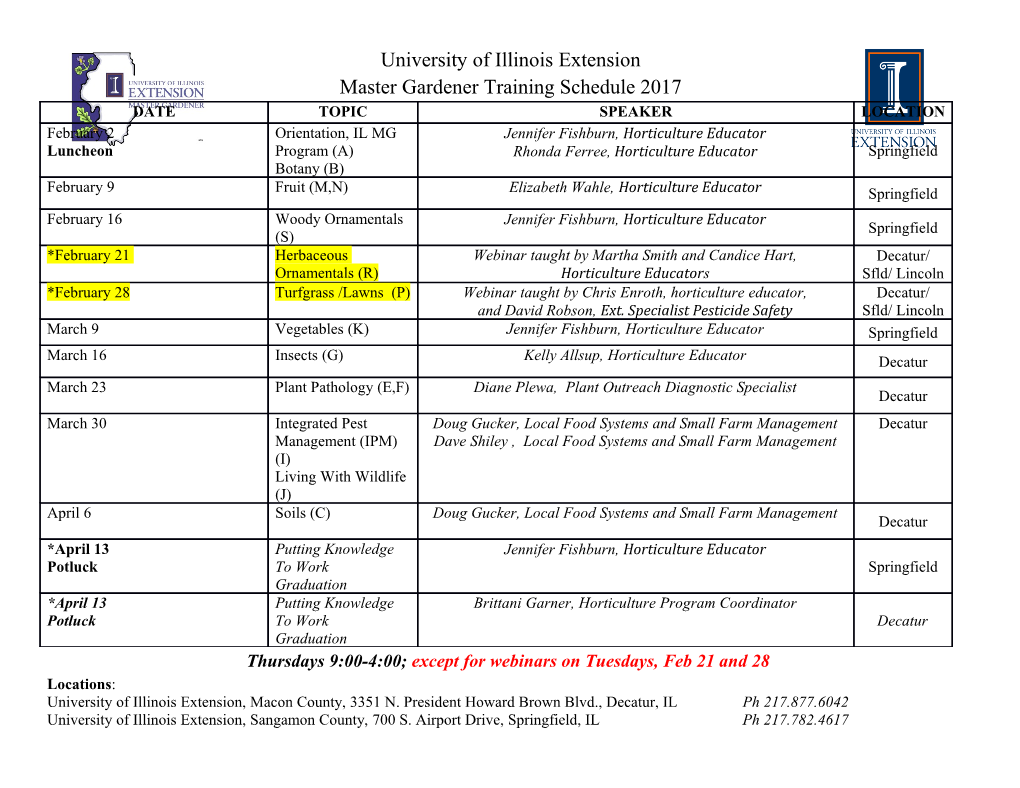
<p> Use of the Statistical Downscaling Model in the Search for Future Climates of the Northwest Territories</p><p>Heather Antoniuk Environment Canada - MSC, Prairie and Northern Region Hydrometeorology and Arctic Lab, Sciences Division Edmonton, Alberta</p><p>ABSTRACT</p><p>Climate change projections are generally produced by global climate models (GCMs). These projections from the GCMs are on too large a scale to be of good use at individual sites for impacts assessments. At a more localized level, results are produced by regional climate models (RCMs). Statistical downscaling (SDS), with an even smaller spatial scale, can be used to assess point site impacts of climate change. As well as being of higher spatial resolution than the RCMs, SDS is also less computationally demanding. SDS is most useful in regions with complex topography and in coastal areas due to the large variations of the climate over these small distances. These variations can be picked up with SDS but generally not with GCMs or RCMs. SDS provides a way to examine how the climate of a specific location is expected to change by using data from the coarsely resolved GCM and effectively “shrinking” it down to that individual site. Currently GCMs project an overall global mean temperature increase of 1.4 – 5.8ºC by 2100 relative to 1990 (IPCC, 2001). However, annual Arctic temperatures are increasing more rapidly than elsewhere, as much as five to ten times faster (Nunatsiaq News, 2004). The SDSM (statistical downscaling model) can produce point site future climate data that would be useful in this type of situation. 1. Introduction Mean annual global temperature has been warming steadily since the industrial revolution in the 1800s. One important question facing scientists today is what effects this warming will generate for the planet. Projecting expected temperatures for the future using climate models can give scientists a basis for impacts assessments. Presently the main type of projected climate data available is in the form of coarse scale GCM output. More local results can be achieved for impacts assessments by using downscaling. Increased spatial resolution picks up more precise details of specifically where and how climate variables are projected to change. There are many different approaches to downscaling. For example, regional climate modelling is a way to downscale GCM data from a scale of 100s of kilometres to a scale of 10s of kilometres. This is a dynamical method of downscaling. This paper will look at two other ways to perform downscaling: a statistical downscaling technique (to a point site scale) and a minimum spatial average technique. </p><p>2. Global Climate Model Review To date there are only two GCMs (three emission scenarios) available for use with the SDSM. One is the first version of the Canadian Centre for Climate Modelling and Analysis’ (CCCma) Coupled Global Climate Model, the CGCM1 (with the ga1 emission scenario storyline). The CGCM1 has a resolution of 3.75º (lat) x 3.75º (long), giving a grid cell area of 74,000 km2. This model uses the somewhat outdated IS92a1 emission scenario – a middle of the range scenario. The IS92a scenario assumes the population rises to 11.3 billion by 2100, economic growth averages 2.3% year -1 between 1990 and 2100, and a mix of conventional and renewable energy sources are used. The emission scenario available with the CGCM1 for use with the SDSM is the ga1, or GHG+A1. Data from ensemble member one (of three) is offered for the time period 1961-2100 using the IPCC IS92a forcing scenario; the change in greenhouse gases forcing