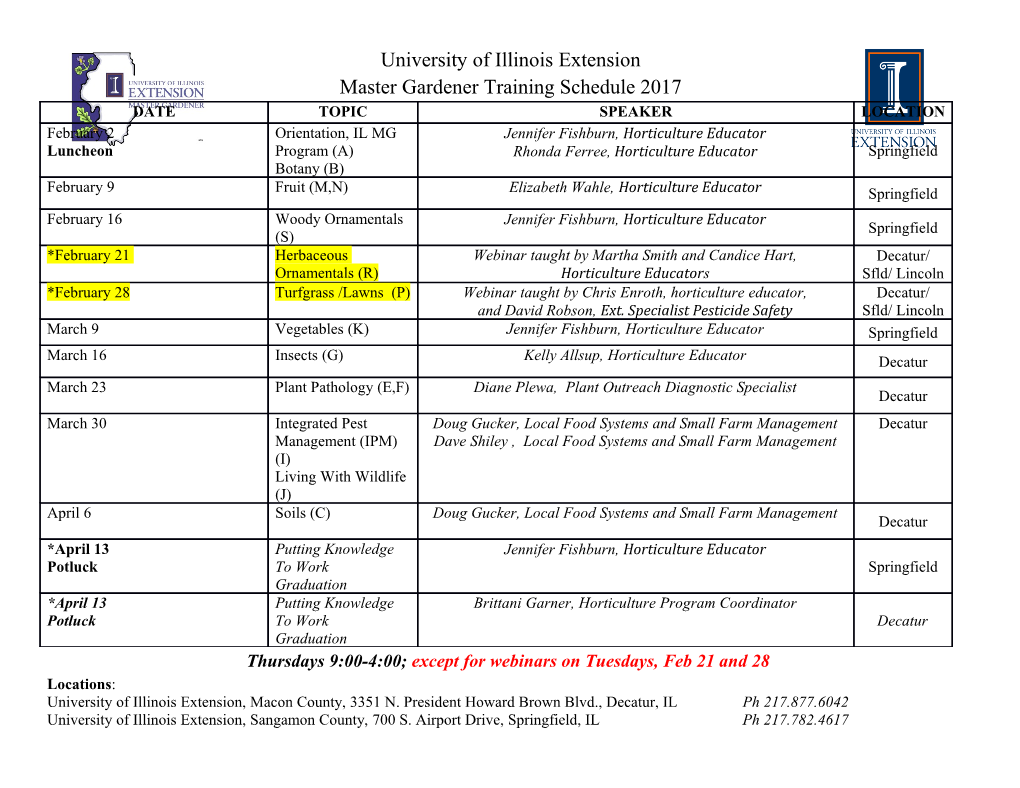
<p>Section Analysis of Algorithm with Big Oh Preview of section I. Code demo Binary Search and run CompareSearches.java with Sequential Search II. As a section, count the instructions together III. In teams of two, answer questions 1..4 IV. Go over answers</p><p>I. Write method public int binarySearch(Integer[], int n, Integer search) to return the integer index of search or -1 of search is not in the array. Program to run with the method written as a method stub is located here. Run with n = 10,000 50,000 150,000. http://www.cs.arizona.edu/~mercer/Sections/CompareSearches.java</p><p>II. Count the instructions. The answer should be a polynomial (several answers are possible depending on how things are counted). </p><p> double[] data = { 76.0, 91.0, 100.0, 62.0, 89.0 };</p><p> int n = data.length;</p><p> int indexOfSmallest = 0;</p><p> for (int left = 0; left < n - 1; left++) {</p><p> indexOfSmallest = left;</p><p> for (int index = left + 1; index < n; index++) { if (data[index] < data[indexOfSmallest]) indexOfSmallest = index; }</p><p> double temp = data[left]; data[left] = data[indexOfSmallest]; data[indexOfSmallest] = temp; }</p><p>III. In teams of two, answers questions 1..4</p><p>1. Sort these functions by order of growth from highest to lowest. Write 1 above the fastest growing function, 2 above the second fastest growing function, and 8 above the slowest growing function. (Note: log24 = 2, log28 = 3 and log216 = 4)</p><p>2 n n 3 100*n n 1000 2 10 n 2*n log2n</p><p>2. Which term dominates this function when n gets really big, n2, 10n, or 100? ______</p><p> n2 + 10n + 100</p><p>3. When n=500 in the function above, what percentage of the function is the term 100? _____ Before answering question 4, here is a review of order of magnitudes in increasing growth rates: O(1) O(log n) O(n) O(n log n) O(n2) O(n3) O(2n)</p><p>… and some example big-O run times:</p><p>O(1) O(log n) O(n) int sum = 0; for(int j = n; j > 0; j = j / 2) for(int j = 1; j <= n; j++) sum += j; sum += j;</p><p>O(n2) product O(n) sum for(int r = 0; r < n; r++) for(int j = 1; j <= n; j++) for(int c = 0; c < n; c++) sum += j; sum += a[r][c]; for(int j = 1; j <= 2*n; j++) sum += j;</p><p>And some big-O style guidelines</p><p> use highest order term (drop lower order terms) Drop coefficients and drop constants Use the tightest upper bound. It could be said that most algorithms run O(2n), a function that grow very fast. But use the tightest upper bound instead.</p><p>4. Determine the runtimes of the following loops expressed in big-O notation a. ______d. ______int sum = 0; for(int j = 0; j < n; j++) int n = 1000000; sum++; for(int k = 0; k < n; k++) sum--; b. ______int sum = 0; e. ______for (int j = 0; j < n; j++) for(int j = 0; j < n; j++) for (int k = 0; k < n; k++) System.out.println(x [ j ]); sum += j * k; c. ______for (int j = 0; j < n; j++) f. ______for (int k = 0; k < n; k++) for(int j = 1; j < n; j = 2 * j) for (int l = 0; l < n; l++) sum += j; sum += j * k * l;</p><p>IV Go over answers</p>
Details
-
File Typepdf
-
Upload Time-
-
Content LanguagesEnglish
-
Upload UserAnonymous/Not logged-in
-
File Pages2 Page
-
File Size-