corresponds to that observed from 1900 to 1990 and increases at a rate 1% per year thereafter until year 2100. The direct effect of sulphate aerosols (A) is also included by increasing the surface albedo. The CGCM2 (the second version of the CCCma model) has been slated for release this fall as the CGCM1 is rather dated. The other GCM is the third version of the Hadley Centre coupled atmosphere-ocean general circulation model, the HadCM3 (with both the A21 and B21 emission scenario storylines). The HadCM3 has a resolution of 2.5º (lat) x 3.75º (long), giving a grid cell area of 50,000 km2. The Special Report on Emission Scenarios (SRES2) A2 emission scenario this model uses is one of two emission scenarios available for this model. The A2 scenario assumes that this is a very diverse world; the underlying theme is self-reliance and preservation of local identities. This scenario presumes continuously increasing population, economic development is primarily regionally oriented, and technological change more fragmented and slower than other storylines. </p><p>1 The IS92 emission scenarios were created as part of the 1992 Supplement to the Intergovernmental Panel on Climate Change’s (IPCC) first report in 1990. Six scenarios were proposed – IS92a-f. 2 SRES are the next generation of scenarios updated (from the IS92 scenarios) with new information about global climate. The CGCM2 also uses SRES. The forcing changes implied by the CO2 concentrations were used to scale the equivalent CO2 values to be consistent with the 1990 value in the IS92a simulation. All IS92a or SRES scenario storylines are assumed to be equally probable. The third and final set of GCM data for use with the SDSM is the HadCM3 B2. Here the model uses the SRES B2 emission scenario. The B2 scenario assumes that this is a world in which the emphasis is on local solutions to economic, social and environmental sustainability. It also presumes continuously increasing global population, but at a rate lower than A2, intermediate levels of economic development, and less rapid and more diverse technological change than in the B1 and A1 storylines.</p><p>3. Location Five sites were used in this study: Fort Smith, Fort Simpson, Yellowknife, Norman Wells, and Inuvik, all of the Northwest Territories (NWT). </p><p>4. Data Daily maximum temperature (maxT) and daily minimum temperature (minT) were used in this study. 4.1 Observed Data Sets The observed temperature data was extracted from the National Archive System (NAS). The data was quality controlled by NAS before its use. A 30-year baseline period of 1961-1990 was used as recommended by the IPCC. This baseline period is suggested because the 1971- 2000 period may show beginnings of climate change already. A prior time period is needed to obtain unbiased results. Although the 1980s was the warmest decade on record, the more recent observations are improved from the 1951-1980 time period, which would be the other baseline period of choice. 4.2 Observed Predictor Sets The National Centre for Environmental Protection (NCEP) Re-analysis observed predictor data sets were re-gridded to correspond to their respective GCMs and provided by the CCIS project. 4.3 Forecast Predictor Sets The GCM projected outputs over the NWT were prepared by the CCIS project. Available predictors range from basic variables such as mean sea level pressure and mean temperature at 2m to calculated variables such as specific humidity at 850hPa. The three time-slices available with the GCM future predictor data sets are the 2020s (2010-2039), the 2050s (2040-2069), and the 2080s (2070-2099). Each time-slice represents a 30-year mean. The predictors are available from 1961-2000, for the current time period, as well as for three future time periods of the 2020s, the 2050s, and the 2080s for the CGCM1 and for 1961-2099 for the HadCM3. Note that the HadCM3 data sets needed to be manually divided into the three 30-year periods to match the CGCM1 data. This data is normalized with respect to 1961-1990. To obtain the predictor data available from the CCIS project (http://www.cics.uvic.ca/scenarios/index.cgi) only an email address is needed to register. </p><p>5. Methodology The methodology is described in detail in Using SDSM Version 3.1 – A decision support tool for the assessment of regional climate change impacts by C.W. Dawson and R.L. Wilby, which can be downloaded from https://co-public.lboro.ac.uk/cocwd/SDSM/index.html. This is also where the user will download the software. What follows is a summary of the SDSM procedure. 5.1 Overview Statistical downscaling itself is based on the view that the regional climate is conditioned by the large scale climatic state and regional/local physiographic features (topography, land use, land-sea distribution, and land cover). It develops quantitative relationships between predictors (large scale – GCM data) and predictands (local – observed data sets). The SDSM is a regression model type – a means of representing linear or non-linear relationships between predictands and the large scale atmospheric forcing – combined with a weather generator. The SDSM facilitates the development of daily surface weather variables by taking the needed data (predictors) from the larger GCM grid cell and effectively “shrinking” it to provide site-specific information. This can be done for both current and future climate forcing. 5.2 Quality Control Before the downscaling process begins, a quality control check can be done on the predictand. This will report the mean, maximum, minimum, etc. of the data file you will be using so you can compare to the values in your file. Transformations of either the predictors and/or predictand may also be done here (usually done after at least one trial with the SDSM). 5.3 Screen Variables The first and central step of the SDSM is to choose your predictors. The screen variables facility assists in identifying empirical relationships between the large scale predictors and the local scale predictands. Three tests can be done to analyze the predictor’s relationships with the predictand: explained variance, correlation matrix, and scatter plot. By setting the significance level you choose how important the relationship needs to be (default is 0.05, or 5%). When the explained variance is calculated any blank spaces mean predictor/ predictand relationship wasn’t significant enough to report the value. Once acceptable predictors are chosen, the model can be calibrated. 5.4 Calibration Using the selected predictors, the predictand, and linear regression equations, downscaling models are created. The percentage of explained variance (R2) and the standard error are reported. When these values are within acceptable limits the model is calibrated. In this step only the first half of the data is used (January 1, 1961 to December 31, 1975) so the remaining data can be used to validate the model with the Weather Generator. 5.5 Weather Generator Using the NCEP predictors, ensembles (size can be between 20 and 100 members) of daily weather series are synthesized. The user should specify the period (here use the second half of the observations – January 1, 1976 to December 31, 1990) of the data set to be synthesized. This step verifies the calibration of the model. 5.6 Data Analysis The user can analyze both the observed data and SDSM derived scenarios with the Analyze Data facility. All that is required is an appropriate name for the output file. Whichever statistics you need produced must be specified from the sub-screen. A visual analysis can also be done using the Compare Results facility. A monthly bar or line graph is produced by the SDSM, according to the choice of the user, for the chosen files created with the Analyze Data facility. Two data files may be compared at a time. This can visually confirm the performance of the SDSM with the user’s chosen predictors. If the SDSM output is following the annual temperature cycle well, you can accept that the SDSM is performing well enough to use for downscaling. 5.7 Generate Scenario Lastly, ensembles of daily weather series are synthesized using the GCM predictors in the same way as the Weather Generator, but now with the climate model predictors rather than the observed predictors. Before you undertake this step you must be sure to set the year length correctly. If the length is incorrect, the results output by the SDSM will be completely invalid. As noted on the CCIS website, as well as in the User’s Manual, the year length should be set to 366 when using the observed data sets (from the quality control step to the end of the analysis and comparison of the Weather Generator results). When using the CGCM1 data for generating your scenario set the year length to 365 and the HadCM3 uses 360 day year length. The results can be analyzed with the GCM predictors used following the Data Analysis steps from above as well as creating graphs in Excel for comparison of the current climate with the three future time slices all on one graph. The expected temperature changes can easily be determined from these results. </p><p>6. Other Methods There are many different methods to consider when downscaling. You can use weather generators like the LARS-WG 3, weather classification schemes, and so on (please see the report Guidelines for Use of Climate Scenarios Developed from Statistical Downscaling Methods by R.L. Wilby et al. for more information). I have done an eight surrounding neighbors method (referred to through out as the nine cell method) to see how the SDSM projections of future climate compare to the GCM outputs that are available. The GCM outputs required to use with the nine cell method can be retrieved from the CCIS website. Here the user will need a password for use of the GCM data. Since grid cell size changes with latitude (narrower towards the poles) an areal weighting for each cell was used with the cosine of the latitude (of the center of each of the nine grid cells). Recall the grid size differences between Canadian and Hadley models. For the nine cell method, the Hadley model covers less area. Once an “average” value for the areal weighting is found the user can then compare the nine cell results with the SDSM. Even though temperature is quite constant, the large area the nine cell method covers doesn’t represent a single point as well as the SDSM. </p><p>7. Results Downscaled SDSM results for maxT and minT at the five sites in the NWT for the 2020s, 2050s, and 2080s are shown in Figures 1-10. Based on comparison with the NCEP output we have confidence that SDSM can be applied to the GCM predictors. The predictors chosen for this study were: p500=500hPa geopotential height; shum=near surface specific humidity; temp=mean temp at 2m; p__u=surface zonal velocity; p__v=surface meridional velocity; p__z=surface vorticity; s850=specific humidity at 850 hPa. The first two trials predictors were picked based on the screen variables facility results that gave the best correlations. The third trial predictors were based on the G.S. Lines, M. Pancura, and C. Lander paper entitled “Variability and Extremes in Statistically Downscaled Climate Change Parameters in Atlantic Canada”. From the different set of predictors chosen for Atlantic Canada as in the Lines paper you can see there is a difference in the predictors needed to represent the northern areas, and probably all other regions of the world. Three sets of predictors and the three model/emission scenarios were used for each site giving nine SDSM runs per location. </p><p>3 LARS-WG = Long Ashton Research Station – Weather Generator Looking at Figures 1-10, you can see that the changes projected by the SDSM are very similar to the changes from the 9 cell (even thought the current temps are quite different). These results are consistent with Chris Burns’ report showing more warming in the north of the Mackenzie Basin than the south. The inconsistent plotting of the SDSM (3 different points/GCM) is due to the different predictors used for each run. You can see it does make a difference what predictors are chosen. The HadCM3 fit with the SDSM results quite well. The CGCM1 performed poorly due to outdated data, but in both cases you can see the warming trend for the future is present. The SDSM appeared to be an effective and useful technique to relate the expected temperature changes at a local level. Variations between the modelled and observed are mainly attributed to the CGCM1 model. </p><p>8. Future Work Precipitation was also looked at to try and expand the capabilities of the SDSM. However, the results weren’t promising. The precipitation issue being resolved would be another step to try. The variance inflation and bias correction were manipulated (advanced settings) as recommended by the manual but this didn’t seem to give better results – different but not better. Maybe trying different predictors for the precipitation variable would work. Also, precipitation is highly variable so may need a more complex approach to modelling it or transformation of data. Excluded predictors which are not important in today’s climate may be relevant in future climates. For example, moisture may play more of a key role in the future because warmer air can hold more water vapor than cooler air. Trying to find a way to include this in the predictor choice of the SDSM may be key to getting realistic results from the model for future temperatures. Other things to try may include: 1. Mean temperature, and other variables 2. Examine precipitation in more detail 3. More variations of predictors 4. Different GCMs as they become available; even better if could use more than one GCM per trial 5. POT = peak over threshold; could see how many days above 30C, etc 6. Test other variables such as sunshine hours 7. Explore other methodologies and to other areas of Canada </p><p>9. Conclusions Even though the SDSM can bring the future temperatures to a much smaller scale, the user still can’t assume that because of increased resolution that the results are more accurate. For a more accurate picture, multiple GCM outputs are needed. Also, more confidence would come with more GCM data available. </p><p>10. Acknowledgements Barrow, Elaine (Canadian Institute for Climate Studies, c/o Environment Canada): preparation of the NCEP, CGCM1, and HadCM3 predictor data sets Bonsal, Barrie (National Hydrology Research Centre): input on results Dawson, C.W. (Loughborough University) and R.L. Wilby (Environment Agency of England and Wales): creation of the SDSM Kochtubajda, Bob (Environment Canada): supervision of the project Lapalme, Monique (Environment Canada): helping gather and organize the observed data sets and creation of the NWT map NAS for the observed data</p><p>11. References CCIS website: http://www.cics.uvic.ca/scenarios/index.cgi)</p><p>Climate Change Scenarios for Mackenzie River Valley by C.R. Burns, E. Barrow, and B. Bonsal (9 cell method)</p><p>Guidelines for Use of Climate Scenarios Developed from Statistical Downscaling Methods by R.L. Wilby et al.: http://ipcc-ddc.cru.uea.ac.uk/guidelines/guidelines_home.html</p><p>Using SDSM Version 3.1 – A decision support tool for the assessment of regional climate change impacts by C.W. Dawson and R.L. Wilby: https://co- public.lboro.ac.uk/cocwd/SDSM/index.html</p><p>Variability and Extremes in Statistically Downscaled Climate Change Parameters in Atlantic Canada by G.S. Lines, M. Pancura, and C. Lander Expected Annual Changes in Maximum Temperature for Fort Smith 8.5 8 7.5 7 6.5 ) C ( 6 e r u t 5.5 a r e</p><p> p 5 m e T</p><p>4.5 n i</p><p> e</p><p> g 4 n a</p><p> h 3.5 C</p><p>M 3 S D</p><p>S 2.5 2 1.5 1 0.5 0 0 0.5 1 1.5 2 2.5 3 3.5 4 4.5 5 5.5 6 6.5 7 7.5 8 8.5 GCM Change in Temperature (C)</p><p>CGCM1 ga1 2020s HadCM3 A21 2020s HadCM3 B21 2020s CGCM1 ga1 2050s HadCM3 A21 2050s HadCM3 B21 2050s CGCM1 ga1 2080s HadCM3 A21 2080s HadCM3 B21 2080s Figure 1: Projected change in maximum temperature for the 2020s, 2050s, and 2080s for each of the three models/emission scenarios at Fort Smith. Expected Annual Changes in Minimum Temperature for Fort Smith</p><p>8.5 8</p><p>7.5 7</p><p>6.5 ) C ( 6 e r u t</p><p> a 5.5 r e</p><p> p 5 m e T 4.5 n i</p><p> e</p><p> g 4 n a</p><p> h 3.5 C</p><p>M 3 S D</p><p>S 2.5 2</p><p>1.5 1</p><p>0.5 0 0 0.5 1 1.5 2 2.5 3 3.5 4 4.5 5 5.5 6 6.5 7 7.5 8 8.5 GCM Change in Temperature (C)</p><p>CGCM1 ga1 2020s HadCM3 A21 2020s HadCM3 B21 2020s CGCM1 ga1 2050s HadCM3 A21 2050s HadCM3 B21 2050s CGCM1 ga1 2080s HadCM3 A21 2080s HadCM3 B21 2080s Figure 2: Projected change in minimum temperature for the 2020s, 2050s, and 2080s for each of the three models/emission scenarios at Fort Smith. Expected Annual Changes in Maximum Temperature for Fort Simpson 8.5</p><p>8</p><p>7.5</p><p>7</p><p>6.5</p><p>6</p><p>5.5 )</p><p>C 5 (</p><p> e r</p><p> u 4.5 t a r</p><p> e 4 p m e</p><p>T 3.5</p><p> n i</p><p> e 3 g n a</p><p> h 2.5 C</p><p>M 2 S D</p><p>S 1.5</p><p>1</p><p>0.5</p><p>0 0 0.5 1 1.5 2 2.5 3 3.5 4 4.5 5 5.5 6 6.5 7 7.5 8 8.5 GCM Change in Temperature (C) CGCM1 ga1 2020s HadCM3 A21 2020s HadCM3 B21 2020s CGCM1 ga1 2050s HadCM3 A21 2050s HadCM3 B21 2050s CGCM1 ga1 2080s HadCM3 A21 2080s HadCM3 B21 2080s Figure 3: Projected change in maximum temperature for the 2020s, 2050s, and 2080s for each of the three models/emission scenarios at Fort Simpson. Expected Annual Changes in Minimum Temperature for Fort Simpson 8.5</p><p>8</p><p>7.5</p><p>7</p><p>6.5</p><p>6</p><p>5.5 )</p><p>C 5 (</p><p> e r</p><p> u 4.5 t a r</p><p> e 4 p m e</p><p>T 3.5</p><p> n i</p><p> e 3 g n a</p><p> h 2.5 C</p><p>M 2 S D</p><p>S 1.5</p><p>1</p><p>0.5</p><p>0 0 0.5 1 1.5 2 2.5 3 3.5 4 4.5 5 5.5 6 6.5 7 7.5 8 8.5 GCM Change in Temperature (C) CGCM1 ga1 2020s HadCM3 A21 2020s HadCM3 B21 2020s CGCM1 ga1 2050s HadCM3 A21 2050s HadCM3 B21 2050s CGCM1 ga1 2080s HadCM3 A21 2080s HadCM3 B21 2080s Figure 4: Projected change in minimum temperature for the 2020s, 2050s, and 2080s for each of the three models/emission scenarios at Fort Simpson. Expected Annual Changes in Maximum Temperature for Yellowknife 8.5 8</p><p>7.5 7</p><p>6.5 ) C ( 6 e r u t</p><p> a 5.5 r e p 5 m e T 4.5 n i</p><p> s</p><p> e 4 g n a</p><p> h 3.5 C</p><p>M 3 S D</p><p>S 2.5 2</p><p>1.5 1</p><p>0.5 0 0 0.5 1 1.5 2 2.5 3 3.5 4 4.5 5 5.5 6 6.5 7 7.5 8 8.5 GCM Changes in Temperature (C) CGCM1 ga12020s HadCM3 A21 2020s HadCM3 B21 2020s CGCM1 ga1 2050s HadCM3 A21 2050s HadCM3 B21 2050s CGCM1 ga1 2080s HadCM3 A21 2080s HadCM3 B21 2080s Figure 5: Projected change in maximum temperature for the 2020s, 2050s, and 2080s for each of the three models/emission scenarios at Yellowknife. Expected Annual Changes in Minimum Temperature for Yellowknife 8.5</p><p>8 7.5</p><p>7</p><p>6.5 ) C ( 6 e r u t</p><p> a 5.5 r e p 5 m e T 4.5 n i</p><p> s</p><p> e 4 g n a</p><p> h 3.5 C</p><p>M 3 S D</p><p>S 2.5</p><p>2</p><p>1.5 1</p><p>0.5</p><p>0 0 0.5 1 1.5 2 2.5 3 3.5 4 4.5 5 5.5 6 6.5 7 7.5 8 8.5 GCM Changes in Temperature (C) CGCM1 ga12020s HadCM3 A21 2020s HadCM3 B21 2020s CGCM1 ga1 2050s HadCM3 A21 2050s HadCM3 B21 2050s CGCM1 ga1 2080s HadCM3 A21 2080s HadCM3 B21 2080s Figure 6: Projected change in minimum temperature for the 2020s, 2050s, and 2080s for each of the three models/emission scenarios at Yellowknife. Expected Annual Changes in Maximum Temperature for Norman Wells 8.5 8 7.5 7 6.5 ) C ( 6 e r u t</p><p> a 5.5 r e</p><p> p 5 m e T 4.5 n i</p><p> e</p><p> g 4 n a</p><p> h 3.5 C</p><p>M 3 S D</p><p>S 2.5 2 1.5 1 0.5 0 0 0.5 1 1.5 2 2.5 3 3.5 4 4.5 5 5.5 6 6.5 7 7.5 8 8.5 GCM Change in Temperature (C)</p><p>CGCM1 ga1 2020s HadCM3 A21 2020s HadCM3 B21 2020s CGCM1 ga1 2050s HadCM3 A21 2050s HadCM3 B21 2050s CGCM1 ga1 2080s HadCM3 A21 2080s HadCM3 B21 2080s Figure 7: Projected change in maximum temperature for the 2020s, 2050s, and 2080s for each of the three models/emission scenarios at Norman Wells. Expected Annual Changes in Minimum Temperature for Norman Wells 8.5</p><p>8 7.5 7 6.5 ) C (</p><p>6 e r u t 5.5 a r e</p><p> p 5 m e T 4.5 n i</p><p> e</p><p> g 4 n a</p><p> h 3.5 C</p><p>M 3 S D</p><p>S 2.5 2 1.5 1</p><p>0.5 0 0 0.5 1 1.5 2 2.5 3 3.5 4 4.5 5 5.5 6 6.5 7 7.5 8 8.5 GCM Change in Temperature (C) CGCM1 ga1 2020s HadCM3 A21 2020s HadCM3 B21 2020s CGCM1 ga1 2050s HadCM3 A21 2050s HadCM3 B21 2050s CGCM1 ga1 2080s HadCM3 A21 2080s HadCM3 B21 2080s Figure 8: Projected change in minimum temperature for the 2020s, 2050s, and 2080s for each of the three models/emission scenarios at Norman Wells. Expected Annual Changes in Maximum Temperature for Inuvik 8.5</p><p>8</p><p>7.5 7</p><p>6.5</p><p>) 6 C (</p><p> e 5.5 r u t a</p><p> r 5 e p</p><p> m 4.5 e T</p><p> n 4 i</p><p> e</p><p> g 3.5 n a h</p><p>C 3</p><p>M</p><p>S 2.5 D S 2</p><p>1.5 1</p><p>0.5</p><p>0 0 0.5 1 1.5 2 2.5 3 3.5 4 4.5 5 5.5 6 6.5 7 7.5 8 8.5 GCM Change in Temperature (C)</p><p>CGCM1 ga1 2020s HadCM3 A21 2020s HadCM3 B21 2020s CGCM1 ga1 2050s HadCM3 A21 2050s HadCM3 B21 2050s CGCM1 ga1 2080s HadCM3 A21 2080s HadCM3 B21 2080s Figure 9: Projected change in maximum temperature for the 2020s, 2050s, and 2080s for each of the three models/emission scenarios at Inuvik. Expected Annual Changes in Minimum Temperature for Inuvik 8.5</p><p>8</p><p>7.5</p><p>7</p><p>6.5</p><p>6 ) C ( 5.5 e r u t a</p><p> r 5 e p</p><p> m 4.5 e T</p><p> n 4 i</p><p> e</p><p> g 3.5 n a h</p><p>C 3</p><p>M</p><p>S 2.5 D S 2</p><p>1.5</p><p>1</p><p>0.5</p><p>0 0 0.5 1 1.5 2 2.5 3 3.5 4 4.5 5 5.5 6 6.5 7 7.5 8 8.5 GCM Change in Temperature (C) CGCM1 ga1 2020s HadCM3 A21 2020s HadCM3 B21 2020s CGCM1 ga1 2050s HadCM3 A21 2050s HadCM3 B21 2050s CGCM1 ga1 2080s HadCM3 A21 2080s HadCM3 B21 2080s Figure 10: Projected change in minimum temperature for the 2020s, 2050s, and 2080s for each of the three models/emission scenarios at Inuvik. </p>
Details
-
File Typepdf
-
Upload Time-
-
Content LanguagesEnglish
-
Upload UserAnonymous/Not logged-in
-
File Pages17 Page
-
File Size-